The primary task of quantum computers is to enhance artificial intelligence.
- Transfer
The idea of merging quantum computing and machine learning is in its prime. Can she live up to high expectations?

In the early 90's Elizabeth Berman [Elizabeth Behrman], professor of physics at Uichitskom university started working on the merger of quantum physics with artificial intelligence - in particular in the area of the then unpopular neural network technology. Most people thought she was trying to mix oil with water. “It was damn difficult for me to publish,” she recalls. “The journals on neural networks said“ What is this quantum mechanics? ”, And the journals on physics said“ What is this neural network nonsense? ”
Today, a mixture of these two concepts seems the most natural thing in the world. Neural networks and other machine learning systems have become the most sudden technology of the 21st century. They succeed in human activities better than people, and they excel us not only in the tasks in which most of us didn’t shine - for example, in chess or in-depth analysis of data, but also in those tasks for which the brain evolved - for example, facial recognition, translation of languages and determination of the right of way at a four-way intersection. Such systems became possible due to the enormous computer power, so it is not surprising that technology companies began searching for computers not just bigger, but belonging to a completely new class.
After decades of research, quantum computers are almost ready to perform calculations ahead of any other computers on Earth. As their main advantage is usually the expansion of factors of large numbers - an operation that is key to modern encryption systems. True, up to this point there are still at least ten years. But today's rudimentary quantum processors are mysteriously perfect for machine learning needs. They manipulate huge amounts of data in a single pass, seek out elusive patterns that are invisible to classic computers, and do not quench themselves before incomplete or uncertain data. “There is a natural symbiosis between the statistical nature of quantum computing and machine learning,” says Johann Otterbach, a physicist at Rigetti Computing, a company
For that matter, the pendulum has already swung to another maximum. Google, Microsoft, IBM and other tech-giants are pouring funds into quantum machine learning (KMO) and the startup incubator dedicated to this topic, located at the University of Toronto. “Machine learning” is becoming a buzzword, ”says Jacob Biamont , a specialist in quantum physics from the Skolkovo Institute of Science and Technology . "And by mixing it with the concept of" quantum ", you will learn the mega-modular word."
But the concept of "quantum" never means exactly what is expected of it. Although you could decide that a CMO system should be powerful, it suffers from "locked up" syndrome. It works with quantum states, not human-readable.data, and the translation between these two worlds can level all its distinct advantages. It's like an iPhone X, with all of its impressive characteristics, is no faster than an old phone, because the local network works disgustingly. In some special cases, physicists can overcome this bottleneck of input-output, but it is not yet clear whether such cases will appear when solving practical problems with MOs. “We don’t have clear answers yet,” said Scot Aaronson , a computer scientist at the University of Texas at Austin, who is always trying to really look at things in the field of quantum computing. “People are quite cautious about the question of whether these algorithms will give any speed advantage.”
Quantum neurons
The main task of a neural network, be it classical or quantum, is to recognize patterns. It is created in the image of the human brain and is a grid of basic computational units - "neurons". Each of them may be no more difficult than an on / off switch. A neuron monitors the output of many other neurons, as if voting on certain issues, and switches to the “on” position if many neurons vote “for”. Usually neurons are ordered into layers. The first layer accepts input (for example, image pixels), the middle layers create different combinations of input (representing structures such as faces and geometric shapes), and the last layer produces output (a high-level description of what is contained in the picture).

Depth neural networks are trained by adjusting the weights of their connections so as to best transmit signals through several layers to the neurons associated with the necessary generalized concepts.
What is important is that this whole scheme is not worked out in advance, but is adapted in the process of learning by trial and error. For example, we can feed the network images, signed "kitten" or "puppy." For each picture, it assigns a label, checks whether it has succeeded, and if not, corrects neural connections. At first, it works almost randomly, but then improves the results; after, say, 10,000 examples, she begins to understand pets. There can be a billion internal connections in a serious neural network, and all of them need to be adjusted.
On a classic computer, these connections are represented by a fabulous matrix of numbers, and the operation of the network means performing matrix calculations. Usually, these operations with the matrix are given for processing to a special chip - for example, a graphics processor . But no one can handle matrix operations better than a quantum computer. “Processing large matrices and vectors on a quantum computer is exponentially faster,” says Seth Lloyd, a physicist at MIT and the pioneer of quantum computing.
