Hackathon on Data Science in SIBUR: how it was
Hello!
Since the beginning of the year, we have conducted about 10 hackathons and workshops throughout the country. In May, together with the AI-community, we organized a hackathon in the direction of “Digitalization of Production”. Before us, the hackathon about data science in production has not yet been done, and today we decided to tell in detail about how it was. The goal was simple. It was necessary to digitize our business at all its stages (from the supply of raw materials to production and direct sales). Of course, applied tasks were also to be solved, for example:
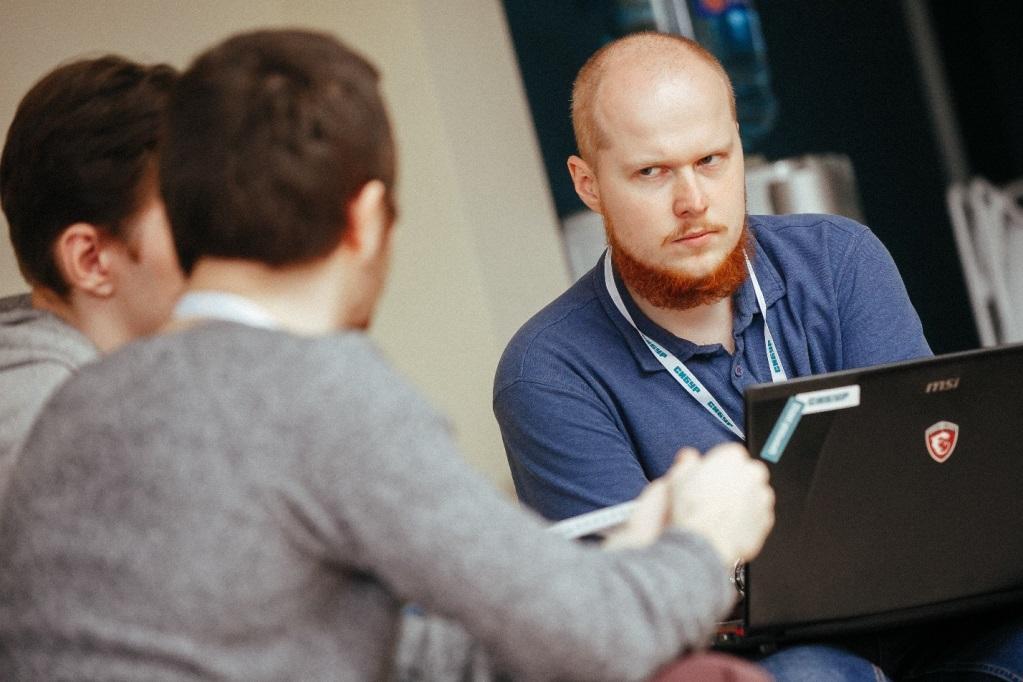
What is the main value of such tasks? That's right, as close as possible to real business cases, and not to abstract projects. The first task is already described in detail in Habré by one of the participants (thanks, cointegrated David!). And the second task imposed on the hackathon was the need to optimize the process of combining the planned repairs of the wagons of the logistics park. It was taken directly from our current backlog, adapting a little for the participants in order to make it clearer.
So, the description of the problem.
Logistics specialists have a special calendar, where information about sending cars for scheduled maintenance is entered. Since there are more than two cars (much more than two), you need a solution that will simplify the work of the employee, making his work easier, more intuitive, and also help him to quickly make certain decisions based on preliminary data analysis.
Therefore, the decision itself should include two components:
We provided participants with a dataset about sending 18,000 wagons for repair with data on all distances, timing and other (information for several years). Plus, they had the opportunity to talk live with the business manager of the process and clarify with him all the necessary details, as well as collect suggestions.
It would seem, well, made a calendar of repair of cars and everything, what else can you optimize? And most importantly - how and how to measure the effectiveness of the solution?
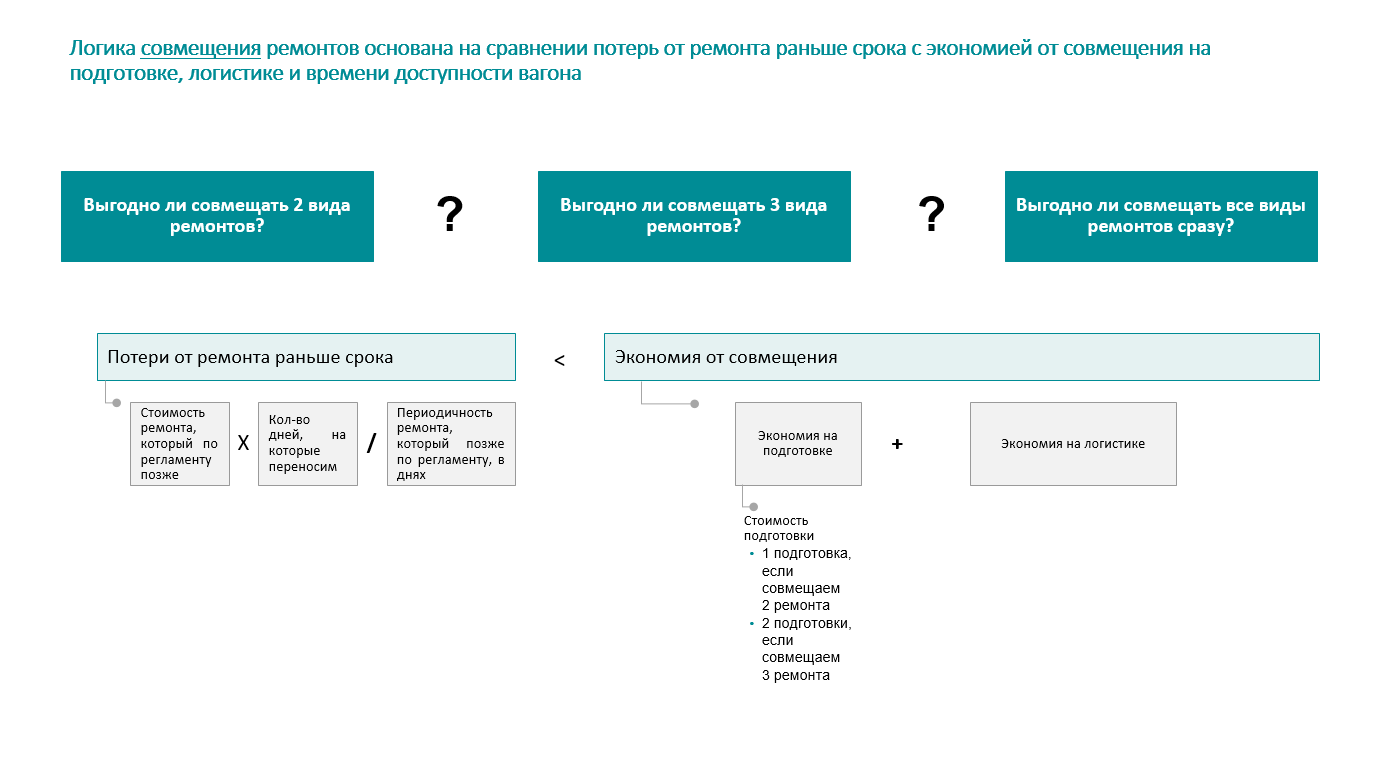
Criteria for the optimization of scheduled repairs
Here it is worth starting with the fact that the repair of a car is not just a car repair. Each of our cars can have 4 types of repairs.
