Increase it! Modern resolution increase
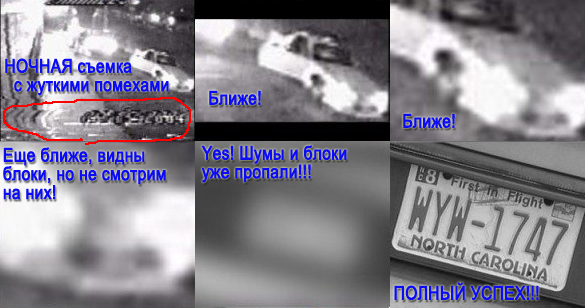
I have ceased to shudder and wonder when the phone rings and the tube is dealt a hard confident voice: "Are you concerned about the captain of such a (Major-so), you can answer a few questions?" Why not talk to the native police ...
Questions always same. "We have a video with a suspect, please help restore the face" ... "Help increase the number from the DVR" ... "Here you can not see the hands of a person, please help increase" ... And so on in the same vein.
To make it clear what I am talking about - here is a real example of a highly compressed video file sent where it is asked to restore a blurred face (the size of which is equivalent to about 8 pixels)
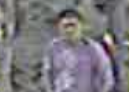
And it would be fine if only the Russian uncles of the Steppe were disturbed; the Western Pinkertons also write.
For example, a letter from the police of England <***** @ *****. Fsnet.co.uk>:
I have been using my filter for my work. I’m currently getting a lot of CCTV video footage. I could use them.Or a policeman from Australia writes:
Thank youTransferЯ уже пользовался вашими фильтрами для личных целей, чтобы спасти свои плохие видео с семейных праздников. Но мне бы хотелось использовать коммерческие фильтры в своей работе. В данный момент я офицер полиции в небольшом подразделении. Мы получаем большое количество видео с камер видеонаблюдения, иногда очень плохого качества, и ваши фильтры действительно помогут. Не могли бы вы сказать мне их стоимость, и могу ли я их использовать?
Спасибо
Hi,Note that the letter is very thoughtful, the person is worried about the algorithm being published and about the responsibility for incorrect restoration.
I am in the video and Audio forensics unit. We occasionally receive video cameras from either hand-held or vehicle-mounted cameras. Often these captures interlaced footage of fast-moving events. In particular, the footage of which usually has the most promise is the footage of vehicle number plates. We often find that the vehicle has been captured. If you’ve been on the field, you can’t get it, sometimes it’s rotated, sometimes it’s a bit different. can be difficult.
If you’re looking for footage, you can’t need it. To be honest, as our budget is, you can not afford a commercial license. We do not sell the product, of course, we do not need to sell it. In any case, I’ve been asking for anyway. How much would it cost for a license? It is possible to test the product on the footage, to see if it is appropriate? Does it need some of what we need? Lastly, has the algorithm been published? Working with unknown algorithms is dangerous practice for a court of law. It is good practice to know why!
Any information you can offer would be appreciated.
Regards,
Caseworker
Audio Visual Unit
Victoria Police Forensic Services DepartmentTransferПривет,
Я работаю в полиции Виктории в Австралии в отделе видео и аудио криминалистики. Время от времени мы получаем видео с ручных камер и видеорегистраторов. Часто эти видео — чересстрочная съемка быстродвижущихся объектов. В частности, наиболее важный материал — номерные знаки транспортных средств. Мы часто обнаруживаем, что рассматриваемое транспортное средство сильно перемещается между первым и последним отснятым полем. В результате мы пытаемся восстановить целый кадр из двух полей, причем второе — сдвинутое, иногда повернутое и время от времени отличающееся размером (когда автомобиль едет к или от камеры). Объединение этих двух полей, желательно в полупиксельной точности, и восстановление целого кадра, содержащего номерной знак, может быть затруднительным.
Я вижу, как вы применяете деинтерлейсинг к кадрам, и может быть ваши фильтры могут сделать что-то, если не все, что нам нужно. Честно говоря, возможно, мы не сможем позволить себе коммерческую лицензию, потому что наш бюджет достаточно мал. Мы не продаем продукт, конечно, мы используем его для доказательств в полицейских делах. В любом случае, я думал, что напишу письмо и все равно спрошу. Сколько будет стоить лицензия? Можно ли протестировать продукт на материале, чтобы узнать, подходит ли он? Делает ли он часть того, что нам нужно? Наконец, был ли опубликован алгоритм?.. Работа с неизвестными алгоритмами — опасная практика в суде. Если доказательства приводят к тому, что человек садится в тюрьму на 20 лет, полезно знать, почему.
Будем благодарны за любую информацию, которую вы можете предоставить нам.
С уважением,
Следователь
Отдела аудио и видео
Департамента судебно-медицинской экспертизы полиции Виктории
Sometimes they only admit to the process of correspondence that they are from the police. For example, the Italian Carabinieri would like to get help:
Dr. VatolinAnd, of course, many appeals of ordinary people ...
Thanks for the answer.
Carabinieri investigation
scientific for PARMA ITALY?
To which software they have been associates of your algorithms to you.
We would be a lot.TransferДр. Ватолин
Спасибо за ответ.
Подходит ли это для полиции (Подразделение расследований Карабинеров для PARMA ITALY)?
Они интересуются, в каком программном обеспечении используются ваши алгоритмы?
Будем признательны.
Increase it! What, you feel sorry for the right button to press?
It is clear that this whole stream of appeals does not appear from scratch.
"Blame" in the first place movies and TV shows.
For example, here in 3 seconds a frame of compressed video is increased 50 times and from the reflection in glasses they see a clue:
And there are many such moments in modern films and serials. For example, in this video absolutely epic collected similar episodes from a pack of serials, do not take two minutes to watch:
And when you see this in every movie, then the last hedgehog becomes clear that all you need is to have a competent computer genius, a combination of modern algorithms, and all you have to do is give STOP! And Enhance it! Just in time . And voila! A miracle will happen!
