Data Science Week 2016. Overview of the first and second day
Habr, hello! We publish a review of the first two days of Data Science Week 2016 , in which our speakers talked about customer relationships and internal optimization.
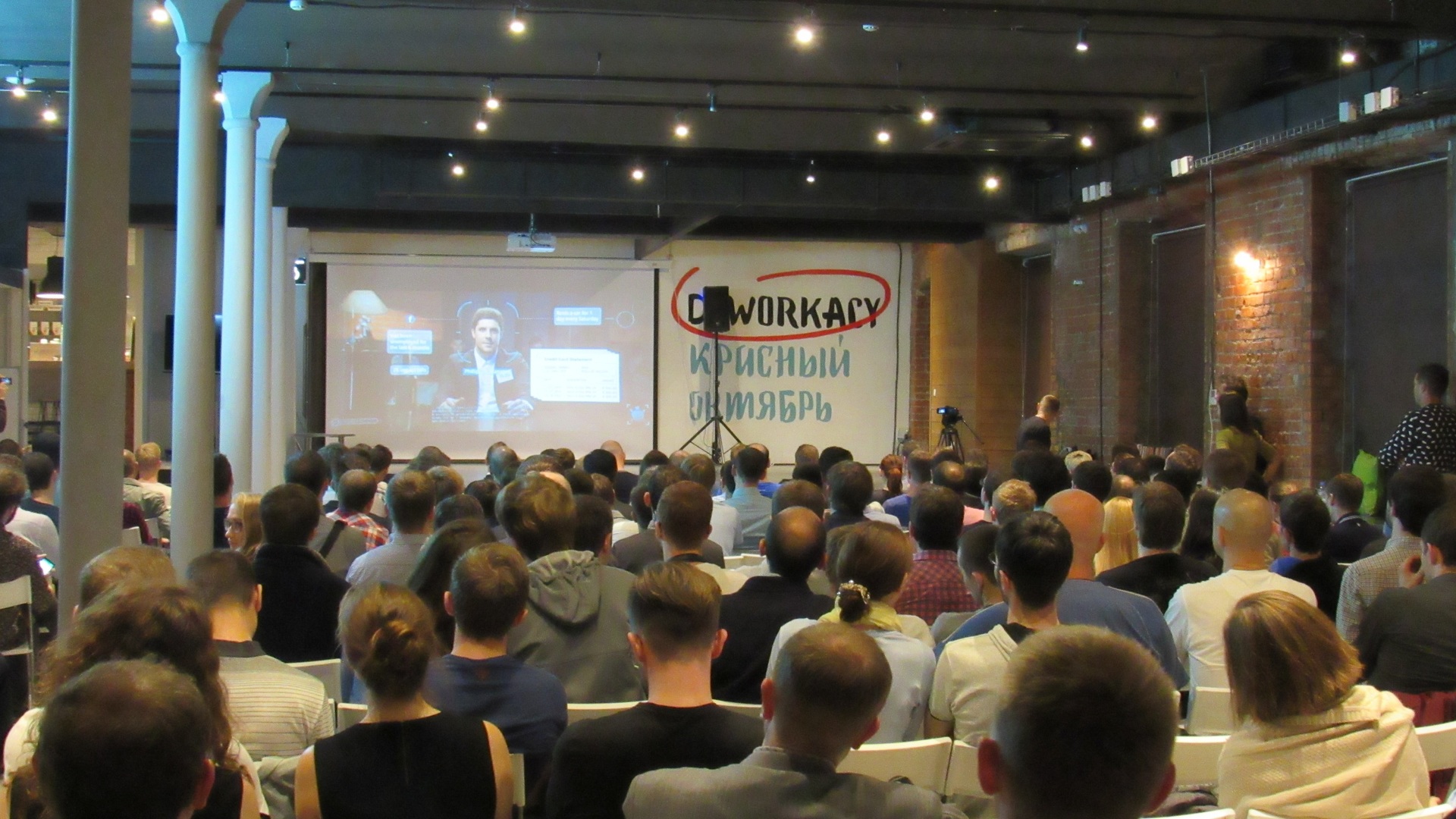
The first day of Data Science Week 2016 was dedicated to the use of big data in customer relationships. The specific algorithms and technologies used were hardly mentioned; the emphasis was placed on the results obtained and the directions of their application in business.
