
Cheating automated surveillance cameras

In recent years, interest in machine learning models has increased, including for recognition of visual images and faces. Although the technology is far from perfect, it already allows you to calculate criminals, find profiles on social networks, track changes and much more. Simen Thys and Wiebe Van Ranst proved that by making only minor changes to the input information of the convolutional neural network, the final result can be replaced. In this article, we will look at visual patches for conducting recognition attacks.
The first attacks on recognition systems were small changes in the pixels of the input image to deceive the classifier and derive the wrong class.
The goal was to create a patch that could successfully hide a person from the detector. The result was an attack scheme that could be used, for example, to bypass surveillance systems. Attackers can sneak imperceptibly, holding in front of themselves a small cardboard tablet with a “patch”, aimed at the surveillance camera.
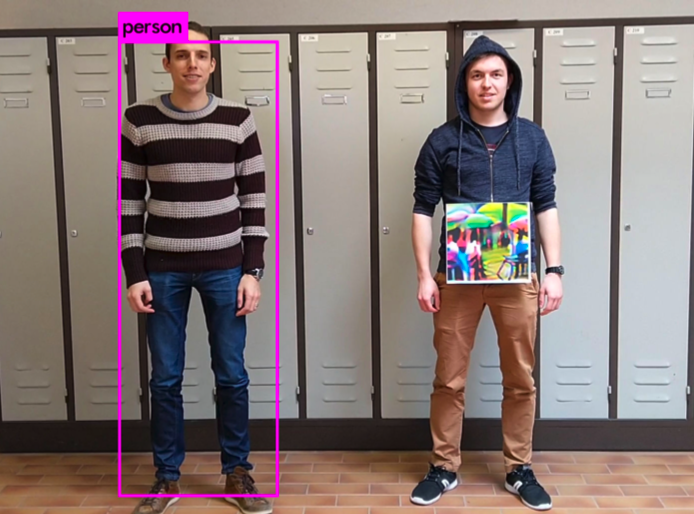
Development of convolutional neural networks(SNA) has led to tremendous advances in computer vision. A data-driven end-to-end conveyor in which SNAs are trained on images has shown the best results in a wide range of computer vision tasks. Due to the depth of these architectures, neural networks are able to study the most basic filters at the bottom of the network (where the data comes in) to achieve abstract high-level functions at the top. For this, a typical SNA contains millions of parameters studied. Although this approach leads to very accurate models, interpretability is drastically reduced.
In research, a variety of images were used to trick surveillance systems, including abstract “noise” and blur.
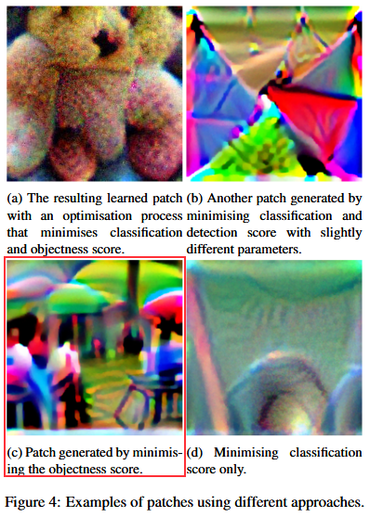
To create a patch, the original image was used, which underwent the following transformations:
- rotation by 20 degrees;
- noise overlay;
- blur
- brightness modification;
- contrast modification.
Researchers have conducted many Inria tests to determine the best “concealment” of a person.
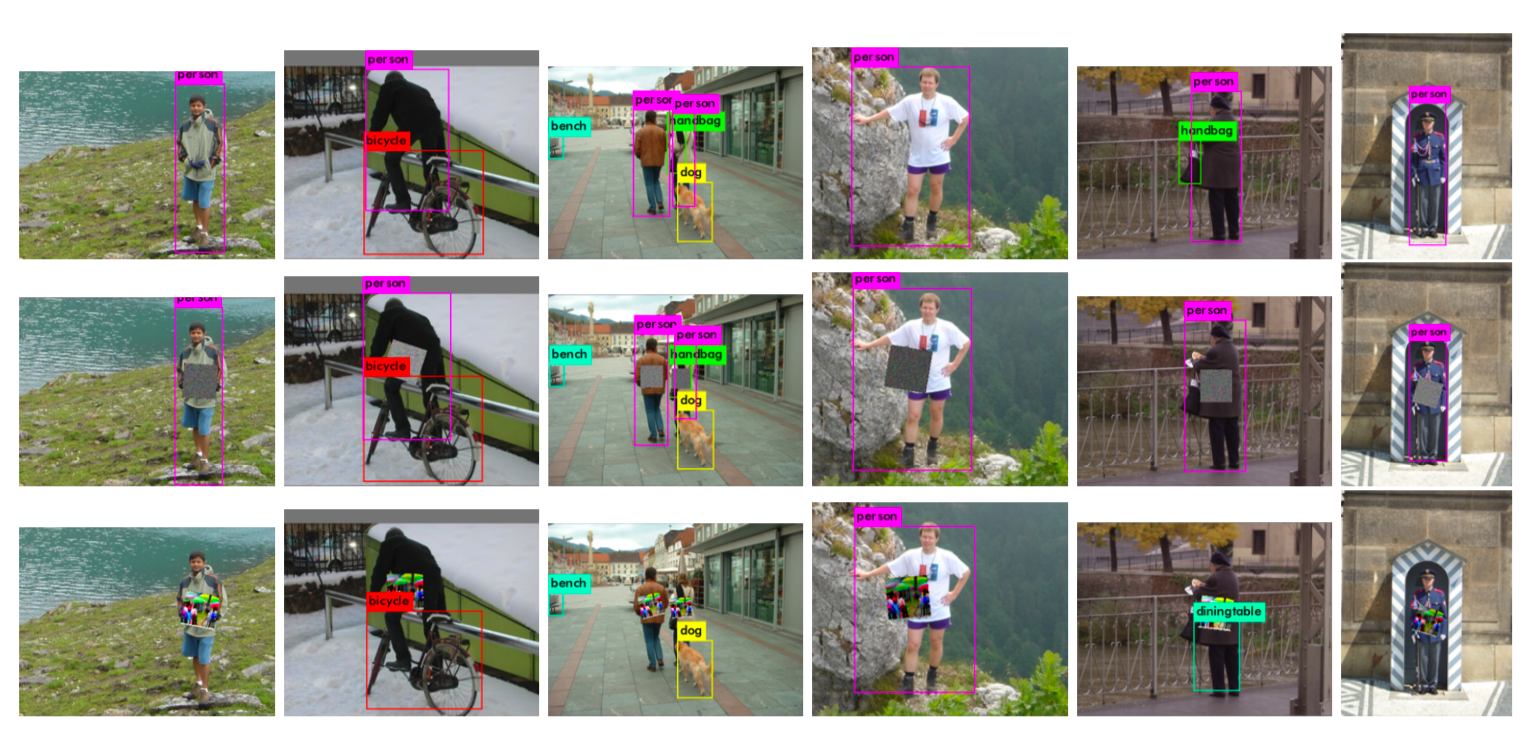
To achieve the desired effect, an image of 40x40 centimeters (which is indicated by the word patch in the expert report) should be located in the middle of the camera’s detection box and constantly in its field of view. Of course, this method will not help a person hide his face, however, the algorithm for detecting people in principle will not be able to detect a person in the frame, which means that subsequent recognition of facial features will also not be launched.
As a demonstration, researchers published a video demonstration of the capabilities of visual patches:
GitHub project code .
Research .