
Why fintech machine learning
Computers appeared in the 80s, and gradually we watched how their use for storing and processing information became the norm for most companies. In the 90s, we witnessed an Internet boom that truly changed the world. Gathering information about anything today is a mere trifle. In the middle of the last decade, social networks appeared and entrepreneurs noticed that customers began to spend as much time in them as they had before on any other site. As a result, businessmen around the world began to invest in social media to increase audience reach and for marketing purposes. When Android and iOS were introduced to the general public, a paradigm shift took place. People began to spend more time with their smartphones than personal computers. Over time, consumers began using smartphones to make decisions, make purchases, and even make payments. Today, realizing that smartphones have become an integral part of the consumer decision-making process, companies are striving to provide them with omni-channel interaction experience. In this regard, the question arises: “What other innovative tools exist that can change the market?” Companies should probably pay attention to the use of machine learning algorithms.
Let me illustrate the benefits of algorithms with a small example. Dash Financial , one of the leaders in the fintech market, recently won the Waters Technology Awards in the category “Best Algorithmic or Direct Market Entry Product for Investors”. Let's take a look at the functionality that the algorithms of this company offer. The Dash toolkit is designed to optimize performance and reduce commission costs. The ability to customize the behavior of the system at any level and in any category is one of the distinguishing features of the product. Combined with the desire to provide full transparency in real time for all operations, the company's customers receive a full, investor-oriented trading infrastructure.
The set of sensory algorithms Dash has its own configuration wizard, a patented development of the company that allows you to adjust the parameters, behavior and techniques in accordance with the goals and needs of the investor without the need to rewrite the program code or work within long development cycles. The results of any changes are instantly and clearly displayed in the control panel - a detailed, real-time web interface that allows you to access each component of the process of accepting and executing applications. Dash customers get the opportunity to gradually monitor where their applications are sent, as well as the state of the market and the depth of quotes up to the microsecond during their execution. This tool is a key element of algorithmic identification, analysis and further optimization and adaptation to the goals of the investor.
Over the past 10 years, companies have managed to collect a lot of data using a variety of channels, and now it's time to apply algorithms to this array of information. They will help companies go beyond traditional reporting and gain deeper insight into the information they collect. They will also be useful in analytic forecasting, helping companies make decisions instantly. Data collection, identification of patterns, smart classification and machine learning will change the situation in various industries in the next five years. And the Fintech market is definitely not going to stand aside. Today, fintech segments such as exchange trading and lending have already integrated algorithms in their activities to accelerate decision-making. It is safe to say
Below is a list of companies that use in their work an extensive and one of the most popular types of artificial intelligence today - machine learning.
18 fintech machine learning players
Machine learning is a form of artificial intelligence that provides computers with the opportunity to learn without any strictly programmed behavior scenarios. Specialists in this field are developing computer programs that can learn on their own, grow and change based on new data. Young fintech companies use this technology to adapt to constantly changing market conditions.
Here are some of these companies:
Kensho: The company's developments combine the use of machine intelligence, work with natural languages, graphical user interfaces and secure cloud computing and represent a new class of analytical tools for investment professionals. Kensho smart computer systems are able to answer complex financial questions asked in plain English and, according to information on the company's official website, "are able to solve the most complex analytical tasks of our time." The company was founded by graduates of Harvard and MIT, it employs experienced former employees of Google, Apple and the US Federal Reserve, and its investors include such names as Google Ventures, Goldman Sachs, In-Q-Tel (CIA venture unit).
Affirm: A financial company whose technological tools collect huge amounts of data for their effective application in assessing lending parameters. Machine learning is used to protect against fraud and collect credit data.
Lending Club : The world's largest online marketplace for borrowers and investors. The platform uses machine learning to predict potentially bad loans.
Kabbage: An online company in Atlanta specializing in financial technology and data collection. Kabbage offers direct financing services to small businesses and consumers through an automated lending platform. The Kabbage team specializes in developing next-generation machine learning tools and a set of analytical tools for creating models for assessing credit risk and analyzing existing loan portfolios.
ZestFinance : The firm uses machine learning techniques and large data analysis to make more accurate loan decisions. According to the company’s website, the traditional approach to assessing a credit rating uses only 50 parameters, which is only a small part of the amount taken into account by ZestFinance algorithms.