To solve this problem, quantum computers are able to take advantage of the exponential nature of the quantum system. Most of the information capacity of a quantum system is not contained in its individual data units — qubits, quantum analogs of the bits of a classical computer — but in the joint properties of these qubits. Two qubits together have four states: both on, both off, on / off, and off / on. Everyone has a certain weight, or “amplitude”, which can play the role of a neuron. If you add a third qubit, you can imagine eight neurons; the fourth is 16. The capacity of the machine grows exponentially. In fact, neurons are spread throughout the system. When you change the state of four qubits, you process 16 neurons in one fell swoop, and a classic computer would have to process these numbers one by one.
Lloyd estimates that 60 qubits will be enough to encode as much data as mankind produces in a year, and 300 may contain the classic content of the entire Universe. The largest quantum computer currently available, built by IBM, Intel, and Google, has about 50 qubits. And this is only if we assume that each amplitude represents one classic bit. In fact, amplitudes are continuous (and represent complex numbers), and with an accuracy suitable for solving practical problems, each of them can store up to 15 bits, says Aaronson.
But the ability of a quantum computer to store information in a compressed form does not make it faster. You need to be able to use these qubits. In 2008, Lloyd, physicist Aram Harrow of MIT andAvinatan Hassidim , a computer science specialist from Bar-Ilan University in Israel, showedhow you can perform an important algebraic operation to invert the matrix. They broke it into a sequence of logical operations that can be performed on a quantum computer. Their algorithm works for a huge number of MO technologies. And he does not need as many steps as, say, the decomposition of a large number of factors. A computer can quickly perform a classification task before noise — a major limiting factor in modern technology — can ruin everything. “Before you have a completely universal, error-tolerant quantum computer, you may have just a quantum advantage,” said Kristan Temme from the Research Center. Thomas Watson's IBM Company.
Let nature solve the problem
So far, machine learning based on quantum matrix computing has been demonstrated only on computers with four qubits. Much of the experimental success of quantum machine learning uses a different approach, in which a quantum system does not just simulate a network, but is a network. Each qubit is responsible for one neuron. And although there is no reason to talk about exponential growth, such a device can take advantage of other properties of quantum physics.
The largest of these devices, containing about 2000 qubits, was manufactured by D-Wave Systems, located near Vancouver. And this is not exactly what people imagine when thinking about a computer. Instead of receiving some input data, performing a sequence of calculations and showing the output, it works by finding internal consistency. Each of the qubits is a superconducting electrical loop, working like a tiny electromagnet, oriented up, down, or up and down — that is, being in a superposition. Together, qubits are connected by magnetic interaction.
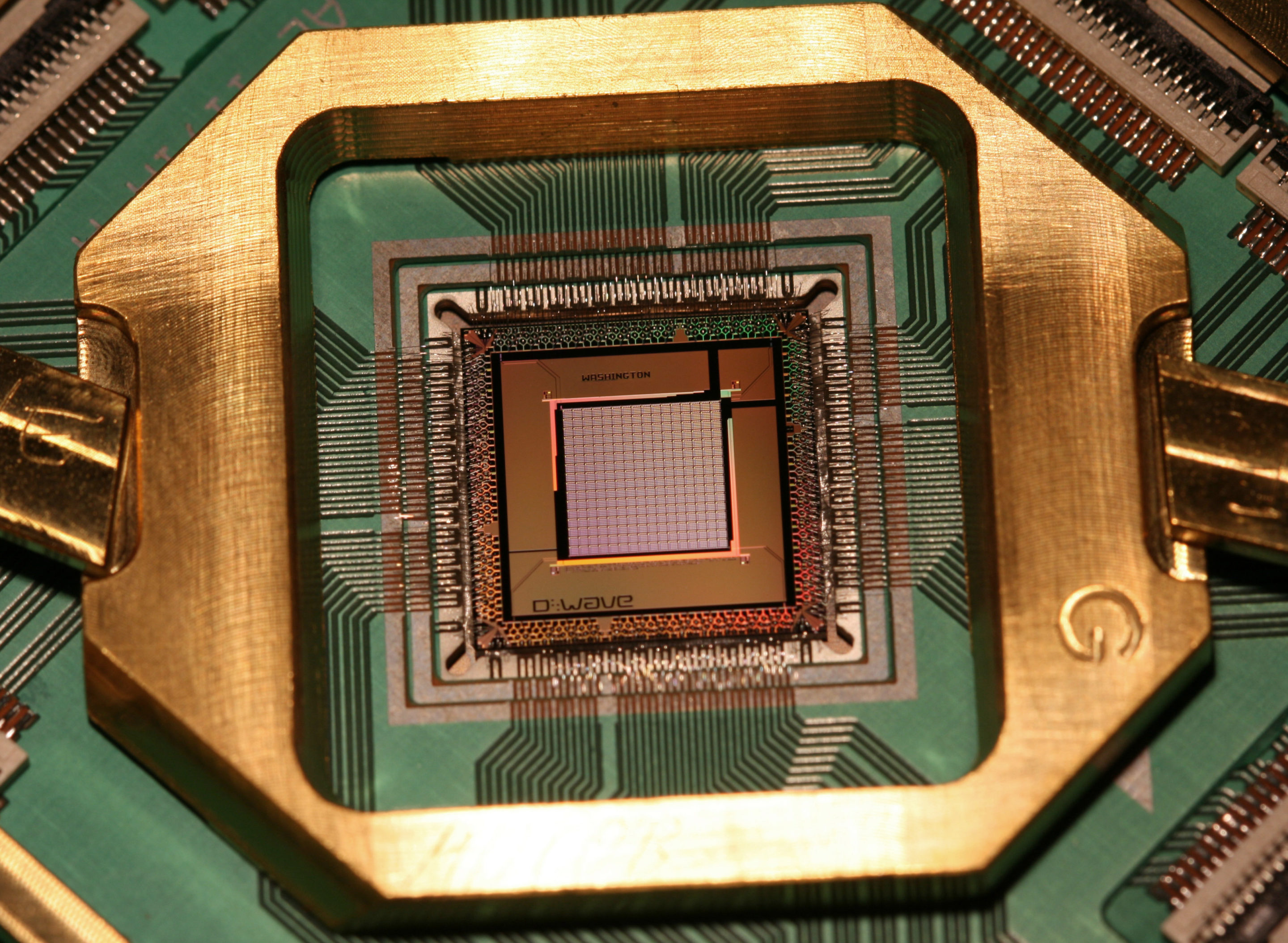
