Theft schemes in RBS systems and five levels of counteraction to them
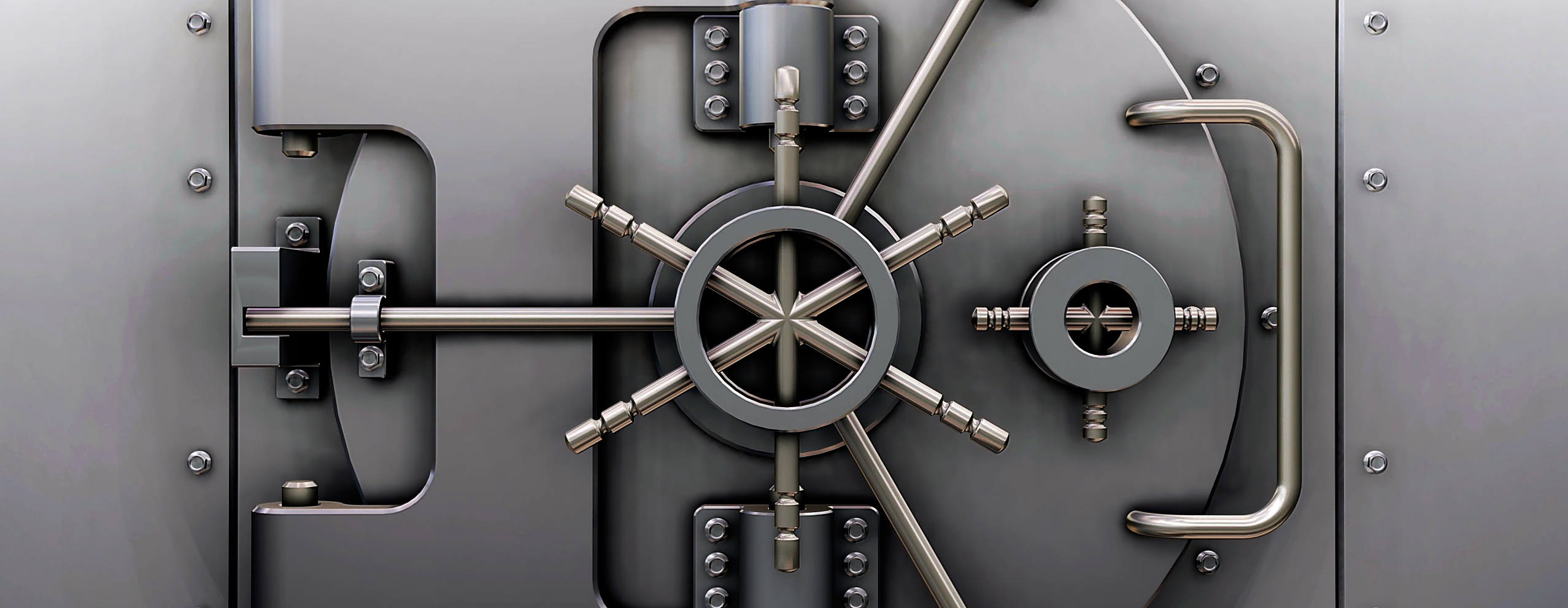
In May of this year, the K Department of the Ministry of Internal Affairs of Russia, with the assistance of Group-IB, detained a 32-year-old resident of the Volgograd Region accused of embezzlement of money from customers of Russian banks using a fake Internet banking application, which in fact turned out to be a malicious program - Android a trojan. Every day, with its help, from 100,000 to 500,000 rubles were stolen from users, part of the stolen money for further cashing out and hiding fraudulent activity was transferred to cryptocurrency.
Analyzing the “digital traces” of thefts committed, Group-IB specialists found that the banking trojan used in the criminal scheme was disguised as the “Banks in the palm” financial application, which acts as an “aggregator” of mobile systems
banking of the leading banks of the country. It was possible to download all your bank cards to the application, so as not to carry them with you, but at the same time be able to view the card balance based on incoming SMS for all transactions, transfer money from card to card, pay for online services and purchases in online stores .
Interested in the capabilities of the financial aggregator, bank customers downloaded the Banks in the Palm app and entered their card details. The launched trojan sent bank card data or logins / passwords for entering the Internet bank to the server for attackers. After that, the criminals transferred money to pre-prepared bank accounts in amounts from 12,000 to 30,000 rubles. for one transfer, entering the SMS-code of the operation confirmation intercepted from the victim’s phone. The users themselves did not suspect that they were victims of cybercriminals - all SMS confirmations of transactions were blocked.
Text: Pavel Krylov, Head of Product Development,
Secure Bank Group-IB
At the moment, the “market” of banking Android Trojans is the most dynamic and fastest growing. According to the Group-IB report for 2017, the damage from malware on Android OS in Russia grew by 136% compared to the previous reporting period - it amounted to $ 13.7 million. This figure covers the damage from trojans for personal computers by 30%.The main theft schemes through RBS: why antiviruses do not work
Group-IB forensics identify seven common theft schemes that are used by cybercriminals in attacks on remote banking systems:
1) social engineering;
2) transfers from card to card;
3) transfers through online banking;
4) interception of access to mobile banking;
5) fake mobile banking;
6) purchases using Apple Pay and Google Pay;
7) theft through SMS banking.
The usual antivirus protection tools are practically useless against such theft schemes. For example, in the case of using social engineering, when a client who got caught, believed a fraudster and transferred money to his account on his own (ie, “hacked” the person himself), or when the victim’s details were removed from a third-party device, antiviruses do not help.