Each of these four types of repair has its own cost of direct repair (repair materials + payment for repairs), as well as the cost of preparing for repair. In addition, there is also the cost of delivery of the car to the depot. And since the car goes purposefully for repairs, it goes empty, which means that we exclude the possible profit for the trip.
The guys started, of course, with hypotheses.
Hypothesis №1. If you combine several repairs in one day, you can save on preparatory work.
The hypothesis met a sentence like "Yes, then let's just do everything else with every repair, so as not to get up two times on the same day."
It sounds cool. Sometimes even logical. But not everything is so simple.
Repair (any of the four) has not only cost, but also recycling. In general, as with the machine. You passed the inspection in January, and you drag it to the next inspection as long as possible so that every ruble spent on the first inspection is spent efficiently. If you do THAT too often, without generating a resource, you lose money.
Yes, with a car the example does not quite coincide with ours, nevertheless, the situations are different, and sometimes it is worth going through THAT in advance (or even 2-3 times a year), say, before an important long trip. But in the case of a huge number of cars, such a false start of repairs can bring quite serious losses.
Hypothesis №2. Then you can simply combine these repairs so that the disposal of each of them was as complete as possible.
Already better. There are questions:
From which station is it more profitable to send the car for repairs?
We know the way from each station to the depot. And the path between the stations themselves is not. Maybe the car will be able to transport a little more cargo and go to the depot from a farther station, but having earned it on a trip?
Hypothesis №3. We take into account the distances between the stations and the profit from the delivery of products - we optimize the logistics points of dispatch for repair.
So that the hypothesis was not just an unfounded statement, it is better to express it in financial terms.
That is, here, in order to solve the problem, ideally, it is necessary to build such a model that will be able to maximally link these indicators to each other. At the same time giving the opportunity to change the input parameters (the number of cars sent for repairs, the date of repairs, staying at the stations, etc.) and show the real savings.
And again, the main thing. This is a program that people will work with. Therefore, it is necessary to make an interface for people, and not a hell of a heap of molds and filter plates. Each of the employees who will work with this interface should quickly understand what is happening at all, where the car is traveling from and what kind of carriages they have come up with.