Almost all speakers touched upon the topic of recommendation systems: what media content, what housing rental options, what advertising to offer the user? It was also talked about using big data to attract and retain customers, create effective and transparent forms of working with them, and improve the quality of customer service. We also talked about aggregating and verifying the offers available on the market, about using big data to analyze the effectiveness of marketing channels.
The companies represented on this day collect a lot of data about users, analyze and extract business value from them.
In the media industry, this is, first of all, the history of users consuming content: there is a lot of such data, because people listen to music daily, read articles and books, watch films and videos. The information specified by the user during registration is also used if the user is authorized through social networks, information about him is taken from there as well. Based on this data from E-Contentamanages to solve a wide range of problems. This is a personalized attraction (offering interesting content instead of advertising the resource as a whole), highlighting the profiles of individual users when sharing devices (for example, when the family has one TV), recommending trending content, customer retention, and transferring to new content (for example, from one series to another), remarketing (offering new interesting content to a “tired” or disinterested user), recommending future content that will only be created and about which little else estno.
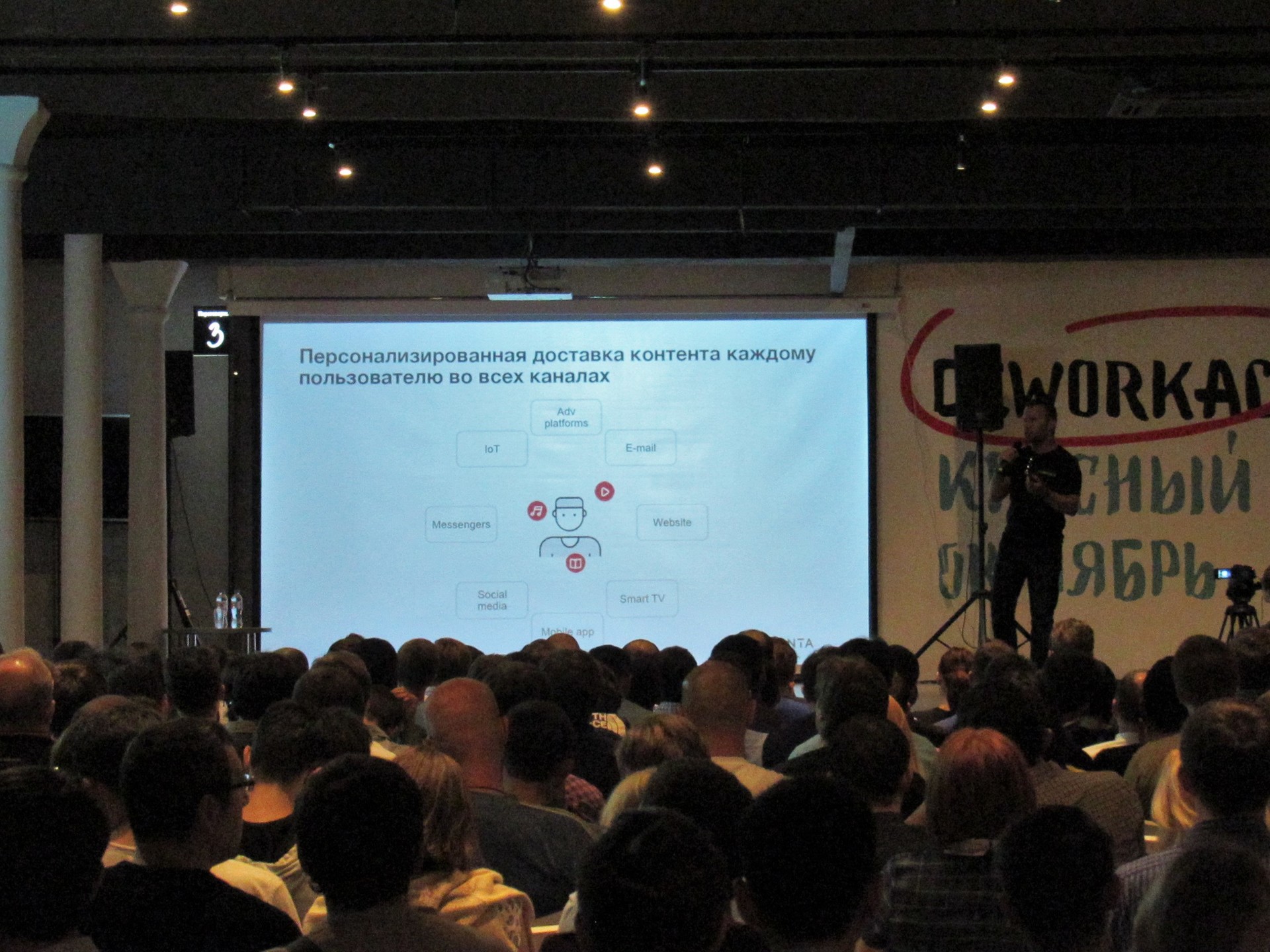
Users on the real estate market make transactions very often, so the company HomeAppcollects only information about the user's browsing history of rental advertisements for a given period. This information, as well as the collected database of announcements and the results of price monitoring, are visualized and used by company employees to recommend specific offers to customers. The company makes extensive use of expert methods; automatic recommendations have not yet been built. The main emphasis is made precisely on the preparation of a database of offers: data on renting apartments is collected from social networks, from websites for posting ads, from websites of agencies and various aggregators (for example, CIAN, Avito). Then, data analysis methods eliminate duplicates, exclude fraudulent ads used only to attract customers, and verify the information specified in the ads.
Company RockStat is analyzing the effectiveness of digital marketing channels, the definition of what kind of visit of the resource had the desired effect, led to the conversion of view in the purchase, etc. To do this, the following data is collected and analyzed: page views, events that occurred on the pages, activity (mouse movements, scrolling, focus changes), data of third-party services via http requests, data on calls from visitors to the site and applications left on the site, as well as CRM system data (in order to understand exactly which appeal led to the sale). From these data, sessions are built: it is determined where the user came to the site from, where he is located, from which device he logged in to collect them in chains by users, clear of “noise” and calculate the value of each session in the chain.