To start this system, you first need to apply a horizontally oriented magnetic field that initializes qubits with the same up and down superposition — the equivalent of a blank sheet. There are a couple of ways to enter data. In some cases, it is possible to fix a layer of qubits in the necessary initial values; more often, input is included in the interaction force. Then you allow qubits to interact with each other. Some try to line up the same way, some - in the opposite direction, and under the influence of a horizontal magnetic field, they switch to their preferred orientation. In this process, they can force other qubits to switch. At first, this happens quite often, because so many qubits are located incorrectly. Over time, they calm down, then you can turn off the horizontal field and lock them in this position. At this point, the qubits lined up in a sequence of “up” and “down” positions, representing the output based on the input.
It is not always obvious what the final layout of the qubits will be, but this is the point. The system, simply behaving naturally, solves the problem over which a classical computer would have fought for a long time. “We don’t need an algorithm,” explains Hidetoshi Nisimori , a physicist at the Tokyo Institute of Technology, who developed the principles of D-Wave machines. - This is a completely different approach from conventional programming. The problem is solved by nature. ”
Switching qubits is due to quantum tunneling, the natural tendency of quantum systems to the optimal configuration, the best possible. It would be possible to build a classic network operating on analog principles that use random jitter instead of tunneling to switch bits, and in some cases it would actually work better. But, interestingly, for problems appearing in the field of machine learning, the quantum network seems to reach the optimum faster.
The car from D-Wave has its drawbacks. It is extremely susceptible to noise, and in the current version it can perform few variations of operations. But machine learning algorithms are noise tolerant by nature. They are useful precisely because they can recognize the meaning in an untidy reality, separating the kittens from the puppies, despite the distractions. “Neural networks are known for their resistance to noise,” said Berman.
In 2009, the team led by Hartmut Niven, a computer scientist from Google, a pioneer of augmented reality (he co-founded the Google Glass project), who switched to the field of quantum information processing, showed how D-Wave's early prototype machine is able to perform the very real machine learning task. They used the car as a single-layer neural network, sorting images into two classes: “car” and “not car” in a library of 20,000 photos taken on the streets. In the car there were only 52 workers qubit, not enough to completely enter the image. Therefore, the Niven team combined the car with a classic computer, analyzed various statistical parameters of the images and calculated how sensitive these values are to the presence of a car in the photo - they were usually not particularly sensitive, but at least different from random. Some combination of these values could reliably determine the presence of a car, it was simply not obvious what kind of combination it was. And the neural network was engaged in the definition of the necessary combination.
