Can the researchers of artificial intelligence trust him to check their work?
- Transfer
A machine learning researcher at Virginia Institute of Technology has proposed a way to review scientific work using AI, which evaluates the appearance of text and graphics in a document. Will its methods of evaluating the “completeness” of scientific work suffice to speed up the process of independent review?
In the field of machine learning, there is a whole avalanche of research. Cliff Young, an engineer from Google, compared this situation with Moore's law , adapted for publications on the topic of AI - the number of academic papers on this topic appearing on the arXiv website doubles every 18 months.
And this situation creates problems when reviewing works - experienced AI researchers are simply not enough to carefully read every new work. Can scientists trust AI to work on accepting or rejecting work?
This interesting question raises a report recently published on the arXiv website; Jia-Bin Huang [Jia-Bin Huang], the author of the work, a machine learning researcher, called it "Deep Gestalt of Work."
Juan used the convolutional neural network — a common machine learning tool used for image recognition — to sift 5,000 papers published since 2013. Juan writes that only one appearance of the work - a mixture of text and images - his neural network can distinguish "good" work, worthy of inclusion in scientific archives, with an accuracy of 92%.
For researchers, this means that a couple of things play the most important role in the appearance of their document: vivid pictures on the title page of the research work and filling all pages with text, so that at the end of the last page there is no empty space.
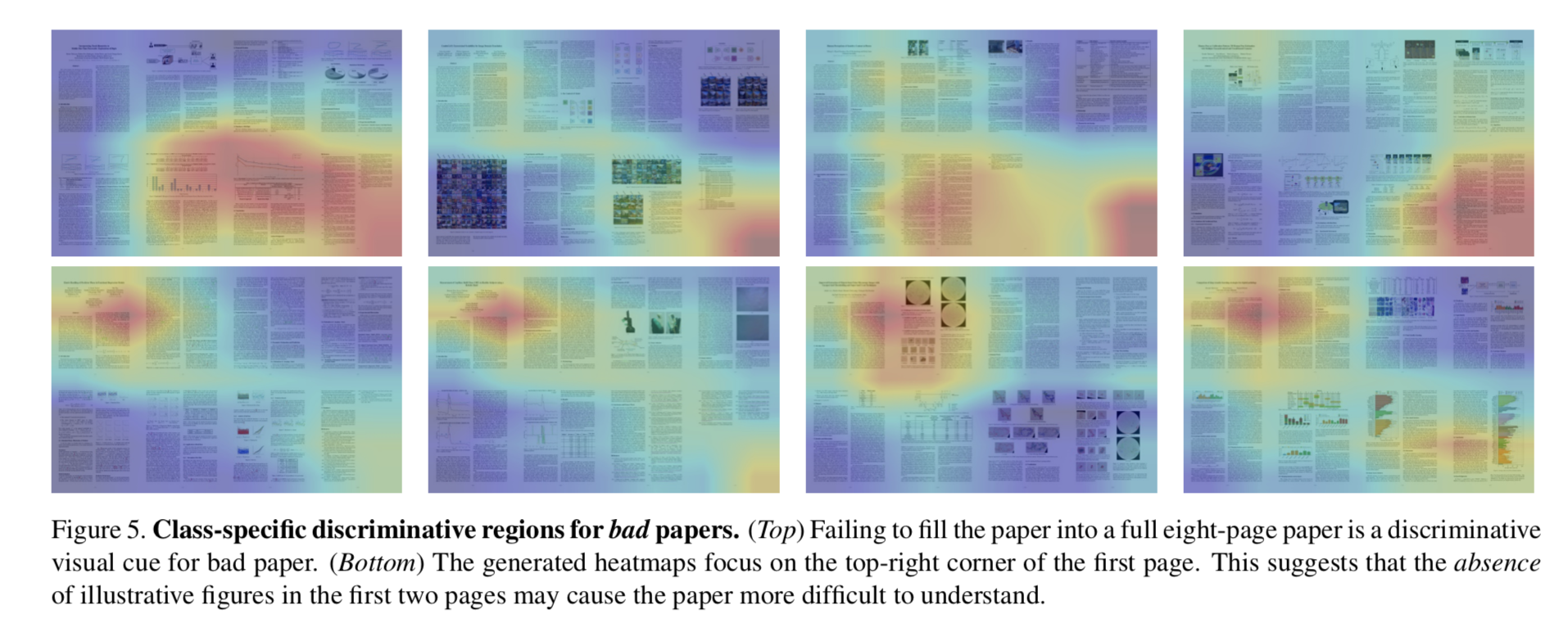
Juan's convolutional neural network digests thousands of approved and unapproved scientific papers, creating a “heat map” of strengths and weaknesses. The biggest mistakes of the works that did not pass the selection: the absence of color pictures and an empty space at the end of the last page.
