Calltouch multi-channel attribution
Introduction
In recent years, the toolkit of a modern Internet marketer is expanding more and more rapidly. Today, in addition to search engine optimization (
По оценке Calltouch cложность эта связана в первую очередь с тем, что пользователь со своей стороны обладает по сути тем же самым инструментарием, что и маркетолог: он может прийти на сайт как по прямой ссылке, так и по переходу из соцсетей, из рекламной выдачи Яндекса и. т. д. Более того, прежде чем совершить на сайте целевое действие (конверсию) пользователь может неоднократно посещать сайт из разных «точек входа»: первый раз он перешел на сайт, кликнув по рекламному объявлению (
Thus, when evaluating the effectiveness of advertising channels, the marketer first of all needs to answer the question: how to evaluate the contribution of a particular source to the formation of conversion on the site? In another way, this question can be formulated as follows: what will happen to the conversion on the site if one or another marketing channel is excluded? To answer this question, there are a number of methodologies called attribution models. Consider these models in more detail.
Attribution models
The attribution model is a way of distributing the conversion “weight” between channels. Depending on the choice of the attribution model, the weight of the channel (source) will be calculated, which can conditionally be considered the contribution that this source made to the formation of the conversion. Practically every user of Yandex Metrics or Google Analytics (the section “multi-channel sequences”) encountered these models. Currently, the following main attribution models are distinguished:
- By last interaction (last indirect interaction, last click in AdWords, last significant transition) -
- The first interaction is
- Linear Model -
- Temporary recession -
- Based on position -
As already noted, the main difference between attribution models among themselves is the method of calculating the weight of the channel in the sequence. Let's consider each model in more detail. For clarity, suppose that we have the following multi-channel sequence:
Last click model
This model, due to its simplicity and intuitive "correctness", is most widely used in practice. In the most general case, within
In practice, there are different varieties
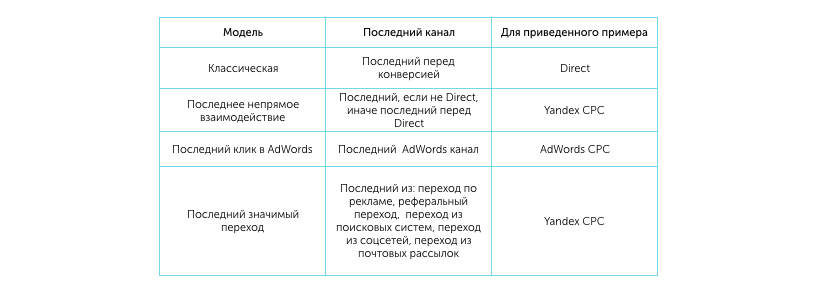
First click model
In this model
Linear model
Linear Model (
Time decay
Attribution model
Position Type Model
Attribution model
How to choose an attribution model?
The choice of attribution model is the most important step in evaluating the effectiveness of advertising. Depending on the model, the analyst may receive completely opposite conclusions about the profitability of a particular channel. This is especially clearly observed in topics where the decision-making process takes a lot of time (for example, in real estate or in automotive topics). A natural question arises: what attribution model should be taken as a reference? Unfortunately, there is no single answer to this question. Only a deep analysis of user behavior on the site (user sessions) will allow you to make an informed decision about choosing a particular method for linking conversions to a traffic source.
As a rule, the choice stops on the model
Separately, it is worth noting that the attribution model is the most important factor that should be taken into account when optimizing contextual advertising. Choosing a model directly affects the statistics that are used to calculate bids. If we consider that each key phrase is a separate advertising channel, then we can significantly enrich the statistics that go to the input of the optimizer, in addition, the analysis of successive user clicks between keywords will increase the efficiency of optimization. A separate chapter of this work will be devoted to the discussion of this topic.
Прежде чем перейти к описанию подхода, используемого нами для анализа многоканальных последовательностей, приведем «шуточный» пример, который с одной стороны покажет ограниченность классических моделей атрибуции, а с другой стороны позволит сформировать те основные вопросы, на которые следует найти ответ.
Допустим, целью является C=«увезти девушку к себе домой, чтобы посмотреть кино» .
Предположим, что мы имеем следующую цепочку действий (по сути каналов), которые привели к желаемой цели:
Познакомиться с девушкой → Пригласить в кино → Подарить цветы → Гулять вместе в парке → Проводить до дома → Пригласить на свидание в ресторан → Подарить цветы → Угостить ужином → Угостить коктейлем → Угостить еще одним коктейлем →… и еще одним → рассказать анекдот → C
If we are dealing with a model
As we see, none of the classical models can adequately describe the situation considered above, and even more so will not allow to correctly answer the question, which channel (action) turned out to be the most important in reality.
