
CampusInsight: From Infrastructure Monitoring to User Experience Analysis
Wireless network quality is already included by default in the concept of service level. And if you want to satisfy the high demands of customers, you need to not only quickly deal with the network problems that have arisen, but also to predict the most massive of them.
How to do it? Only by tracking what is really important in this context is user interaction with the wireless network.
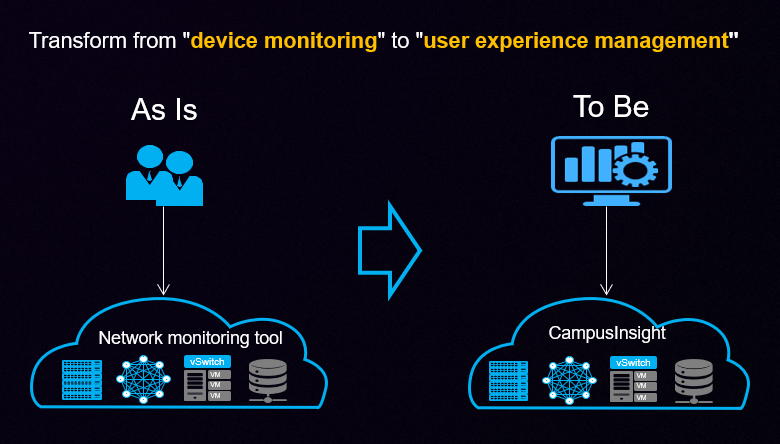
Network loads continue to grow, and this especially affects wireless segments - at least because of the openness of their interface. With the increasing number of devices and data transfer rates, problems multiply at once at several levels. On the physical - many radio signal transmitters affect each other, even if they work in neighboring parts of the frequency spectrum. Logically, a large number of connected devices begin to compete for the right to start transmission at the selected frequency, increasing the delay in packet delivery for each user.
At the same time, the expectations of each client from using the network are growing. A 5-second page loading in the browser, which 20 years ago seemed like the “top of the technology”, will not surprise anyone. Give customers HD video without fading.
The new versions of wireless transmission standards that use the frequency spectrum more efficiently can partially solve the problem. Each subsequent version of Wi-Fi aims to deploy more and more loaded networks. But in a large-scale network, where more than a dozen access points operate, it will not be possible to give everything to the next standard (all the more, the devices work in backward compatibility mode as soon as they meet an old user device). As it doesn’t succeed in continuing to live with old monitoring tools, the network environment is constantly getting complicated.
The classic stamp, which still haunts the administrators of all networks, including wireless, is work exclusively on request. “Alarm” worked - we wake up and understand what went wrong. In the meantime, there is no “alarm”, you can limit yourself to checking the load on the main components - network and user devices.
In accordance with this task, traditional monitoring and maintenance tools work on the principle of strict rules and do not always promptly show existing problems, not to mention some kind of predictive analysis.
The main problem here is the data collection interval. Information about the state of wireless network connections is collected once every minute, and incidents may well occur in the intervals between the collection of readings (a great example is the rare bursts of load that "hang" the network). Not receiving real-time data, it’s quite difficult to understand what became the root cause of the problem. Is this misuse of network coverage? Or, perhaps, external interference that is not related to business in any way (for example, the military unit nearby “poured” it on the air). There is no data where it would be possible to see the gradual degradation of certain characteristics of the network, and therefore localizing the problem is not so simple. IT staff will have to spend extra hours searching for such a “needle in a haystack.”
But end users notice the problem almost immediately. A connection error, a broken video broadcast are excellent markers.
To get an answer to this question, it will be necessary to change not just the tool, but the approach to monitoring organization itself. From "fire" work on requests (in fact, control of the performance and load of a specific iron), we proceed to control user experience and identify situations that could lead to incidents.
This transformation involves the introduction of more complex problem determination algorithms than simple warnings when certain values are reached. In the Huawei CampusInsight network intelligence platform, these algorithms are based on wireless service experience and self-learning techniques.
Huawei CampusInsight is a scalable platform for monitoring wireless networks of various sizes. Built on the basis of microservice architecture. Each service is deployed on several instances, the messages between which are distributed by the corresponding bus. Additional instances can be deployed dynamically, increasing tool throughput.
In fact, CampusInsight collects, analyzes, and displays data in its UI in five steps.

The first and second step is access to data (to devices that provide their generation) and the collection of "readings". Using Google’s GPB streaming telemetry capture and “traditional” Syslog (where possible), Huawei CampusInsight accumulates data in near real time:
To circumvent the limitations of traditional tools that use SNMP to collect data and send fixed structures, CampusInsight was based on a subscription model for the necessary logs and data encoding and decoding algorithms.