Each value team associated qubit. If the qubit was set to 1, he noted the corresponding value as useful; 0 means it is not needed. The magnetic interactions of qubits encoded the requirements of this problem — for example, the need to take into account only the most strongly different quantities in order for the final choice to be the most compact. The resulting system was able to recognize the car.
Last year, a team led by Maria Spiropoulou, a specialist in particle physics from the California Institute of Technology, and Daniel Lidar, a physicist from the University of Southern California, used an algorithm to solve a practical problem in physics: the classification of proton collisions into the Higgs boson and non-boson categories Higgs. By limiting the estimates to collisions that generated photons, they used the basic theory of particles to predict which properties of the photon should indicate a short-term appearance of the Higgs particle — for example, the magnitude of the impulse that exceeds a certain threshold. They examined eight such properties and 28 combinations of them, which together gave 36 candidate signals and allowed the D-Wave chip to find the optimal sample. He identified 16 variables as useful, and three as the best. "Given the small size of the training set,
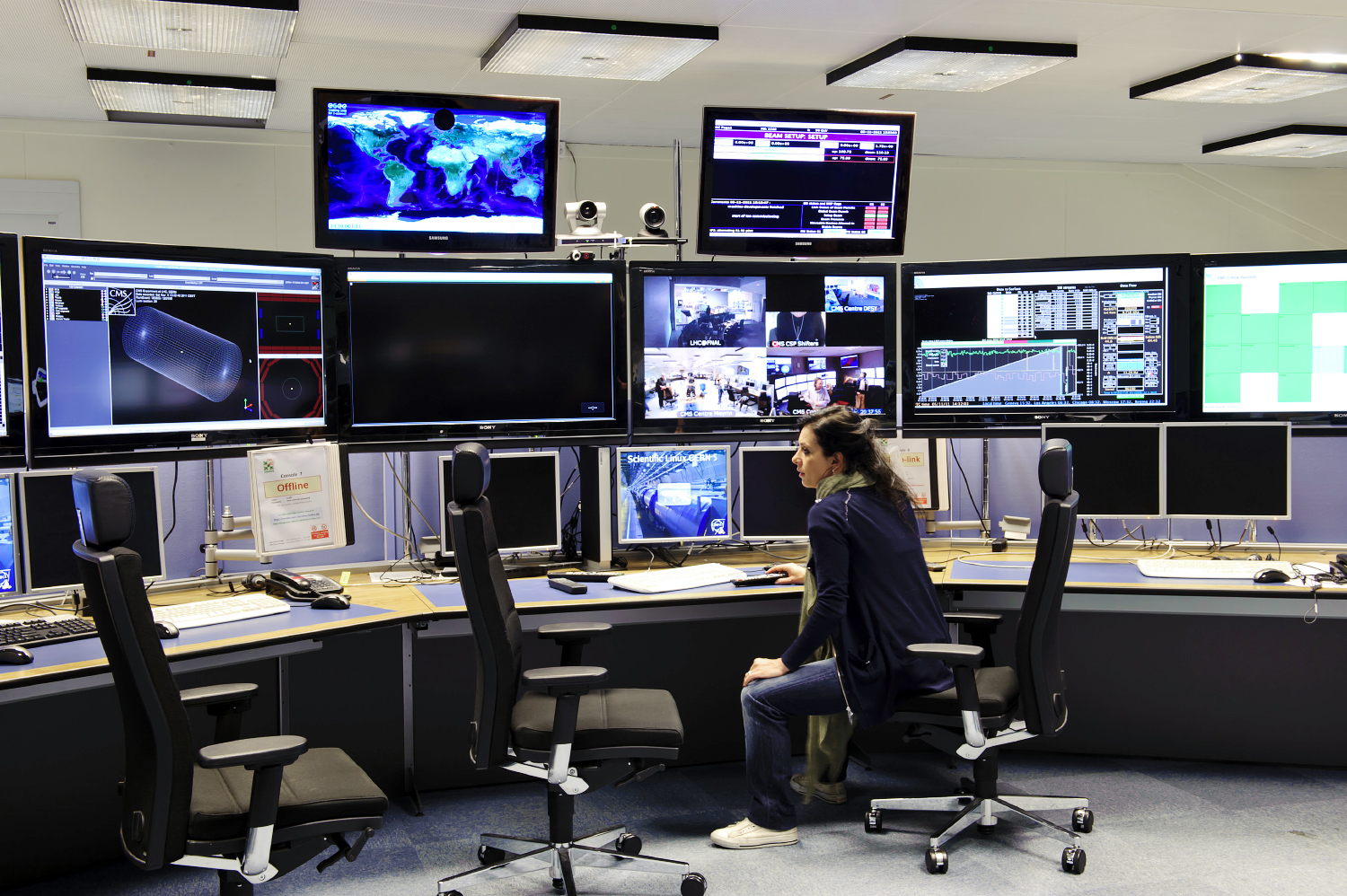
Maria Spiropulu, a physicist at Caltech, used machine learning to find the Higgs bosons.