However, the scriptwriters do not stop at this already beaten reception, and their unrestrained fantasy goes further. Here is a completely monstrous example. Brave detectives on reflection in the PENTER of the victim received a photo of the criminal. Indeed, the reflection in the glasses already. This is trite. Let's go ahead! It was just by chance that the video surveillance camera in the stairwell turned out to be quite accidentally like that of the Hubble telescope:
In the Prophet (00:38:07):
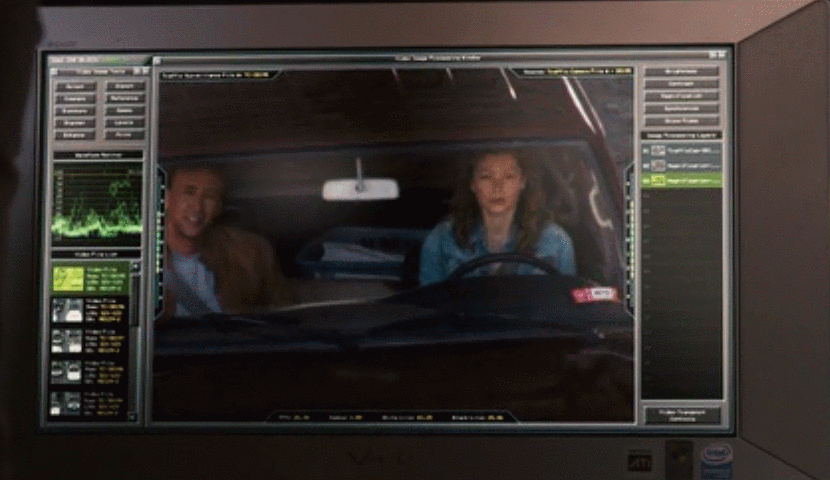
In Avatar (1: 41: 04–1: 41: 05), the sharpening algorithm is, by the way, some unusual compared to other films: it first sharpens in some places, and after a split second it draws the rest of the image . first the left half of the mouth, and then the right:
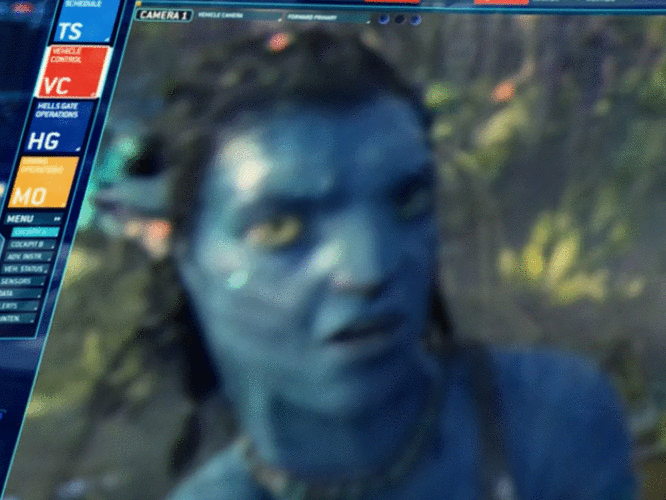
In general, in very popular films that watch hundreds of millions, the increase in sharpness of a picture is done in one click. All people (in movies) do it! So why you, such clever experts, cannot make it ???
- I know it is done easily! And they told me exactly what you are doing! Are you too lazy to press this button?
// Oh my God ... Damned scriptwriters with their wild imagination ...
- I understand that you are loaded, but this is about your help to the state in solving an important crime!
// We understand.
- Maybe it's money? How much do you need to pay?
// Well, how to explain briefly that it’s not that we don’t need money ... And then again, and then again ...
Any matches of quotes above with real dialogs are completely random, but, in particular, this text is written in order to send a person to first carefully read it, and then call back.
Conclusion: Due to the fact that the scene with the increase in images from CCTV cameras in one click has become a stamp of modern cinema, a huge number of people sincerely believe that to enlarge a fragment of a cheap camera or a cheap DVR frame is very simple. The main thing - how to ask (well, or command, it's as lucky).
Where do legs grow
It is clear that all this stream of calls is taken not from scratch. We are really engaged in improving the video for about 20 years, including various types of video recovery (and there are several types of them, by the way) and later in this section there will be our examples.
A “smart” increase in resolution in scientific articles is usually called Super Resolution (abbreviated as SR). Google Scholar for the query Super Resolution finds 2.9 million articles, i.e. the topic was dug pretty well enough, and a huge number of people were busy with it. If you follow the link , then there is a sea of results, one more beautiful than the other. However, it is worth digging deeper, the picture, as usual, becomes not so pastoral. There are two directions in the SR theme:
- Video Super Resolution (0.4 million articles) - the actual restoration using the previous (and sometimes subsequent) frames,
- Image Super Resolution (2.2 million articles) - “smart” increase in resolution using only one frame. Since in the case of a single picture, to take information about what was actually in this place is nowhere, the algorithms in one way or another complete (or, relatively speaking, “think out”) the picture - what could be there. The main criterion for this is that the result should look as natural as possible, or be as close as possible to the original. And it is clear that to restore what was “in fact”, such methods are not suitable, although to enlarge the picture so that it looks better, for example, when printing (when you have a unique photo, but there is no version in higher resolution ) such methods are very possible.
As you can see, 0.4 million versus 2.2 - that is, 5 times fewer people are engaged in the actual restoration. Fortunately, the “make it bigger, just beautiful” theme is very much in demand, including in the industry (the notorious digital zoom of smartphones and digital soap cases). Moreover, if you dive even deeper, it will quickly become clear that a noticeable number of articles on Video Super Resolution is also an increase in video resolution without recovery, because restoration is difficult. As a result, we can say that those who "make beautiful", about 10 times more than those who are really trying to recover. Quite a common situation in life, by the way.
We go even deeper. Very often, the results of the algorithm are very good, but it needs, for example, 20 frames forward and 20 frames back, and the processing speed of one frame is about15 minutes using the most advanced GPU. Those. for 1 minute video you need 450 hours (almost 19 days). Oops-ss ... Agree, this is not at all like the instant "Zoom it!" From the movies. Algorithms that work several days per frame occur regularly. For articles, a better result is usually more important than work time, because acceleration is a separate complex task, and it is easier to eat a large elephant in parts. This is how the difference in life and cinema looks like ...
A request for algorithms running on video at a reasonable speed spawned a separate direction Fast Video Super Resolution- 0.18 million articles, including “slow” articles, which are compared with “fast” ones, i.e. The real number of articles about such methods is too high. Note that among the “fast” approaches, the percentage is speculative, i.e. without real recovery, above. Accordingly, the percentage of honestly restoring is lower.
The picture, you see, clears up. But this, of course, not all.
What other moments significantly affect getting a good result?