The third step is distribution and buffering - i.e. sending raw data to Kafka for distribution to higher-level analysis services.
The fourth step is analysis. Big Data and AI algorithms help you quickly process raw data. As a result, certain problems are identified associated with:
AI algorithms are used to solve some particular problems, for example, to detect interference between channels during wireless transmission.

The fifth and final step is to save the data in a distributed column database Druid for later use.
An analysis of the information collected, taking into account the “baseline” constructed using the same historical data, allows us to identify typical “failure patterns” - identifying KPIs corresponding to problem situations and localizing problems by suggesting ways to solve them. Thus, about 85% of all network problems fall into account of the tool.
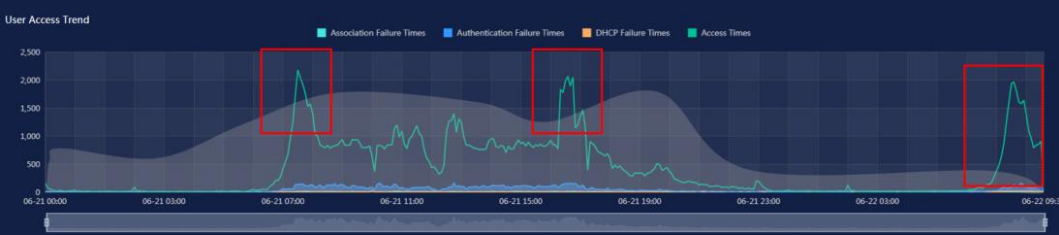
The data is presented to the administrator in a graphical form in accordance with the hierarchy or topology of the space (for example, the layout of the office). You can build “heat maps”, analyze how affected the equipment of certain platforms or manufacturers, etc. It’s easier to understand what exactly caused the problem.

In general, CampusInsight provides quite a few tools to classify problems, compare affected users, examine data about a particular client, and even “play back” events that preceded the incident in order to quickly identify the source. At the same time, the product also supports the new Wi-Fi 6, not to mention its predecessors.
CampusInsight has already been tested in practice, although most of the cases are closed by the NDA. The most revealing open case is the use of a monitoring tool in Huawei’s own wireless network.
The network covers enterprises where about 180 thousand people are employed, of which 80 thousand belong to the R&D division (these are offices in more than 170 countries, where a total of 62 thousand access points are installed).
The implementation of CampusInsight has helped to optimize more than 630 access points, while increasing incident analysis efficiency by 30%.
Below are a couple of specific situations.
The high-level problems observed on a large number of users are often the result of low-level errors. And to identify such problems is not so simple. For example, in one of the offices, many mobile clients immediately experienced difficulties with authentication, despite the correct settings and the absence of problems with the authentication server. Visualization of the data at different levels helped to quickly identify that the switch was the source of the problem and generated too many errors. And to correct the situation, it was only necessary to replace a piece of cable. Localization and correction of the problem took 90 minutes.
Collecting data along the path of a specific client within a distributed network allows you to identify non-obvious problems of roaming. A common case is when in certain areas of the building mobile users have problems connecting to the network (although, it would seem, the corresponding access point is in order). One of the sources of such problems may be the too high power of the access point in the neighboring room - so instead of connecting to the nearest point, the client tries to connect to the one that is currently serving a large number of users (real case: connecting to an access point in a conference in the hall when the user simply passes by).
Tracing the development trends of wireless networks, we can expect that in the foreseeable future, not only giants, whose networks have thousands of access points, but also a medium-sized business, which may be limited to work on incidents, will encounter service problems. Assuming such a development of events, it is logical to look closely at new, more efficient standards and high-performance equipment. But it is worth remembering the necessary paradigm shift in network service, while clients have not yet begun mass migration to competitors due to the quality of service.
Of course, an onsite CampusInsight class product will be most useful in large-scale deployments, but now a cloud subscription is also available for the service from the local Public Cloud Huawei, designed for implementations in the SMB sector. In general, those who wish can try everything and "twist" right now.
How to do it? Only by tracking what is really important in this context is user interaction with the wireless network.