In December, Rigetti demonstrated how to automatically group objects using a 19-Qbit quantum general-purpose computer. The researchers fed the car a list of cities and distances between them and asked her to sort the cities into two geographic regions. The difficulty of this task is that the distribution of one city depends on the distribution of all the others, so you need to find a solution for the entire system at once.
The company's team, in fact, assigned each city a qubit and noted which group they assigned to it. Through the interaction of qubits (in the Rigetti system, it is not magnetic, but electric), each pair of qubits sought to take opposite values, since in this case their energy was minimized. Obviously, in any system containing more than two qubits, some pairs will have to belong to the same group. Closer located cities more willingly agreed to this, since for them the energy cost of belonging to the same group was lower than in the case of distant cities.
To bring the system to the lowest energy, the Rigetti team chose an approach that was somewhat similar to the D-Wave approach. They initialized qubits with superposition from all possible distributions into groups. They allowed Qbits to interact with each other for a short time, and this inclined them to accept certain values. Then they applied an analogue of the horizontal magnetic field, which allowed the qubits to change the orientation to the opposite, if they had such a tendency that it pushed the system a little towards the energy state with minimal energy. Then they repeated this two-step process — interaction and a coup — until the system minimized energy, distributing cities to two different regions.
Similar classification tasks, although useful, are fairly simple. Real breakthroughs of MO are expected in generative models that not only recognize puppies and kittens, but are able to create new archetypes - animals that have never existed, but are as cute as they are real. They are even able to independently bring out such categories as "kittens" or "puppies", or reconstruct an image that does not have a paw or tail. “These technologies are capable of much and are very useful in the MoD, but very difficult to implement,” said Mohammed Amin , chief scientist at D-Wave. The help of quantum computers would come in handy here.
D-Wave and other research teams accepted this challenge. To train such a model is to adjust the magnetic or electrical interactions of the qubits so that the network can reproduce certain test data. To do this, you need to combine the network with a regular computer. The network is involved in complex tasks — it determines what this set of interactions means in terms of the final network configuration — and the partner computer uses this information to fine-tune the interactions. In one demonstration last year, Alejandro Perdomo-Ortiz, a researcher at NASA's Quantum Artificial Intelligence Laboratory, together with a team, gave the D-Wave an image system of handwritten numbers. She determined that there were a total of ten categories, compared the numbers from 0 to 9, and created her own scribbles in the form of numbers.
Bottlenecks leading to tunnels
This is all good news. And the bad news is that no matter how cool your processor is, if you can't provide it with data to work with. In matrix algebra algorithms, a single operation can process a matrix of 16 numbers, but to load a matrix, it still requires 16 operations. “The issue of state preparation — the placement of classical data in a quantum state — is avoided, and I think this is one of the most important parts,” said Maria Schuld, a researcher at the Xanadu quantum computer startup and one of the first scientists who received a degree in KMO. Physically distributed MO systems face parallel difficulties - how to enter a task into a network of qubits and make qubits interact as needed.
Once you have been able to enter data, you need to store them in such a way that the quantum system can interact with them without collapsing the current calculations. Lloyd and colleagues suggested quantum RAM using photons, but no one has yet an analog device for superconducting qubits or trapped ions — technologies used in leading quantum computers. “This is another huge technical problem besides the problem of building a quantum computer itself,” said Aaronson. - When communicating with experimenters, I get the impression that they are scared. They have no idea how to approach the creation of this system. ”
And finally, how to display the data? It means to measure the quantum state of the machine, but the measurement not only returns one number at a time, chosen randomly, it also destroys the entire state of the computer, erasing the rest of the data before you have a chance to reclaim them. We'll have to run the algorithm again and again to remove all the information.
