Neurobiology and Artificial Intelligence: Part Three - Data Presentation and Memory
To be continued.
Chapter 1: Neurobiology and artificial intelligence: part one - educational program.
Chapter 1.5: Neurobiology and Artificial Intelligence: Part One and a Half - News from the Blue Brain Project.
Chapter 2: Neurobiology and artificial intelligence: part two - intelligence and the presentation of information in the brain.
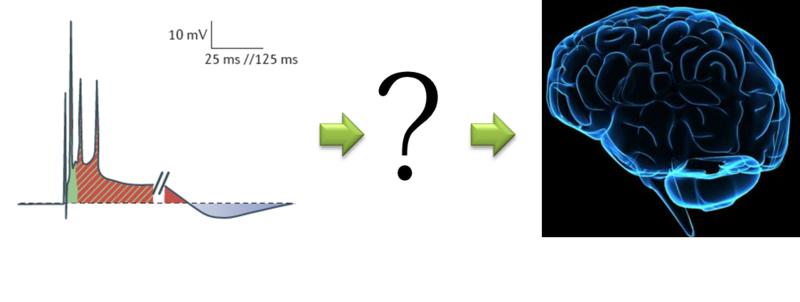
Fig. 1
Well, common truths are over. Now we turn to controversial things.
I will not describe how important memory is for any information processing system. However, with human memory, everything is very difficult. In principle, we found out that there are signals that travel through neurons, there are excitatory and inhibitory synapses that control the passage of signals, there are neuromodulators that change the sensitivity of synapses to signals, but how does it all work together, which ultimately results in meaningful cognitive activity? It is far from a fact that if you assemble such a system with millions of neurons, it will work adequately, and not like, say, an epileptic.
This gives rise to speculate about alternative theories of consciousness, in which they take some little-known effect and declare it a panacea. One of these theories was put forward by R. Penrose and S. Hameroff - the quantum theory of consciousness, based on the theoretical premises for the quantum interaction of tubulin microtubules that make up the cytoskeleton of neurons. If there are people willing, I can discuss this theory in a separate chapter, but for now let us return to more proven theories.
So, before embarking on a man with his hundred billion neurons, it would be nice to see what happens in simpler cases. And in the simplest case, when the memory effect can be defined as “a change in the reaction to repeated irritation”, it can be observed on the example of the nervous system of C. elegans worms, which has 302 neurons and 5,000 synapses. Despite such a rudimentary nervous system, C. elegans have developed the ability to feel temperature with an accuracy of 0.1 ° C and can learn to associate it with the amount of food available, thereby giving us the opportunity to argue that the mechanisms of associative memory can work with a small number of neurons. The simplicity of the neural system, in turn, helped scientists to study in detail the mechanisms responsible for the ability to associate. In this case, it was found out that the amount of Ce-NCS-1 calcium-binding protein produced is directly proportional to learning ability. Moreover, this protein is produced both in neurons directly sensing temperature and in signal-conducting neurons (insertion, intermediate neuron, interneuron), and directly affects the properties of synapses between them. Insertion neurons already collect and integrate information from temperature and olfactory neurons. An interesting thing here is that this protein belongs to the group of calcium-sensitive proteins that can be found everywhere, from yeast to humans [1]. and directly affects the properties of the synapses between them. Insertion neurons already collect and integrate information from temperature and olfactory neurons. An interesting thing here is that this protein belongs to the group of calcium-sensitive proteins that can be found everywhere, from yeast to humans [1]. and directly affects the properties of the synapses between them. Insertion neurons already collect and integrate information from temperature and olfactory neurons. An interesting thing here is that this protein belongs to the group of calcium-sensitive proteins that can be found everywhere, from yeast to humans [1].
More complex mechanisms, such as the formation of conditioned and unconditioned reflexes, are studied, for example, on Drosophila flies, mainly by turning off certain genes. For example, flies with dopamine and serotonin expression turned off refused to absorb new associative information. If the expression was simply reduced, the degree of learning depends on the number of these neurotransmitters. It is important to note that if such flies were able to be trained in something, then they no longer forgot it. It turns out that these neutrotransmitters are responsible for learning rather than memory.
An important aspect is that Drosophila already has a division into short-term and long-term memory, for which different areas of the brain are responsible. In particular, you can grow flies that will remember the associative rules for only a few seconds. The division into short-term and long-term memory comes from the dynamic nature of neurons (they communicate with patterns of spikes), the need to maintain a certain activity for a certain period of time (so that information from different parts of the brain has time to integrate), and the need to store the resulting experience (but he already unlikely to be stored as spike patterns). For the theory of (artificial) intellect, such a separation, generally speaking, is not necessary: if we already know how to represent and process information, then we already imagine how to store it on a computer. Plus, I have not yet encountered studies showing the processing of knowledge in long-term memory without involving short-term memory (although the processing itself takes place, for example, in cases where we recall something from the past. Then, by the way, long-term memory, generally speaking, overwritten again, and therefore, if you often remember something, then over time it becomes difficult to separate reality from speculation).