First, the noise is very influential. Below is an example of double resolution recovery on a very noisy video:
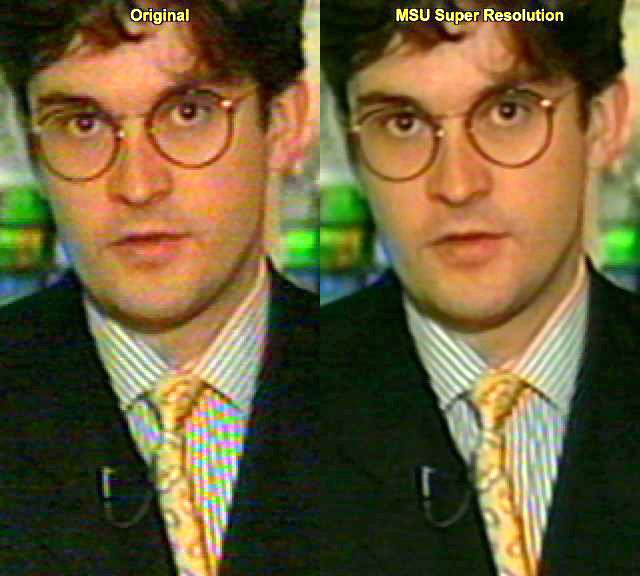
Source: materials of the author
The main problem on this fragment is not even with ordinary noises, but with a color moire on the shirt, which is difficult to handle. Some may say that today big noises are not a problem. This is not true. Look at the data from car DVRs and video surveillance cameras in the dark (just when they are more often in demand).
However, moire can also occur on relatively “clean” in terms of video noise, such as the city below (examples below are based on this work of ours ): Source: author's materials

Secondly, for optimal recovery, near-ideal prediction of motion between frames is needed. Why this is difficult is a separate big topic, but it explains why scenes with panoramic camera movement are often restored very well, and scenes with relatively chaotic movement are extremely difficult to restore, but with them you can get quite good results in some situations:

And finally, we give an example of text recovery:
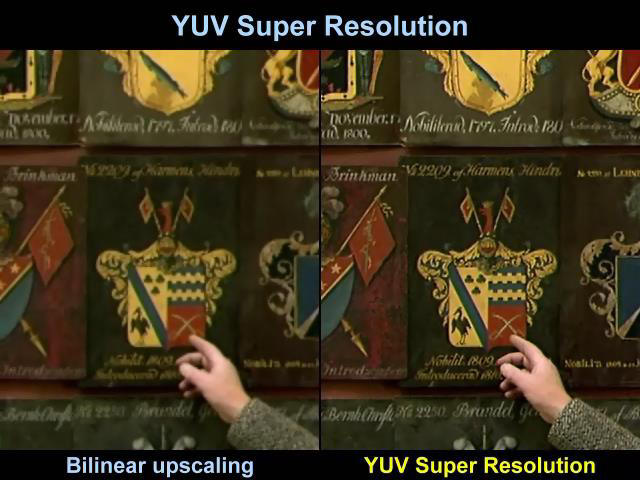
Source: materials of the author.
Here the background moves quite smoothly, and the algorithm has the ability to “roam”:
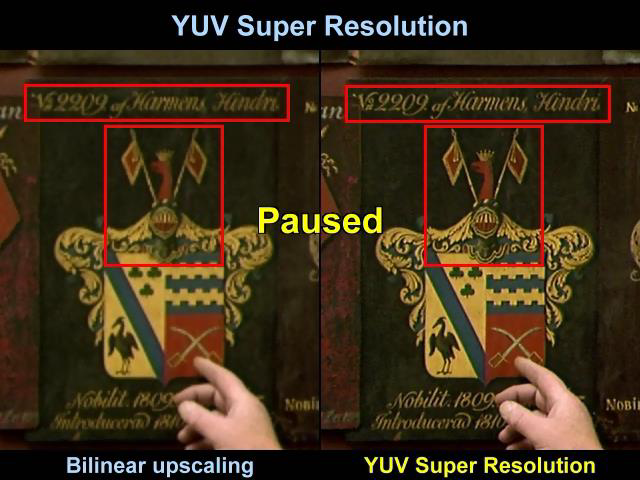
In particular, if we compare a very small inscription to the right of the hand, including the magnification with the classical bicubic interpolation , then the difference is clearly visible:
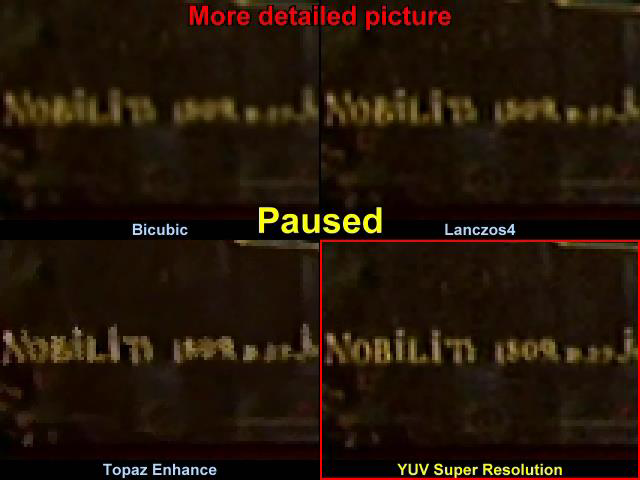
It can be seen that in the bicubic interpolation it is almost impossible to read the year, Lanczos4 , who is loved for great sharpness by those who semi-professionally change the video resolution, edges are clearer, of course, but it is still impossible to read the year. We do not comment on commercial Topaz, but we clearly read the inscription, and you can see that this is most likely the year 1809.
Findings:
- Thousands of researchers in the world are engaged in increasing the resolution, and millions of articles have been published on this topic. Due to this, every smartphone has a “digital zoom”, which is usually objectively better than the algorithms to increase conventional programs, and each FullHD TV can show SD video, often even without the characteristic resolution changing artifacts.
- Less than 10% of those involved in Super Resolution are involved in recovering a real image from video; moreover, most recovery algorithms are extremely slow (up to several days of calculations per frame).
- In most cases, recovery is designed to ensure that the high frequencies in the video are more or less saved, and therefore do not work on videos with significant compression artifacts. And since compression settings are often chosen in the video surveillance camera settings based on the desire to save more hours (i.e., the video is compressed harder and the high frequencies are “killed”), it becomes almost impossible to restore such video.
How SR looks in the industry
For the sake of fairness, we note that today all TV manufacturers have their own (or at least purchased) resolution increasing algorithms (all you need to do is to make HD images from SD on the fly), all smartphone manufacturers (what is called “digital zoom” in advertising) and .d We'll talk about the results of Google (and not only). Firstly, because Google describes the results on its blog very well and without much pathos and marketing, and this is extremely nice. Secondly, because smartphone manufacturers (for example, one very well-known Korean company) do not shy away from using, let's say, Photoshop in advertising their technologies (what a difference - people will swallow anyway) - and this is unpleasant. In general, let's talk about those who describe their technology fairly enough.