The last echelon of defense is antifraud. Most anti-fraud systems are focused on the analysis of transactional information or data that goes directly to the bank’s servers (client’s IP address, information about his browser, pace of work in a web or mobile application, etc.). Given that fraud as such is a certain process that includes not only the moment of the transaction, but also the stages of preparation and
Withdrawal of funds, it becomes obvious that transactional antifraud systems "close" only a limited range of scammers.
Gartner analysts identify five levels of fraud prevention. We will analyze each of them in detail and with examples:
1) analysis of the user's device (infected devices, device identification);
2) monitoring user actions at the session level (anomalies in the user's work: navigation, time, geography);
3) behavioral analysis of the user in a particular channel (what actions are performed in the channel? How (behavior)? Who performs them?);
4) cross-channel analysis of users and their behavior (analysis of user behavior in different channels, data correlation);
5) analysis of the relationship between users and accounts (behavior on different resources, global client profile).
Level one: analyze the user's device
This level of fraud prevention includes all endpoint protection technologies, such as antiviruses, tokens for generating electronic signatures, two-factor authentication tools, additional device identification tools, etc. Biometric means of identification by voice, fingerprint or face belong to the same level.
One of the striking examples of attacks on remote banking systems was the activity of the Lurk group, which at the end of its “career” in 2016 reached a rather impressive scale: about 50 people worked for it. Having started her career with auto-loading for desktop versions of RBS (“thick” client), at the end of 2014 she implemented this theft method for Internet banks (“thin” clients that do not require installation on a user's PC), which significantly expanded the scope group activities. The client formed the payment with only the details of the recipient, but this payment was received by the bank already with the details of the Trojan program changed. Many experts still recognize this type of attack as the most dangerous, since it allows you to manipulate its behavior and data at the thinnest level directly from the client’s device. How did this happen?
The Trojan was injected directly into the client’s browser and made changes to its code directly in the memory, which made it possible for a client to visit the bank’s official website to intercept and modify the original HTML pages in a way necessary for a fraudster even when using an HTTPS connection.
In fig. Figure 1 shows an example of a malicious injection that was added by a trojan to the code of pages. The lines highlighted in gray are the URLs by which this script interacted with the fraudster's command servers, where logins / passwords, balances and other information were sent, and the fraudster's payment details were sent in response to substitute in the client’s original payment.

Fig. 1 Script introduced by the trojan to the original page of the Internet bank in the client’s browser.
A less sophisticated but effective way to steal money is to remotely control a client’s device (Fig. 2). After automated collection with the help of a keylogger program (literally, “key interceptor”) of all the necessary logins / passwords, PIN from tokens, etc. The fraudster, through remote control, connects to the client’s device (Fig. 3) and creates a fraudulent payment directly from and on behalf of the client’s device.
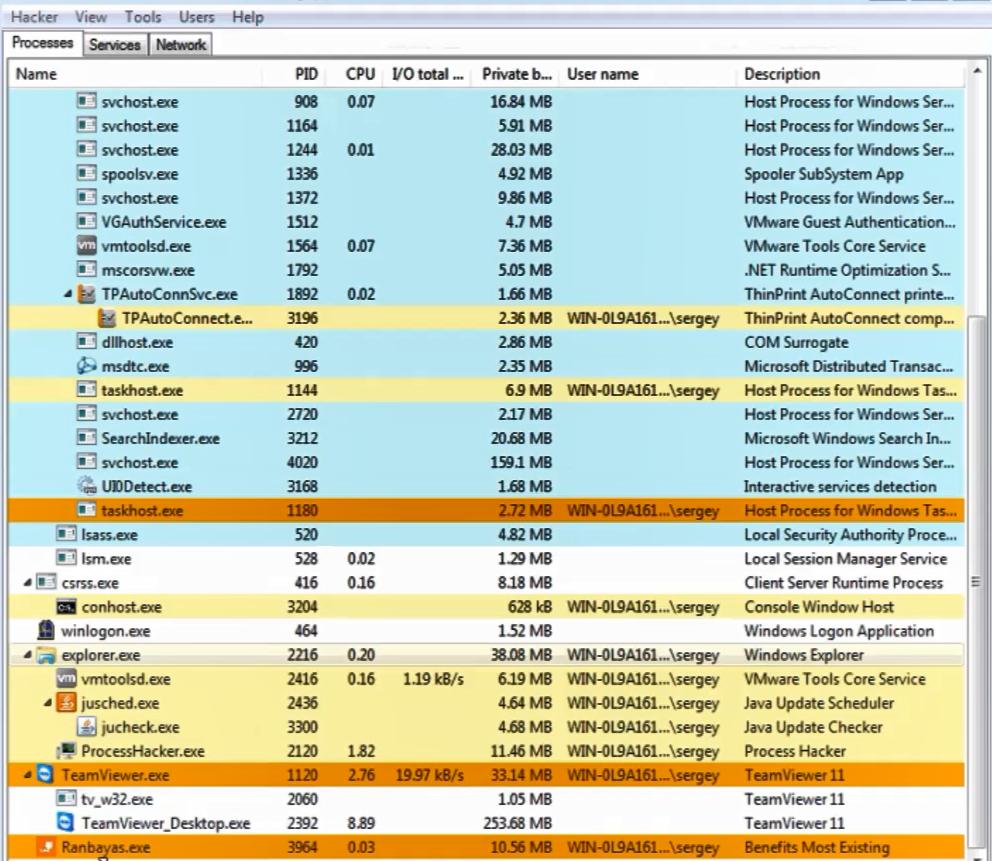
Fig.
