How to analyze paying users. Part 1 - RFM Analysis
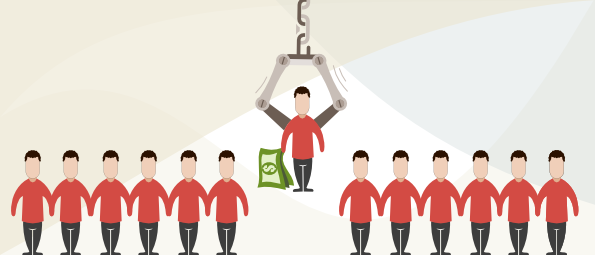
With this article, we open a whole series of articles on the analysis of paying users.
So, paying users, those guys who bring money to your product. It is very important to know all the nuances of their behavior: what they pay for, how quickly, in what volume. It’s important to know how they feel about whether they are satisfied with the investment you make in your product. In fact, even in the case of f2p online game, each player’s payment is his investment: at the entrance he pays money, at the output he receives some ROI expressed in virtual or real (game with currency withdrawal, or just black market) currency and in the emotions that he experiences. Therefore, consider paying users as investors, albeit minority ones.
In order to better understand the behavior, distribution and needs of paying users, there are analytical methods and reports. And today we’ll talk about RFM analysis as one of the main methods for understanding the structure of your paying audience.
RFM is deciphered as follows:
R - Recency - prescription of the last purchase.
F - Frequency - frequency of purchases;
M - Monetary - the volume of purchases for all time.
For each paying user, you give three ratings that correspond to these parameters. Typically, in theoretical materials, the user is evaluated using a three-point system (relatively speaking, good, normal or bad), but in practice we have come up with five- and even ten-point ratings in RFM analysis. Consider an example of a three-point system to simplify the perception:
R = 1 - the user last paid a long time ago;
R = 2 - the last user payment occurred relatively recently;
R = 3 - the user paid recently;
F = 1 - the user pays very rarely (or even made only one payment);
F = 2 - the user pays with average regularity;
F = 3- the user pays often;
M = 1 - the sum of all user payments is small;
M = 2 - the user in the amount paid the average amount of money for the project;
M = 3 - the user paid a lot of money.
Of course, the question arises of what in this case is long / recent, often / infrequently and a lot / a little. There are two ways to answer this question:
1) Expert assessment. No one knows your project better than yourself. Therefore, establish for yourself what is long and recent, what is a lot and a little. Say, for a long time - this is a month or more ago, rarely - it is once a month or less, and a little - it is no more than 100 rubles for the entire history of payments.
2) Quantiles and quartiles.We recall the mathematical statistics. Order your users according to one of the parameters (for example, by the amount of payments for the whole time), take, for example, the top 5% of all users and say that these users paid a lot. Congratulations, you just got a five percent sample quantile from your users. Or you can take quartiles (quartile = quantile of 25%), and evaluate the first quartile as a lot, the last quartile as small, and what is between them as the average payment. Be that as it may, even when using quantiles and quartiles you can’t do without a subjective assessment, and therefore, see paragraph 1 again.
In any case, having worked a little in Excel (or elsewhere), you will assign to each paying user three estimates: for prescription, for the frequency and volume of payments.
And now the fun begins.
You can see how these ratings are distributed by your paying users, which users are the most. This will allow you to segment your paying audience and plan marketing actions to increase revenue.
A simple example:
- Recently bought, but rarely (or one payment) - new paying users. What to do with them? Thank! Your task is to encourage them to repeat purchases. Studies show that it is repeat purchases, their regularity and size, that increase the chances of the application to earn a million dollars.
- Bought recently, often bought - loyal users. They don’t need additional stimulation, but it makes sense to find a way and thank them for their loyalty (unexpected bonus, surprise, just “Thank you”, it all works).
- Bought often, but for a long time - loyal users on the verge of leaving. That is, money that is flowing right through your fingers right now. Your task is to remind them of yourself. Perhaps a simple push notification is enough. Perhaps you should go to them with a survey - what has changed, why are they leaving?
- Bought rarely and for a long time - an outflow of users. They never became loyal, something prevented them then, in the past. You can offer them (and only them) a promotion, even if it is not very profitable for you, which would stimulate them to re-purchase and return to the product. Otherwise, you can at least try to find out what they did not like and adjust the product based on their feedback.
Suppose there is the following case.
1) The X project wants to increase its income.
2) An RFM analysis was carried out, which showed that:
3) the outflow of loyal paying users is very high;
4) Many users make just one purchase.
5) Triggers are introduced into the product, which allow you to recognize the moment when the user's stay in the status of 'one purchase' dragged on, or when a user who was previously loyal, stops paying. At these moments, the users are given an “offer that cannot be refused” (special offer, big discount, information is given by a push notification or a pop-up window at the entrance).
6) The percentage of repeat purchases increases, the retention of loyal users increases.
7) Profit.
Both of the above examples operate with only two dimensions: Recency, Frequency.
Adding the Monetary dimension to this report will also be guided by the volume of payments from each client.

Moreover, the analysis can be performed both by the number of users and by the amount of money received from them.

In addition, you can separately consider combinations of Monetary-Recency (how much users pay and how long they paid), Monetary-Frequency (how much and how often users pay).
The easiest way is to analyze paying users in one dimension - to get the distribution of users and their payments by time (just - recently - long ago), by frequency (often - sometimes - rarely), by size (many - medium - small).
In particular, in f2p games, it is customary to describe the analysis of paying users by the size of their payments with the help of the inhabitants of the deep sea:
- Whales - users who bring large amounts of money;
- Dolphins - users who bring average amounts;
- Minnows - users who bring small amounts.
This is not about the amounts from one payment, but about the total amounts accumulated over the entire history of the user's payments. And the distribution of large, medium and small amounts is again made expertly.
By analyzing the number of users in each segment and the amount of money received from each segment, you can understand what actions are better to take to increase revenue: lower prices? raise prices? focus on whale retention?

In my service devtodevWe divided paying users by payment volumes into five segments, separately highlighting large whales (grand whales) and large dolphins (grand doplhins). In particular, the example examined shows that the bulk of the income comes from whales and big dolphins, and therefore it is on them that it is worth focusing the bulk of the marketing efforts.
This is only part of the methods by which paying users can be analyzed. There are many more questions, the answers to which will help you better customize the monetization of the project. Here are just a few of them:
- How quickly do your users convert to paying? First, second, tenth purchase?
- What do users pay for? Why are they generally converted to paying?
- How much money do you earn from the first payments of players, and how much - from repeated payments?
- How much money do newcomers bring to you, and how much do old people bring?
We will certainly tell you in detail about each of the analysis methods in future articles.
And if you don’t want to wait, we invite you to a free webinar that will take place on October 26, 2015 at 18:00 Moscow time.
At the webinar we will talk about all the methods of analyzing paying users, describe cases, how analytics of paying users helps to increase revenue. Come!