Back in 2016, Google publishedquite interesting results of the RAISR (Rapid and Accurate Image Super Resolution) algorithm used in the Pixel 2 smartphone. On the most successful pictures, the result looked just great:
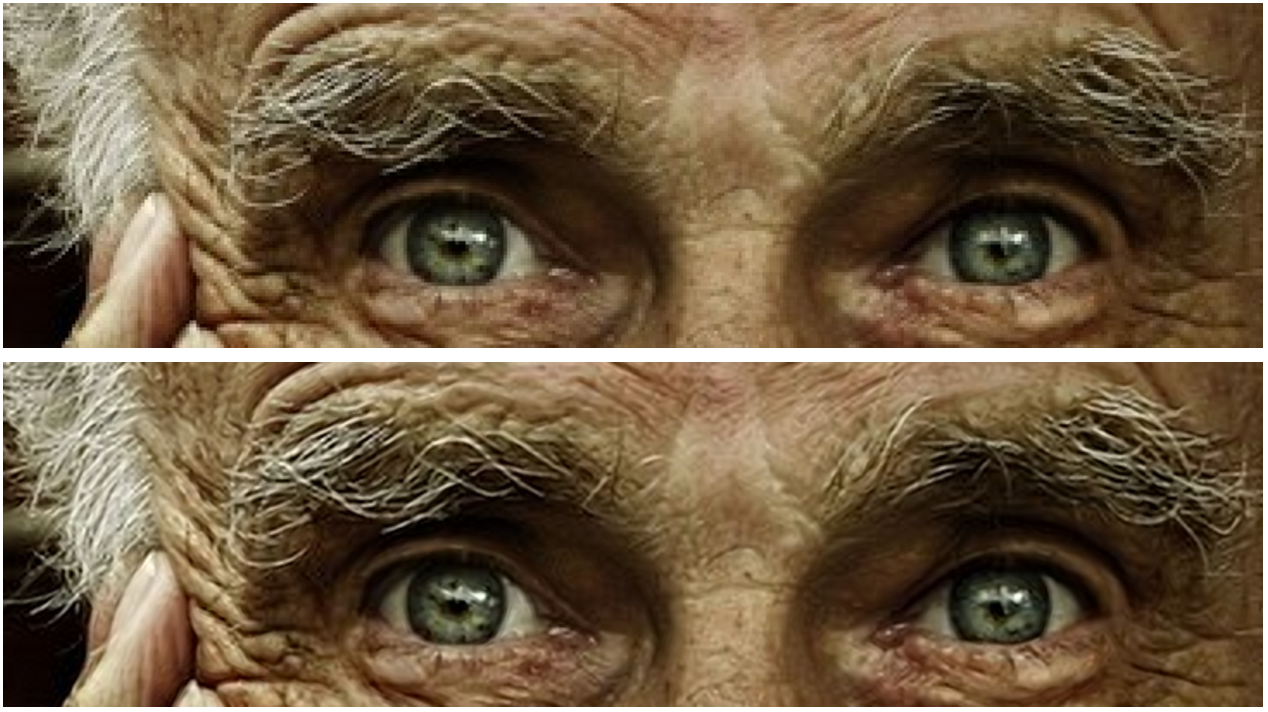
Source: AI blog Google
Algorithm was a set of filters used after the ML classification, and compared with bicubic interpolation (the traditional whipping boy), the result pleased:
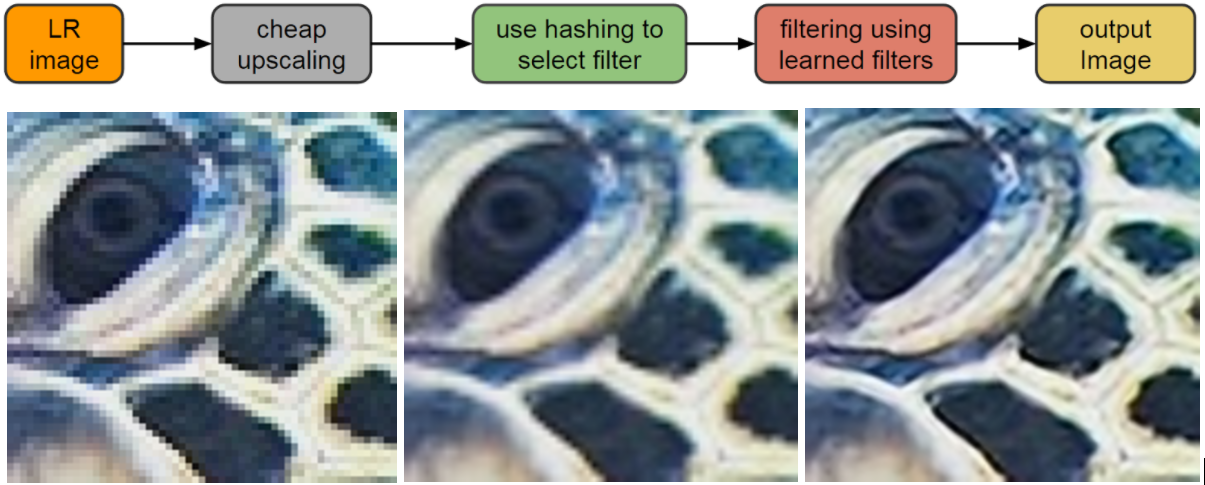
In order: the original, bicubic interpolation, RAISR
But it was a Single Frame Interpolation, and on the “unsuccessful” examples, like the foliage below, the picture became very unpleasantly distorted - after the increase, the photo became noticeably “synthetic”. It showed exactly the effect for which they do not like the digital zoom of modern smartphones:

Miracle, in fact, did not happen, and Google honestly and immediately published a counter-example, i.e. immediately set limits on the applicability of their approach and saved people from excessive expectations (typical of regular marketing).
However, in less than two years, the continuation of the work used in the Google Pixel 3 and dramatically improving the quality of its shooting, which is already an honest multi-frame Super Resolution, that is , was published . algorithm for restoring the resolution for several frames:

Source: AI Google Blog
The picture above shows a comparison of the results of Pixel 2 and Pixel 3, and the results look very good - the picture has really become much clearer and it can be clearly seen that this is not “thinking up”, but really restoring the details. And the attentive professional reader will have questions about the two vertical twin tubes on the left. The resolution has obviously grown, while the aliasing step (a sign of the real resolution) looks strangely close. What was it?