As a starting point, we showed the participants several of our drafts. It was not a guide to action, but just an example.
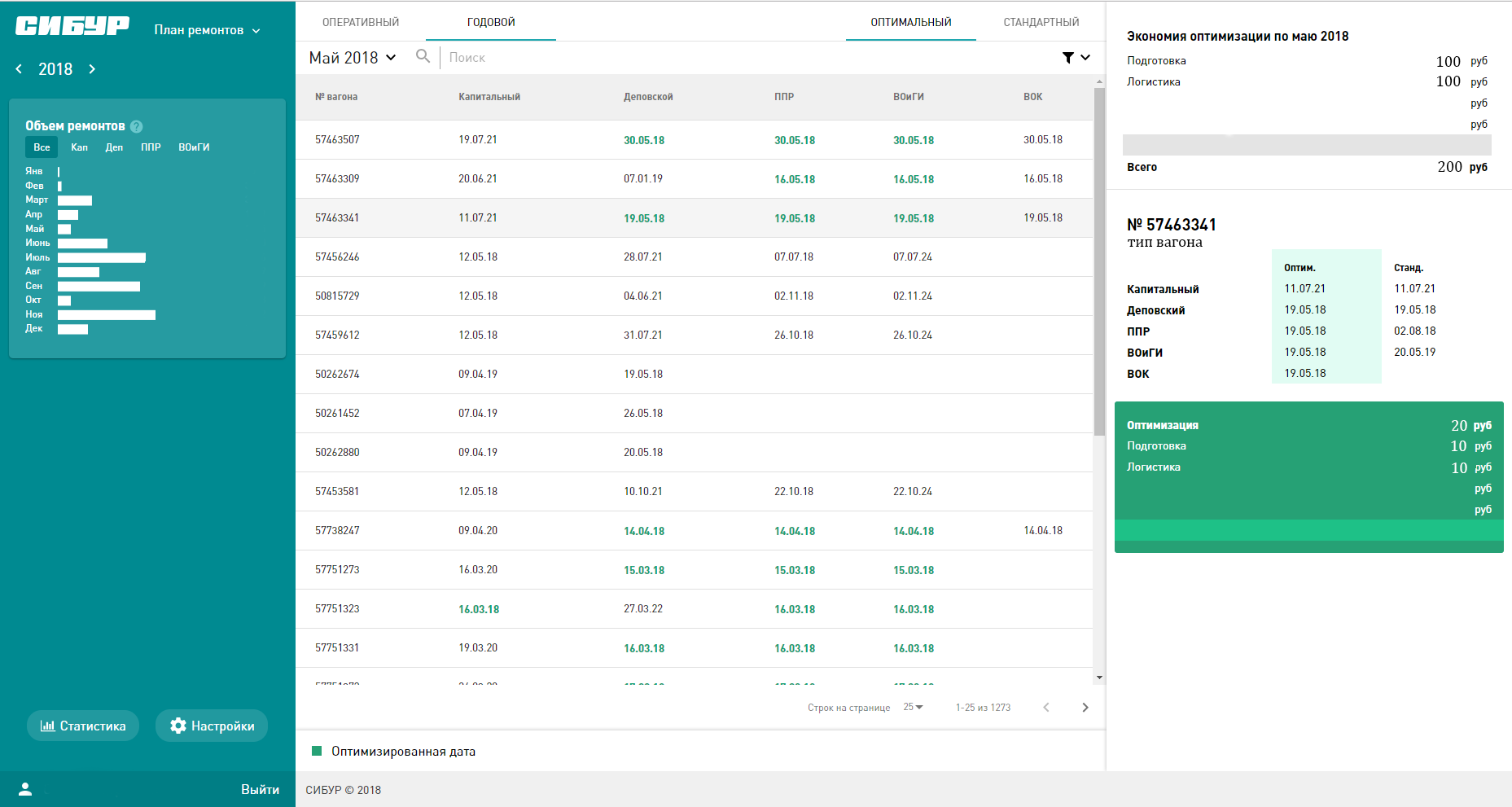
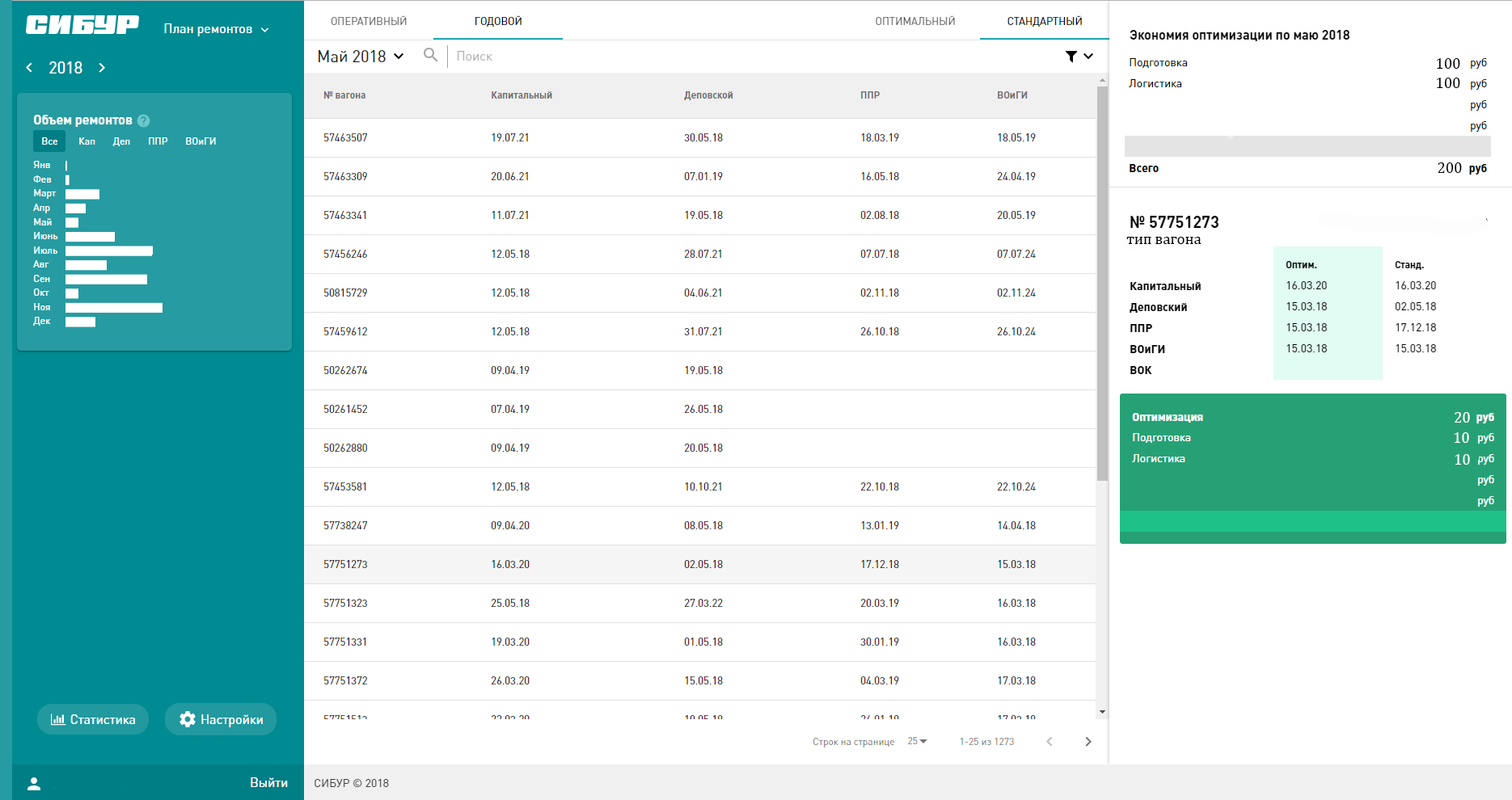
Draft designs sketches The
participants accepted all sketches and wishes and left to think.
A couple of days passed in the format of constant updates - teams came to us, showed approximate sketches, clarified something, received answers, left to finish the decision further.
In fact, from the side of the organizer, this looks very cool - people are creative on the go, adjusting to the new, refined introductory data, finding a few minuses in their own decisions and immediately eliminating them. Moreover, in the format of full-fledged teamwork - while one paints the design of all this stuff, the date-cyntists are already finishing writing the first scripts.
We are now trying to make our digital division work on the daily tasks of the company in the same atmosphere, because it is very exciting.
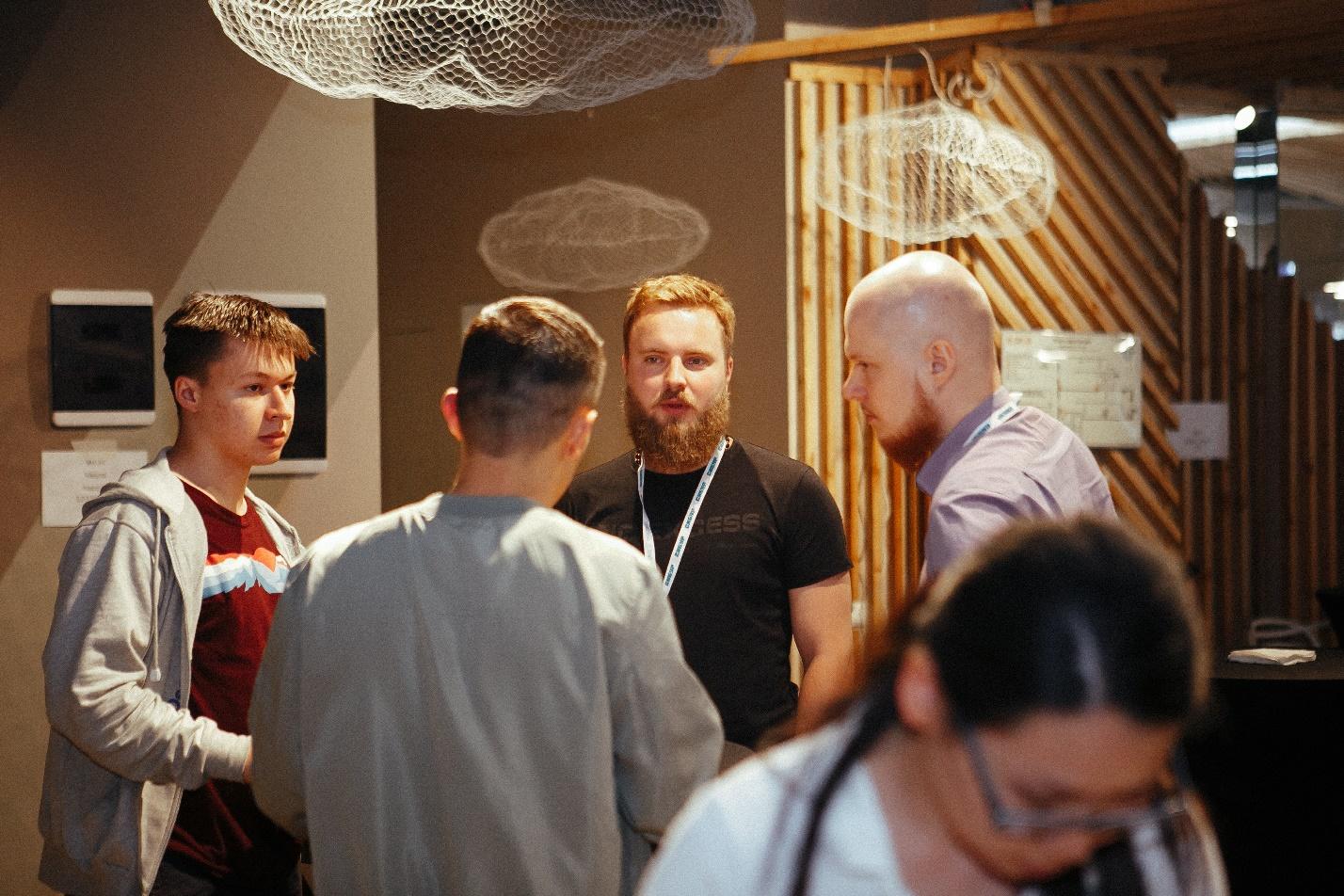
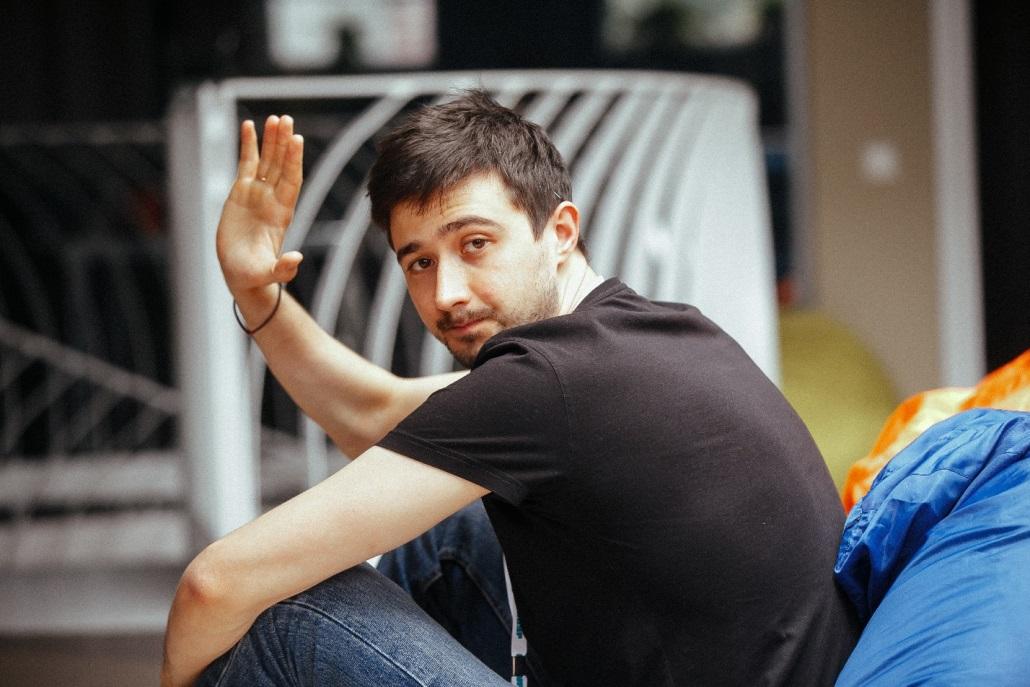
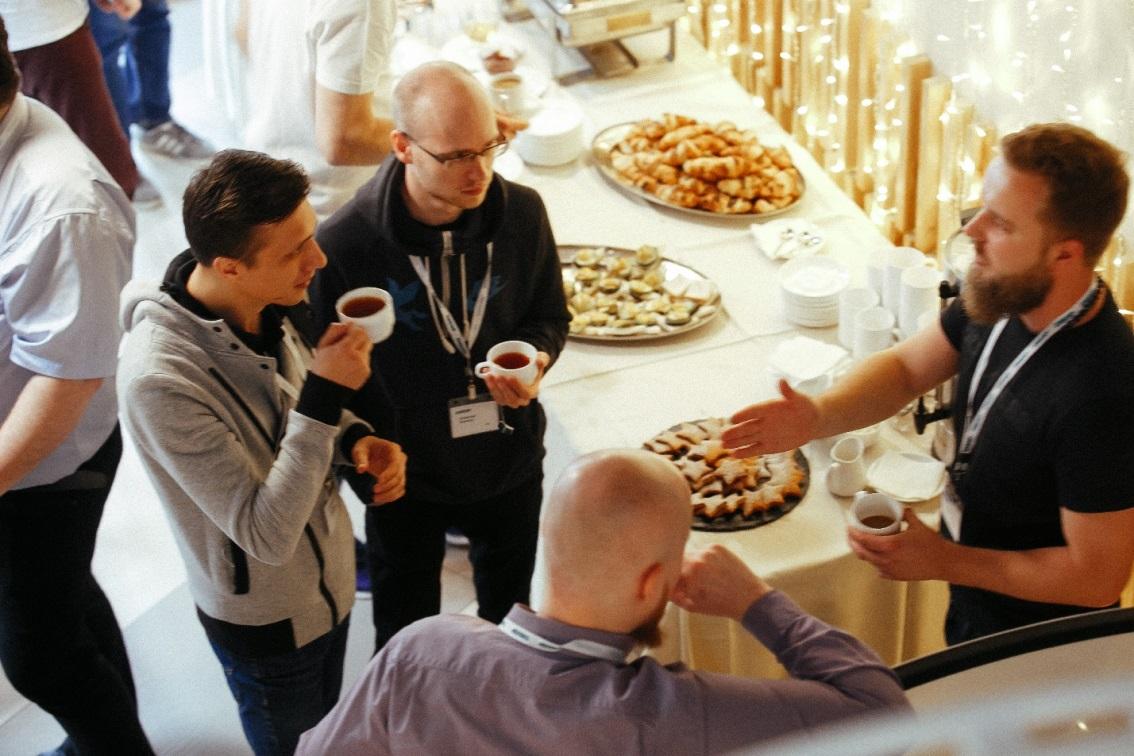
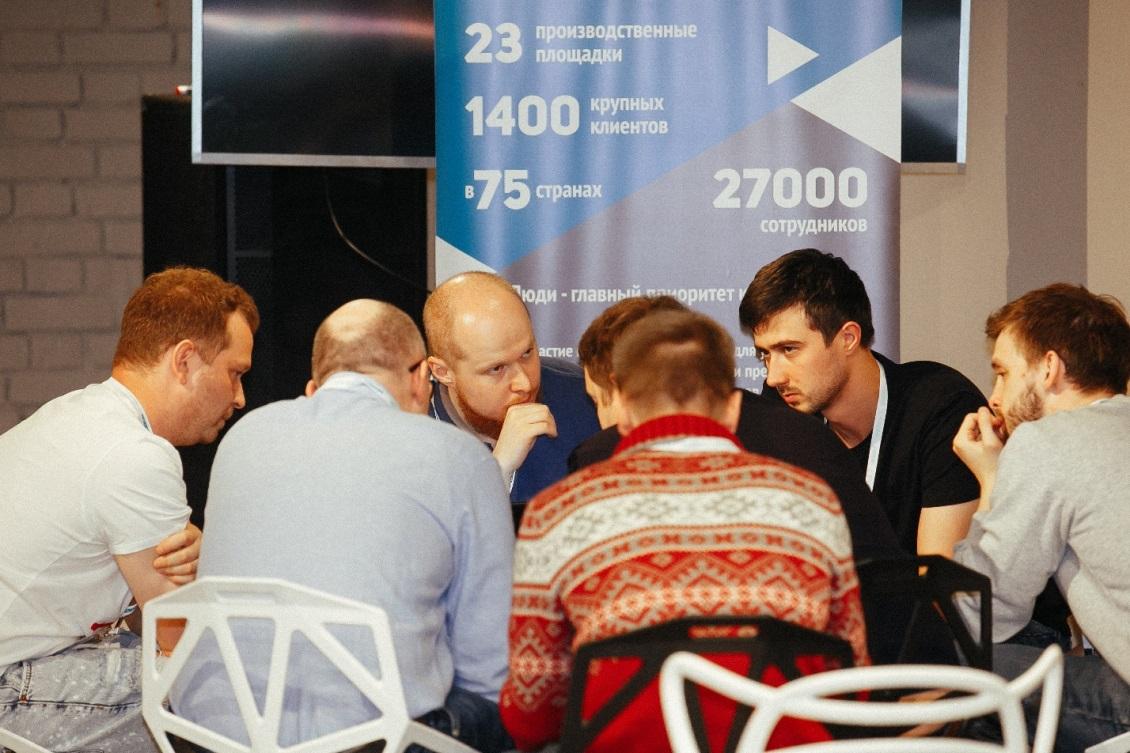
Everything was simple and familiar. Each team has 5 minutes to speak, and the organizers have 5 minutes to answer questions. Of course, the framework was not very hard, and sometimes we went out during this time.