Billguard: Provides personal financial security services. Billguard protects users from theft of personal data during financial transactions, as well as erroneous charges and gray transactions. The company specializes in the use of technologies such as data mining, machine learning algorithms, security, and the design of user-friendly web interfaces.
LendUp : The company specializes in microcredit, including allowing other organizations to provide similar services using their own API. LendUp uses machine learning and algorithms to accurately identify those 15% of borrowers who are statistically most likely to be able to repay loans.
Bloomberg: One of the leading providers of information for professional participants in financial markets. Bloomberg quickly provides accurate business and financial information, news and expert opinions from around the world. Using methods of statistics, natural language processing and machine learning, the company offers analytical solutions for the financial community.
AlphaSense : A financial search engine that solves the fundamental problems of excess information and its fragmentation. The target audience of the company is professionals from various fields of the financial sphere. AlphaSense effectively leverages its proprietary proprietary natural language processing and machine learning algorithms, providing users with a powerful and highly differentiated product with an intuitive interface.
Fingenius: A platform focused on working with banks and insurance companies. The FinGenius technology suite is a combination of different artificial intelligence techniques, including machine learning, natural language processing, and human logic modeling to simplify the processing of complex data arrays.
Dataminr : The company provides information retrieval services for clients from the financial sector. Dataminr tools in real time “comb through” social networks and other open sources of information using machine learning algorithms in search of important information elements and their subsequent transformation into useful recommendations and practical tips.
Binatix: A stock broker that uses modern patented artificial intelligence algorithms that take into account the factors of biological behavior of people and use them for large-scale data analysis.
Brighterion : The company offers one of the world's largest sets of artificial intelligence and machine learning technologies, capable of collecting and analyzing information from sources of any type, complexity and volume.
Feedzai : The company uses machine learning and Big Data to ensure the security of its customers ’business operations. Feedzai’s self-learning models recognize fraud 30% earlier than traditional methods.
Nymi (formerly Bionym): The company has developed and is promoting a biometric authentication device using an electrocardiogram, using, among other things, machine learning algorithms.
EyeVerify : EyeVerify proprietary software uses the so-called eye prints - vascular patterns in eye proteins as an identifier, also using machine learning technology for these purposes.
BioCatch : A leading provider of behavioral biometrics, authentication, and malware detection solutions for mobile and web applications. Banks and online stores use Biocatch to avoid conflicts associated with risky transactions and protect users from cyber threats, such as account hijacking, browser trojans and remote access attacks.
KFL Capital: The company specializes in analyzing financial patterns and price fluctuations and finding the most profitable ones. For these purposes, KFL Capital uses machine learning, forecasting algorithms, statistical methods and powerful computing computers.
MasterCard Machine Learning Network Disrupting ATM Attack
Special attention should be paid to the news about the machine learning technology of the payment giant MasterCard, which made it possible to quickly take control of 3 separate cyber attacks aimed at the ATM network, limiting the total damage to about $ 100 thousand in each case.
The operations monitoring system, which also includes visualization tools, recorded three attacks that occurred, according to MasterCard, in January-February 2016. The
global Safety Net system, launched last year, analyzes more than 1.3 billion transactions involving MasterCard debit and credit accounts, merchants and ATMs per day. For this, the system applies algorithms for assessing customer behavior in real time.
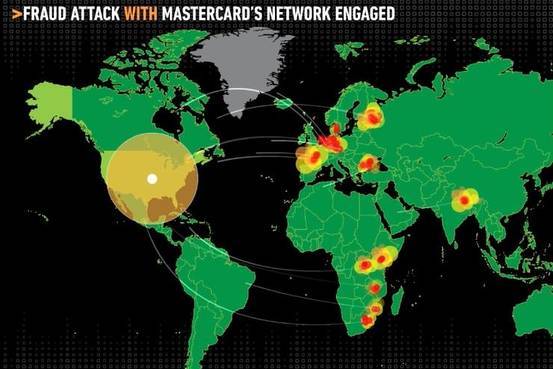
Scheme of the 2013 cyber attack, which began in one of the banks of the UAE and in an American company engaged in the processing of data on credit and debit cards.
MasterCard Safety Net's Fraud Detection System has detected activity spanning over 300 ATMs in 26 countries. Then, within 11 hours, the criminals tried to withdraw more than $ 40 million. ATMs are shown in red circles and attack intensity in yellow.