Figure 2. Example of a computer interface infected with a Ranbayas Trojan
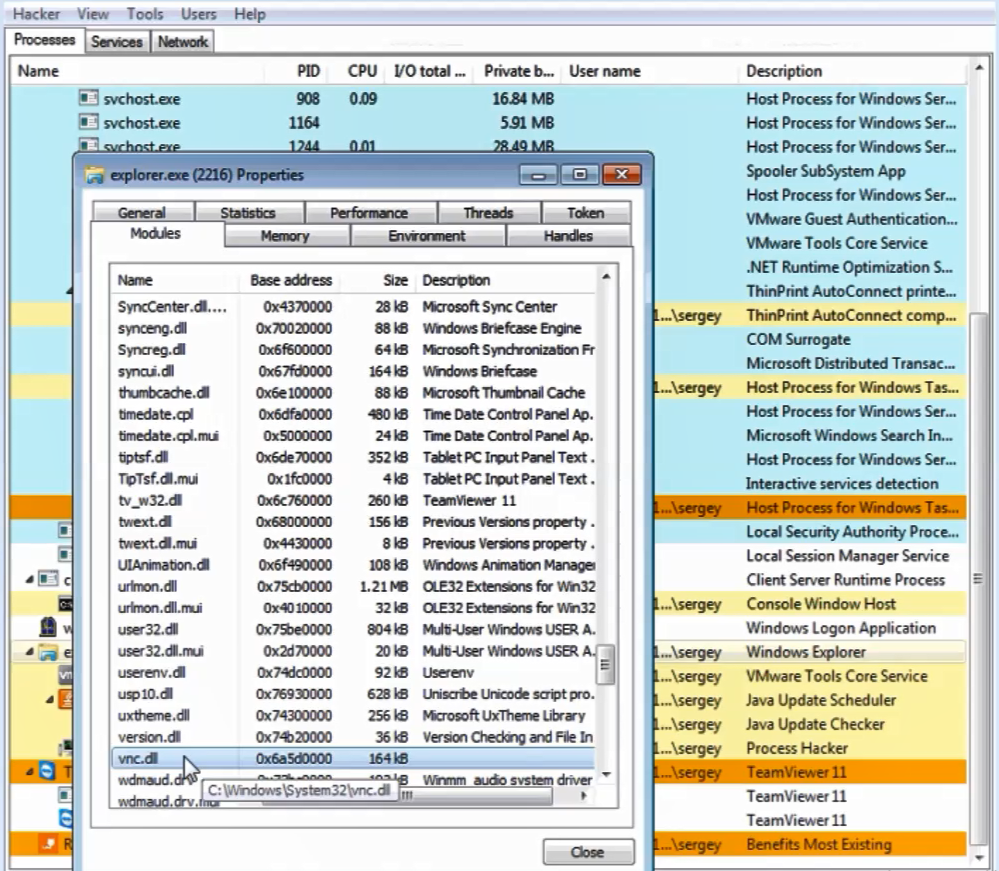
. 3. Implementing VNC Remote Management in Explorer
So what is the first level of fraud prevention technology? First of all, those that allow you to effectively identify remote control when working in remote banking systems using several independent methods. However, they do not require the installation of additional software on the client device.
Also at the first level of technology are systems and solutions that produce identification of the device and the user. This allows you to collect and analyze information about the devices used by a particular client (on the left in Fig. 4 is a fragment of the graph of connections between accounts and the devices used by them). For example, the appearance of an unknown device in a client previously known to us is an important factor for deciding on a suspension of payment and conducting additional control procedures.
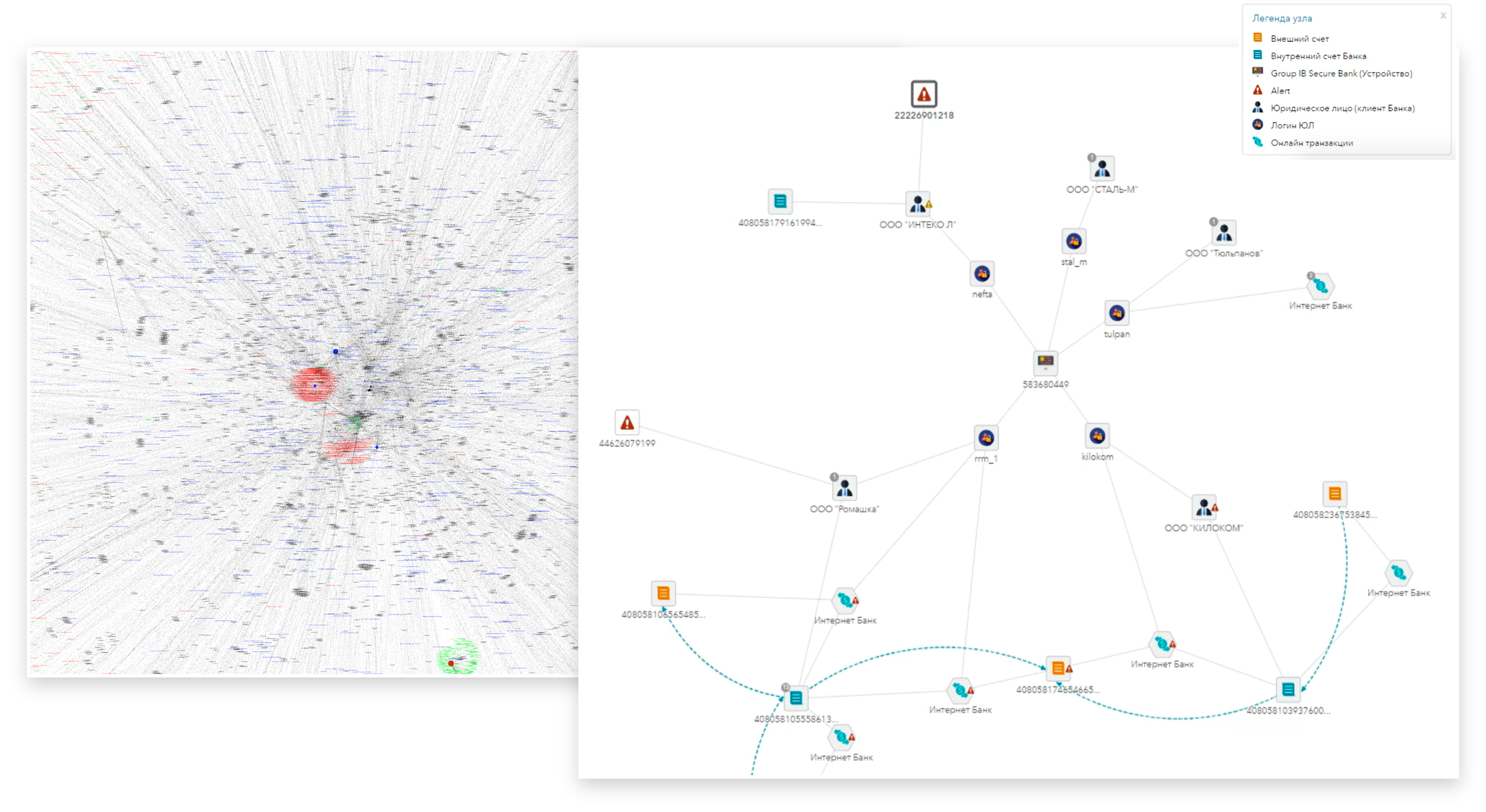
Fig. 4. Graphical Account Relationships
In fig. Figure 4 on the right shows another example of identification of a device for proactive identification of legal entities intended for money laundering. In this example, the bank employees identified, using financial analysis, a company that was engaged in money laundering (the lower part of the column on the