Now we formulate the main questions that I would like to receive answers from the attribution model:
- Is it enough to just tell a joke? And if so, how often?
- How typical is the practice of telling jokes to achieve a goal?
- What happens if you don’t tell a joke?
- Is it possible to replace the joke with some other action? If so, which should be replaced?
For the correct answer to most of the questions posed, it is not enough for us to consider only one sequence. It is necessary to collect some statistics that would allow one to predict user behavior, on the one hand, and would assess the likelihood of conversion on the site for each of the interaction points.
The model we are considering was originally developed for the combined assessment of multichannel sequences, assuming that the channels are interdependent. It allows you to answer most of the questions formulated above. In addition, we will show how the methods described by us allow us to predict the conversion rate for each key phrase, which is a necessary element in optimizing bids in contextual advertising.
First of all, we will describe the data format with which our model works.
Custom sessions
Suppose that for some period of time we are analyzing
Where:
- the channel through which the transition to the site was made
- session start time
- session end time
- the address of the page that the user visited when going to the site
- unique user identifier
- whether the conversion was made as a result of the session (
- Yes,
- not)
Further, for simplicity, we will assume that the period of time
- Yandex CPC
- Google CPC
- Vkontakte
- Direct
- Referal
- etc.
For simplicity, we will encode advertising channels as follows:
Now suppose that
Where
Where
We introduce two additional “pseudo-channels”
- If during the session
user with source
there was a conversion, then after
add
having received
- If as a result of the last current session
with source
conversion did not happen then after
add
having received
In addition, we additionally draw attention to the situation when we are dealing with chains of the form:
Sequences with such a structure cannot occur according to the rules formulated above, but nevertheless they can occur in a number of cases, for example, in caller topics, when in addition to the session parameters indicated above we have a unique combination:
In this case, the first call in the above chain will be a unique call, and all subsequent calls will be repeated calls of the subscriber with the specified
We note a key feature of the methodology described above for forming user-site interaction chains. It lies in the fact that any chain of interaction (multi-channel sequence) always ends with one of two “events”:
We give typical examples of sequences formed according to the described rules. For simplicity, we restrict ourselves to 3 different channels
The next step required to build a multi-channel attribution model is to transform the sequences so that the event
We demonstrate this technique using typical sequences as an example:
- chains 1-4 are already reduced to the "elementary" form
- chain 5 "split" into:
and
- chain 6 "split" into:
and
- chain 7 is “split” into:
and
- chain 8 is “split” into:
,
and
As a result of splitting, all chains have become "elementary", and now we can begin to describe the model. However, before moving on to this step, at this stage we can already answer the question: how to assess the impact of the channel on the conversion on the site.
Calculation of the influence of channels on conversion
Consider the set of
Obviously, for any
Calculate the effects of channels
It is easy to replace that the sum of the channel influences is not equal to unity. For convenience, you can enter a normalization and consider the normalized effect
In this case, obviously
The formula for calculating the influence of the channel on conversion can be easily modified for the case when it is necessary to evaluate the influence of one channel on another. In particular, if the task is to find out how the channel affects
In general, the function
Assessment of changes in basic metrics when a channel is disabled
After answering the question, how will the number of conversions change when you delete a channel from all chains
- consumption
- conversion cost (CPA)
It is rather difficult to answer these questions without involving additional assumptions. Our basic axiom is that when you delete a channel
This assumption means that if you remove the channel that the user used to interact with the site, then there will be no further user interaction with this site.
To evaluate the basic metrics, we also need to add an indicator such as “transition cost” to the parameters of user sessions. It can be interpreted as the cost paid by the advertiser for the user’s click on this channel, if the channel is free (such as a direct transition), then we assume that the cost of the transition is
At the same time, the total cost per channel
The total cost of attracting users to the site when using channels
The duality of the formula is explained by different ways of calculating the total costs: in the first case, we summarize the costs for each of the chains for all
To estimate new costs after removing from all channel chains
It's obvious that
which means
The last inequality means that removing any channel
Now, after we learned how to measure the change in spending after deleting the channel
If we assume that before the removal of the channel, we had the previous conversion cost:
That is, if the removal of the channel leads to a decrease in the cost of conversion (with a reasonable decrease in their number), then it can be excluded from the chain and stop spending the budget on it.
In addition, you can estimate the cost of "lost conversions" when you delete a channel:
Now we proceed to describe the basic model required to calculate the probability of channel conversion.
Model description
Прежде чем мы приступим к описанию многоканальной модели атрибуции, нам бы хотелось сослаться на замечательные статьи Сергея Брыля, и вторую статью, в которых автор использовал красоту и функциональность марковских цепей для описания многоканальной атрибуции. В рамках данной статьи мы более подробно описали основные моменты, связанные с расчетом вероятности конверсии в рамках марковских процессов, а также предложили эффективный метод вычисления вероятности конверсии – на основании стохастических матриц.