Briefly we will sort algorithm. Colleagues went from changing the interpolation of the Bayer pattern :

The fact is that 2/3 of the information in the real image is actually interpolated information. Those. Your picture is already blurred and “blurred”, but with a real noise level this is not so significant. By the way, the ability to use more complex interpolation algorithms has made popular the RAW maximum quality conversion programs for photos (the difference between the simple algorithm built into each camera and the complex algorithm of a specialized program is usually well noticeable when the image is enlarged).
Colleagues from Google use the fact that the vast majority of smartphone photos are hand-made, i.e. the camera will shake slightly:
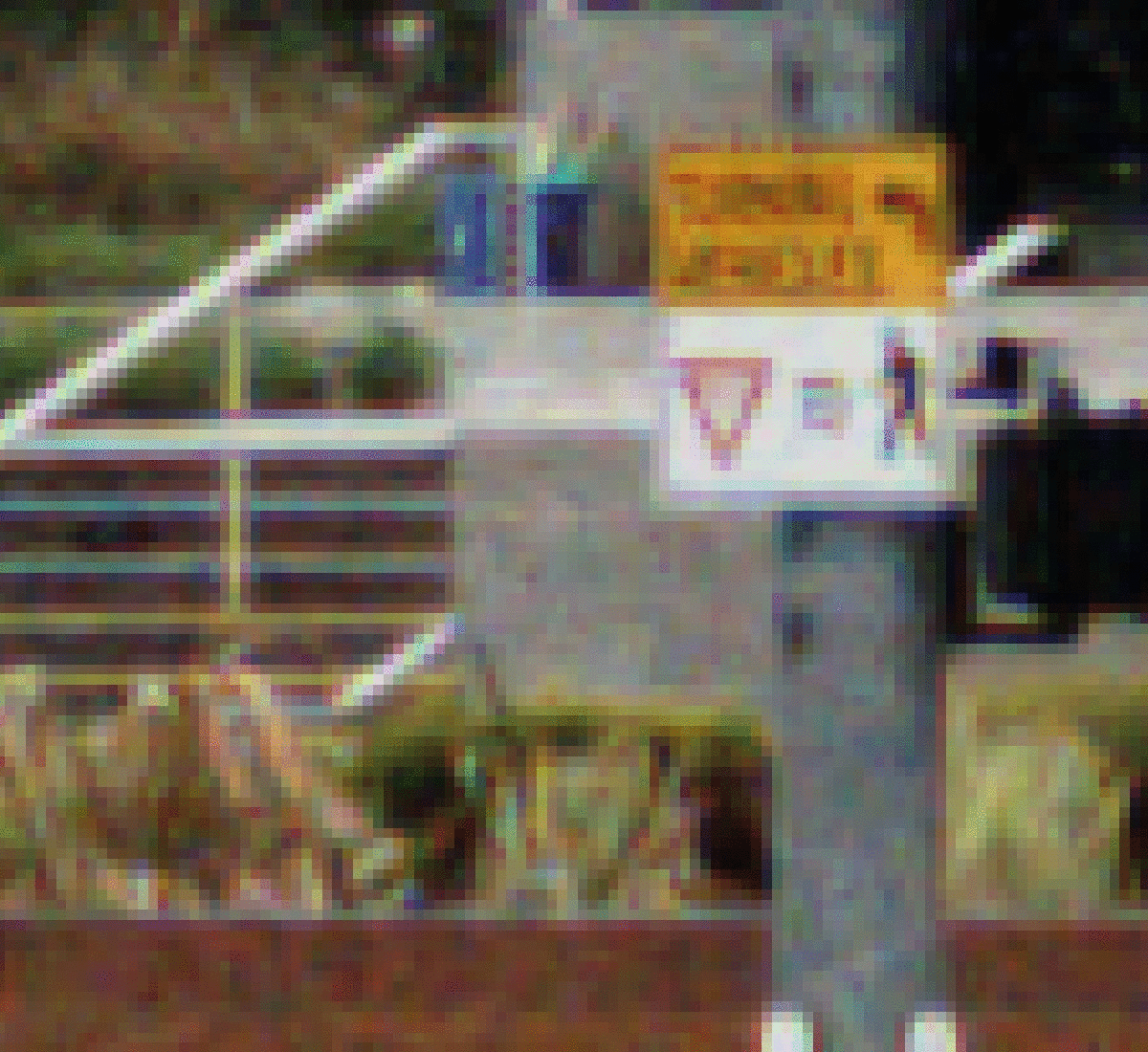
Source: AI Google Blog (multi-frame image aligned on pixel level to show subpixel shift)
As a result, if you take a few frames and estimate the shift (and the iron, which is able to build a quarter-pixel accuracy of motion estimation in any smartphone with H.264 support), we get a shift map. However, the animation above clearly shows that with a real noise level, the construction of a shift map with subpixel accuracy is a very nontrivial task, but over the past 20 years very good algorithms have appeared in this area. Of course, at times they have a hard time. For example, in the example above, something flashes on one frame at the top of the staircase handrail. And this is still a static scene, there are no moving objects, which sometimes do not just move, but rotate, change shape, move quickly, leaving large areas of discovery (the loop of which should not be visible after processing). The example below clearly shows

Source: AI Google Blog (recommended to click and see in high resolution)
Hard examples are flames, ripples, sun glares on water, etc. In general, even in the “simple” problem of determining the shift, there are many non-trivial moments that significantly complicate the life of the algorithm. However, now it's not about that.
Interestingly, even if the camera is completely stationary (for example, mounted on a tripod), the sensor can be made to move through the control of the optical stabilization module (OIS). As a result, we get the desired sub-pixel shifts. In Pixel 3, OIS support is implemented, and you can press the phone to the glass and observe with interest how OIS will begin to move the image on an ellipse (approximately, like this link), that is, even in this difficult case of fixing on a tripod, Super Resolution will be able to work out and raise the quality. However, the lion's share of shooting from smartphones is handheld shooting.
As a result, we have additional information for building a photo of higher resolution:
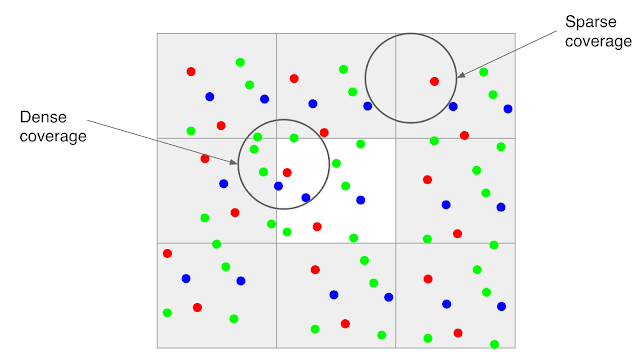
As mentioned above, a direct consequence of SR is a strong decrease in the noise level, in some cases - very tangible:

Source: AI Google Blog
Note that recovery also means recovery by the number of bits per component. Those. formally solving the problem of increasing the resolution, the same engine under certain conditions can not only suppress noise, but also turn the frame into HDR. It is clear that today, HDR is rarely used, but this, you see, is a good bonus.