right). Additionally, by the identifier of the legal entity, you can get the identifiers of the devices that they used (center of the right column). And then - all the other accounts that were used from the same devices (the upper part of the right column) and with which the identified “launder” worked. This fits very well with the nature of the work of scammers. The fraudster always has in stock companies that have not yet been used for money laundering. The bank should increase attention to the operations of identified legal entities.
Second level: monitoring user actions
At this level, it is analyzed what a person does directly as part of a work session in a remote banking system or other system. At this level, we can identify abnormal user behavior or typical fraudster scenarios. In particular, such an analysis already makes it possible to increase the efficiency of fraud detection using social engineering (i.e., when a fraudster uses the credulity, imprudence or ignorance of the user to entice him from information or force him to take actions beneficial to the fraudster).
For example, a common case is the enticement of card details and SMS codes in the process of buying expensive goods through popular classified sites under the pretext of making a deposit. In fact, the fraudster uses this data to go through the registration procedure in the RBS and gain access to the victim's accounts. A real example of such a scenario is shown in Fig. 5.
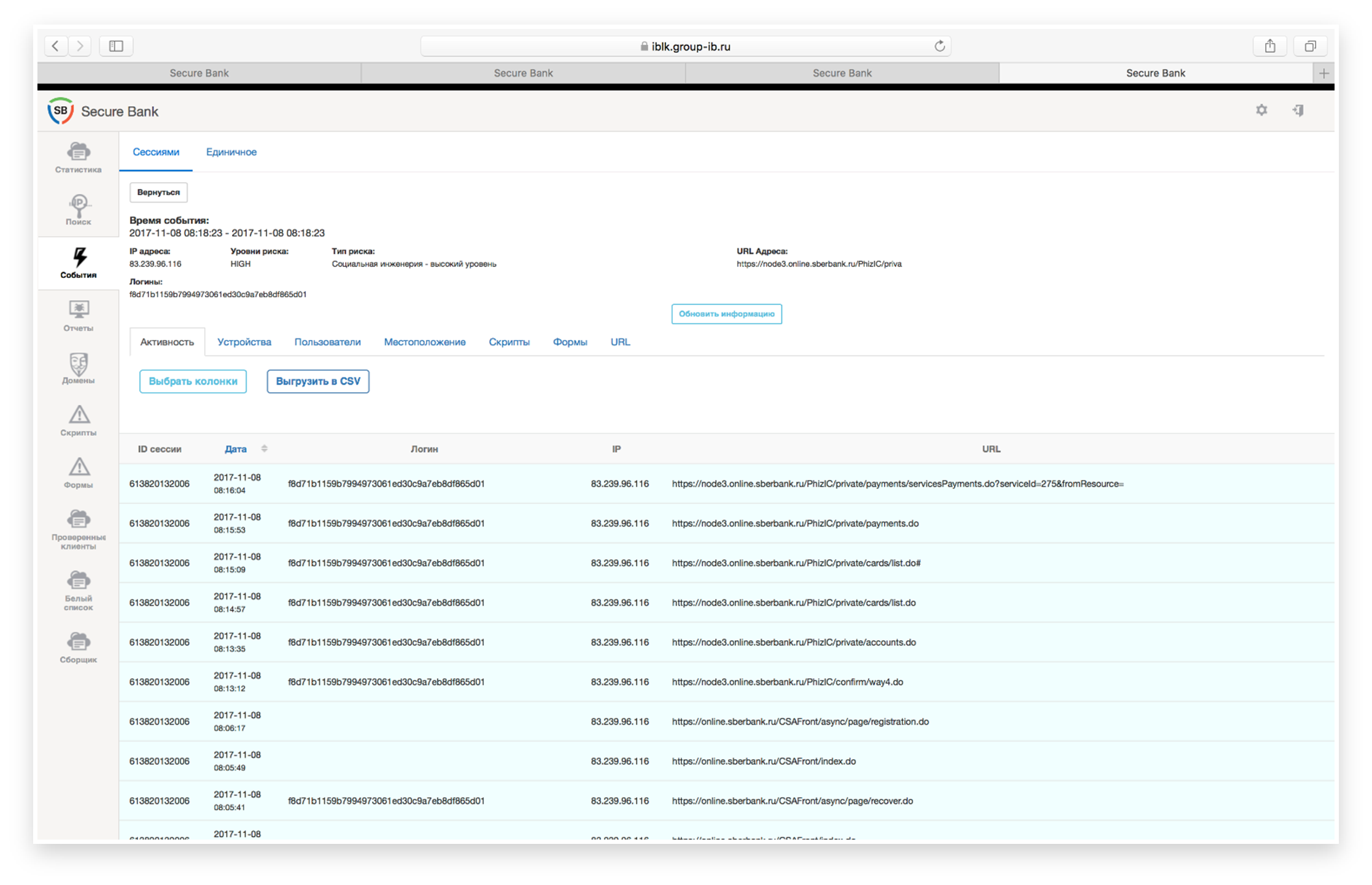
Fig. 5. Passing the registration procedure of a fraudster in the banking system and gaining access to the victim's accounts.
Analysis of the sequence of steps performed by the user in the RBS allows you to identify the scenario described above. In this process, the results of the first-level technologies (analysis of the user's device) are taken into account: which device was used, is it typical for a given client, has the geography changed; the session time is taken into account and in addition - the scenario that the fraudster uses.
This also applies to other scam schemes. For example, a similar method identifies some of the scenarios of the “launders”. It is clear that not all cases of money laundering can be tracked only by behavioral characteristics. For example, if a company is engaged in transit, then only one analysis of behavior - what a person does in Internet banking - will not be enough to understand whether it is a transit payment or a standard one. But most cases nevertheless make it clear that something abnormal is happening and, most likely, this activity is fraudulent.
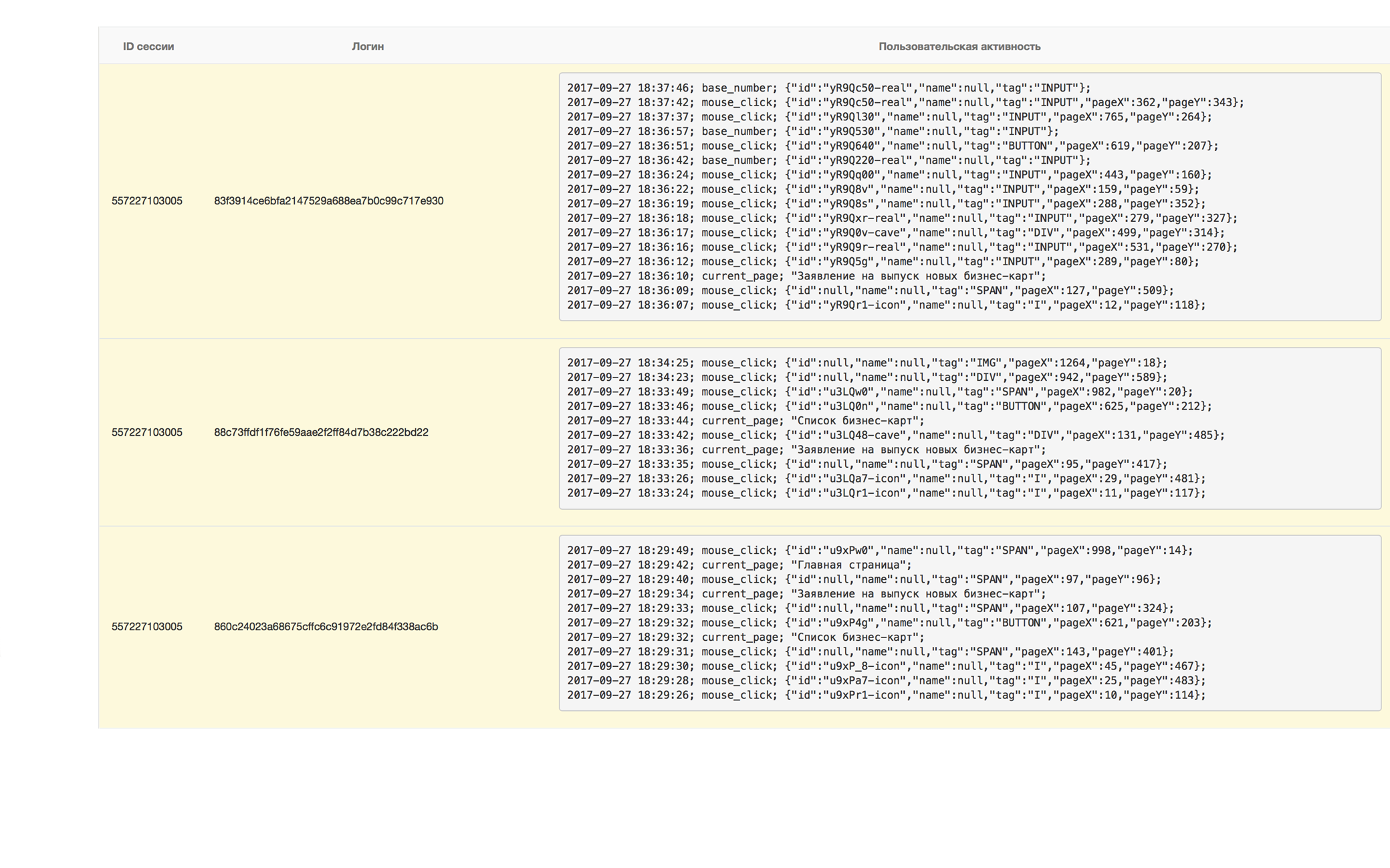
Fig. 6. Preparation for the implementation of a fraudulent scheme: entry of more than 100 legal entities with a request for a bank card issue.