Therefore, further I will mainly talk about short-term memory. It, in turn, can be divided into “instant” (immediate memory), which has a large volume, but covering the time range from fractions of a second to several seconds, and working (working memory) - covering from several seconds to several minutes. Studies on monkeys have shown that stimulus information can be maintained by continuous activation of neurons for several seconds after the stimulus itself disappears, and there are models of neural networks that successfully model such processes [2]. The general rule for storing information can be described as follows: the longer the memory, the less detailed information about the stimulus is stored in it and the more abstract, conceptualized.wood of internal structures, such as the hippocampus (through which, by the way, there is a consolidation of short-term memory into long-term).
What molecular mechanisms can be used to implement short-term memory? Short-term synaptic plasticity is manifested as attenuation or amplification of the next signal after the previous one. Usually, if 2 signals arrive quickly (<20 ms), then the second one is attenuated due to a local decrease in the number of bubbles with a neurotransmitter or inactivation of voltage-dependent proteins. If between two signals passes 20-500 ms, then the second signal often produces a greater effect. In the case of weak synapses, one signal may not be enough to depolarize the post-synaptic membrane, and the second signal, as it were, will use the released neurotransmitters from the first as a launching pad, increasing the likelihood of successful signal transmission. Longer effects are also observed, such as strengthening (facilitation) of synaptic communication (divided into high-speed - about 10 ms and long - hundreds of ms), post-convulsive potentiation (post-tetanic potentiation) - up to several minutes, as well as weakening (depression), associated with the depletion of neurotransmitters , and with the effect of the accumulating number of mediators inside the synapse (homosynaptic) and / or coming from neighboring synapses (heterosynaptic). Together, these processes may be responsible for the localization of excitation patterns in a certain area: some do not allow patterns to creep along the neural network, while others maintain the activity of the pattern. associated both with the depletion of neurotransmitters, and with the effect of the accumulating number of mediators inside the synapse (homosynaptic) and / or coming from neighboring synapses (heterosynaptic). Together, these processes may be responsible for the localization of excitation patterns in a certain area: some do not allow patterns to creep along the neural network, while others maintain the activity of the pattern. associated both with the depletion of neurotransmitters, and with the effect of the accumulating number of mediators inside the synapse (homosynaptic) and / or coming from neighboring synapses (heterosynaptic). Together, these processes may be responsible for the localization of excitation patterns in a certain area: some do not allow patterns to creep along the neural network, while others maintain the activity of the pattern.

Fig. 2. Activation of place neurons depending on the position in the maze (the activity of different neurons is shown in different colors).
The importance of the possibility of localizing excitation patterns could already be estimated in the previous chapter from Fig. 6. It is interesting that in the hippocampus (and not only) of rats (and not only), a structure not directly connected with the visual cortex, one can distinguish such highly specialized cells that are activated if the rat is already visually familiar with the site [5] (Fig. 2 ) Moreover, their activity depends little, for example, on the illumination of the area, i.e. they are more likely connected with the current view of the rat about the place of stay, rather than with direct observation of it. Such cells are called “place cell” - place cells. So in such cells, excitation is maintained throughout the entire time that the rat is in the appropriate place. And since such cell behavior was seen not only in the hippocampus, theories are being put forward,
I would also like to note that “all roads lead to the hippocampus,” and despite the fact that not all types of memory are tied to the hippocampus (for example, you can learn to play musical instruments with a damaged hippocampus), it plays an important role in the formation of memory, and in the integration of current visual information and related information in working memory.

Fig. 3. The interaction of various brain structures to coordinate movement.
Something like that.
From here follow the principles laid down in artificial intelligent systems: associativity and content-addressability for memory models, as well as training opportunities, classification, planning, methods for solving other problems. Many questions remain about data reporting. Jeff Hawkins, who in particular invented Palm and its handhelds, proposes to model the memory and presentation of information in it using the hierarchy temporal model, which has already been described here and which follows directly from the structure of the neocortex. Although with all the undoubtedly interesting ideas, so far no one has managed to make a strong AI. So the truth is somewhere nearby.
[1] - Elements of Molecular Neurobiology, 3d ed., C. Smith, 2002
[2] - A Spiking Network Model of Short-Term Active Memory, D. Zipser et al., The Journal of Neuroscience, 1993, 13 (8), 3406-3420
[3] - Neuroscience, 3d ed., D. Purves 2004
[4] - The Prefrontal Cortex as a Model System to Understand Representation and Processing of Information, Representation and Brain, S. Funahashi, 2006, XII, 311-366.