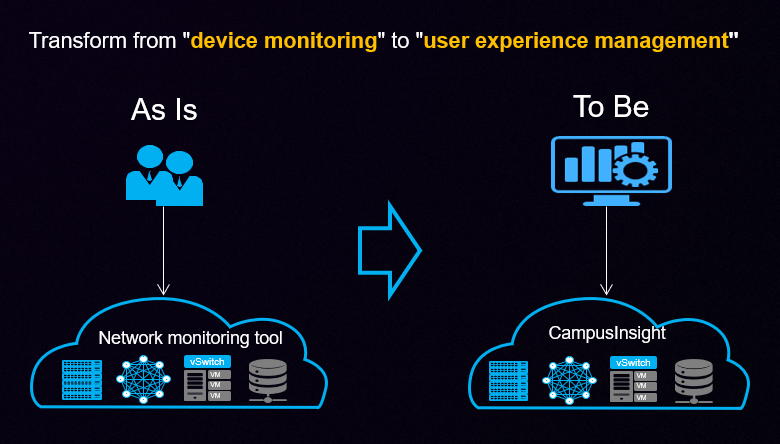
Network loads continue to grow, and this especially affects wireless segments - at least because of the openness of their interface. With the increasing number of devices and data transfer rates, problems multiply at once at several levels. On the physical - many radio signal transmitters affect each other, even if they work in neighboring parts of the frequency spectrum. Logically, a large number of connected devices begin to compete for the right to start transmission at the selected frequency, increasing the delay in packet delivery for each user.
At the same time, the expectations of each client from using the network are growing. A 5-second page loading in the browser, which 20 years ago seemed like the “top of the technology”, will not surprise anyone. Give customers HD video without fading.
The new versions of wireless transmission standards that use the frequency spectrum more efficiently can partially solve the problem. Each subsequent version of Wi-Fi aims to deploy more and more loaded networks. But in a large-scale network, where more than a dozen access points operate, it will not be possible to give everything to the next standard (all the more, the devices work in backward compatibility mode as soon as they meet an old user device). As it doesn’t succeed in continuing to live with old monitoring tools, the network environment is constantly getting complicated.
Why normal monitoring no longer works
The classic stamp, which still haunts the administrators of all networks, including wireless, is work exclusively on request. “Alarm” worked - we wake up and understand what went wrong. In the meantime, there is no “alarm”, you can limit yourself to checking the load on the main components - network and user devices.
In accordance with this task, traditional monitoring and maintenance tools work on the principle of strict rules and do not always promptly show existing problems, not to mention some kind of predictive analysis.
The main problem here is the data collection interval. Information about the state of wireless network connections is collected once every minute, and incidents may well occur in the intervals between the collection of readings (a great example is the rare bursts of load that "hang" the network). Not receiving real-time data, it’s quite difficult to understand what became the root cause of the problem. Is this misuse of network coverage? Or, perhaps, external interference that is not related to business in any way (for example, the military unit nearby “poured” it on the air). There is no data where it would be possible to see the gradual degradation of certain characteristics of the network, and therefore localizing the problem is not so simple. IT staff will have to spend extra hours searching for such a “needle in a haystack.”
But end users notice the problem almost immediately. A connection error, a broken video broadcast are excellent markers.
Classic monitoring tools report network packets coming. But they cannot answer the question in any way, but whether the user has solved his task.
To get an answer to this question, it will be necessary to change not just the tool, but the approach to monitoring organization itself. From "fire" work on requests (in fact, control of the performance and load of a specific iron), we proceed to control user experience and identify situations that could lead to incidents.
This transformation involves the introduction of more complex problem determination algorithms than simple warnings when certain values are reached. In the Huawei CampusInsight network intelligence platform, these algorithms are based on wireless service experience and self-learning techniques.
Under the hood CampusInsight
Huawei CampusInsight is a scalable platform for monitoring wireless networks of various sizes. Built on the basis of microservice architecture. Each service is deployed on several instances, the messages between which are distributed by the corresponding bus. Additional instances can be deployed dynamically, increasing tool throughput.
In fact, CampusInsight collects, analyzes, and displays data in its UI in five steps.

The first and second step is access to data (to devices that provide their generation) and the collection of "readings". Using Google’s GPB streaming telemetry capture and “traditional” Syslog (where possible), Huawei CampusInsight accumulates data in near real time:
- on utilization of the frequency spectrum;
- the functioning of access points and other network devices (performance indicators, number of connected users, etc.);
- about the path of specific users - about network profiles, about who, when and to which access point connected or not connected (and with what connection parameters);
- about the work of audio-video applications (using eMDI, implemented in one of the additional packages).
To circumvent the limitations of traditional tools that use SNMP to collect data and send fixed structures, CampusInsight was based on a subscription model for the necessary logs and data encoding and decoding algorithms.
