The concept of the interaction of advertising channels and its application in practice
The meaningfulness of reports on advertising campaigns (RK) greatly simplifies the lives of people ... optimizing costs. In the process of raising the level of this meaningfulness, we found one very effective way to maximize profitability, taking into account what we called “channel interaction” (channel interaction). Original: caricatura.ru
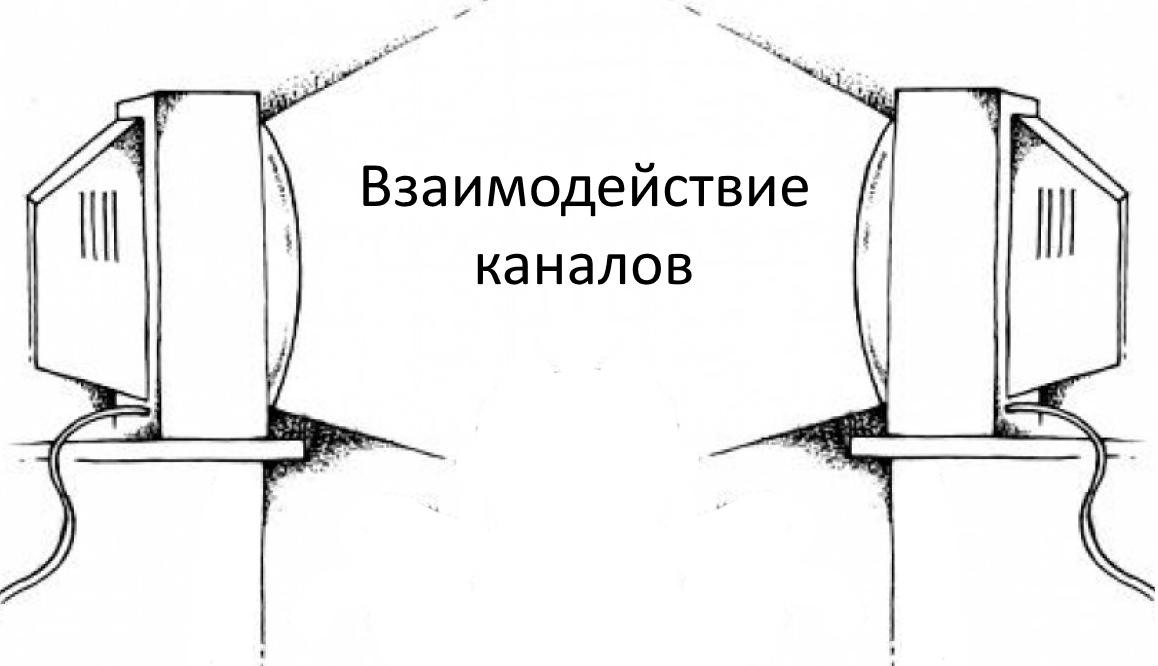
First, let's repeat the basics of digital marketing in two sentences. As you probably know, the marketing channel is a group of traffic sources grouped into certain categories. The most widely used channels are: organic search, direct email , etc. When a user visits several channels within a campaign, he creates a thread . Since users are not made on the conveyor, so everyone can touch different channels and in a different order. Well, or not at all, but we do not care. To measure the influence of various structures in the chains on the conversion rate, we decided to use the metric, which we called the interaction of channels.
Our experiments have shown that when users interact with only one marketing channel throughout their entire chain, the conversion rate (CR) drops 3-5 times. We also learned how to increase CR by eliminating the least useful marketing channel. We will tell all about it now.
Attribution models that are in Google Analytics (GA) often give fairly uniform results, since most RK chains contain only one channel. We call such chains that contain only one channel simple . And you can safely use one " Last Touch ", which will say whether a particular channel is effective compared to others. But I want to express myself more expressively, and now we will tell you how. Suppose a user touched two different marketing channels, before making a purchase, we would call them channels A and B. The conversion rate for this chain would be different from if the user only clicked on channels A or B.
Our study showed that the more a channel interacts with the user, the higher the probability of its conversion, with the same chain length. (hello from the captain obvious)
In order not to be unfounded, we will show a couple of graphs that we took from the real data of the Republic of Kazakhstan. Data Parameters:
The first graph shows the number of unique marketing channels found in the chain. The second graph shows how the conversion rate depends on the number of unique channels in each chain. As the number of unique marketing channels in the chain grows, the conversion rate increases.
The graphs show that although chains with 4 unique channels are quite rare, they are converted 3 times more often than simple chains. Thus, a careful study of the number of channels that users had time to touch before the conversion can significantly affect the conversion rate.