We spent about 3 hours on everything about everything in such a rhythm. We
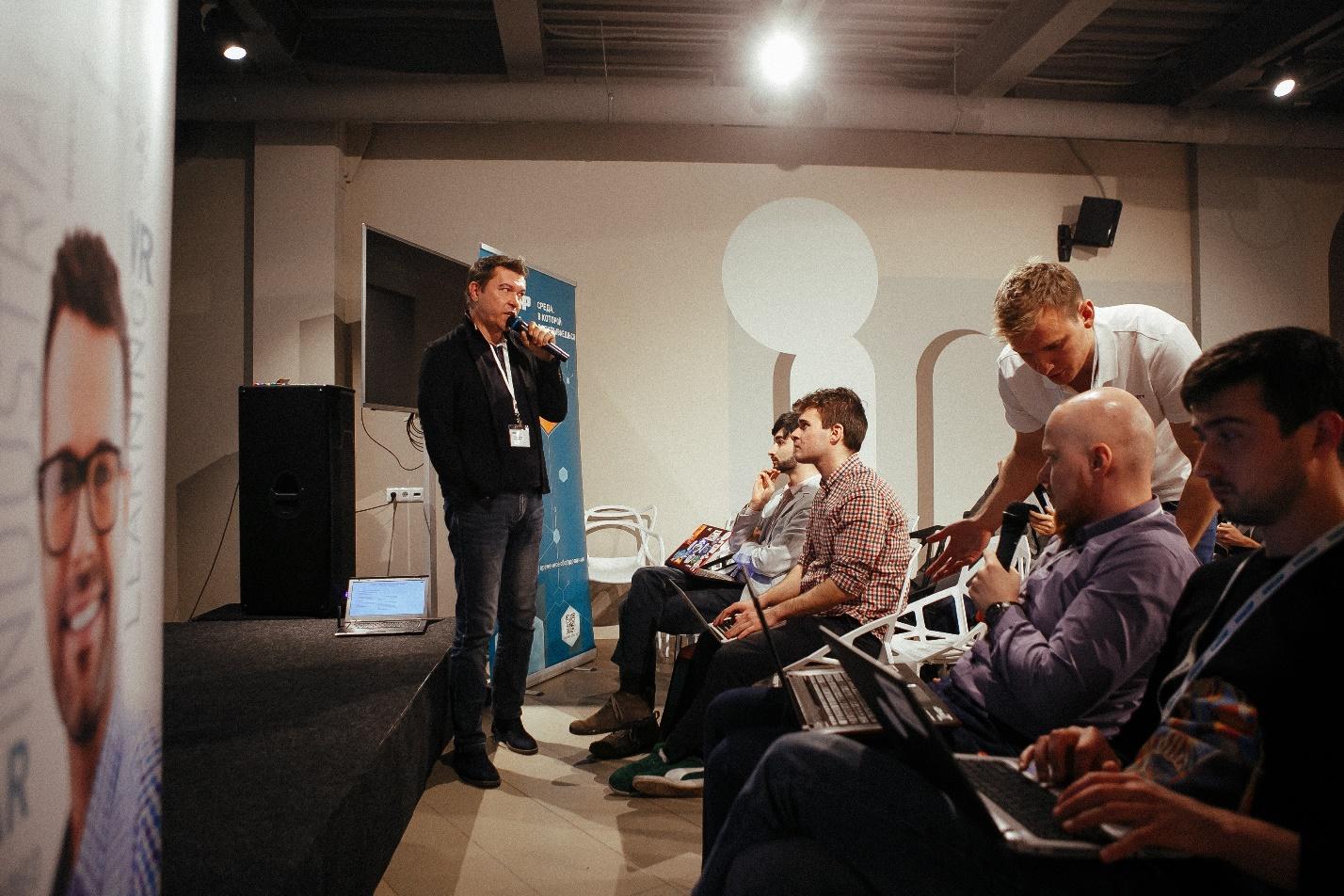
evaluated the solutions obtained comprehensively - approaches to solving the problem in general, visualization, the applicability of the proposals in reality. Here the approach of the AI-community helped, by which the intermediate results of the process were also recorded.
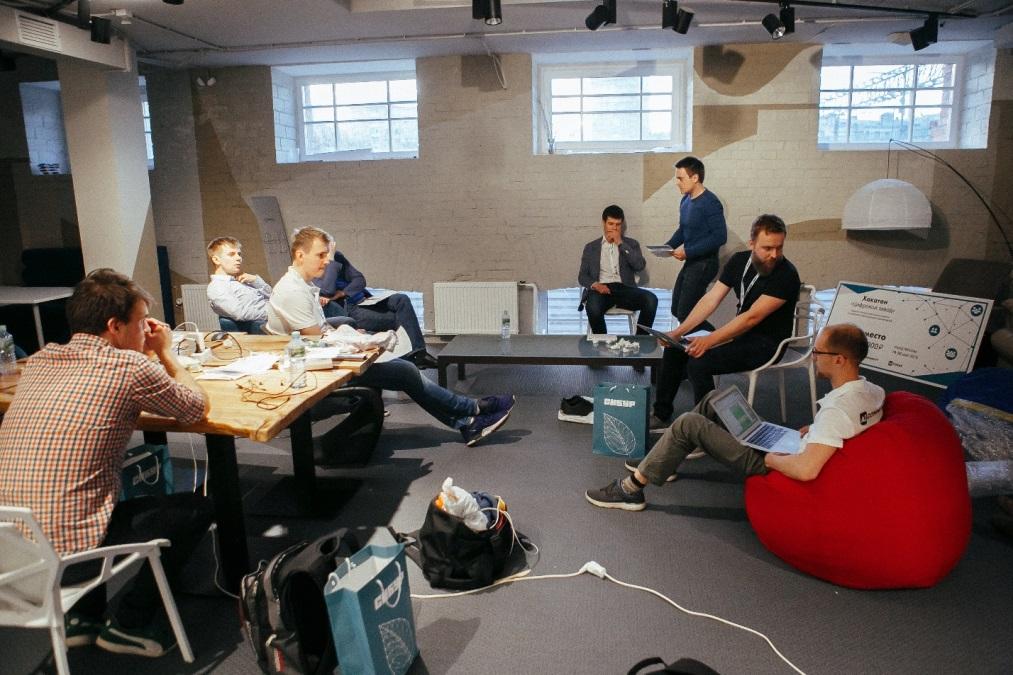
The main prize (300,000 rubles) went to the Hack.zamAI team.
