Theoretical and informational approach to the analysis of sales funnels in contextual advertising
In fact, this post is a continuation of the article on the methods of clustering key phrases in optimizers: why are conversion optimizers in contextual advertising needed and why the quality of their work decreases if they deal with key phrases for which insufficient statistics are collected (more about this in the first two parts of the article ) As a solution to the problem of lack or lack of data for forecasting conversion, we considered the classic and alternative methods of pooling - increasing statistics for a phrase by borrowing statistics for other phrases.
In this post, Calltouch Senior Product Manager Fedor Ivanov will talk about the importance of another approach to forecasting conversions by key phrases - analyzing microconversions as key metrics that affect the final conversion on a site (which we will call macroconversion).
In everyday practice, we often come across the concept of "conversion." By this term we mean some targeted action performed by the user on the site. Examples of such targeted actions can be a transaction, a phone call, sending an application, registering on a site, etc. Which action is considered to be conversion is primarily the choice of the advertiser, which he makes based on the specifics of the business, the functionality of the site, etc.
It is intuitively clear that a conversion has some value, and because the more conversions occur on a site per unit of time, the more benefits (direct or indirect) an advertiser receives. In this regard, the conversion rate can be considered as the share of those site visitors who ultimately converted, and CPA as the average cost of attracting one “conversion” visitor.

So, this model is the simplest sales funnel: the entire audience of the site gets into the entrance, and at the output that part of it that made the conversion (macro).
However, in the process of their “life” on the site, visitors can perform certain actions that in one way or another make it possible to evaluate their loyalty and willingness to perform a targeted action on the site. Examples of such actions include:
Such actions are called "microconversions." To summarize, micro-conversions are intermediate actions of the user on the site, which to a certain extent reflect his measure of involvement and interest in the offered goods and services. If the analytics system is configured in such a way that these actions are monitored, then we can significantly enrich our initial sales funnel:

It is obvious that statistics on micro-conversions can accumulate several times faster than statistics on major macro-conversions. For example, there are significantly more users who view 5 pages of the site than those who purchase the product. This fact will significantly accelerate the collection of statistics necessary to run the optimizer.
On the other hand, microconversion analysis will allow you to track down weaknesses in the sales funnel, which will simplify website analytics. For example, if out of 1000 visitors to the site 200 went to the “basket” section, and only 10 placed an order from the “basket”, it would be reasonable to assume that the form where the user is invited to place an order needs processing and improvement.
Nevertheless, when we gave the definition of microconversion, we did not in vain mention that the indicator of one or another microconversion “to a certain extent” reflects the user's loyalty and his readiness to make macroconversion. For example, if the goal “user session length is at least 10 minutes” is selected as a microconversion, then a high conversion rate to this goal does not necessarily mean a high conversion rate of the site as a whole.
Therefore, the important issue is the analysis of the impact of a particular microconversion on macroconversion. In the next section, we will discuss in detail the existing approaches to such an analysis.
The simplest method for assessing the effect of one quantity on another (in our case, micro-conversion for a given goal on macro-conversion) is a correlation analysis. This approach was developed in one of the sections of mathematical statistics, science, which operates with random variables.
A random variable is an event that occurs with a certain probability, which obeys some distribution law. Therefore, we abstract from the concepts of conversion and microconversion, and we will talk about random variables
- the number of macro conversions and
- the number of micro conversions for some purpose
. The values of these indicators that we observe in any sections (on the site, on a separate advertising campaign, and even on a separate keyword) are called implementations (values) of this random variable.
Correlation coefficient
(Pearson linear correlation) allows you to evaluate the relationship of random variables
and
according to their observed N values (specific implementations). This value takes a value from -1 to 1. The closer the value
to unity (or to -1), the more “random” (directly or inversely) are “connected”. In other words, the more accurately we can “guess” the value
having information about
. In the first case, the dependence is direct:
and in the second - the opposite:
.
In our interpretation, this means that if
, the more microconversions happened
, the more you should expect macro conversions
. If
, the more microconversions happened
, the less macro conversions you should expect
.
An example of directly correlating random variables is the number of users who made a purchase and the number of users who put the product in the basket. An example of inverse correlating random variables is the number of transactions and the number of failures.
The correlation coefficient makes it possible to accurately predict the behavior of one random variable relative to another, but there are a number of limitations. First, the volume of samples (observed values) should be quite large (several dozen observations), which will not automatically reveal the effect of one quantity on another at the micro level (for example, at the level of a key phrase). In addition, correlation calculation formulas are accurate only when the distributions for
and
are Gaussian (normal). In addition, when calculating the coefficient
only observable values are used, during smoothing of which (for example, pooling) a systematic error increases.
In addition, an interesting fact is that the lack of correlation between the two quantities does not mean that there is no connection between them. For example, the dependence can be complex nonlinear in nature, which correlation does not reveal. That is, the widespread belief that zero correlation means independence of events is wrong. The following picture is a clear demonstration of the above: The