In Figure 1 (b) we are interested in the form of the graph. It grows faster than linear. This tells us that the channels together have some significant interaction, allowing to increase the overall conversion. And what other variants of the form of the graph of conversion dependence on the number of unique channels in the chains can be?
Fig.2. Hypothetical variants of the dependence of conversion on the number of unique channels in the chain.
The green line is the interaction between channels is limited, it is not necessary to strive for people to go through all these channels. A few are enough.
The blue line - the interaction between the channels is significant. The more people pass channels, the greater the likelihood of conversion.
Red line - no interaction. All channels can be viewed separately.
Yellow line - channels harm each other. The more channels the user passes, the less likely the conversion.
You can calculate two types of coefficients. One will tell us about the shape of the graph, the other about some absolute value of the interaction.
Let us have
chains of the same length
. From these chains, the maximum chain is obtained with
unique channels with conversions. There is a function of the average CR of the number of unique channels.
where
,
. Then the form of the chart will tell the indicator:
- interaction is limited if
- interaction is significant. If a
- Channels harm each other. If a
- the dependence is not monotonous. In essence, this is a strongly inspired AUC ROC indicator .
The absolute value of the interaction can be calculated as follows:
It remains only to tell how to compare specific channels in this way. If we remove from consideration all the chains, except those containing the two channels we need, we can evaluate how they affect each other by simple subtraction, because we have only two channels left (C = 2).
Respectively:
On the diagonal of this table are 0, because this interaction of the channel with itself and the table is symmetrical with respect to the diagonal. It shows that the zero channel has a good effect on the first and third in terms of conversion, but with the other interactions of the problem. To practice this is usually enough.
We have seen, for example, that everything is good with the interaction of channels and they have a positive effect on each other. But we still do not know how strong the effect is. Maybe there is interaction between the channels, and there is little interaction.
Let's try to do the same as in the previous case, look at the picture. But this time 1 (a). By the slope of this “straight line”, one can judge how the number of chains falls as the number of unique elements increases. It is logical to approximate it with the usual linear regression . In this case, the regression slope will be -522. Those. For chains of length 4, the number of chains in which there are 2 unique channels, rather than 1, is 522 fewer than the chains completely composed of one channel.
If we assume that we have 1600 chains with one channel and a positive conversion shift when moving from chains with one unique channel to chains with two 0.05 to 0.1, then reducing the slope from -500 to -200, for example, we will get 30 more conversions with the same conversion rate for chains with two unique channels. But there is a well-founded assumption that if this slope decreases, then the conversion will increase too, and not just the number of people for the same conversion will increase.
Well, here it is. The main question everyone constantly asks is: “What marketing channels should we use to make the most effective combination?”. There is a generally accepted rule of thumb: The more channels you initially use, the better. Then, after testing, you can theoretically determine which ones to remove from the campaign. The main problem here in practice has always been the criterion that would allow to remove one or another channel from the Republic of Kazakhstan. Suddenly you delete some, and he will do everything else worse, although he himself seems to bring nothing directly? We conducted an experiment to resolve this difficulty.
The simplest: Simply eliminating the channel with the least amount of interaction, we raised the average hospital CR from 1.58% to 2.1% . The experiment was conducted under the condition that the marketing costs were the same across all channels.
But do not rush to run and do it right away. A low interaction channel is often a popular platform, such as Facebook. Its elimination may lead to the loss of customers. This is due to the fact that these large platforms do not always have many conversions, but always a lot of hits. Therefore, we decided to normalize the data using impressions, not clickthroughs, to determine overall performance. This was also done under the condition that each channel is the same.
When comparing channels with different costs, we divided the conversion rates by the cost of the channels in order to evaluate their true effectiveness. When we excluded the two lowest-performing channels for this criteria from our campaign, the average conversion rate dropped from 1.58% to 1.54%. However, costs decreased by 28.9%, which led to a 28% reduction in CPC!
Thus, a decrease in CR does not necessarily mean that you are wasting advertising dollars. The overall effect depends on the selected variables for calculating the ROI for this product.
An experienced analyst will try to prove to you that the concept of channel interaction is simply cunning data manipulation. For example, we can assume that there is a very effective channel that also likes to be embedded in all the chains in a row. And therefore, the more unique channels in a particular chain, the more likely it is this MEGA channel, which increases conversion. From this and the increasing dependence in Figure 1 (b).
But no.
To refute the foregoing, we present two graphs from the same data sets. The first figure shows the number of touches that each channel receives if all simple chains are selected for it. The second graph shows how each specific channel is converted if you select all simple chains for it.