The company DCAsuggests using rather unexpected data sources: information about applications installed on the user's phone, including even the color of the icons. The fact is that advertising platforms do not provide enough information about the user, and this is one of the available open sources. By the range of installed applications (those in which ads are shown), you can predict the gender and preferences of the user. To evaluate the audience of applications, the reviews written in the Play Market are used (for example, by literacy, blackmail and expression of general opinion, you can determine the child's age, by name - gender), Google Play recommendations on similar applications. The company also uses geolocation data to determine the time zone and generate geo-targeted offers (for example, order food from a restaurant near the client).
Thus, the first day of Data Science Week showed a number of examples of how big data analysis makes it possible to understand what to offer to a specific user and through which channels, to form a reliable and transparent database of offers, improve the quality of customer service, customer satisfaction and loyalty, and thereby increase efficiency business.
The second day of Data Science Week was devoted to optimizing the internal processes of companies. Part of the speeches was devoted to optimizing the work with data, another part - optimizing internal processes using big data, one presentation was devoted to improving the quality of work with clients and the services provided to them, and most likely related to the first day.
The first speaker, Andrei Kotov, representing the company GlowByte, spoke about the culture of working with data within companies. In many big data projects in which he had to participate, clients were not ready to provide quality data. In addition to typical data problems: duplicates, errors and contradictions, lack or redundancy of information, the report also highlighted the problem of the lack of a unified standard for data recording, inconsistency of recorded data with objectively necessary categories. For example, in one of the fashion industry companies, the color and type of clothes were fixed very subjectively and in different ways by designers, storekeepers and other participants in the process, and in food retail tulips were categorized as vegetables of first freshness, which made it difficult to make recommendations. According to the speaker, companies need to instill a culture of working with data, so that employees understand their value, carefully and unambiguously write them down, trying to maintain relevant information. This will help the market as a whole, facilitate the work of ordinary analysts and big data companies.