But all is not lost. For some types of problems, quantum interference can be used. You can control the course of operations so that the wrong answers are mutually destroyed, and the correct answers reinforce themselves; thus, when you measure a quantum state, you will be returned not just a random value, but the desired answer. But only a few algorithms, for example, brute-force search, can use the interference, and the acceleration is usually small.
In some cases, researchers have found workarounds for data input and output. In 2015, Lloyd, Silvano Garnerone from the University of Waterloo in Canada and Paolo Zanardi from the University of Southern California showed that it is not necessary to enter or store the entire data set in certain types of statistical analysis. Similarly, it is not necessary to read all the data when several key values are sufficient. For example, technocompanies use MOs to issue TV recommendations for viewing or products to buy based on a huge matrix of human habits. “If you make such a system for Netflix or Amazon, you do not need a matrix recorded somewhere, but recommendations for users,” says Aaronson.
All this raises the question: if a quantum machine demonstrates its abilities in special cases, maybe the classical machine can also show itself well in these cases? This is the main unresolved issue in this area. After all, ordinary computers can do a lot too. The usual selection method for processing large data sets — random sampling — is actually very similar in spirit to a quantum computer, which, whatever happens inside it, ultimately produces a random result. Schuld notes: “I implemented a lot of algorithms to which I reacted like:“ This is so great, this is acceleration, ”and then, just for fun, wrote sampling technology for a classic computer, and realized that the same can be achieved with sampling assistance. "
None of the successes achieved by KMO to date is complete without a trick. Take the car D-Wave. When classifying images of cars and Higgs particles, it worked no faster than a classic computer. “One of the topics not discussed in our work is quantum acceleration,” said Alex Mott, a computer scientist from the Google DeepMind project, who worked on the team that investigated the Higgs particle. Approaches with matrix algebra, for example, the Harrow-Hassidimi-Lloyd algorithm demonstrate acceleration only in the case of sparse matrices — almost completely filled with zeros. "But no one asks the question - are sparse data generally interesting for machine learning?" Schuld said.
Quantum intelligence
On the other hand, even rare improvements in existing technologies could please the tech company. “The resulting improvements are modest, not exponential, but at least quadratic,” says Nathan Wieb , a researcher in the field of quantum computers from Microsoft Research. "If we take a sufficiently large and fast quantum computer, we could make a revolution in many areas of MO." And in the process of using these systems, computer scientists will probably solve a theoretical riddle - is it really, by definition, faster, and in what way.
Schuld also believes that there is a place on the software side for innovation. MO is not just a bunch of calculations. This is a complex of tasks with its own particular, defined structure. “The algorithms created by people are separated from the things that make the MoD interesting and beautiful,” she said. “Therefore, I started working from the other end and thought: If I already have a quantum computer — small-scale — what model of MO can be implemented on it? Maybe this model has not yet been invented. ” If physicists want to impress experts on MO, they will have to do something more than just create quantum versions of existing models.
Just as many neuroscientists have come to the conclusion that the structure of a person’s thoughts reflects the need for a body, MO systems also materialize. Images, language and most of the data flowing through them come from the real world and reflect its properties. KMO is also materializing - but in a richer world than ours. One of the areas where it will no doubt shine is in the processing of quantum data. If this data represents not the image, but the result of a physical or chemical experiment, the quantum machine will become one of its elements. The input problem disappears, and classic computers are far behind.
As if in a vicious circle situation, the first KMOs can help develop their successors. “One of the ways we can really want to use these systems is to create quantum computers themselves,” said Waibe. “For some error recovery procedures, this is the only approach we have.” Maybe they can even eliminate the mistakes in us. Without addressing the subject of whether the human brain is a quantum computer - and this is a very controversial issue - it still behaves this way sometimes. Human behavior is extremely contextual; our preferences are formed through the choices provided to us and do not obey the logic. In this we are like quantum particles. “The way you ask questions and in what order matters, and that’s typical of quantum datasets,” said Perdomo-Ortiz.
Neural networks and quantum processors have something in common: surprisingly, they generally work. The ability to train a neural network was never obvious, and for decades most people doubted that it would be possible at all. Likewise, it is not obvious that quantum computers can ever be adapted to computing, since the distinguishing features of quantum physics are so well hidden from all of us. And yet both of them work - not always, but more often than we might expect. And considering this, it seems likely that their union will find a place for itself under the sun.