The example below shows a comparison of images taken on Pixel 2 and Pixel 3 after SR with a comparable sensor quality. You can clearly see the difference in noise and the difference in clarity:

For those who like to look at the details, there is an album in which Google's Super Resolution (marketing name Super Res Zoom) can be assessed in all its glory in the spectrum of the image approximation scale on a smartphone (changing FoV ): As they modestly write, they brought the shooting quality of smartphones a step further to the quality of professional cameras. In fairness, we note that professional cameras are also not in place. Another thing is that with a smaller scale of sales the same technologies will cost more for the user. However, SR in professional cameras already appear. UPD: As an example (the last link is a comparison):

- Testing Sony's New Pixel Shift Feature in the a7R III , about increasing the resolution by 2 times in both dimensions (and the advertising video in which is beautifully drawn, as we magically shift exactly pixel in each direction as the Bayer pattern accumulates)
- Increasing the resolution in the Olympus E-M5 Mark II from 16 to 40 megapixels,
- An article describing Super Resolution in a Pentax K-1 camera ,
- Magnificent article: Pixel-Shift Shootout: Olympus vs. Pentax vs. Sony vs. Panasonic - a comparison of the increase in resolution Pentax K-1, Sony a7R III, Olympus OM-D E-M1 Mark II and Panasonic Lumix DC-G9. It is characteristic that the processing of moving objects, which was discussed above and which is very nontrivial, is only for Pentax K-1.
Findings:
- The Super Resolution algorithms today are acquired by all the companies whose products work professionally with video and photos, this is especially evident from the manufacturers of televisions and smartphones.
- Simple SR: Image Super Resolution - creates noticeable artifacts (unnatural picture), since it is not a recovery algorithm.
- The main bonuses of the recovery algorithms are noise reduction, clarification of details, “more honest” HDR, clearly visible higher quality pictures on large-diagonal TVs.
- All this splendor became possible due to a cardinal (approximately by 3 orders of magnitude in the number of operations) increase in the complexity of photo processing algorithms, or more precisely, one frame of video.
Yandex results
Since I’ll still ask in the comments, I’ll say a few words about Yandex, which last year published its version of Super Resolution:
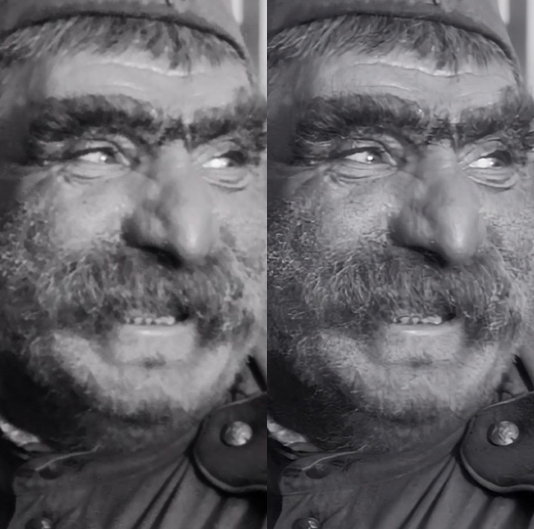
Source: https://yandex.ru/blog/company/oldfilms
And here are some examples on cartoons:
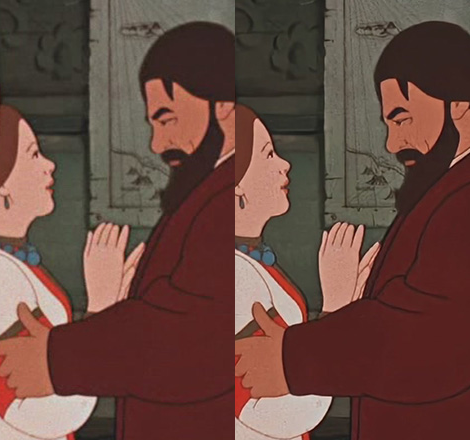
Source: https://yandex.ru/blog/company/soyuzmultfilm
What was it? Did Yandex repeat Google technology 2016 ?
On the technology description page from Yandex (marketing name DeepHD) there are links only to Image Super Resolution. This means that there are deliberately counterexamples on which the algorithm spoils the picture and they are found more often than for the algorithms of "fair" recovery. But about 80% of articles are devoted to the topic and the algorithm is easier to implement.
On Habré, this technology was also described(It is interesting that the author of the article is a graduate of our laboratory), but, as can be seen from the comments, the authors did not answer one of my questions, while others answered. And this is, rather, not the authors of the villains, but the company's policy (in other posts, if you look closely, there are also often no answers to the questions of specialists). For blogs of technology companies reluctance to go deeper into the discussion of the details of implementations or technologies is normal. Especially if this creates a better impression of the technology / product. Or competitors can cut the same thing faster. Again, marketing is responsible for the posts, and this is their direct work - creating a favorable impression of the company's products, regardless of the quality of the products themselves. From here frequent mistrust to the information proceeding from marketing.
In general, it is worth being very skeptical about the pictures of companies from the series “as we did everything to everyone well” for the following reasons:
- The authors of processing algorithms are well aware that there are practically no algorithms that in some cases would not generate artifacts. And, actually, one of the key tasks of the developer is to reduce the percentage of such cases (or the visibility of artifacts in such cases) while maintaining the quality in other cases. And very often this is NOT possible:
- Either the artifacts are so strong and difficult to fix, that the whole approach is rejected. Actually this is the case, perhaps (surprise-surprise!), Most articles. Divine pictures in some cases (which were sharpened) and "it does not work at all" in the rest.
- Or (and this is a common situation for practical technology companies) have to sacrifice a little quality on average in order to endure artifacts in the worst cases.
- Either the artifacts are so strong and difficult to fix, that the whole approach is rejected. Actually this is the case, perhaps (surprise-surprise!), Most articles. Divine pictures in some cases (which were sharpened) and "it does not work at all" in the rest.