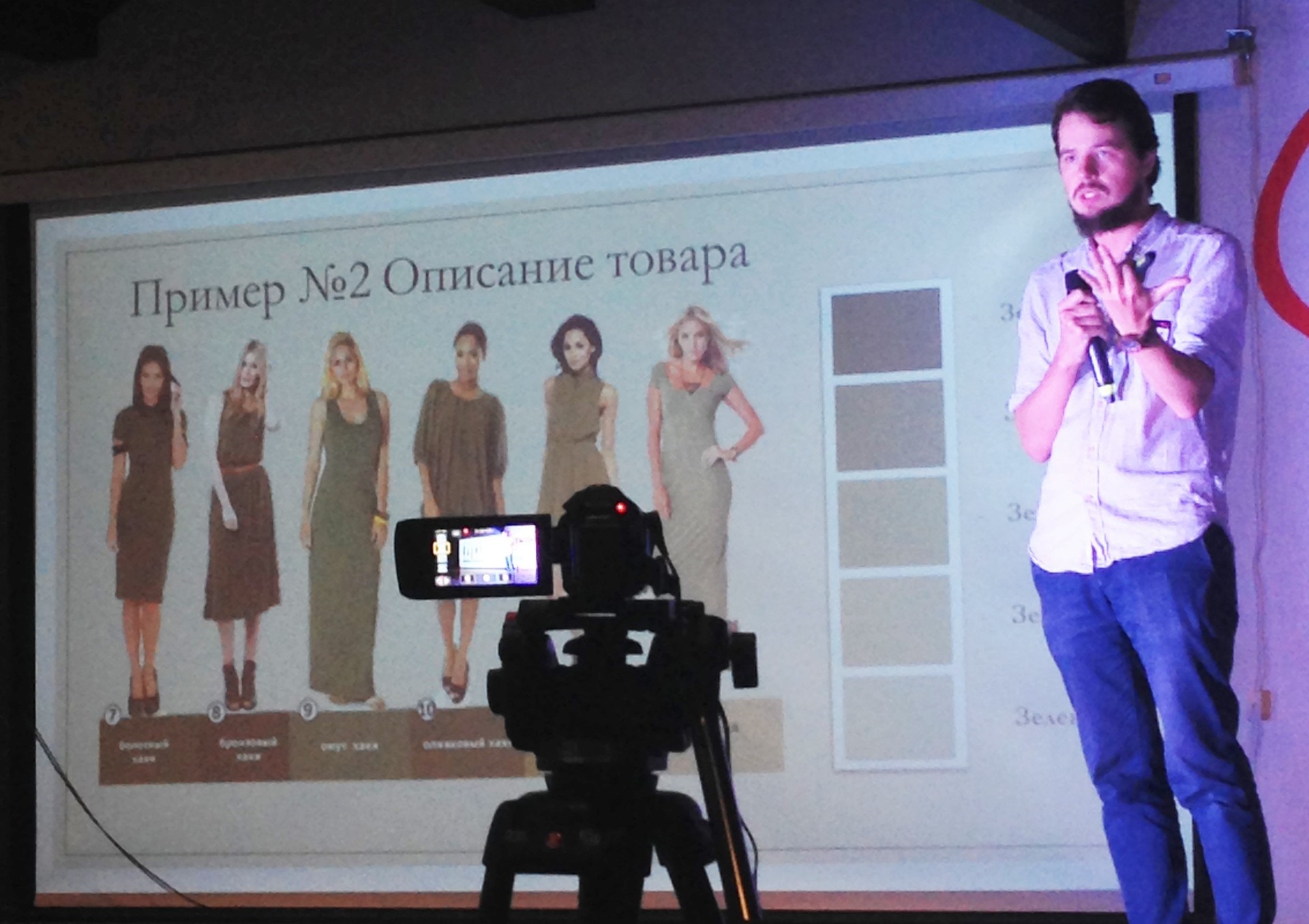
Vadim Chelyshkov from Microsoft spoke about the use of data from various sensors that monitor the condition of equipment to increase its reliability and also for personal purposes. Through the Internet of Things, sensors send huge amounts of real-time data to servers. Based on the analysis of such data, systems were developed that predict the date and type of possible breakdown, in particular, elevators and pumps on oil platforms. As an example of using this data for personal purposes, the speaker cited the product of the Russian company Raxel Telematics, which allows for several months to confirm the status of a neat driver and reduce the price of insurance based on data from car sensors.
Dmitry Garmashev from QIWIHe spoke about the analysis of money transfer graphs between customers of the Kiwi Wallet service: using an algorithm developed at the Belgian University of Leuven, it was possible to establish a quick breakdown of service customers into communities and identify the roles of individuals within them. For example, it was possible to identify a community of users of one of the online games, within which sellers and buyers of cheat codes stood out. Combining them in one platform allowed to increase the number of transactions. An analysis was also made of the content of messages and the lifetime of wallets in order to detect fraud. To work with graphs, the speaker recommended the Python NetworkX library, as well as the visualization tools Gephi and D3.