As can be seen from the graphs, the most popular channel is not the most efficient, and the effectiveness of any uniform chain does not exceed 17.5%. Let us reveal the consequences of this fact:
As can be seen from fig. 3 (a), channel 1 is the most popular. Therefore, if we have a chain and we are not sure which channels are in it, we can be sure that channel 1 will most likely appear somewhere in the (not simple) chain.
If channel 1 had the best CR, we could attribute the increase in CR for difficult chains to this channel, but no. Channel 1 for the chain length 4 has an average CR of 0.075 in Fig. 3 (b), not 0.3, as it was in fig. 1 (b), in which there were 4 unique channels in the chain.
Thus, the conversion rate of a particular individual channel or its “popularity” cannot explain the higher conversion rates found in non-simple chains. From this it becomes clear that the interaction of channels still exists.
We used the available data to create a simple mathematical model that allows us to recalculate the probability of conversion without taking into account the interaction of the channels. In the “no interaction” simulation, we obtained a total conversion rate of 0.3%, rather than 1.58%.
We also run this simulation for two more datasets.
The plots for both datasets clearly show that a lack of channel interaction significantly reduces CR. This is another additional evidence that the interaction thing is quite important.
If it is interesting to someone, we will write the model itself in a separate article, but it is so simple that you yourself can guess how to make it.
PS We publish our articles on several sites Runet. Subscribe to our pages on the VK , FB or Telegram channel to learn about all of our publications and other news from Maxilect.
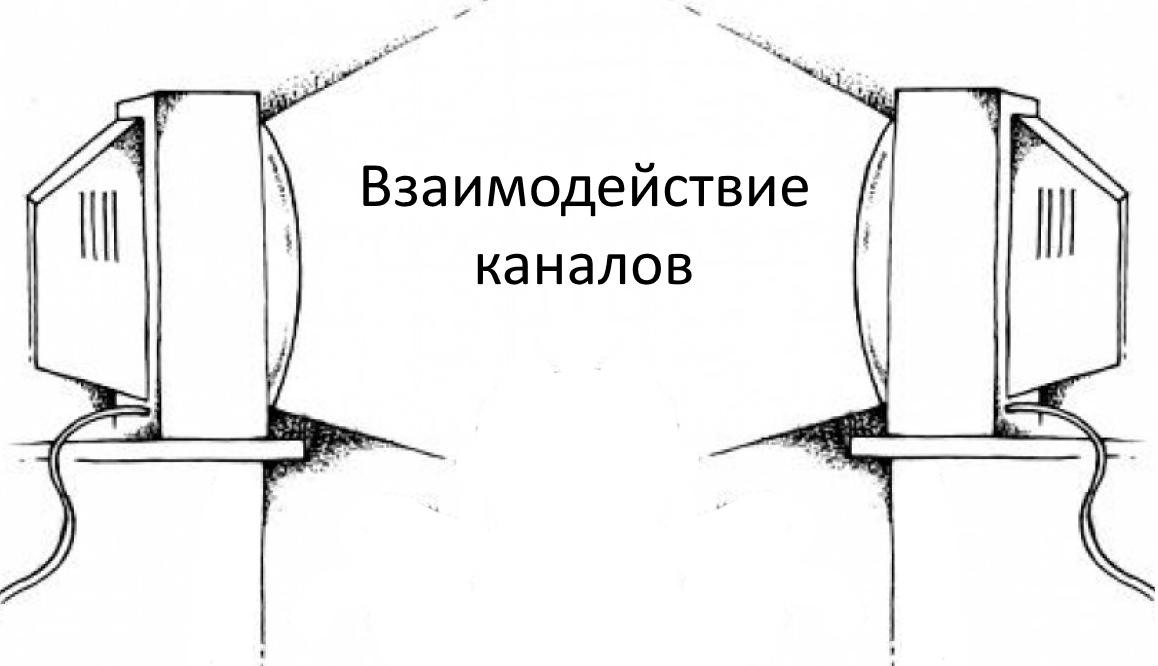
First, let's repeat the basics of digital marketing in two sentences. As you probably know, the marketing channel is a group of traffic sources grouped into certain categories. The most widely used channels are: organic search, direct email , etc. When a user visits several channels within a campaign, he creates a thread . Since users are not made on the conveyor, so everyone can touch different channels and in a different order. Well, or not at all, but we do not care. To measure the influence of various structures in the chains on the conversion rate, we decided to use the metric, which we called the interaction of channels.
Our experiments have shown that when users interact with only one marketing channel throughout their entire chain, the conversion rate (CR) drops 3-5 times. We also learned how to increase CR by eliminating the least useful marketing channel. We will tell all about it now.