Juan bases his work on another work from 2010, authored by Carven von Bearnensquash of the University of Phoenix. We used non-depth learning, traditional computer vision technology, to find a way to “at a glance assess the overall appearance” of the work and to conclude whether or not to approve the work.
Using this idea, Juan fed the computer 5,618 papers adopted at the two most important conferences on computer vision, CVPR and ICCV over the past five years. Juan also collected works presented at the workshops of conferences that played the role of rejected works - because there is no access to works rejected at conferences.
Juan trained the network to associate the past and not the past works with the binary outcome “good” and “bad”, in order to isolate from them the signs of “completion” or gestalt. Gestalt is something whole, exceeding in size the sum of its parts. This is what machine learning pioneer Terry Seinovsky called “general organized perception”, something more meaningful than the hills and ravines of the area in your immediate vicinity.
The trained network was then checked on a subset of the work that she had not seen before. The training balanced false positives - accepted jobs that were worth rejecting - with false failures, rejected jobs that were worth accepting.
By limiting the number of “good”, but rejected works, 0.4% - that is, only 4 works - the network was able to correctly reject half of the “bad” works, which had to be rejected.
The author even thought of feeding his own work of his own neural network. As a result, the neural network rejected it: “We applied a trained classifier to this work. Our network ruthlessly predicted that, with a 97% probability, this work should be rejected without independent review. ”
As for these cosmetic requirements - beautiful pictures in the article - Juan does not just describe the results of the work. He also offers code that allows you to create good-looking works. He feeds "good" work into the training database of the generative-adversary network, which can create a new plan, learning from examples.
Juan also offers a third component, “reworking” the rejected work into a valid, “automatically giving advice on what needs to be changed in the incoming work,” for example, “add a picture to attract attention and pictures on the last page.”
Huang suggests that a similar work approval process can become a “pre-filter” that lightens the burden on reviewers, since it can see thousands of papers in a few seconds. And yet "it is unlikely that such a classifier will be used at a real conference," the author concludes.
One of the limitations of the work that may affect its use is that even if the appearance of the work, its visual gestalt, coincides with the historical results, this does not guarantee the presence of real value in the work.
As Juan writes, “ignoring the content of the work, we may reject works with good material, and poor visual design, or accept fucking works that look good.”
In the field of machine learning, there is a whole avalanche of research. Cliff Young, an engineer from Google, compared this situation with Moore's law , adapted for publications on the topic of AI - the number of academic papers on this topic appearing on the arXiv website doubles every 18 months.
And this situation creates problems when reviewing works - experienced AI researchers are simply not enough to carefully read every new work. Can scientists trust AI to work on accepting or rejecting work?
This interesting question raises a report recently published on the arXiv website; Jia-Bin Huang [Jia-Bin Huang], the author of the work, a machine learning researcher, called it "Deep Gestalt of Work."
Juan used the convolutional neural network — a common machine learning tool used for image recognition — to sift 5,000 papers published since 2013. Juan writes that only one appearance of the work - a mixture of text and images - his neural network can distinguish "good" work, worthy of inclusion in scientific archives, with an accuracy of 92%.
For researchers, this means that a couple of things play the most important role in the appearance of their document: vivid pictures on the title page of the research work and filling all pages with text, so that at the end of the last page there is no empty space.