The guys created a comprehensive solution, not only optimizing financial performance, but also adding a bunch of additional buns there, displaying the finished business process in the product.
At the same time, it still looks decent and friendly.
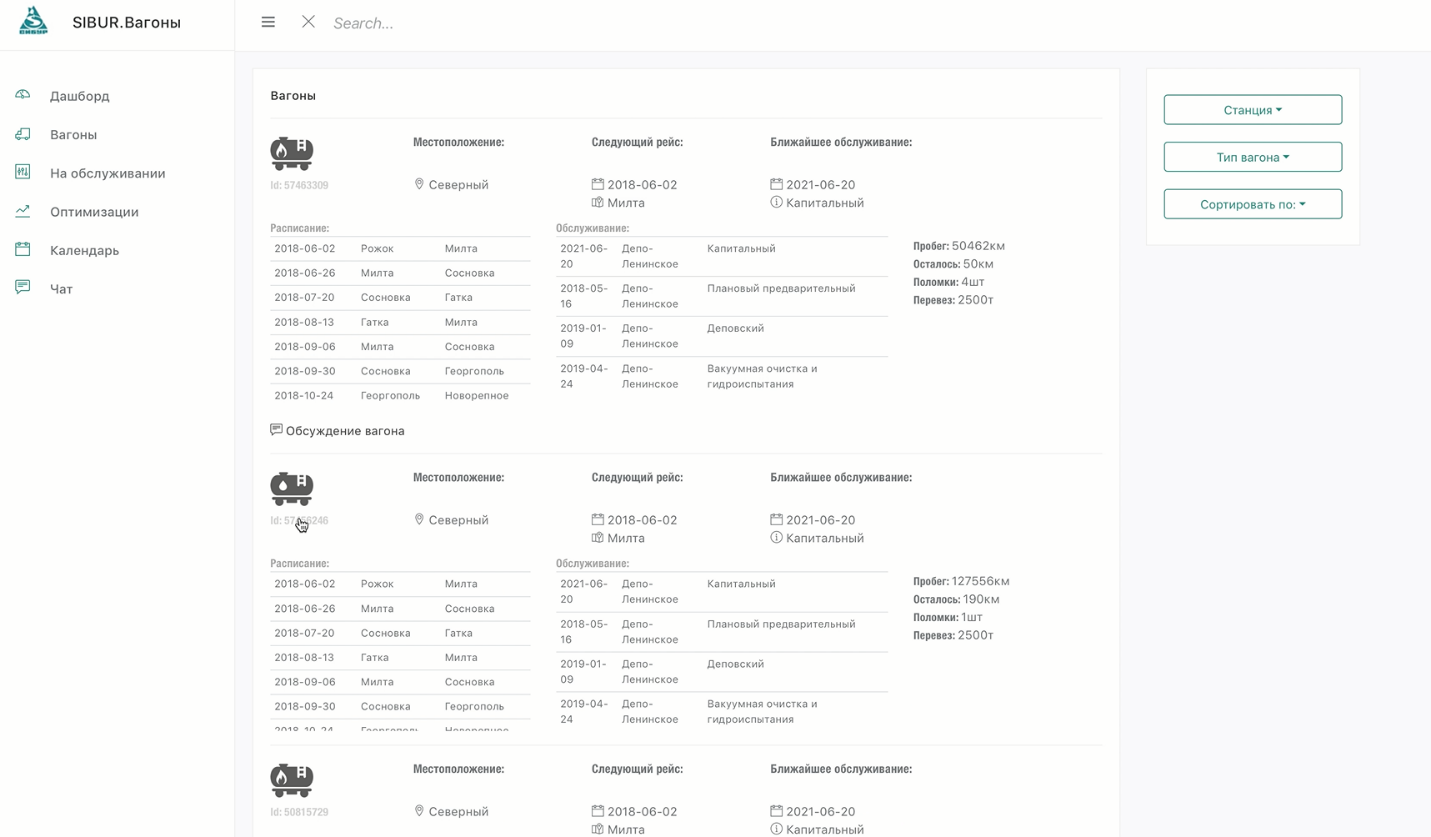
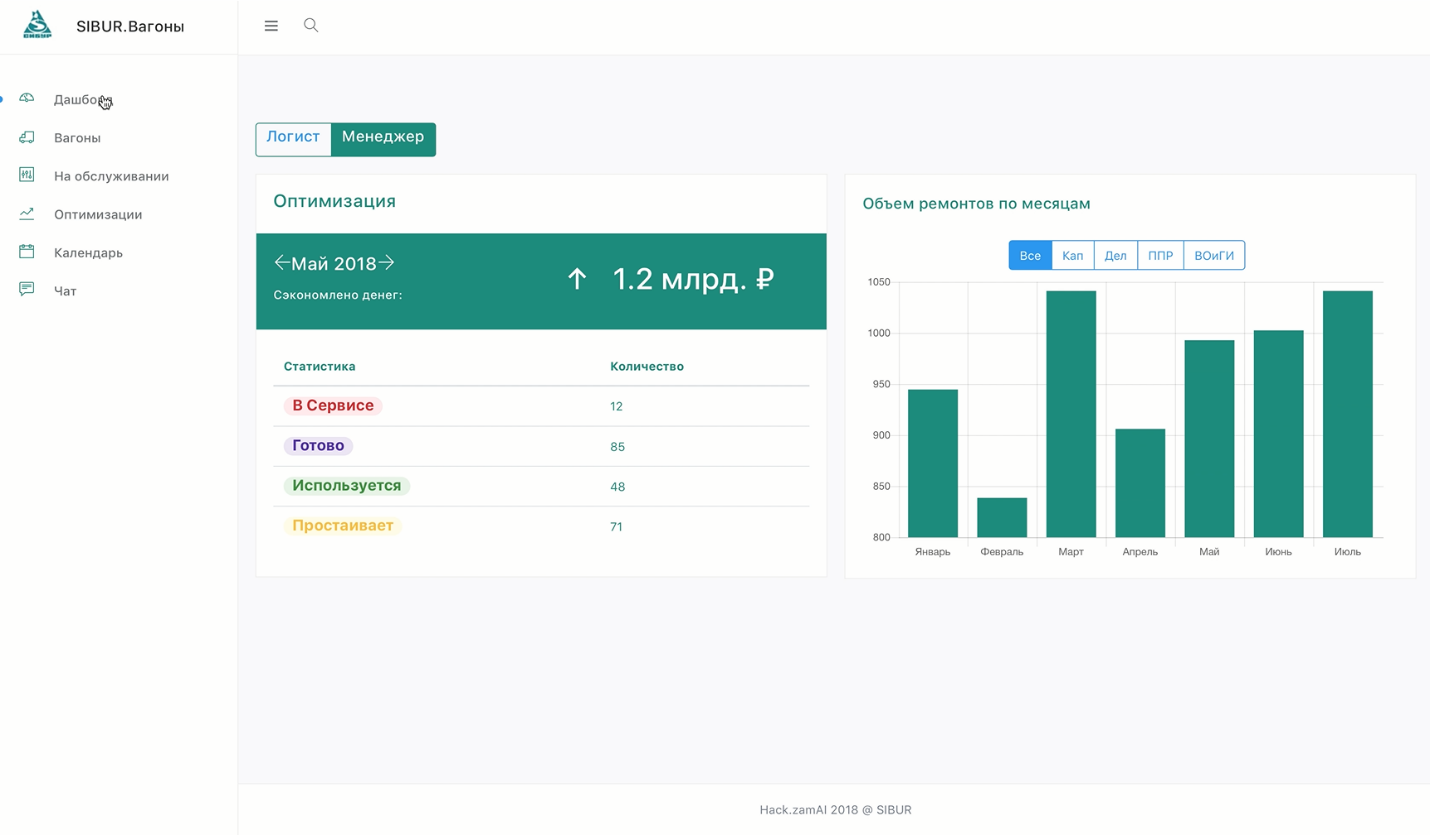
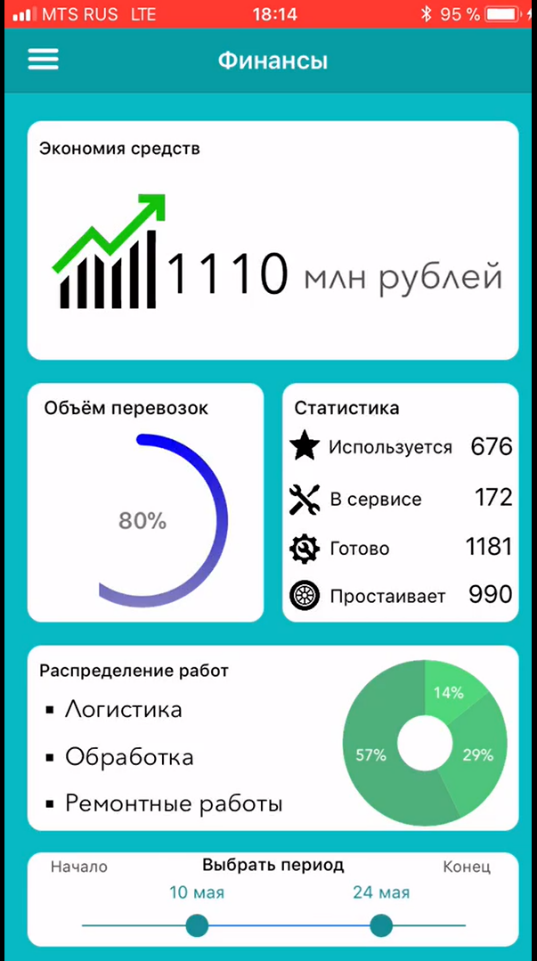
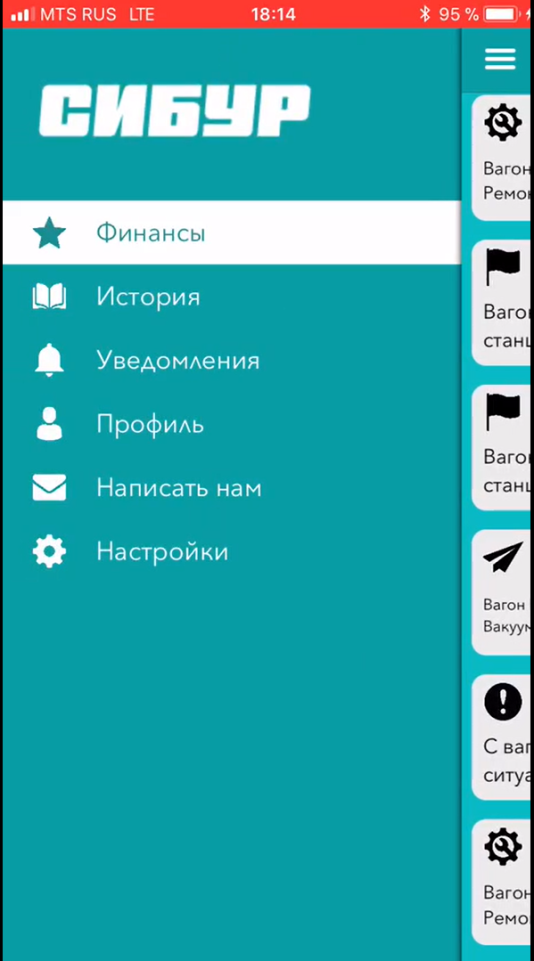
Here you can see a demonstration of their decision.
(video on GoogleDrive)
Of course, this is not our last hackathon.
We want to say thank you to everyone who participated in this. And be sure to post the announcement of the following.
Dmitry Arkhipov, Architect, Digitization of Processes, SIBUR
