The AlphaStar neural network beat the StarCraft II professionals with a score of 10−1
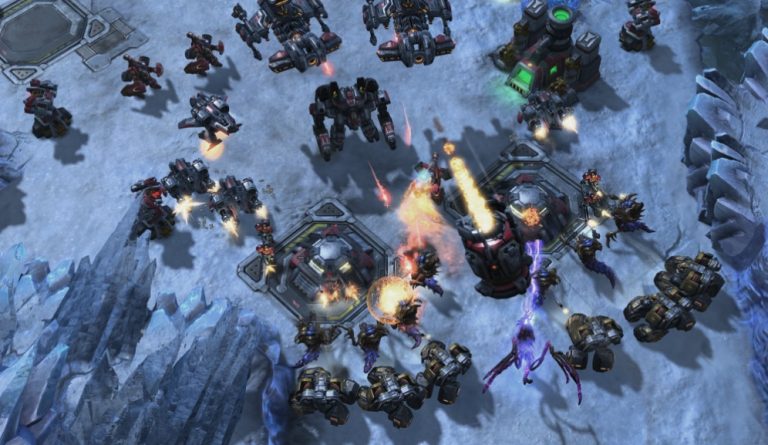
DeepMind, a subsidiary of Alphabet, which is engaged in research in the field of artificial intelligence, announced a new milestone in this grand quest: for the first time, AI beat a man in Starcraft II strategy . In December 2018, the convolutional neural network called AlphaStar spread professional players TLO (Dario Wünsch, Germany) and MaNa (Grzegorz Comints, Poland), winning ten victories. On this event, the company announced yesterday in a live broadcast on YouTube and Twitch.
In both cases, both the people and the program played for the protoss. Although TLO does not specialize in this race, but MaNa had a serious resistance, and then even won one game.
In the popular real-time strategy, players represent one of three races that compete for resources, build structures, and fight on a large map. It is important to note that the speed of the program and its field of view on the battlefield were limited so that AlphaStar would not get an unfair advantage over people (amendment: it appears that they only restricted the area of view in the last match). Actually, according to statistics, the program even performed fewer actions per minute than people: on average, 277 for AlphaStar, 390 for MaNa, 678 for TLO.

The video shows the game from the point of view of an AI agent in the second match against MaNa. The view from the person is also shown, but it was not available for the program.
AlphaStar was trained to play for the protoss in an environment called the AlphaStar League. At first, for three days the neural network was reviewing the recordings of games, then playing with itself, using a technique known as reinforcement training, honing skills.
In December, they first organized a gaming session against TLO, in which five different versions of AlphaStar were tested. On this occasion, TLO complained that he could not adapt to the game of the enemy. The program won with a score of 5−0.
After optimizing the neural network settings, a match against MaNa was organized a week later. The program again won five games, but MaNa took revenge in the last game against the newest version of the algorithm live, so he has something to be proud of.
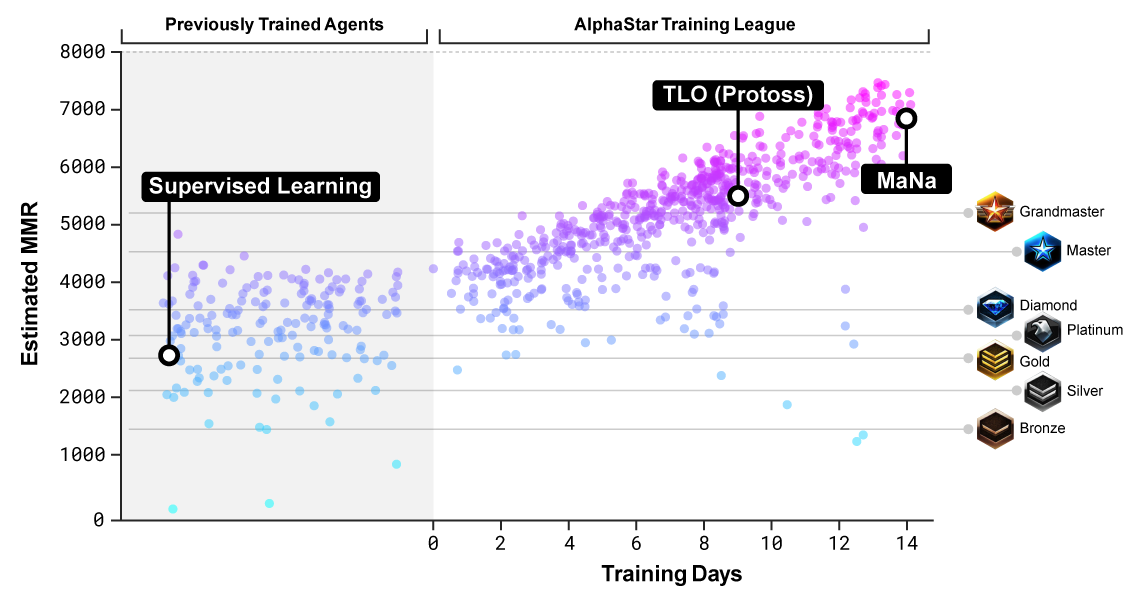
Evaluation of the level of opponents at which the neural network was trained
To understand the principles of strategic planning, AlphaStar had to master a particular mindset. The methods developed for this game can potentially be useful in many practical situations that require a complex strategy: for example, trade or military planning.
Starcraft II is not only an extremely difficult game. It is also a game with incomplete information, where players can not always see the actions of their opponent. It also does not have an optimal strategy. And it takes time for the results of the player’s actions to become clear: this also makes learning difficult. The DeepMind team used a very specialized neural network architecture to solve these problems.
Limited learning on games
DeepMind is known as a software developer who beat the world's best professionals in go and chess. Prior to this, the company developed several algorithms that have learned to play simple Atari games. Video games are a great way to measure progress in artificial intelligence and compare computers with people. However, this is a very narrow area of testing. Like previous programs, AlphaStar performs only one task, albeit incredibly well.
We can say that a weak narrow-purpose AI mastered the skills of strategic planning and tactics of combat operations. Theoretically, these skills can come in handy in the real world. But in practice this is not necessarily the case.
Some experts believe that such highly specialized applications of AI have nothing to do with a strong AI: “Programs that have learned to skillfully play a specific video game or board game on a“ superhuman ”level are completely lost with the slightest change in conditions (changing the background on the screen or changing the position virtual "platform" for beating the "ball"), - says professor of computer science at Portland state University Mitchell Melanie article "Artificial intelligence ran into a barrier ponima tions " . - These are just a few examples that demonstrate the unreliability of the best AI programs, if the situation is slightly different from those in which they were trained. The errors of these systems range from ridiculous and harmless to potentially catastrophic. ”
The professor believes that the race for the commercialization of AI has put tremendous pressure on researchers to create systems that work "quite well" in narrow tasks. But ultimately, the development of reliable AI requires a deeper study of our own abilities and a new understanding of the cognitive mechanisms that we ourselves use:
Our own understanding of the situations we encounter is based on broad, intuitive “common sense” concepts about how the world works, and about the goals, motives and likely behavior of other living beings, especially other people. In addition, our understanding of the world is based on our basic abilities to summarize what we know, to form abstract concepts and to draw analogies - in short, to flexibly adapt our concepts to new situations. For decades, researchers have experimented with teaching AI to intuitive common sense and sustainable human abilities to generalize, but there is little progress in this very difficult matter.
Neural network AlphaStar is able to play only for protoss. The developers have announced plans to train her in the future to play for other races.