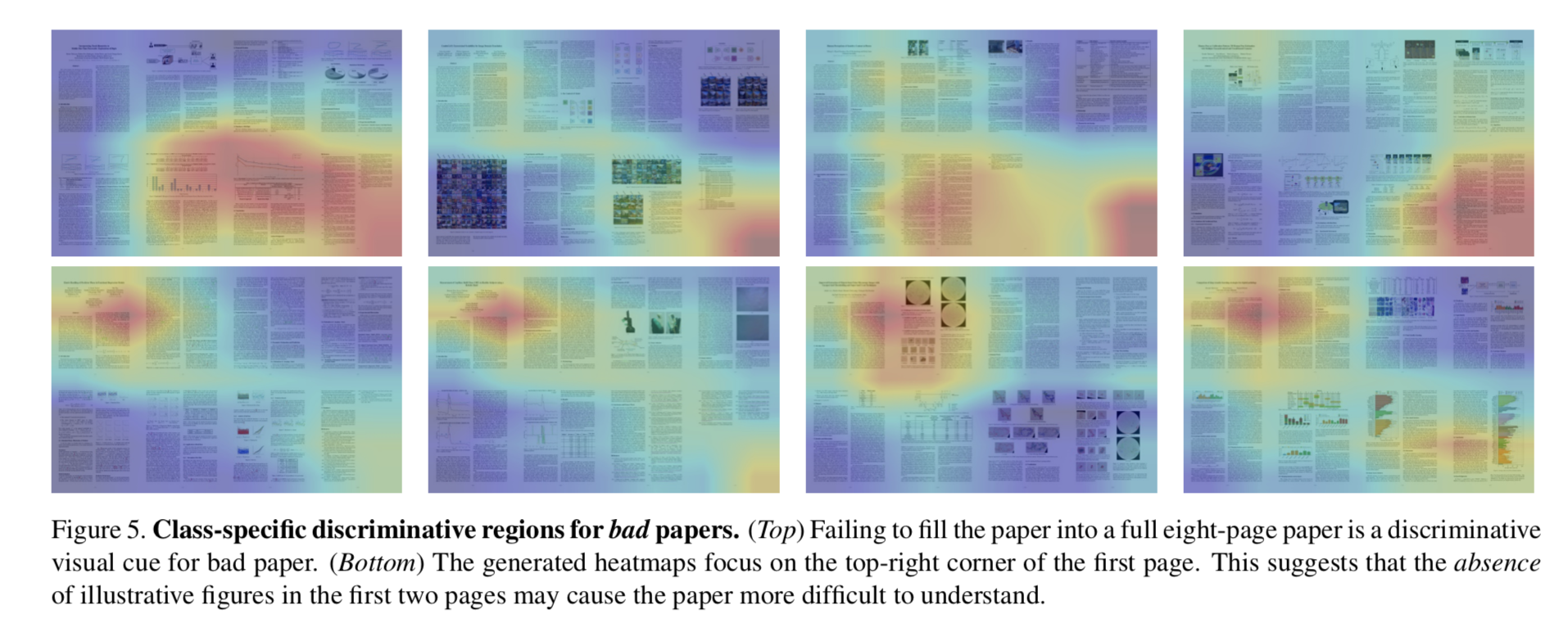
Juan's convolutional neural network digests thousands of approved and unapproved scientific papers, creating a “heat map” of strengths and weaknesses. The biggest mistakes of the works that did not pass the selection: the absence of color pictures and an empty space at the end of the last page.
Juan bases his work on another work from 2010, authored by Carven von Bearnensquash of the University of Phoenix. We used non-depth learning, traditional computer vision technology, to find a way to “at a glance assess the overall appearance” of the work and to conclude whether or not to approve the work.
Using this idea, Juan fed the computer 5,618 papers adopted at the two most important conferences on computer vision, CVPR and ICCV over the past five years. Juan also collected works presented at the workshops of conferences that played the role of rejected works - because there is no access to works rejected at conferences.
Juan trained the network to associate the past and not the past works with the binary outcome “good” and “bad”, in order to isolate from them the signs of “completion” or gestalt. Gestalt is something whole, exceeding in size the sum of its parts. This is what machine learning pioneer Terry Seinovsky called “general organized perception”, something more meaningful than the hills and ravines of the area in your immediate vicinity.
The trained network was then checked on a subset of the work that she had not seen before. The training balanced false positives - accepted jobs that were worth rejecting - with false failures, rejected jobs that were worth accepting.
By limiting the number of “good”, but rejected works, 0.4% - that is, only 4 works - the network was able to correctly reject half of the “bad” works, which had to be rejected.
The author even thought of feeding his own work of his own neural network. As a result, the neural network rejected it: “We applied a trained classifier to this work. Our network ruthlessly predicted that, with a 97% probability, this work should be rejected without independent review. ”
As for these cosmetic requirements - beautiful pictures in the article - Juan does not just describe the results of the work. He also offers code that allows you to create good-looking works. He feeds "good" work into the training database of the generative-adversary network, which can create a new plan, learning from examples.
Juan also offers a third component, “reworking” the rejected work into a valid, “automatically giving advice on what needs to be changed in the incoming work,” for example, “add a picture to attract attention and pictures on the last page.”
Huang suggests that a similar work approval process can become a “pre-filter” that lightens the burden on reviewers, since it can see thousands of papers in a few seconds. And yet "it is unlikely that such a classifier will be used at a real conference," the author concludes.
One of the limitations of the work that may affect its use is that even if the appearance of the work, its visual gestalt, coincides with the historical results, this does not guarantee the presence of real value in the work.
As Juan writes, “ignoring the content of the work, we may reject works with good material, and poor visual design, or accept fucking works that look good.”