correlation coefficient of two random variables is indicated above the figure, and the values of these quantities are plotted on the figure itself. It is easy to see that the correlation well “predicts” only a linear dependence, without detecting any other.
We have proposed an alternative approach to the analysis of microconversions, based on the principles and fundamental concepts of information theory, which can significantly simplify the search for the relationship between conversions at different levels even if this dependence is complex, non-linear.
Mutual information is one of the basic concepts of the general theory of information. It is defined as follows: Mutual information is a statistical function of two random variables that describes the amount of information contained in one random variable relative to the other.
Thus, knowing the distributions of two random variables, we can calculate how much they are interconnected (with any form of dependence) in terms of how much information about one event (for example, macroconversion) indicates the occurrence (or not occurrence) of another event (for example, microconversion for some purpose).
General formula for calculating mutual information
between random variables
and
following:
- entropies of quantities
and
, a
- conditional entropy of the event
upon the occurrence of an event
. A little later, we will consider ways of calculating each of the quantities included in the formula, but first we will discuss the basic properties of mutual information.
• It is symmetrical:
• It is limited to:![$ 0≤I (A; B) ≤min [H (A), H (B)] $](https://habrastorage.org/getpro/habr/formulas/38e/a1b/11b/38ea1b11b9ac6fcfad6f06379c12f754.svg)
• It is equal to entropy if the events are connected by any functional dependence.
The presence of all the above properties allows us to consider mutual information as a good analogue of correlation in calculating the relationship between events. In particular, the latter property will make it possible to detect a dependence of an arbitrary nature, but unfortunately the establishment of its form is a separate and rather nontrivial task.
All the properties that we examined above indicate that mutual information can be considered as a metric between events (conversions and microconversions). However, in practice it is convenient to use normalized mutual information:
. Moreover, the smaller the interrelated values, the closer the metric value to 1, and vice versa - the more the onset of one event speaks of another, the closer the metric to 0. Formulas of this kind are often used in machine learning problems to select significant features (which helps to reduce the dimension of the problem ) In addition, a similar approach is actively used in classification and clustering problems. We suggest using it to assess the effect of microconversion on the formation of the final conversion (macro).
Since mutual information can only be calculated for random variables with given distribution functions, it is first necessary to choose the distributions for
and
. Due to the fact that conversions (and micro-conversions) occur independently of each other with some probabilities (probabilities of conversion and micro-conversions)
and
, then the behavior of such quantities is best described using the binomial distribution law, namely:
where under
and
we understand the probability that a conversion (microconversion) has occurred and the probability of the opposite event, respectively. Such a model, in addition to its simplicity, is convenient because with insufficient statistical data necessary for calculating
and
, these values can be calculated using the pooling method (see ppcworld ). In addition, for a binomially distributed random variable
simple enough to calculate
:
Conditional entropy between random variables
and
can be calculated by the formula:
Where:
Based on the definition of conditional probability, we can calculate:
.
By definition:
The calculation of these four probabilities requires the accumulation of the following statistics: for each click that we number with some clickID, it is necessary to compile a vector indicator of whether the user session initiated by this click led to microconversion on the target and to macroconversion on the site:
if there was no conversion for goal i,
if there was a conversion for goal i.
If we additionally add keywordID, bannerID, campaignID to the statistics, we can calculate the effect of micro-conversions on the conversion for each keyword, banner, campaign. If it turns out that your own statistics are not enough for calculation, then it can always be "inherited" from a higher level using the pooling method. Thus, we finally have:

Aggregated statistics in this way will allow us to calculate both numerators and denominators in
which ultimately will make it possible to calculate the conditional entropy between the conversion and each microconversion, which means that the task of calculating the effect of microconversions on the final conversion on the site is completely solved.
In addition, by summing up the data at the levels of keyword, banner, campaign, we can calculate the dependencies between conversions and micro-conversions not only at the level of the site as a whole, but also at lower levels, which will significantly increase the effect of optimization.
In the next chapter, we will consider the question of how, based on the data obtained, to estimate the weight of micro-conversion, and how to use this information for optimization.
To assess the effect of micro-conversion for some purpose on the overall conversion on the site, it is convenient to use the normalized formula of weights:
If we calculated the impact coefficient (weight) for all conversions to the site, then choosing the goals with the greatest influence, we can optimize (for example, using A / B testing methods ) those parts of the site where microconversion data is collected to increase overall conversion.
If we are facing the goal of bid optimization for contextual advertising, then we need to calculate
at the level of each keyword
(it’s for them that the conversion optimizer calculates and sends optimal bids):
Is some function
which depends on statistics
(primarily on the macro conversion factor
) and from KPIs that are set as target:
is a certain monotonously decreasing function of the number of clicks and macro conversions by keyword: the more clicks and macro conversions, the smaller the value
(the less we need knowledge about the behavior of micro-conversions for calculating bids).
Thus, information on microconversions is especially valuable in the case of a small volume of statistics on keywords.
The algorithms described in this paper are an integral part of Calltouch conversion optimizer. Optimization based on microconversions is especially effective if the number of conversions that the optimizer tries to maximize within the established KPIs is small. This is the case either for small advertising accounts, or in the case of choosing a specific type of conversion. If we consider call optimization (it is Calltouch that specializes in this type of optimization), then a tagged call is a specific type of conversion. The system allows you to tag calls both manually and using the Calltouch Predict automatic tagging tool.
In this section, we present the results of a joint case study with the Ashmanov & Partners agency on optimizing the context for BIIKS (Well Drilling). The purpose of the test was to increase the number of targeted calls heard within the current CPA.
Conversion cost: by connecting advertising campaigns to Calltouch Optimizer, the client expected to keep the current cost of conversion, while receiving maximum calls. For the first 4 weeks of the test, the following results were achieved:
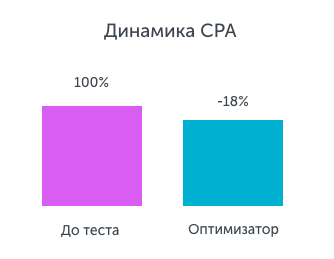
Conversion rate: on advertising campaigns connected to Calltouch Optimizer, the conversion rate for the test period increased by 55%.

The number of conversions: the number of conversions during the test period increased significantly: the increase when using Optimizer was 91%

The paper considers a new approach to the analysis of microconversions and their impact on the main conversion on the site. This approach is based on the principles of information theory. For its implementation, it is enough to choose certain goals that will be monitored by the analytics system, and select among them the main (conversion) and accumulate statistics on clicks to the site at least 30 days in advance. The technique proposed in the article can be used both to identify "weak" spots on the site, and to optimize rates in contextual advertising.
In this post, Calltouch Senior Product Manager Fedor Ivanov will talk about the importance of another approach to forecasting conversions by key phrases - analyzing microconversions as key metrics that affect the final conversion on a site (which we will call macroconversion).
What can microconversions give us?
In everyday practice, we often come across the concept of "conversion." By this term we mean some targeted action performed by the user on the site. Examples of such targeted actions can be a transaction, a phone call, sending an application, registering on a site, etc. Which action is considered to be conversion is primarily the choice of the advertiser, which he makes based on the specifics of the business, the functionality of the site, etc.
It is intuitively clear that a conversion has some value, and because the more conversions occur on a site per unit of time, the more benefits (direct or indirect) an advertiser receives. In this regard, the conversion rate can be considered as the share of those site visitors who ultimately converted, and CPA as the average cost of attracting one “conversion” visitor.