So, in fig. Figure 6 on the right shows an example when from one device you are logged in to more than a hundred accounts of legal entities, the only activity of which was a request to issue a bank card and expand its limit. As was confirmed later - in this way the base of cards for money laundering was prepared. Also to the second level is the problem of bots. If the customer does not have any system for protecting against bots, then, as a rule, very primitive bots (accessing the API directly) perform all necessary actions (brutforce, password-check), bypassing the bank’s web application. But there are more “smart” bots used by scammers to bypass anti-bot protection.
Such bots imitate the work of the user. Often botnets are used for this. That is, the work of bots is distributed, and not concentrated in any particular hosting. Such bots are identified by the scenarios of their work on sites and the nature of the action. This smoothly brings us to the third level.
Third level: we analyze user behavior in a specific channel
If at the second level we analyze what the user does in the system, then at the third level we additionally analyze how the user performs certain actions. We show this with a real example (Fig. 7).
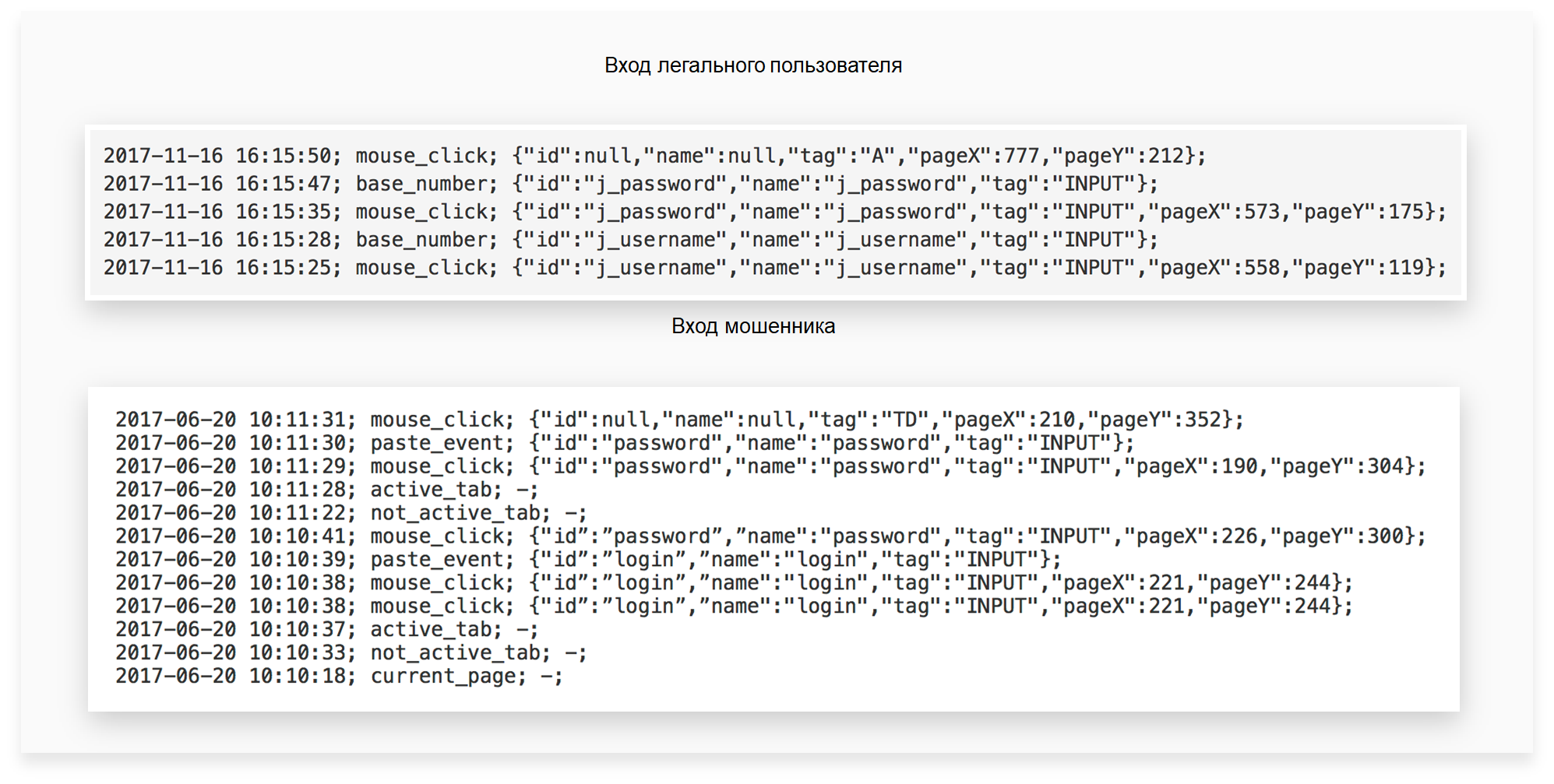
Fig. 7. Comparison of work in the system of a legal user and a fraudster: identification of uncharacteristic and suspicious actions
In the upper part of the figure, the sequence of actions of a legal user is visible. That is, he goes to the page to access the RB system, uses the upper numeric keypad to enter the login and password, then clicks “Enter”. The lower part of the figure shows a typical version of the work of a fraudster who somehow collected logins and passwords, for example, using fake (phishing) sites or using a trojan. He has their whole base. Naturally, the fraudster does not retype the data he received, but copies it from the clipboard when entering the RB. And this is clearly seen in the screenshot.