We will offer two alternative interpretations of the multichannel attribution model: graph and matrix. The first allows you to clearly describe the model, while the second allows you to effectively calculate the required characteristics. We show that both descriptions actually represent the same random process, which is called the Markov process, and the model corresponding to the process, the Markov chain.
Graph model
A graph is an abstract mathematical object, which is a set of graph vertices and a set of edges, that is, connections between pairs of vertices. For example, for many peaks you can take many airports served by a certain airline, and for many edges take regular flights of this airline between cities.
A graph is called oriented if each of its edges has a direction, i.e., it is essentially a vector: for an edge it is precisely indicated from which vertex it originates and to which it ends.
A graph is called weighted if each of its edges is assigned a numerical value called a weight. A typical example of a weighted oriented graph is a network of roads between cities (vertices of the graph), where by the weight of an edge (road) we understand its length.
In order to represent the set of chains in the form of a graph, we need to fix two sets: the set of vertices
As
In view of the fact that in the set

As can be seen, even for a small number of sessions, such a graphical representation is rather cumbersome, which complicates the analysis. Some simplification can be achieved by replacing duplicated ribs with one rib, taking the number of takes as a weight. Then the original graph is transformed into a directed weighted graph:
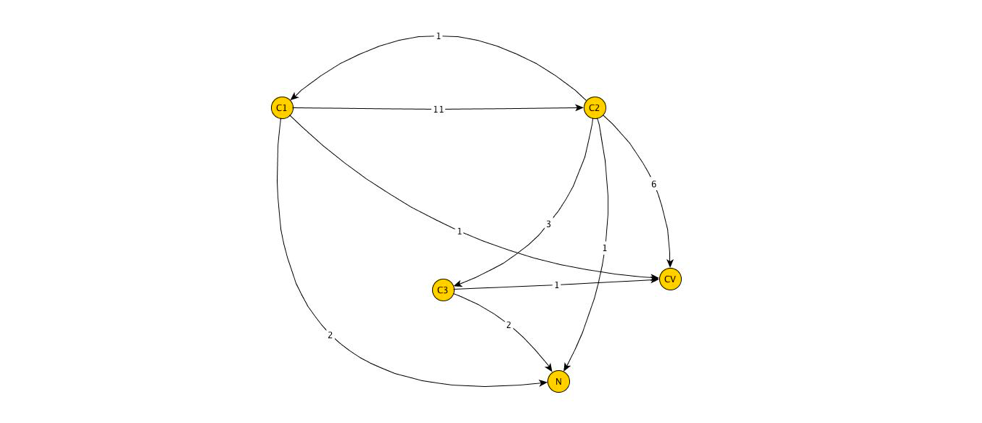
This graph is already more suitable for analysis. Our next goal is to convert the edge weight to probabilistic notation. Replace the weight of the edge connecting the two vertices with the probability of transition from one vertex to another.
In particular, consider the top
Easy to replace that

Based on this model, we can calculate the total conversion probability for a particular channel. For calculation, the following recursive formula is used:
Смысл этой формулы в том, что для того, чтобы рассчитать полную вероятность конверсии некоторой вершины, требуется выбрать все вершины, достижимые из данной, затем рассчитать вероятности перехода в эти вершины из исходной, а затем для каждой достижимой вершины снова рассчитать полную вероятность конверсии. Данная формула тут же дает полную вероятность конверсии, если граф является однонаправленным, т. е. если есть ребро, соединяющее вершины
Например, рассчитаем полную вероятность конверсии
Так как
In turn from
then
For convenience, we denote
Now calculate
Finally, we have the following equation:
Where from
The main advantage of the above model is its visibility, while obvious disadvantages (which can be seen even with a simple example) include high computational complexity for the case of a large number of traffic sources. Moreover, if different keywords are used as sources, the volume of calculations increases by orders of magnitude, which will make all subsequent calculations unrealizable. In addition, if we allow the possibility of transitions in a graph of the form:
Matrix model
In the previous chapter, we examined the graph model of multichannel attribution. In order to convert it to a more convenient form for calculations, we again consider a set of
From the observed sequences compiled for each of the users, we can easily calculate the transition probabilities (in other words, conditional probabilities)
and
In particular, for the above example, we get:
It is easy to see that for any
A matrix for which this condition is satisfied is called stochastic. It is known that an arbitrary stochastic matrix defines some random process called Markov process. Let us give such a process a more formal (although not rigorous from a mathematical point of view) definition.
A Markov process is such a random process with a certain number of states that the probability of transition to the next state depends only on the current state in which the system is located.