The third step is distribution and buffering - i.e. sending raw data to Kafka for distribution to higher-level analysis services.
The fourth step is analysis. Big Data and AI algorithms help you quickly process raw data. As a result, certain problems are identified associated with:
- authentication (Dot1x protocol supported) and DHCP operation;
- stability and connection speed;
- wireless interfaces;
- the operation of individual devices, including “particulars,” such as problems with PoE or switching a dual-band device to 2.4 GHz;
- quality of audio-video streams - however, the function is supported only for unencrypted SIP or for some switches;
- roaming between different access points.
AI algorithms are used to solve some particular problems, for example, to detect interference between channels during wireless transmission.

The fifth and final step is to save the data in a distributed column database Druid for later use.
An analysis of the information collected, taking into account the “baseline” constructed using the same historical data, allows us to identify typical “failure patterns” - identifying KPIs corresponding to problem situations and localizing problems by suggesting ways to solve them. Thus, about 85% of all network problems fall into account of the tool.
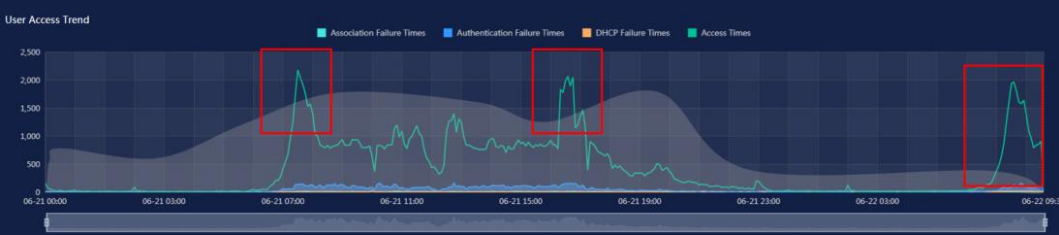
The data is presented to the administrator in a graphical form in accordance with the hierarchy or topology of the space (for example, the layout of the office). You can build “heat maps”, analyze how affected the equipment of certain platforms or manufacturers, etc. It’s easier to understand what exactly caused the problem.

In general, CampusInsight provides quite a few tools to classify problems, compare affected users, examine data about a particular client, and even “play back” events that preceded the incident in order to quickly identify the source. At the same time, the product also supports the new Wi-Fi 6, not to mention its predecessors.
Cases
CampusInsight has already been tested in practice, although most of the cases are closed by the NDA. The most revealing open case is the use of a monitoring tool in Huawei’s own wireless network.
The network covers enterprises where about 180 thousand people are employed, of which 80 thousand belong to the R&D division (these are offices in more than 170 countries, where a total of 62 thousand access points are installed).
The implementation of CampusInsight has helped to optimize more than 630 access points, while increasing incident analysis efficiency by 30%.
Below are a couple of specific situations.
Example 1. Group Failure
The high-level problems observed on a large number of users are often the result of low-level errors. And to identify such problems is not so simple. For example, in one of the offices, many mobile clients immediately experienced difficulties with authentication, despite the correct settings and the absence of problems with the authentication server. Visualization of the data at different levels helped to quickly identify that the switch was the source of the problem and generated too many errors. And to correct the situation, it was only necessary to replace a piece of cable. Localization and correction of the problem took 90 minutes.
Example 2. Tracking the quality of roaming
Collecting data along the path of a specific client within a distributed network allows you to identify non-obvious problems of roaming. A common case is when in certain areas of the building mobile users have problems connecting to the network (although, it would seem, the corresponding access point is in order). One of the sources of such problems may be the too high power of the access point in the neighboring room - so instead of connecting to the nearest point, the client tries to connect to the one that is currently serving a large number of users (real case: connecting to an access point in a conference in the hall when the user simply passes by).
To solve the problem, sometimes it is enough to reduce the signal strength of the loaded point, however, the identification requires a deep analysis of recurring problems in the rooms adjacent to the conference room.
Tracing the development trends of wireless networks, we can expect that in the foreseeable future, not only giants, whose networks have thousands of access points, but also a medium-sized business, which may be limited to work on incidents, will encounter service problems. Assuming such a development of events, it is logical to look closely at new, more efficient standards and high-performance equipment. But it is worth remembering the necessary paradigm shift in network service, while clients have not yet begun mass migration to competitors due to the quality of service.
Of course, an onsite CampusInsight class product will be most useful in large-scale deployments, but now a cloud subscription is also available for the service from the local Public Cloud Huawei, designed for implementations in the SMB sector. In general, those who wish can try everything and "twist" right now.