Speech by Pavel Klemenkov from Rambler & CoIt was devoted to optimizing the processing and analysis of big data within the company based on Apache Spark. He talked about collecting and visualizing data, about the work of running procedures, in particular, about the success and time of their execution, about the causes of errors. The speaker shared his experience in separating experiments with data and production code, writing tests for all operations before running them on large amounts of data, developing a “showcase of features” - a means of quickly selecting data in a training set, creating a system of timely alerts about emerging problems with the ability to call responsible persons . As a result of the implementation of the described system in the company, the number and speed of experiments was increased, simple and convenient testing, debugging and implementation of the code became possible, the reliability of operations increased, it became easier to understand and eliminate the causes of errors.
Finally, Alexander Laryanovsky of companies SkyEng, specializing in private English lessons, spoke about the use of data in building customer relationships and optimizing the content of lessons. For example, it turned out that according to a number of behavioral characteristics, it is possible to predict whether the client will quit classes and how much he will be willing to pay. “Larks”, who prefer early classes, turned out to be more motivated, like those who formulated any requirements for him when looking for a teacher. The content of the lessons was brought into line with the interests of the client based on the data of his profiles on social networks, which allowed to increase the conversion after the trial lesson by 20%. Based on the statistics collected from students, it was possible to optimize teaching methods: remove unnecessary exercises that most students cope with,
»All presentations are available here.
»Access to video speeches can be obtained here.
Day 1
The first day of Data Science Week 2016 was dedicated to the use of big data in customer relationships. The specific algorithms and technologies used were hardly mentioned; the emphasis was placed on the results obtained and the directions of their application in business.
Almost all speakers touched upon the topic of recommendation systems: what media content, what housing rental options, what advertising to offer the user? It was also talked about using big data to attract and retain customers, create effective and transparent forms of working with them, and improve the quality of customer service. We also talked about aggregating and verifying the offers available on the market, about using big data to analyze the effectiveness of marketing channels.
The companies represented on this day collect a lot of data about users, analyze and extract business value from them.
In the media industry, this is, first of all, the history of users consuming content: there is a lot of such data, because people listen to music daily, read articles and books, watch films and videos. The information specified by the user during registration is also used if the user is authorized through social networks, information about him is taken from there as well. Based on this data from E-Contentamanages to solve a wide range of problems. This is a personalized attraction (offering interesting content instead of advertising the resource as a whole), highlighting the profiles of individual users when sharing devices (for example, when the family has one TV), recommending trending content, customer retention, and transferring to new content (for example, from one series to another), remarketing (offering new interesting content to a “tired” or disinterested user), recommending future content that will only be created and about which little else estno.
Users on the real estate market make transactions very often, so the company HomeAppcollects only information about the user's browsing history of rental advertisements for a given period. This information, as well as the collected database of announcements and the results of price monitoring, are visualized and used by company employees to recommend specific offers to customers. The company makes extensive use of expert methods; automatic recommendations have not yet been built. The main emphasis is made precisely on the preparation of a database of offers: data on renting apartments is collected from social networks, from websites for posting ads, from websites of agencies and various aggregators (for example, CIAN, Avito). Then, data analysis methods eliminate duplicates, exclude fraudulent ads used only to attract customers, and verify the information specified in the ads.
Company RockStat is analyzing the effectiveness of digital marketing channels, the definition of what kind of visit of the resource had the desired effect, led to the conversion of view in the purchase, etc. To do this, the following data is collected and analyzed: page views, events that occurred on the pages, activity (mouse movements, scrolling, focus changes), data of third-party services via http requests, data on calls from visitors to the site and applications left on the site, as well as CRM system data (in order to understand exactly which appeal led to the sale). From these data, sessions are built: it is determined where the user came to the site from, where he is located, from which device he logged in to collect them in chains by users, clear of “noise” and calculate the value of each session in the chain.