In addition to this, all methods of analyzing the device and user behavior described at previous levels are used. At this level, machine learning algorithms are actively used. One of the most striking examples is the use of biometric technologies such as keyboard and cursor handwriting, taking into account the behavioral nature and habits of the user's work in the system. In fig. Figure 8 shows the script for using keyboard handwriting on the user authorization page, “captured” from the Group-IB Secure Bank system.
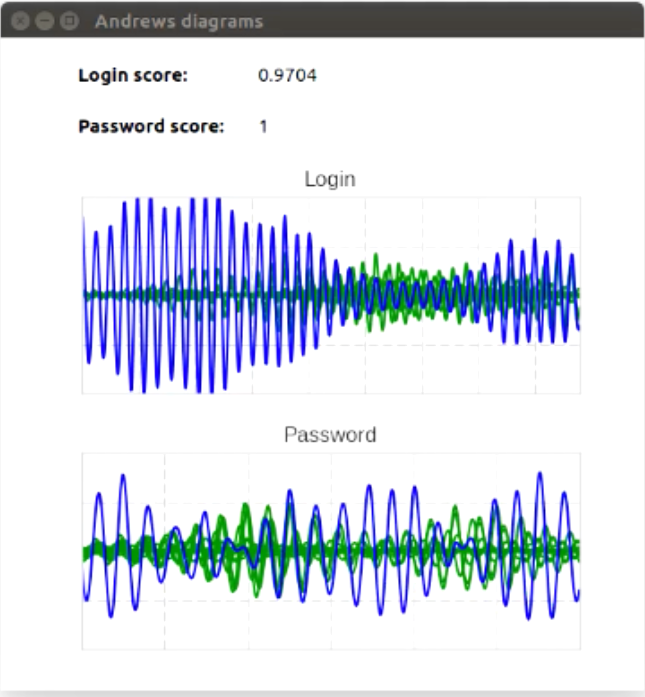
Fig. 8. The script for using keyboard handwriting on the user authorization page.
On the graph along the axis, the accumulated handwriting of a legitimate user is highlighted when entering the login and password. More noticeable fluctuations characterize the keyboard handwriting of the fraudster. It is seen that the keyboard handwriting is different. Above the graphs, two integral estimates of the differences between them are indicated. Values of estimates exceed the established thresholds, which indicates atypical behavior for a legitimate user.
The combination of the above technologies of behavioral analysis allows you to identify fraud committed using social engineering. Also, these technologies can reduce the number of false positives of transactional antifraud systems. For example, with an accuracy of 91%, it turned out to drop 78% of false positives of the transactional antifraud system for cases of social engineering, which significantly frees up the bank’s internal resources, including from the mass of calls from annoyed customers.
Fourth level: we implement cross-channel analysis of users and their behavior
At the fourth level, technologies for analyzing and correlating data on user behavior on his devices when working through various channels of interaction with the bank are used.
How effective this is is demonstrated in one of the real cases, the graphic connections of which are shown in Fig. 9.
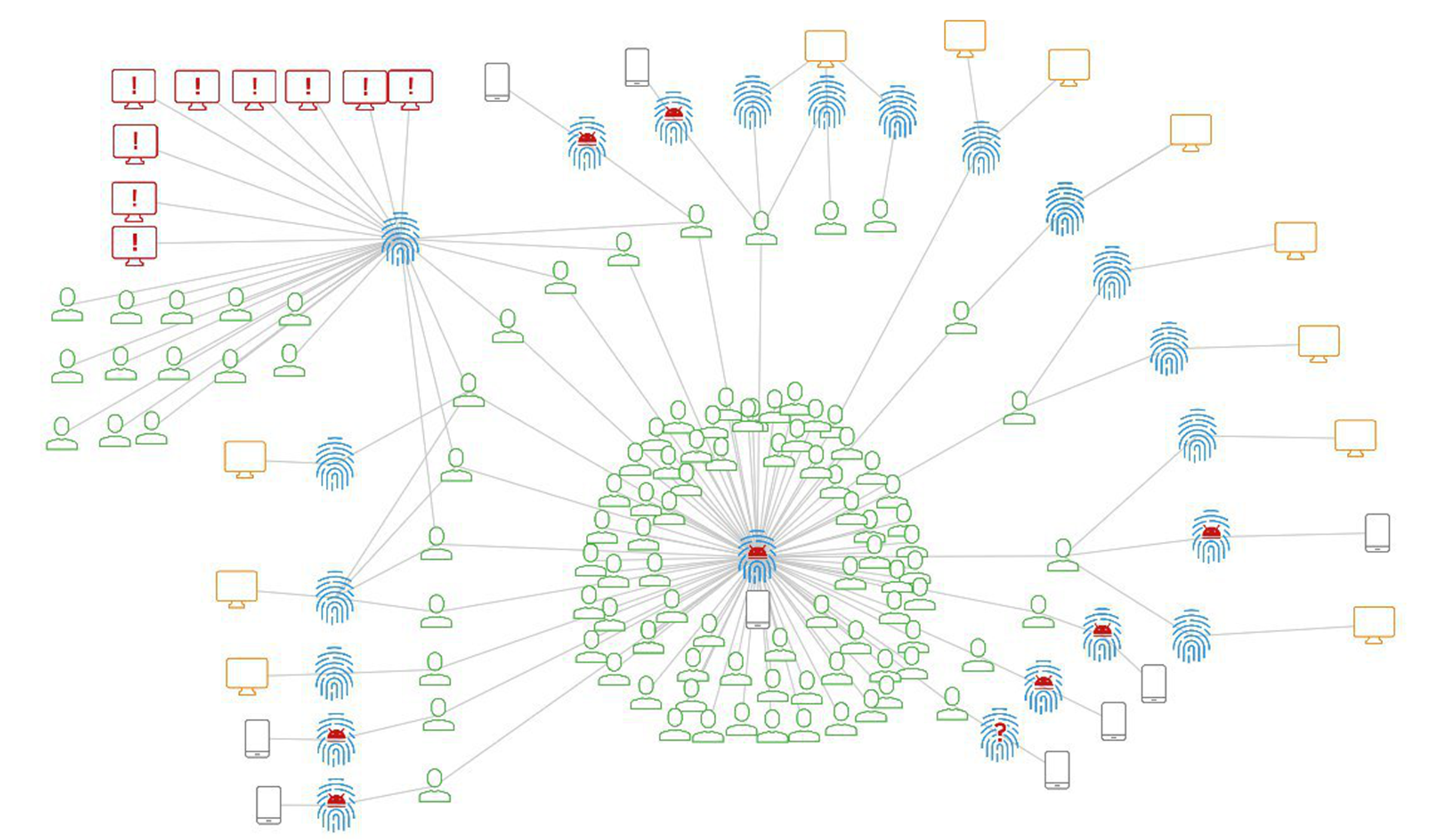
Fig. 9. Graphic connections showing the user's work with various devices, among which there is a fraud device.
The scammer was originally detected on a mobile device. He used a mobile Trojan to collect logins and passwords, payment card details and intercept SMS confirmations from the bank for unauthorized payments. An analysis of the relationship between the accounts and the devices they use made it possible to detect a fraudster’s mobile device, which is shown in the center and at the bottom of the picture in the accounts cloud. As you can see, only part of the compromised accounts previously worked through the mobile application. Another significant part of the identified accounts was previously used in Internet banking. Later it turned out that the same scammer used methods of social engineering to compromise them. The fraudster’s device, which he used to access the victims’ online bank, was also identified.
The fraudster erased the browser history after sequentially using from 3 to 8 accounts, trying to cover up the traces of his work. But all the devices had the same digital fingerprint (remember level 1). It was through this fraudster device that other victims were identified. Moreover, through this analysis of ties, a case emerged when a fraudster, using social engineering methods, “bred” a victim to receive an express loan with the subsequent theft of issued credit funds.
