
Dangerous industries: we are watching you,% username% (video analytics)
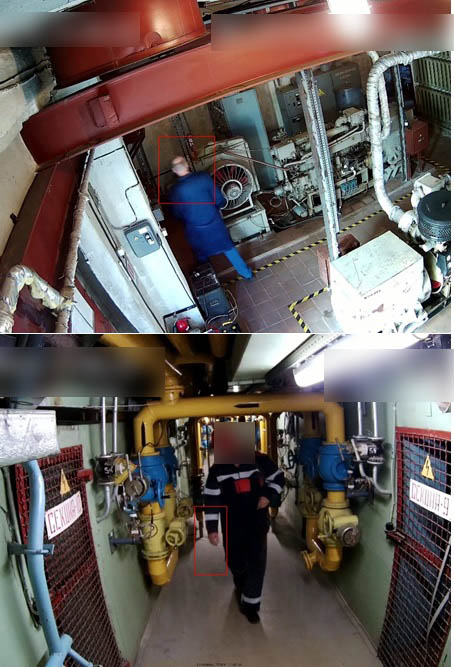
One comrade - without a helmet, the second - without a glove.
There are many not-so-good cameras at production facilities, the quadrants of which are not the most attentive grandmothers. More precisely, they simply go crazy with uniformity there and do not always see incidents. Then they slowly call, and if it was a call to the danger zone, then sometimes it makes no sense to call the workshop, you can immediately relate the worker.
Progress has come to the point where the robot can see everything and give lyuli to everyone who violates. For example, reminding via SMS, a light current discharge to the siren, vibration, a nasty squeak, a flash of bright light, or simply tell the head.
Specifically:
- It is very easy to recognize people without a helmet. Even bald. They saw a man without a helmet - immediately an alert to the operator or the head of the workshop.
- The same applies to goggles and gloves in hazardous industries, belt insurance (though we only look at the carabiner for now), reflective vests, respirators, hair caps and other PPE. Now the system is trained to recognize 20 types of PPE.
- You can accurately count people at the facility and take into account when and how many there were.
- It is possible to give an alarm when a person enters a danger zone, and this zone can be configured upon the start-stop of the machines.
Etc. The simplest example is the color differentiation of bricklayers and concrete pourers by helmet color. To help the robot. In the end, living in a society with a lack of color differentiation means not having a goal.
How to steal at a construction site
One type of widespread theft is when the contractor promised to bring 100 workers to the facility, and in fact brought 40–45. And the house is being built and built. All the same, no one can accurately calculate them in fact. As in a famous joke: if a bear settles on a construction site and eats people, no one will notice. So the general contractor does not have any opportunity to control the brigades. More precisely, even if you use an ACS, it will still be deceived, as in this post about the terminator cat .
Typically, there are no ACS at construction sites or they are only for entry.
We traveled to exchange experiences with highly developed civilizations and saw that each profession (more precisely, its role) has its own helmet color. The bricks are laid by the pavers - their helmets are blue, the concrete is poured by the pourers - they are green, all kinds of wise guys go around - they are yellow, so you need to do “ku” twice before them. Etc.
And all this is necessary to very easily detect each role. There are several dozens of rather cheap cameras on site that give something like 320x200 in color. Helmet workers are considered in real time, and a specific construction site is tied to each cell. As a result, all this at the end of the day in analytics is sewn into the accounting of charts by zones: who, in what quantity and in what section worked.
In general, we took over the experience. Only while we looked closely, the neural networks stepped far forward, and many new detectors appeared. A few years ago they were rather capricious and unstable, and now they allow you to very accurately catch the most interesting situations. Not least because of the processing speed, the detectors are often mistaken on separate frames, and on the video stream with small changes in angle, we get an excellent practical result.
And if I fasten the second helmet to the belt?
First, we learned that a worker can get two helmets and one of them to fasten on his ass. We immediately had two detectors: search for a skeleton and determining a color spot to match the top of this skeleton and searching for synchronously moving objects. The second turned out to be easier to detect: for example, a person with a helmet on his ass almost never looks around this helmet. Because for this you need to turn your head. And this movement is very easily detected. More precisely, we don’t know what exactly is detected there (it’s a neural network), but she learned very quickly and catches violators, one might say, by gait.