[5] - A Neural Systems Analysis of Adaptive Navigation, S. Mizumori et al., Molecular Neurobiology, 2000, 21, 57-82
Chapter 1: Neurobiology and artificial intelligence: part one - educational program.
Chapter 1.5: Neurobiology and Artificial Intelligence: Part One and a Half - News from the Blue Brain Project.
Chapter 2: Neurobiology and artificial intelligence: part two - intelligence and the presentation of information in the brain.
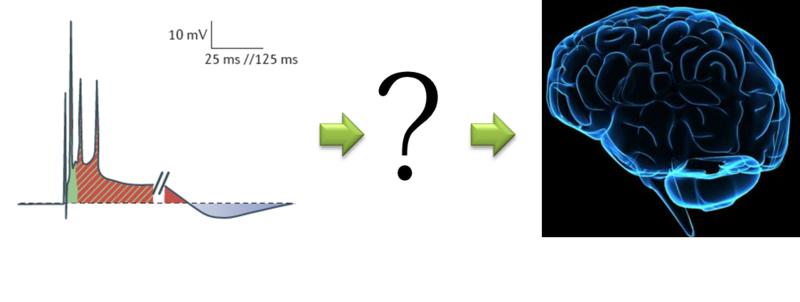
Fig. 1
Well, common truths are over. Now we turn to controversial things.
I will not describe how important memory is for any information processing system. However, with human memory, everything is very difficult. In principle, we found out that there are signals that travel through neurons, there are excitatory and inhibitory synapses that control the passage of signals, there are neuromodulators that change the sensitivity of synapses to signals, but how does it all work together, which ultimately results in meaningful cognitive activity? It is far from a fact that if you assemble such a system with millions of neurons, it will work adequately, and not like, say, an epileptic.
This gives rise to speculate about alternative theories of consciousness, in which they take some little-known effect and declare it a panacea. One of these theories was put forward by R. Penrose and S. Hameroff - the quantum theory of consciousness, based on the theoretical premises for the quantum interaction of tubulin microtubules that make up the cytoskeleton of neurons. If there are people willing, I can discuss this theory in a separate chapter, but for now let us return to more proven theories.
So, before embarking on a man with his hundred billion neurons, it would be nice to see what happens in simpler cases. And in the simplest case, when the memory effect can be defined as “a change in the reaction to repeated irritation”, it can be observed on the example of the nervous system of C. elegans worms, which has 302 neurons and 5,000 synapses. Despite such a rudimentary nervous system, C. elegans have developed the ability to feel temperature with an accuracy of 0.1 ° C and can learn to associate it with the amount of food available, thereby giving us the opportunity to argue that the mechanisms of associative memory can work with a small number of neurons. The simplicity of the neural system, in turn, helped scientists to study in detail the mechanisms responsible for the ability to associate. In this case, it was found out that the amount of Ce-NCS-1 calcium-binding protein produced is directly proportional to learning ability. Moreover, this protein is produced both in neurons directly sensing temperature and in signal-conducting neurons (insertion, intermediate neuron, interneuron), and directly affects the properties of synapses between them. Insertion neurons already collect and integrate information from temperature and olfactory neurons. An interesting thing here is that this protein belongs to the group of calcium-sensitive proteins that can be found everywhere, from yeast to humans [1]. and directly affects the properties of the synapses between them. Insertion neurons already collect and integrate information from temperature and olfactory neurons. An interesting thing here is that this protein belongs to the group of calcium-sensitive proteins that can be found everywhere, from yeast to humans [1]. and directly affects the properties of the synapses between them. Insertion neurons already collect and integrate information from temperature and olfactory neurons. An interesting thing here is that this protein belongs to the group of calcium-sensitive proteins that can be found everywhere, from yeast to humans [1].
More complex mechanisms, such as the formation of conditioned and unconditioned reflexes, are studied, for example, on Drosophila flies, mainly by turning off certain genes. For example, flies with dopamine and serotonin expression turned off refused to absorb new associative information. If the expression was simply reduced, the degree of learning depends on the number of these neurotransmitters. It is important to note that if such flies were able to be trained in something, then they no longer forgot it. It turns out that these neutrotransmitters are responsible for learning rather than memory.
An important aspect is that Drosophila already has a division into short-term and long-term memory, for which different areas of the brain are responsible. In particular, you can grow flies that will remember the associative rules for only a few seconds. The division into short-term and long-term memory comes from the dynamic nature of neurons (they communicate with patterns of spikes), the need to maintain a certain activity for a certain period of time (so that information from different parts of the brain has time to integrate), and the need to store the resulting experience (but he already unlikely to be stored as spike patterns). For the theory of (artificial) intellect, such a separation, generally speaking, is not necessary: if we already know how to represent and process information, then we already imagine how to store it on a computer. Plus, I have not yet encountered studies showing the processing of knowledge in long-term memory without involving short-term memory (although the processing itself takes place, for example, in cases where we recall something from the past. Then, by the way, long-term memory, generally speaking, overwritten again, and therefore, if you often remember something, then over time it becomes difficult to separate reality from speculation).