In this example, we can summarize the following results:
- firstly, a cross-channel analysis of theft attempts using mobile trojans made it possible to identify and prevent theft attempts, which are in no way related to the malicious code, but are performed using social engineering;
- secondly, he also helped to build a complete picture of the work of the fraudster and prevent theft from the entire customer base, regardless of the remote banking channel;
- Thirdly, we received more data for further investigation.
That is why, if now there are very few banking Trojans for desktop computers or iOS, or they are even absent, it is necessary to log and correlate the user’s work through these channels, since this allows you to see an order of magnitude wider picture of the users’s work and, as a result, increase the effectiveness of anti-fraud .
Fifth level: we analyze the relationship between the user and the accounts
The fifth level is a continuation of the fourth level, that is, the analysis of data on the behavior of the user and his devices is carried out not only within one specific bank, but more broadly - between banks, electronic commerce, offline operations, etc. This is the most difficult, but at the same time the most powerful level of combating fraud, since it allows not only to stop the same actions of fraudsters in different organizations, but also to prevent the general chain of actions of a fraudster, which he conducts through different organizations.

Fig. 10. Example of fraudulent activities in Bank A: the entire pool of accounts and devices can be analyzed in other banks (Bank B)
For example, the same scammers are involved in money laundering. Despite the fact that they change the legal entities that they manipulate, they work from the same arsenal of computers and other equipment. If fraudulent activity was detected in one bank (in Figure 10 Bank A), then the entire pool of accounts and devices can be analyzed in other banks (in Figure 10 Bank B). This analysis, in turn, can identify new accounts, new registration data of legal entities, their other devices and continue to be reused among banks, revealing more and more details with each iteration and the full structure of the work of fraudsters. Note that the described synergistic effect also works in relation to other schemes and types of fraud.
This level also includes technologies and cyber-intelligence platforms (Threat Intelligence), which allow you to obtain both strategic knowledge about what the fraudster is preparing for
and tactical data about what he has already done regarding certain users. In the latter case, if the organization’s security systems missed the identified incidents, this makes it possible not only to take advanced actions regarding already compromised data, but also to set up their defense systems to respond to the new threat.
In conclusion, we add that each level of protection flows smoothly from one another. Thanks to this, applying all five levels of preventing cybercrime in the field of banking and banking, banks will receive the most effective protection against external and internal harmful influences.The material was published in the journal "Calculations and operational work in a commercial bank", No3 (145) \ 2018.