Compilation of a rating of territories by the method of thermal potentials using open data
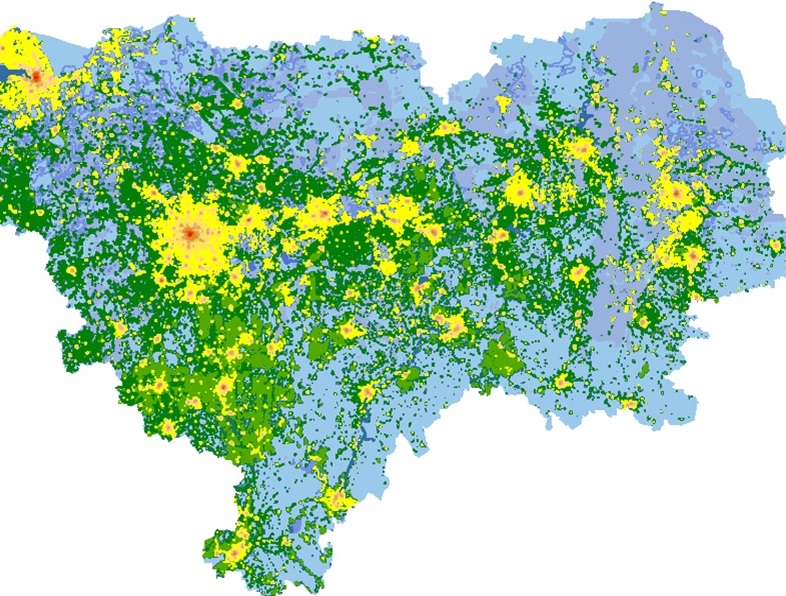
In this article, we consider the algorithm and the results of the analysis of large areas without any restrictions on their borders, using the method of thermal potentials and the method of principal components . As initial information, preference was given to open data, primarily with OSM. The study was conducted on the territory of 40 subjects of the European part of the Russian Federation, as a whole with a total area of 1.8 million sq. Km.
Some tasks solved by the potential method:
- The definition of a set of point estimates at each point of the territory, giving an idea of the level of development of the territory in general, and in some subject area in particular. For example, trade, industry, services, etc.
- Finding homogeneous zones (clusters) of the analyzed territory and their exact boundaries.
- Calculation of recommendations for the placement of a new facility , including a composite facility, including other facilities.
- Finding cost or territory value models for each cluster.
- Comparison of territories, i.e. compilation of ratings of territories (which will be discussed in this article).
Scope of the study
The mentioned problems are solved for the level of cities (several hundred sq. Km with spatial accuracy of 25 meters) and the level of regions (several hundred thousand sq. Km with spatial accuracy of 250 meters). The article describes the methods of analyzing the territory on a new scale - the unification of cities, regions, districts into a single space.
The main research methods:
- It uses open spatial information, primarily OSM, and methods of processing it.
- To take into account the degree of influence of objects of different nature on the territory, the method of potentials is used .
- To reduce the dimension of the problem and improve the quality of the models, the principal component method is used .
- To calculate linear models, the least squares method is used .
- For analysis, clustering methods are used to determine homogeneous regions. Each cluster has its own model; models are stitched together at the boundaries of the clusters.
The study was conducted at the level of constituent entities of the Russian Federation and large cities, with their subsequent unification.
First of all, we present the algorithm and results of the study of homogeneous territories of the constituent entities of the Russian Federation - the Volga Federal District, the Central Federal District, the Southern Federal District, the North-Western Federal District and part of the Ural Federal District, so the total research area was approximately 1.8 million square kilometers.
Description of research technology:
- Collection of source information from open data sources (our preference is OpenStreetMap) in the context of each subject of the Russian Federation. The initial information is downloaded with the maximum radius of influence Rmax for all factors (for example, in our case the maximum radius of influence was 50 km, i.e. we will download the information with a buffer of 50 km).
- The meshing is carried out clearly within the boundaries of the subject of the Russian Federation (the mesh step for this study is 1 kilometer), and the procedure for splitting the factors of influence into fragments for further calculation of potentials is carried out taking into account the constructed buffer in order to take into account the influence of factors at the border of the subject.
- The calculation of potential values and filling in the calculation results in grid nodes is carried out within the boundaries of the subject of the Russian Federation.
- The union of the constituent entities of the Russian Federation into a “single project”. That is, at this stage, we combine all the grids built for each subject earlier into one. Thus, we get a grid covering an area of 1.8 million square kilometers. and containing 1.8 million nodes (points of analysis) and 88 influence factors (in our opinion, the most influential OSM factors are point and extended objects (highways, forests, rivers, infrastructure objects)).
- Calculation of integral indicators using the principal component method.
- Search for homogeneous areas.
The studied territories are united in homogeneous areas according to factors describing any side of the territory's development, for example, the social sphere, trade, industry.
The image below shows images of homogeneous areas of the “single project” in the context of: industry, trade, services.