We are building a model of man.
Then we just build a heatmap in real time and reports at the end of the day.
Accordingly, by the same principle - training neural networks - it is easy to detect:
- Helmets.
- Robes.
- Vests.
- Boots.
- Protruding hair.
- Safety carabiners.
- Respirators
- Protective glasses.
- Correct wearing a jacket (important for electrical equipment: it may shandarahnut in the hall at the factory).
- Removal of large tools around the perimeter.
In total, 29 detectors have already been tested. The only point is that since we work in hazardous industries like chemistry or mining, there are requirements for the types of gloves. For example, long and short. In this case, they must be of different colors: the length under the sleeve on the camcorder is very difficult to determine.
And here there were often rats. We do not have a separate rat detector, but there is a detector of objects that interfere with the operation of the machine:

What else is detected?
We tested the detectors in the chemical industry, in the mining sector, in the nuclear industry and in construction sites. It turned out that with a little effort you can close a few more requirements that were previously solved by the same grandmothers who were stupidly trying to make out something in the picture through poor resolution and with a poor frame rate. Specifically:
- Since we still build the skeletal model of each employee, we can determine the fall. By falling, you can immediately stop the machine, next to which it is located (there was no such integration in the pilot implementations, there were just worries). Well, this is if you have IioT.
- Of course, being in dangerous areas. It is very easy, very accurate and very useful to everyone. At metallurgical enterprises, people work near tanks of boiling steel, it is useful to temper steel, but sometimes it is dangerous to stand on the wrong side. Given the work of different components and equipment, you can change these dangerous zones, set them a schedule, and so on.
- Another very useful detector about the presence of PPE monitors the responsibility of employees and verifies that they are not in danger. Here the grandmother very responsibly approaches the task of accounting and wears all the PPE assigned to her. Commendable!

Behavior control was very easy to implement - specifically, the worker is sleeping or not. While we were testing all this, the rules evolved from “There should be a person in a green helmet in this zone” to “A person in a green helmet should move in this area”. So far, there was only one clever man who cut through a chip and turned on the fan, but this too turned out to be easy to fix.
It was very important for chemists to fix all sorts of steam jets, smoke. In the oil industry, pipe integrity. Fire is generally a standard detector. And there is also a check of closed hatches.

Similarly, forgotten things are detected. We ran it at one of the stations a couple of years ago, there it almost does not make sense because of the large number of events. But in factories, especially chemical ones, it’s very convenient to keep track of things in a clean area.
Interestingly, right from video analytics we can read instrument readings in the camera area. This is true for the same chemists whose production complexes have a high hazard class. Any change like replacing a sensor is a project renegotiation. It is long, expensive and painful. More precisely, long, expensive and painful. Therefore, their Internet of things will come late. Now they want video surveillance on the meters and read the data, quickly respond to them and reduce losses due to unexpectedly and unnoticed equipment failure. Based on the current data from the counters, you can build a digital double of the enterprise, introduce the predictive maintenance and repair, but this is a completely different story ... There is already control: we are writing proactive analytics based on the totality of data. And separately, a battery replacement prediction module.
Another incredible thing - it turned out that in granaries and in storages of materials such as crushed stone, you can remove a bunch from 3-4 angles and determine its edges. And having determined the edges - give the volume of grain or material with an error of up to 1%.
The last detector that we wrote was the control of driver fatigue, such as “pecking,” yawning, and blink rates. This is for HD cameras where the eyes are visible. Most likely, it will be put in control rooms. But the main need is for BelAZ, KamAZ for a career. There, it happens, cars fall, so now they are forced to invent something in production to control the driver. A robot is better than a grandmother.
About cars. For example, the topic of fatigue control is actively used by automakers not only BelAZ, KAMAZ and other MAZ. Already in ordinary ordinary cars, manufacturers integrate warning systems for driver fatigue, but so far they have fairly simple solutions that analyze only the position of the car relative to the markings and the nature of the steering wheel. We went further and detect human behavior, which is much more complicated.
Another case of driver surveillance is the detection of abnormal behavior when using car sharing machines. They can’t talk on the phone without hands free, eat, drink, smoke and much more.

Ah, and the last. For several years now, we have been able to track an object between cameras - when, for example, something is stolen, you need to check how and how. If there are 100 cameras at the object, then you are tormented by raising the material. And then the system will form an action-packed thriller about Ocean and his friends automatically.
What is the difference from a two-year-old system? Now it’s not just recognition like “the bald man in an orange jacket came out of one camera and went into another almost immediately”, but a mathematical model of the building is built, and from it hypotheses about the movement of the object. That is, all this began to work in areas with overlappings and places with blind spots, and sometimes extensive ones. And the detectors are now much better, because there are libraries that determine the age by face. On HD cameras, you can set orientations like “a man of 30 years with a woman of 35 years”.
So, maybe in 5-7 years we will finish the production and go to your home. For safety. It is in your interests, citizen!