In the three attacks mentioned earlier, directed against two US banks and one bank in South America, Safety Net identified abnormal behavior, such as withdrawing a large amount of cash or performing transactions outside the usual geographical area for those accounts. As Ajay Bhalla, president of corporate security solutions, described the situation, MasterCard notified banks and declined operations, limiting losses to less than $ 100,000 in each individual case. The company refused to name the banks to which the attack was directed, citing a non-disclosure agreement.
Opinions about the possibilities of machine learning in fintech
Data Analysis Specialist at Crowd Expert Project for Sentifi Financial Markets:
Among other things, machine learning is used to “crowd-collect” information on the state of financial markets. The idea is that the “crowd”, represented by both experts from various fields of knowledge and amateurs, can provide valuable information due to this diversity of opinions. The purpose of this approach is to get analytics about what the general public thinks about certain companies and their actions, the stock market and other items related to finance.
Useful ideas are extracted from the “crowd consciousness” through large-scale mining of information from social networks, blogs and newspapers. Due to the natural disorder and lack of structure in the data obtained in this way, specialists need to apply machine learning, natural language processing and image recognition to benefit from them.
Most often, investors use technical and fundamental types of analysis to make decisions. The technology of “crowd-collecting” information allows you to add a third component - a social analysis of financial markets - and use the entire possible set of data to improve the decision-making process.
Moreover, this method allows you to democratize the information flow, since not only a group of individual experts (who often turn out to be not experts at all) often has a good understanding of the situation.
Fintech entrepreneur specializing in robot consulting and artificial intelligence:
Today, in the industry of robotic financial advisors, risk assessment and a personal profile of a client are based on a 10-item questionnaire with several possible answers. Now compare this approach with another, in which we use "big data" for image recognition, for example, recurrent neural networks for identifying objects and comparing them with averaged graphic images, or processing natural languages to understand how people express their personality through texts on social networks. Imagine that all this will be used to compile personal characteristics of clients, allowing you to get a holistic view of their personal financial needs and goals. This is the potential of new technologies,
Housing.com IT Specialist , Behavioral Finance:
How can a rise or fall in the market result from rumors? Why is there a “ January effect ” on the stock market ? How can natural disasters and news affect stock price movements? For answers to these questions, researchers turn to a scientific discipline called behavioral finance. It assumes that all individuals act irrationally, and anomalies are nothing but the result of the accumulation and addition of behavioral patterns of rational and irrational agents. Therefore, specialists use Bayesian networks and other techniques to model decision-making processes and identify systematic errors.
Professor of Business School at Baptist University of Hong Kong Kin Lam, along with a group of scientists introducedquantitative pseudo-Bayesian approach to modeling investor behavior. Scientists have created a model that takes into account the mood of investors and compares their practical decisions with the shock of changes in the stock market.
Amazon.com Programming and Machine Learning Officer :
- Determining the credit rating of a borrower who wants to arrange a loan or mortgage based on his activity on social networks, the history of financial transactions and other similar factors. Here, the most commonly used technologies of guided learning, such as neural networks.
- Portfolio Optimization. Here linear and non-linear programming, stochastic gradient descent and genetic algorithms are used.
- Providing the most relevant answers to questions related to financial planning. It uses natural language processing and knowledge graphs.
- Automation of the repurchase and placement of securities (the so-called underwriting)
- Systems for automating the classification and distribution of financial documents.
How algorithms will help companies win the competition:
Today, investors and borrowers from all regions of the world are looking for technologies that can make decisions in a split second, since accelerating this process ensures victory in the race for market leadership. The use of artificial intelligence, machine learning and other similar technologies will allow companies to interpret huge data arrays accumulated over several years in a matter of seconds. Regardless of whether it involves stock trading, lending, or fraud detection, recognizing complex patterns is one of the keys to success for any fintech company. To reduce risks, algorithms can be very useful for pre-experimenting and evaluating scenarios.
New algorithmic technologies are already here, and thanks to them, companies were able to radically change their value propositions for customers. Fintech companies that have used algorithms in the near future have every chance to head ahead of their competitors.
Keep up with PayOnline processing company blog updates and stay updated on technologies changing financial markets.