Homogeneous territories of the European part of the Russian Federation by factor "industry"

Homogeneous territories of the European part of the Russian Federation by factor "industry" (fragment Moscow)

Homogeneous territories of the European part of the Russian Federation by factor "trade"

Homogeneous territories of the European part of the Russian Federation by factor "trade" (fragment of Moscow)

Homogeneous territories of the European part of the Russian Federation by the factor of “service”

Homogeneous territories of the European part of the Russian Federation by the factor of “services” (fragment of Moscow)
The presented centers clearly dominate the subjects of the Russian Federation, but it can also be noted that some homogeneous areas (areas highlighted in the same color) are found in almost every subject. In other words, these homogeneous (in importance) territories in different entities are the most attractive, both in investment and in other plans.
The next stage of the study was the compilation of ratings of the subjects of the European part of the Russian Federation, which are given in the tables below.
Compiled ratings show the best square kilometer, the best 100 square meters. km and the best 20,000 sq. km. It should be noted that this is not a specific fragment of a territory of 1, 100 or 20,000 sq. Km, but a set of grid nodes (analysis points) built on a subject that have the best integral characteristics, i.e. these nodes can be located randomly on the territory of the subject of the Russian Federation.
Table 1. Rating of constituent entities of the Russian Federation (the best 1 sq. Km.)

Table 2. Rating of constituent entities of the Russian Federation (the best 100 sq. Km.)

Table 3. Rating of constituent entities of the Russian Federation (the best 20 sq. Km.)

The first positions in the ranking are held by Moscow and the Moscow Region, St. Petersburg and the Leningrad Region, Sverdlovsk Region, Chelyabinsk Region, Nizhny Novgorod Region, and Tatarstan. However, it is worth paying attention that the rating is “floating” depending on the scale of research (the subjects of the Russian Federation change places), that is, the set of the best nodes is affected by the fact that we consider -1 sq. Km, or 100 sq. Km, or 20 000 sq km
Solving a similar problem for the centers of the constituent entities of the Russian Federation (we selected 40 largest and largest centers), we set the step of building a grid of 100 meters and obtain the following results.
Table 4. Rating of centers of constituent entities of the Russian Federation (best 0.01 sq. Km. - the best point in the territory)

Table 5. Rating of centers of constituent entities of the Russian Federation (best 10 sq. Km)

Table 6. Rating of centers of constituent entities of the Russian Federation (best 200 sq. Km)

Thus, as in the task at the level of the constituent entities of the Russian Federation, the rating turned out to be dependent on the studied scale. This is because the number of grid nodes (analysis points) with the best integral indicators varies depending on the selected scale.
In conclusion, it is worth noting that any rating is a thing that is conducive to discussion, however, we conducted research on open data using new methods of analyzing the territory, allowing us to transfer existing methods to a smaller scale - the level of the constituent entities of the Russian Federation, federal districts and beyond.
The variable scale and new methods allow us to analyze objects distant from each other as a whole, taking into account the mutual influence of territories on each other.