- Another frequent example of misleading about the processing algorithms is the use of parameters (including internal parameters) of the algorithm. Algorithms, it so happened, have parameters, and users - and this is also the norm - like to have at most one “enable” button. And even if the settings are there, then the mass user does not use them. That is why companies when they buy the technology “stopyats” just ask again “Is this exactly a complete machine?” And ask for many examples.
- Accordingly, a frequent story is the publication of the result that was obtained with certain parameters. Benefit the developer knows them well, and even when they are under fifty (the real situation!), Very quickly selects them so that the picture was magic. Exactly these pictures often go into advertising.
- And the developer may even be against it. Marketing sees new examples sent and says “nothing is visible on them, in the last presentation you had normal examples!”. And then they can try to explain that the new examples are what people really see, and in the last presentation they showed potential results that can be achieved by preliminary studies of the beginning of the project. It doesn't bother anyone. People will receive the picture "where it is visible". In some cases, even large companies use "photoshop". Havat filed, gentlemen people! )
- Accordingly, a frequent story is the publication of the result that was obtained with certain parameters. Benefit the developer knows them well, and even when they are under fifty (the real situation!), Very quickly selects them so that the picture was magic. Exactly these pictures often go into advertising.
- In addition, if we are talking about video, it opens up huge spaces for the
colossus ...good marketing! For, as a rule, frames are laid out, and the quality of compressed video always oscillates and depends on the mass of parameters. Again - you can apply several technologies correctly, processing time, again, can be different. And that's not all, the space is great.- The Yandex ad states that DeepHD technology works in real time, so today you can watch TV channels using it . It was explained above that the speed of work is the Achilles' heel of Super Resolution. The advantage of neural networks, of course, is that while studying for a long time, they can work in some cases very quickly, but I would still look (with a huge professional interest) in what resolution and with what quality the algorithm works in real time. Usually, several modifications of the algorithm are created and at high resolutions with real-time, many “chips” (critical for quality) have to be disabled. Very many.
- In black and white examples, upon careful examination, it is clear that the local brightness is changing. Since the correct SR does not change the brightness, it seems that some other algorithm worked, perhaps more than one (the results show that this is not Single Frame Processing, it seems more accurate not only). If you look at a larger piece (at least 100 frames), the picture will be clear. However, the measurement of video quality is a separate, very big topic.
- The Yandex ad states that DeepHD technology works in real time, so today you can watch TV channels using it . It was explained above that the speed of work is the Achilles' heel of Super Resolution. The advantage of neural networks, of course, is that while studying for a long time, they can work in some cases very quickly, but I would still look (with a huge professional interest) in what resolution and with what quality the algorithm works in real time. Usually, several modifications of the algorithm are created and at high resolutions with real-time, many “chips” (critical for quality) have to be disabled. Very many.
Findings:
- It should be understood that marketers often use their techniques exactly because it works (and how!). The vast majority of people
do not read Habrdo not want to deeply understand the topic and do not even seek the opinion of an expert, they have enough advertising (sometimes - golima advertising). That regularly leads to all sorts of imbalances. I wish everyone to spend less on advertising, especially when storytelling is up to par and will really want to believe in a miracle!- And, of course, it’s very good that in Yandex they also work on the topic and make their SR (more precisely, their SR family).
Perspectives
Let's get back to where we started. What to do to those who want to increase compressed video? Is this really all bad?
As described above, the algorithms of "fair" recovery is critical even a weak image change in the field, literally at the level of noise. That is, high frequencies in the image and their change between frames are critical.
The main thing, due to which video compression is performed - is the removal of interframe noise. In the example below, the interframe difference of noisy video before motion compensation, after compensation (with low compression) and after appreciable compression - feel the difference (the contrast is raised about 6 times so that details can be seen):
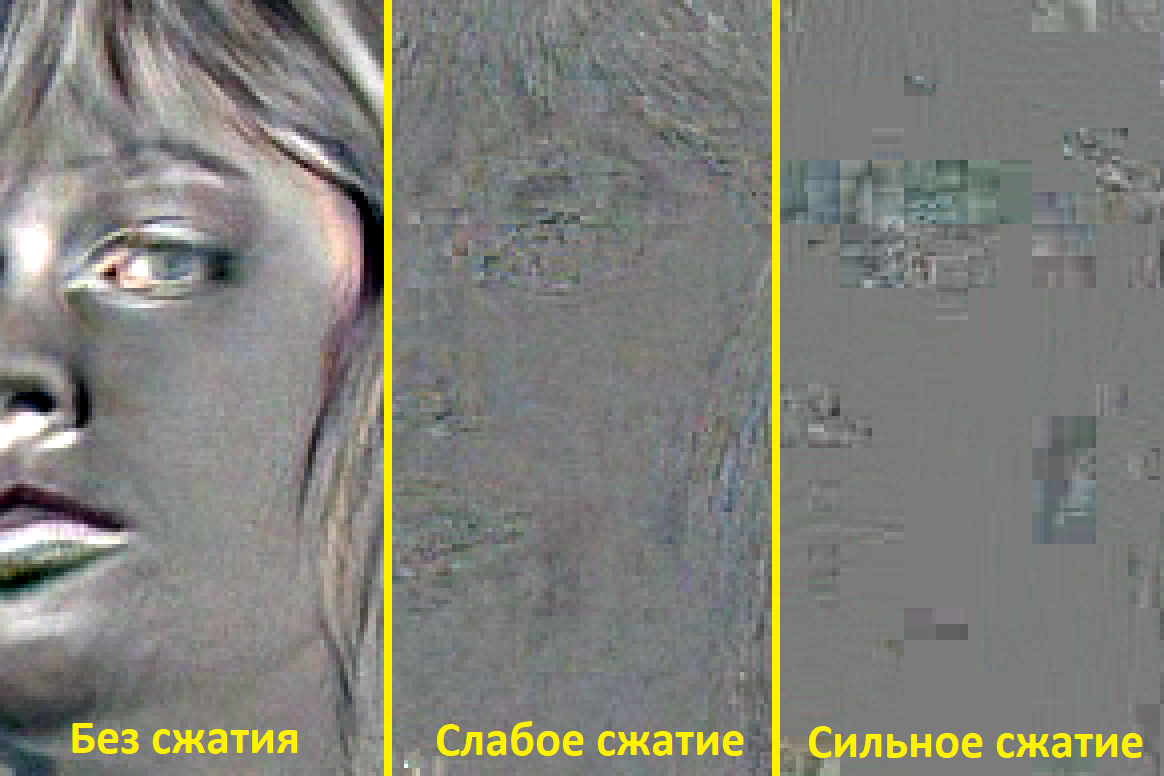
Source: author's lectures on compression algorithms
It is clearly seen that from the point of view of the codec, an ideal area is an area in which movement has been fully compensated and for which no more bits are needed. Well, a little bit can be spent, something minimal to fix. And such areas can be quite a lot. Therefore, Super Resolution loses its “main bread” - information that is located in this place in other frames, taking into account the subpixel shift.