Thus, the transition process we are considering between the various marketing channels can be considered a Markov process, determined by a matrix of transition probabilities
- What is the probability of moving from a state
to state
behind
steps?
- How the probability distribution of being in each channel through
steps?
In our applied problem of estimating the conversion probability of each channel, we need to answer a particular case of the first question:
What is the total probability of passing from the state (channel)
The Markov theory of random processes allows us to give a very simple answer to this question (in the case where the states
It can be rigorously proved that for the case when from the states
In fact, let, for example,
We show on our example the speed of "convergence" of the limit to the probability we need:
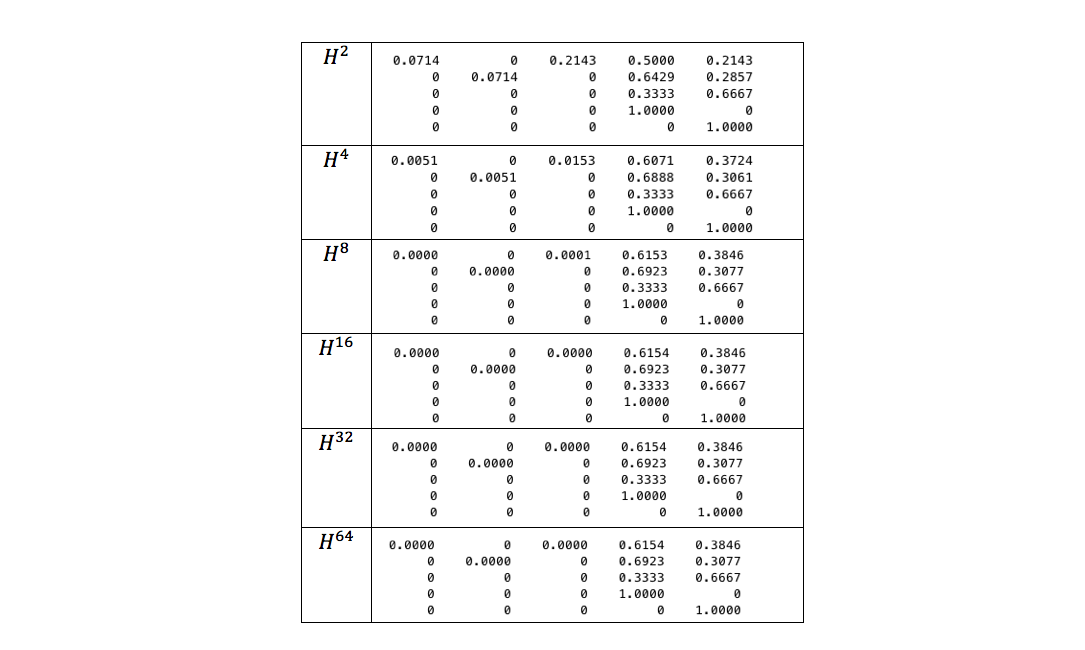
As can be seen from the table, for
From channel assessment to optimization
The constructed analytical model allows us to solve 3 main problems:
- Assess the impact of the channel on the conversion on the site
- Assess the mutual influence of channels on each other
- Assess the likelihood that using the channel will lead to a conversion on the site
When designing a conversion optimizer that allows you to manage bids in contextual advertising based on their performance so that they achieve the desired
The presented conversion attribution model is free from these drawbacks, although it requires significantly more computing resources to calculate probabilities. The flexibility of the described approach also lies in the fact that we can use any integral attribute of a session as a “channel”.
In particular, we consider the parameter
site.ru/?utm_source=YD&utm_medium=cpc&utm_content=kvartiry_ceny&utm_campaign=YD_KVARTIRY_POISK_MSK&calltouch_tm=yd_c:{campaign_id}_gb:{gbid}_ad:{ad_id}_ph:{phrase_id}_st:{source_type}_pt:{position_type}_p:{position}_s:{source}_dt:{device_type}_reg:{region_id}_ret:{retargeting_id}_apt:{addphrasestext}
На основе динамических параметров, которые содержатся в фигурных скобках, мы можем, в частности, отследить «путь» клика на рекламное объявление с точностью до ключевой фразы, которая инициировала показ рекламного объявления, на которое кликнул пользователь. Мы можем выбрать любой «разумный» динамический параметр (или их связку) в качестве канала. В частности, если выбрать в качестве канала параметр
The resulting array of conversion rates can be used as input to the conversion optimizer.
Conclusion
The article gives an overview of currently used classic models of conversion attribution. In addition, a multi-channel attribution model based on Markov processes (chains) is described, which allows you to comprehensively evaluate both the probability of conversion for each advertising channel and calculate the channel’s effect on conversion on the site. Demonstrated approaches to adapt the built model to optimize bids in contextual advertising.