The company DCAsuggests using rather unexpected data sources: information about applications installed on the user's phone, including even the color of the icons. The fact is that advertising platforms do not provide enough information about the user, and this is one of the available open sources. By the range of installed applications (those in which ads are shown), you can predict the gender and preferences of the user. To evaluate the audience of applications, the reviews written in the Play Market are used (for example, by literacy, blackmail and expression of general opinion, you can determine the child's age, by name - gender), Google Play recommendations on similar applications. The company also uses geolocation data to determine the time zone and generate geo-targeted offers (for example, order food from a restaurant near the client).
Thus, the first day of Data Science Week showed a number of examples of how big data analysis makes it possible to understand what to offer to a specific user and through which channels, to form a reliable and transparent database of offers, improve the quality of customer service, customer satisfaction and loyalty, and thereby increase efficiency business.
Day 2
The second day of Data Science Week was devoted to optimizing the internal processes of companies. Part of the speeches was devoted to optimizing the work with data, another part - optimizing internal processes using big data, one presentation was devoted to improving the quality of work with clients and the services provided to them, and most likely related to the first day.
The first speaker, Andrei Kotov, representing the company GlowByte, spoke about the culture of working with data within companies. In many big data projects in which he had to participate, clients were not ready to provide quality data. In addition to typical data problems: duplicates, errors and contradictions, lack or redundancy of information, the report also highlighted the problem of the lack of a unified standard for data recording, inconsistency of recorded data with objectively necessary categories. For example, in one of the fashion industry companies, the color and type of clothes were fixed very subjectively and in different ways by designers, storekeepers and other participants in the process, and in food retail tulips were categorized as vegetables of first freshness, which made it difficult to make recommendations. According to the speaker, companies need to instill a culture of working with data, so that employees understand their value, carefully and unambiguously write them down, trying to maintain relevant information. This will help the market as a whole, facilitate the work of ordinary analysts and big data companies.
Vadim Chelyshkov from Microsoft spoke about the use of data from various sensors that monitor the condition of equipment to increase its reliability and also for personal purposes. Through the Internet of Things, sensors send huge amounts of real-time data to servers. Based on the analysis of such data, systems were developed that predict the date and type of possible breakdown, in particular, elevators and pumps on oil platforms. As an example of using this data for personal purposes, the speaker cited the product of the Russian company Raxel Telematics, which allows for several months to confirm the status of a neat driver and reduce the price of insurance based on data from car sensors.
Dmitry Garmashev from QIWIHe spoke about the analysis of money transfer graphs between customers of the Kiwi Wallet service: using an algorithm developed at the Belgian University of Leuven, it was possible to establish a quick breakdown of service customers into communities and identify the roles of individuals within them. For example, it was possible to identify a community of users of one of the online games, within which sellers and buyers of cheat codes stood out. Combining them in one platform allowed to increase the number of transactions. An analysis was also made of the content of messages and the lifetime of wallets in order to detect fraud. To work with graphs, the speaker recommended the Python NetworkX library, as well as the visualization tools Gephi and D3.
Speech by Pavel Klemenkov from Rambler & CoIt was devoted to optimizing the processing and analysis of big data within the company based on Apache Spark. He talked about collecting and visualizing data, about the work of running procedures, in particular, about the success and time of their execution, about the causes of errors. The speaker shared his experience in separating experiments with data and production code, writing tests for all operations before running them on large amounts of data, developing a “showcase of features” - a means of quickly selecting data in a training set, creating a system of timely alerts about emerging problems with the ability to call responsible persons . As a result of the implementation of the described system in the company, the number and speed of experiments was increased, simple and convenient testing, debugging and implementation of the code became possible, the reliability of operations increased, it became easier to understand and eliminate the causes of errors.
Finally, Alexander Laryanovsky of companies SkyEng, specializing in private English lessons, spoke about the use of data in building customer relationships and optimizing the content of lessons. For example, it turned out that according to a number of behavioral characteristics, it is possible to predict whether the client will quit classes and how much he will be willing to pay. “Larks”, who prefer early classes, turned out to be more motivated, like those who formulated any requirements for him when looking for a teacher. The content of the lessons was brought into line with the interests of the client based on the data of his profiles on social networks, which allowed to increase the conversion after the trial lesson by 20%. Based on the statistics collected from students, it was possible to optimize teaching methods: remove unnecessary exercises that most students cope with,
»All presentations are available here.
»Access to video speeches can be obtained here.