So, this model is the simplest sales funnel: the entire audience of the site gets into the entrance, and at the output that part of it that made the conversion (macro).
However, in the process of their “life” on the site, visitors can perform certain actions that in one way or another make it possible to evaluate their loyalty and willingness to perform a targeted action on the site. Examples of such actions include:
- View at least 5 pages of the site
- Go to the "basket" section
- View contact information
- Newsletter subscription
Such actions are called "microconversions." To summarize, micro-conversions are intermediate actions of the user on the site, which to a certain extent reflect his measure of involvement and interest in the offered goods and services. If the analytics system is configured in such a way that these actions are monitored, then we can significantly enrich our initial sales funnel:

It is obvious that statistics on micro-conversions can accumulate several times faster than statistics on major macro-conversions. For example, there are significantly more users who view 5 pages of the site than those who purchase the product. This fact will significantly accelerate the collection of statistics necessary to run the optimizer.
On the other hand, microconversion analysis will allow you to track down weaknesses in the sales funnel, which will simplify website analytics. For example, if out of 1000 visitors to the site 200 went to the “basket” section, and only 10 placed an order from the “basket”, it would be reasonable to assume that the form where the user is invited to place an order needs processing and improvement.
Nevertheless, when we gave the definition of microconversion, we did not in vain mention that the indicator of one or another microconversion “to a certain extent” reflects the user's loyalty and his readiness to make macroconversion. For example, if the goal “user session length is at least 10 minutes” is selected as a microconversion, then a high conversion rate to this goal does not necessarily mean a high conversion rate of the site as a whole.
Therefore, the important issue is the analysis of the impact of a particular microconversion on macroconversion. In the next section, we will discuss in detail the existing approaches to such an analysis.
Microconversion Impact Assessment Methods
The simplest method for assessing the effect of one quantity on another (in our case, micro-conversion for a given goal on macro-conversion) is a correlation analysis. This approach was developed in one of the sections of mathematical statistics, science, which operates with random variables.
A random variable is an event that occurs with a certain probability, which obeys some distribution law. Therefore, we abstract from the concepts of conversion and microconversion, and we will talk about random variables
Correlation coefficient
In our interpretation, this means that if
An example of directly correlating random variables is the number of users who made a purchase and the number of users who put the product in the basket. An example of inverse correlating random variables is the number of transactions and the number of failures.
The correlation coefficient makes it possible to accurately predict the behavior of one random variable relative to another, but there are a number of limitations. First, the volume of samples (observed values) should be quite large (several dozen observations), which will not automatically reveal the effect of one quantity on another at the micro level (for example, at the level of a key phrase). In addition, correlation calculation formulas are accurate only when the distributions for
In addition, an interesting fact is that the lack of correlation between the two quantities does not mean that there is no connection between them. For example, the dependence can be complex nonlinear in nature, which correlation does not reveal. That is, the widespread belief that zero correlation means independence of events is wrong. The following picture is a clear demonstration of the above: The