The essence of the interaction of channels
Attribution models that are in Google Analytics (GA) often give fairly uniform results, since most RK chains contain only one channel. We call such chains that contain only one channel simple . And you can safely use one " Last Touch ", which will say whether a particular channel is effective compared to others. But I want to express myself more expressively, and now we will tell you how. Suppose a user touched two different marketing channels, before making a purchase, we would call them channels A and B. The conversion rate for this chain would be different from if the user only clicked on channels A or B.
Our study showed that the more a channel interacts with the user, the higher the probability of its conversion, with the same chain length. (hello from the captain obvious)
In order not to be unfounded, we will show a couple of graphs that we took from the real data of the Republic of Kazakhstan. Data Parameters:
- Chain Length: 4
- Number of channels used: 5
![]() | ![]() |
Fig. 1 (a) The number of unique channels in the chain vs the number of such chains | Fig. 1 (b) Chain conversion ratio vs the number of unique channels in the chain. |
The graphs show that although chains with 4 unique channels are quite rare, they are converted 3 times more often than simple chains. Thus, a careful study of the number of channels that users had time to touch before the conversion can significantly affect the conversion rate.
How to count the level of interaction?
In Figure 1 (b) we are interested in the form of the graph. It grows faster than linear. This tells us that the channels together have some significant interaction, allowing to increase the overall conversion. And what other variants of the form of the graph of conversion dependence on the number of unique channels in the chains can be?
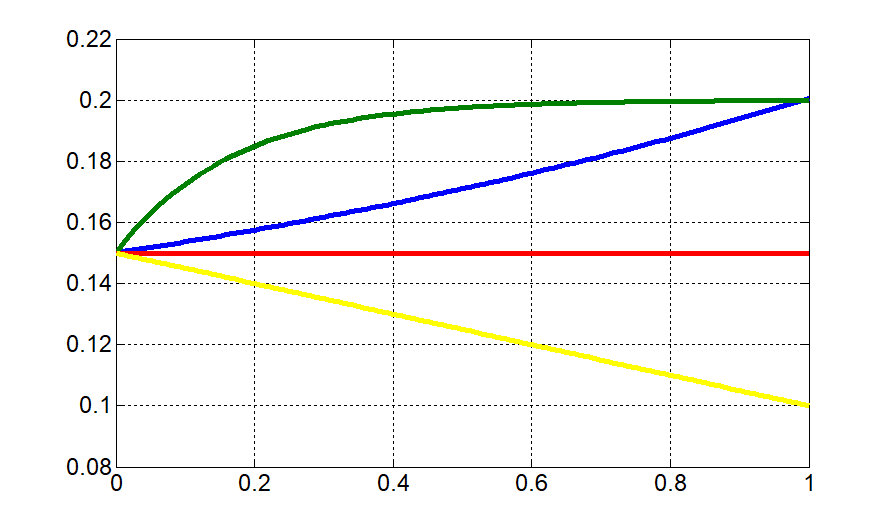
The green line is the interaction between channels is limited, it is not necessary to strive for people to go through all these channels. A few are enough.
The blue line - the interaction between the channels is significant. The more people pass channels, the greater the likelihood of conversion.
Red line - no interaction. All channels can be viewed separately.
Yellow line - channels harm each other. The more channels the user passes, the less likely the conversion.
You can calculate two types of coefficients. One will tell us about the shape of the graph, the other about some absolute value of the interaction.
Let us have
The absolute value of the interaction can be calculated as follows:
It remains only to tell how to compare specific channels in this way. If we remove from consideration all the chains, except those containing the two channels we need, we can evaluate how they affect each other by simple subtraction, because we have only two channels left (C = 2).
Respectively:
Channel names | 0 | one | 2 | 3 |
0 | 0 | 0.064 | -0.036 | 0.082 |
one | 0 | -0.032 | -0.0017 | |
2 | 0 | -0.028 | ||
3 | 0 |
On the diagonal of this table are 0, because this interaction of the channel with itself and the table is symmetrical with respect to the diagonal. It shows that the zero channel has a good effect on the first and third in terms of conversion, but with the other interactions of the problem. To practice this is usually enough.
How to count the number of interactions in Kazakhstan?
We have seen, for example, that everything is good with the interaction of channels and they have a positive effect on each other. But we still do not know how strong the effect is. Maybe there is interaction between the channels, and there is little interaction.
Let's try to do the same as in the previous case, look at the picture. But this time 1 (a). By the slope of this “straight line”, one can judge how the number of chains falls as the number of unique elements increases. It is logical to approximate it with the usual linear regression . In this case, the regression slope will be -522. Those. For chains of length 4, the number of chains in which there are 2 unique channels, rather than 1, is 522 fewer than the chains completely composed of one channel.