If you look at the articles, even for a relatively simple JPEG on jpeg restoration there are 26 thousand results, and for jpeg recovery - 52 thousand, and this, together with the recovery of broken files, etc. For video, the situation is worse than mpeg restoration- 22 thousand, i.e. Of course, work is underway, but the scale of work on Super Resolution is not comparable. The work is about an order of magnitude less than the restoration of video resolution and two orders less than the Image Super Resolution. Two orders is a lot. We also approached the projectile (since we have been doing compression and processing for a long time), there is something to work with, especially if the quality oscillates or something like M-JPEG is used (more recently, a frequent picture in video surveillance). But it will all be special cases.
The results of the articles on the links above also show that the results are sometimes very beautiful, but obtained for very special cases. Those. Alas, this function will not appear in every smartphone tomorrow. This is bad news. Good - the day after tomorrow and on a computer with a good GPU - will appear exactly.
The reasons:
- Gradually, accumulation devices become cheaper (SD cards for recorders, drives for surveillance cameras, etc.) and the average bit rate of video storage increases.
- Also, during compression, they gradually transfer to the standards of the next generations (for example, to HEVC), which means a noticeable improvement in quality at the same bitrate. The last 2 points mean that gradually the quality of the video will be higher, and from some point on, well-developed Video Super Resolution algorithms will start working.
- Finally, algorithms are being improved. The achievements of machine learning based algorithms for the last 4 years are especially good. In this regard, with high probability we can expect something like this:
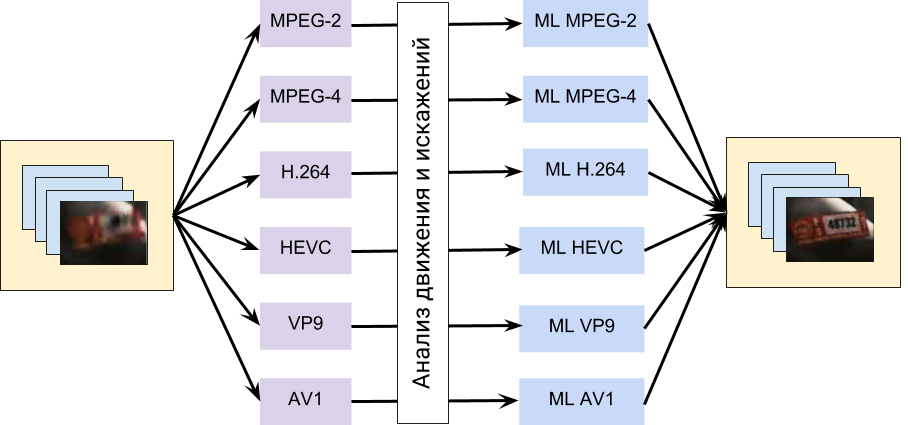
Those. the algorithm will explicitly use the traffic information obtained from the codec, and then these data will be fed to the neural network trained to recover artifacts characteristic of specific codecs. Such a scheme looks at the moment quite achievable.
But in any case, you need to clearly understand that the current recovery is, as a rule, a 2-fold increase in resolution. Less often, in some cases, when the source material is not compressed or almost not compressed, it can be about 3-4 times. As you can see, this is not even close 100-1000 times magnification from movies, when 1.5 pixels of a night record killed by noises turn into an excellent quality car number. The science fiction genre should be appropriated in fact to a larger percentage of films and TV shows.
And, of course, there will be attempts to make something universal, within the framework of the fashion trend “the main thing is to gash more layers”. And here it is worth warning the reaction to advertising materials on this topic against “hurray-hurray”. For the neural network is the most convenient framework for the demonstration of miracles and all sorts of speculations. The main thing is to correctly select the training sample and the final examples. And voila! See the miracle! Very convenient in terms of hilling investors, by the way. That is, it is extremely important that the performance of technologies is confirmed by someone independent on a large number of heterogeneous examples, which is demonstrated extremely rarely. For companies, even to give one or two examples when the technology does not work is today equivalent to a civil feat.
Well, so that life does not seem like honey - let me remind you that so-called transcoding is popular today, when in fact you have to work with a video that was originally shook by some algorithm, and then squeezed by another, while using other motion vectors, the high frequencies, etc. And the fact that a person sees everything well there does not mean that it will actually work wonders for the algorithm processing such a video. It will not be possible to restore the highly compressed videos soon, although in general Super Resolution will develop rapidly in the next 10 years.
Findings:At the end of last year we made a lecture on “Neural networks in video processing - myths and reality”. Perhaps we will get her here to put.
- Remember that what you see in movies and how it is in real life is very different. And not only in terms of restoring heavily compressed video!
- Usually, modern algorithms increase resolutions by 2 times, less often - slightly more, i.e. No 50 times, familiar to the movies, will not have to wait soon.
- The Super Resolution area is booming and we can expect active development of Video Restoration in the coming years, including recovery after compression.
- But the first thing we will see is all sorts of speculations on the topic, when the demonstrated results will greatly exaggerate the real capabilities of the algorithms. Be careful!
Stay tuned!
Thanks
I would like to sincerely thank:
- Laboratory of Computer Graphics VMK MSU. MV Lomonosov for computing power and not only,
- our colleagues from the video group, thanks to which the algorithms presented above were created, and especially by Karen Simonyan, the author of the article, whose results were demonstrated above and which now works in Google DeepMind,
- personally Konstantin Kozhemyakov, who did a lot to make this article better and clearer,
- Google for a great blog and relatively correct descriptions of the created technologies and Yandex for the fact that they are on a broad front very well compete with Google - almost the only successful example in a country where Google services are not prohibited,
- Habrovchan denisshabr , JamboJet and iMADik for guidance and links to SR multi-frame professional cameras,
- and, finally, many thanks to Vyacheslav Napadovsky, Evgeny Kuptsov, Stanislav Grokholsky, Ivan Molodetsky, Alexei Solovyov, Evgeny Lyapustin, Egor Sklyarov, Denis Kondranin, Alexandra Anzina, Roman Kazantsev and Gleb Ishelev, for the large number of patterns and patterns, on the number of hands, and on the numbers). it is better!