correlation coefficient of two random variables is indicated above the figure, and the values of these quantities are plotted on the figure itself. It is easy to see that the correlation well “predicts” only a linear dependence, without detecting any other.
We have proposed an alternative approach to the analysis of microconversions, based on the principles and fundamental concepts of information theory, which can significantly simplify the search for the relationship between conversions at different levels even if this dependence is complex, non-linear.
What is mutual information?
Mutual information is one of the basic concepts of the general theory of information. It is defined as follows: Mutual information is a statistical function of two random variables that describes the amount of information contained in one random variable relative to the other.
Thus, knowing the distributions of two random variables, we can calculate how much they are interconnected (with any form of dependence) in terms of how much information about one event (for example, macroconversion) indicates the occurrence (or not occurrence) of another event (for example, microconversion for some purpose).
General formula for calculating mutual information
• It is symmetrical:
• It is limited to:
• It is equal to entropy if the events are connected by any functional dependence.
The presence of all the above properties allows us to consider mutual information as a good analogue of correlation in calculating the relationship between events. In particular, the latter property will make it possible to detect a dependence of an arbitrary nature, but unfortunately the establishment of its form is a separate and rather nontrivial task.
Mutual information as an event dependency metric
All the properties that we examined above indicate that mutual information can be considered as a metric between events (conversions and microconversions). However, in practice it is convenient to use normalized mutual information:
Random Value Modeling
Since mutual information can only be calculated for random variables with given distribution functions, it is first necessary to choose the distributions for
where under
Calculation of conditional entropy
Conditional entropy between random variables
Where:
- the likelihood that the click did not lead to either micro or macro conversion
- the likelihood that the click led to macro conversion, but there was no micro conversion
- the likelihood that the click led to microconversion, but there was no macroconversion
- the likelihood that a click will lead to both micro and macro conversion
- the probability of the absence of microconversion, provided that there was no macroconversion
- the probability of the absence of microconversion, provided that a macroconversion has occurred
- the probability of microconversion if there was no macroconversion
- the probability of microconversion, provided that a macroconversion has occurred
Based on the definition of conditional probability, we can calculate:
By definition:
The calculation of these four probabilities requires the accumulation of the following statistics: for each click that we number with some clickID, it is necessary to compile a vector indicator of whether the user session initiated by this click led to microconversion on the target and to macroconversion on the site:
If we additionally add keywordID, bannerID, campaignID to the statistics, we can calculate the effect of micro-conversions on the conversion for each keyword, banner, campaign. If it turns out that your own statistics are not enough for calculation, then it can always be "inherited" from a higher level using the pooling method. Thus, we finally have:

Aggregated statistics in this way will allow us to calculate both numerators and denominators in
In addition, by summing up the data at the levels of keyword, banner, campaign, we can calculate the dependencies between conversions and micro-conversions not only at the level of the site as a whole, but also at lower levels, which will significantly increase the effect of optimization.
In the next chapter, we will consider the question of how, based on the data obtained, to estimate the weight of micro-conversion, and how to use this information for optimization.
Micro Conversion Weights & Bid Optimization
To assess the effect of micro-conversion for some purpose on the overall conversion on the site, it is convenient to use the normalized formula of weights:
If we calculated the impact coefficient (weight) for all conversions to the site, then choosing the goals with the greatest influence, we can optimize (for example, using A / B testing methods ) those parts of the site where microconversion data is collected to increase overall conversion.
If we are facing the goal of bid optimization for contextual advertising, then we need to calculate
Thus, information on microconversions is especially valuable in the case of a small volume of statistics on keywords.
Optimization case
The algorithms described in this paper are an integral part of Calltouch conversion optimizer. Optimization based on microconversions is especially effective if the number of conversions that the optimizer tries to maximize within the established KPIs is small. This is the case either for small advertising accounts, or in the case of choosing a specific type of conversion. If we consider call optimization (it is Calltouch that specializes in this type of optimization), then a tagged call is a specific type of conversion. The system allows you to tag calls both manually and using the Calltouch Predict automatic tagging tool.
In this section, we present the results of a joint case study with the Ashmanov & Partners agency on optimizing the context for BIIKS (Well Drilling). The purpose of the test was to increase the number of targeted calls heard within the current CPA.
Test results:
Conversion cost: by connecting advertising campaigns to Calltouch Optimizer, the client expected to keep the current cost of conversion, while receiving maximum calls. For the first 4 weeks of the test, the following results were achieved:
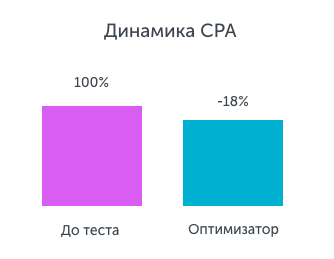
Conversion rate: on advertising campaigns connected to Calltouch Optimizer, the conversion rate for the test period increased by 55%.

The number of conversions: the number of conversions during the test period increased significantly: the increase when using Optimizer was 91%

Conclusion
The paper considers a new approach to the analysis of microconversions and their impact on the main conversion on the site. This approach is based on the principles of information theory. For its implementation, it is enough to choose certain goals that will be monitored by the analytics system, and select among them the main (conversion) and accumulate statistics on clicks to the site at least 30 days in advance. The technique proposed in the article can be used both to identify "weak" spots on the site, and to optimize rates in contextual advertising.