Since the beginning of the year, we have conducted about 10 hackathons and workshops throughout the country. In May, together with the AI-community, we organized a hackathon in the direction of “Digitalization of Production”. Before us, the hackathon about data science in production has not yet been done, and today we decided to tell in detail about how it was. The goal was simple. It was necessary to digitize our business at all its stages (from the supply of raw materials to production and direct sales). Of course, applied tasks were also to be solved, for example:
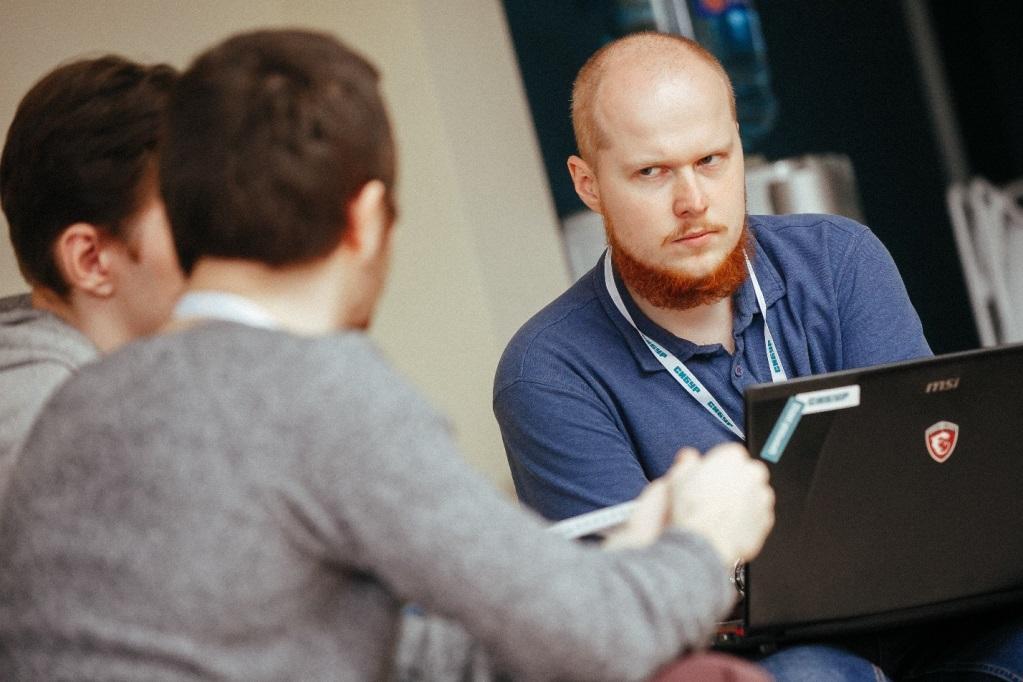
- elimination of equipment downtime, technological violations and failures;
- increased productivity and with it - product quality;
- lower logistics and procurement costs;
- accelerating the launch and launch of new products on the market.