Therefore, further I will mainly talk about short-term memory. It, in turn, can be divided into “instant” (immediate memory), which has a large volume, but covering the time range from fractions of a second to several seconds, and working (working memory) - covering from several seconds to several minutes. Studies on monkeys have shown that stimulus information can be maintained by continuous activation of neurons for several seconds after the stimulus itself disappears, and there are models of neural networks that successfully model such processes [2]. The general rule for storing information can be described as follows: the longer the memory, the less detailed information about the stimulus is stored in it and the more abstract, conceptualized.
What molecular mechanisms can be used to implement short-term memory? Short-term synaptic plasticity is manifested as attenuation or amplification of the next signal after the previous one. Usually, if 2 signals arrive quickly (<20 ms), then the second one is attenuated due to a local decrease in the number of bubbles with a neurotransmitter or inactivation of voltage-dependent proteins. If between two signals passes 20-500 ms, then the second signal often produces a greater effect. In the case of weak synapses, one signal may not be enough to depolarize the post-synaptic membrane, and the second signal, as it were, will use the released neurotransmitters from the first as a launching pad, increasing the likelihood of successful signal transmission. Longer effects are also observed, such as strengthening (facilitation) of synaptic communication (divided into high-speed - about 10 ms and long - hundreds of ms), post-convulsive potentiation (post-tetanic potentiation) - up to several minutes, as well as weakening (depression), associated with the depletion of neurotransmitters , and with the effect of the accumulating number of mediators inside the synapse (homosynaptic) and / or coming from neighboring synapses (heterosynaptic). Together, these processes may be responsible for the localization of excitation patterns in a certain area: some do not allow patterns to creep along the neural network, while others maintain the activity of the pattern. associated both with the depletion of neurotransmitters, and with the effect of the accumulating number of mediators inside the synapse (homosynaptic) and / or coming from neighboring synapses (heterosynaptic). Together, these processes may be responsible for the localization of excitation patterns in a certain area: some do not allow patterns to creep along the neural network, while others maintain the activity of the pattern. associated both with the depletion of neurotransmitters, and with the effect of the accumulating number of mediators inside the synapse (homosynaptic) and / or coming from neighboring synapses (heterosynaptic). Together, these processes may be responsible for the localization of excitation patterns in a certain area: some do not allow patterns to creep along the neural network, while others maintain the activity of the pattern.

Fig. 2. Activation of place neurons depending on the position in the maze (the activity of different neurons is shown in different colors).
The importance of the possibility of localizing excitation patterns could already be estimated in the previous chapter from Fig. 6. It is interesting that in the hippocampus (and not only) of rats (and not only), a structure not directly connected with the visual cortex, one can distinguish such highly specialized cells that are activated if the rat is already visually familiar with the site [5] (Fig. 2 ) Moreover, their activity depends little, for example, on the illumination of the area, i.e. they are more likely connected with the current view of the rat about the place of stay, rather than with direct observation of it. Such cells are called “place cell” - place cells. So in such cells, excitation is maintained throughout the entire time that the rat is in the appropriate place. And since such cell behavior was seen not only in the hippocampus, theories are being put forward,
I would also like to note that “all roads lead to the hippocampus,” and despite the fact that not all types of memory are tied to the hippocampus (for example, you can learn to play musical instruments with a damaged hippocampus), it plays an important role in the formation of memory, and in the integration of current visual information and related information in working memory.

Fig. 3. The interaction of various brain structures to coordinate movement.
Something like that.
From here follow the principles laid down in artificial intelligent systems: associativity and content-addressability for memory models, as well as training opportunities, classification, planning, methods for solving other problems. Many questions remain about data reporting. Jeff Hawkins, who in particular invented Palm and its handhelds, proposes to model the memory and presentation of information in it using the hierarchy temporal model, which has already been described here and which follows directly from the structure of the neocortex. Although with all the undoubtedly interesting ideas, so far no one has managed to make a strong AI. So the truth is somewhere nearby.
[1] - Elements of Molecular Neurobiology, 3d ed., C. Smith, 2002
[2] - A Spiking Network Model of Short-Term Active Memory, D. Zipser et al., The Journal of Neuroscience, 1993, 13 (8), 3406-3420
[3] - Neuroscience, 3d ed., D. Purves 2004
[4] - The Prefrontal Cortex as a Model System to Understand Representation and Processing of Information, Representation and Brain, S. Funahashi, 2006, XII, 311-366.
[5] - A Neural Systems Analysis of Adaptive Navigation, S. Mizumori et al., Molecular Neurobiology, 2000, 21, 57-82