If we assume that we have 1600 chains with one channel and a positive conversion shift when moving from chains with one unique channel to chains with two 0.05 to 0.1, then reducing the slope from -500 to -200, for example, we will get 30 more conversions with the same conversion rate for chains with two unique channels. But there is a well-founded assumption that if this slope decreases, then the conversion will increase too, and not just the number of people for the same conversion will increase.
Choosing the right channels for your campaign
Well, here it is. The main question everyone constantly asks is: “What marketing channels should we use to make the most effective combination?”. There is a generally accepted rule of thumb: The more channels you initially use, the better. Then, after testing, you can theoretically determine which ones to remove from the campaign. The main problem here in practice has always been the criterion that would allow to remove one or another channel from the Republic of Kazakhstan. Suddenly you delete some, and he will do everything else worse, although he himself seems to bring nothing directly? We conducted an experiment to resolve this difficulty.
RK Optimization
The simplest: Simply eliminating the channel with the least amount of interaction, we raised the average hospital CR from 1.58% to 2.1% . The experiment was conducted under the condition that the marketing costs were the same across all channels.
But do not rush to run and do it right away. A low interaction channel is often a popular platform, such as Facebook. Its elimination may lead to the loss of customers. This is due to the fact that these large platforms do not always have many conversions, but always a lot of hits. Therefore, we decided to normalize the data using impressions, not clickthroughs, to determine overall performance. This was also done under the condition that each channel is the same.
When comparing channels with different costs, we divided the conversion rates by the cost of the channels in order to evaluate their true effectiveness. When we excluded the two lowest-performing channels for this criteria from our campaign, the average conversion rate dropped from 1.58% to 1.54%. However, costs decreased by 28.9%, which led to a 28% reduction in CPC!
Thus, a decrease in CR does not necessarily mean that you are wasting advertising dollars. The overall effect depends on the selected variables for calculating the ROI for this product.
What to consider when using channel interaction metrics?
- Do not create the appearance of the interaction of channels, inserting unnecessary links. This can make it difficult to effectively evaluate real interaction.
- If the channel does not interact with the others and is not very effective by itself, get rid of it.
- Perform an A / B test to find out why users choose a particular interaction.
- You can find out that your campaign is moving in the right direction, even before actual conversions. As the number of channel interaction increases, you already know that your campaign is on the right track.
- Do not use only one analytical tool, even if it is clearly the best. A variety of methods always improves results.
Additional evidence of interaction (section for nerds)
An experienced analyst will try to prove to you that the concept of channel interaction is simply cunning data manipulation. For example, we can assume that there is a very effective channel that also likes to be embedded in all the chains in a row. And therefore, the more unique channels in a particular chain, the more likely it is this MEGA channel, which increases conversion. From this and the increasing dependence in Figure 1 (b).
But no.
Certificate 1
To refute the foregoing, we present two graphs from the same data sets. The first figure shows the number of touches that each channel receives if all simple chains are selected for it. The second graph shows how each specific channel is converted if you select all simple chains for it.
![]() | ![]() |
Fig. 3 (a) The number of simple chains of a specific channel | Fig. 3 (b) Conversion rate of simple chains of a specific channel |
As can be seen from fig. 3 (a), channel 1 is the most popular. Therefore, if we have a chain and we are not sure which channels are in it, we can be sure that channel 1 will most likely appear somewhere in the (not simple) chain.
If channel 1 had the best CR, we could attribute the increase in CR for difficult chains to this channel, but no. Channel 1 for the chain length 4 has an average CR of 0.075 in Fig. 3 (b), not 0.3, as it was in fig. 1 (b), in which there were 4 unique channels in the chain.
Thus, the conversion rate of a particular individual channel or its “popularity” cannot explain the higher conversion rates found in non-simple chains. From this it becomes clear that the interaction of channels still exists.
Certificate 2
We used the available data to create a simple mathematical model that allows us to recalculate the probability of conversion without taking into account the interaction of the channels. In the “no interaction” simulation, we obtained a total conversion rate of 0.3%, rather than 1.58%.
We also run this simulation for two more datasets.
![]() | ![]() |
Fig. 4 (a) Data Set 1. Experiment (blue) versus model (green) | Fig. 4 (b) Data set 2. Experiment (blue) versus model (green) |
If it is interesting to someone, we will write the model itself in a separate article, but it is so simple that you yourself can guess how to make it.
PS We publish our articles on several sites Runet. Subscribe to our pages on the VK , FB or Telegram channel to learn about all of our publications and other news from Maxilect.