What is the main value of such tasks? That's right, as close as possible to real business cases, and not to abstract projects. The first task is already described in detail in Habré by one of the participants (thanks, cointegrated David!). And the second task imposed on the hackathon was the need to optimize the process of combining the planned repairs of the wagons of the logistics park. It was taken directly from our current backlog, adapting a little for the participants in order to make it clearer.
So, the description of the problem.
What should have been done
Logistics specialists have a special calendar, where information about sending cars for scheduled maintenance is entered. Since there are more than two cars (much more than two), you need a solution that will simplify the work of the employee, making his work easier, more intuitive, and also help him to quickly make certain decisions based on preliminary data analysis.
Therefore, the decision itself should include two components:
- Special algorithm based on data analysis.
- Convenient interface that allows you to visualize the data obtained and the results of the algorithm in detail and clearly. What exactly to implement (web, mobile application, or even using a bot) - at the discretion of the participants.
Input data
We provided participants with a dataset about sending 18,000 wagons for repair with data on all distances, timing and other (information for several years). Plus, they had the opportunity to talk live with the business manager of the process and clarify with him all the necessary details, as well as collect suggestions.
It would seem, well, made a calendar of repair of cars and everything, what else can you optimize? And most importantly - how and how to measure the effectiveness of the solution?
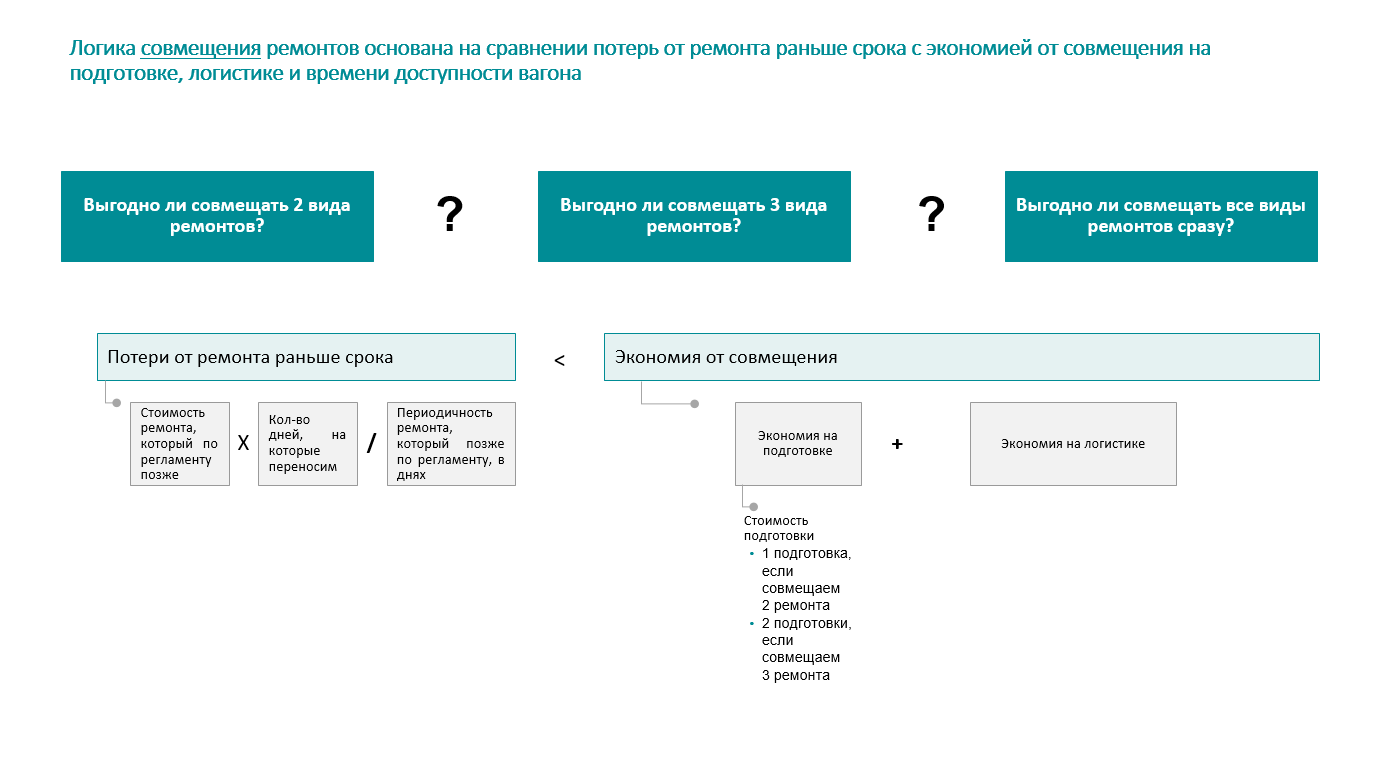
Criteria for the optimization of scheduled repairs
Here it is worth starting with the fact that the repair of a car is not just a car repair. Each of our cars can have 4 types of repairs.
- Capital.
- Depot.
- Planned warning.
- Vacuum cleaning and hydrotesting.
Each of these four types of repair has its own cost of direct repair (repair materials + payment for repairs), as well as the cost of preparing for repair. In addition, there is also the cost of delivery of the car to the depot. And since the car goes purposefully for repairs, it goes empty, which means that we exclude the possible profit for the trip.
The guys started, of course, with hypotheses.
Hypotheses
Hypothesis №1. If you combine several repairs in one day, you can save on preparatory work.
The hypothesis met a sentence like "Yes, then let's just do everything else with every repair, so as not to get up two times on the same day."
It sounds cool. Sometimes even logical. But not everything is so simple.
Repair (any of the four) has not only cost, but also recycling. In general, as with the machine. You passed the inspection in January, and you drag it to the next inspection as long as possible so that every ruble spent on the first inspection is spent efficiently. If you do THAT too often, without generating a resource, you lose money.
Yes, with a car the example does not quite coincide with ours, nevertheless, the situations are different, and sometimes it is worth going through THAT in advance (or even 2-3 times a year), say, before an important long trip. But in the case of a huge number of cars, such a false start of repairs can bring quite serious losses.
Hypothesis №2. Then you can simply combine these repairs so that the disposal of each of them was as complete as possible.
Already better. There are questions:
From which station is it more profitable to send the car for repairs?
We know the way from each station to the depot. And the path between the stations themselves is not. Maybe the car will be able to transport a little more cargo and go to the depot from a farther station, but having earned it on a trip?
Hypothesis №3. We take into account the distances between the stations and the profit from the delivery of products - we optimize the logistics points of dispatch for repair.
So that the hypothesis was not just an unfounded statement, it is better to express it in financial terms.
That is, here, in order to solve the problem, ideally, it is necessary to build such a model that will be able to maximally link these indicators to each other. At the same time giving the opportunity to change the input parameters (the number of cars sent for repairs, the date of repairs, staying at the stations, etc.) and show the real savings.
And again, the main thing. This is a program that people will work with. Therefore, it is necessary to make an interface for people, and not a hell of a heap of molds and filter plates. Each of the employees who will work with this interface should quickly understand what is happening at all, where the car is traveling from and what kind of carriages they have come up with.
As a starting point, we showed the participants several of our drafts. It was not a guide to action, but just an example.
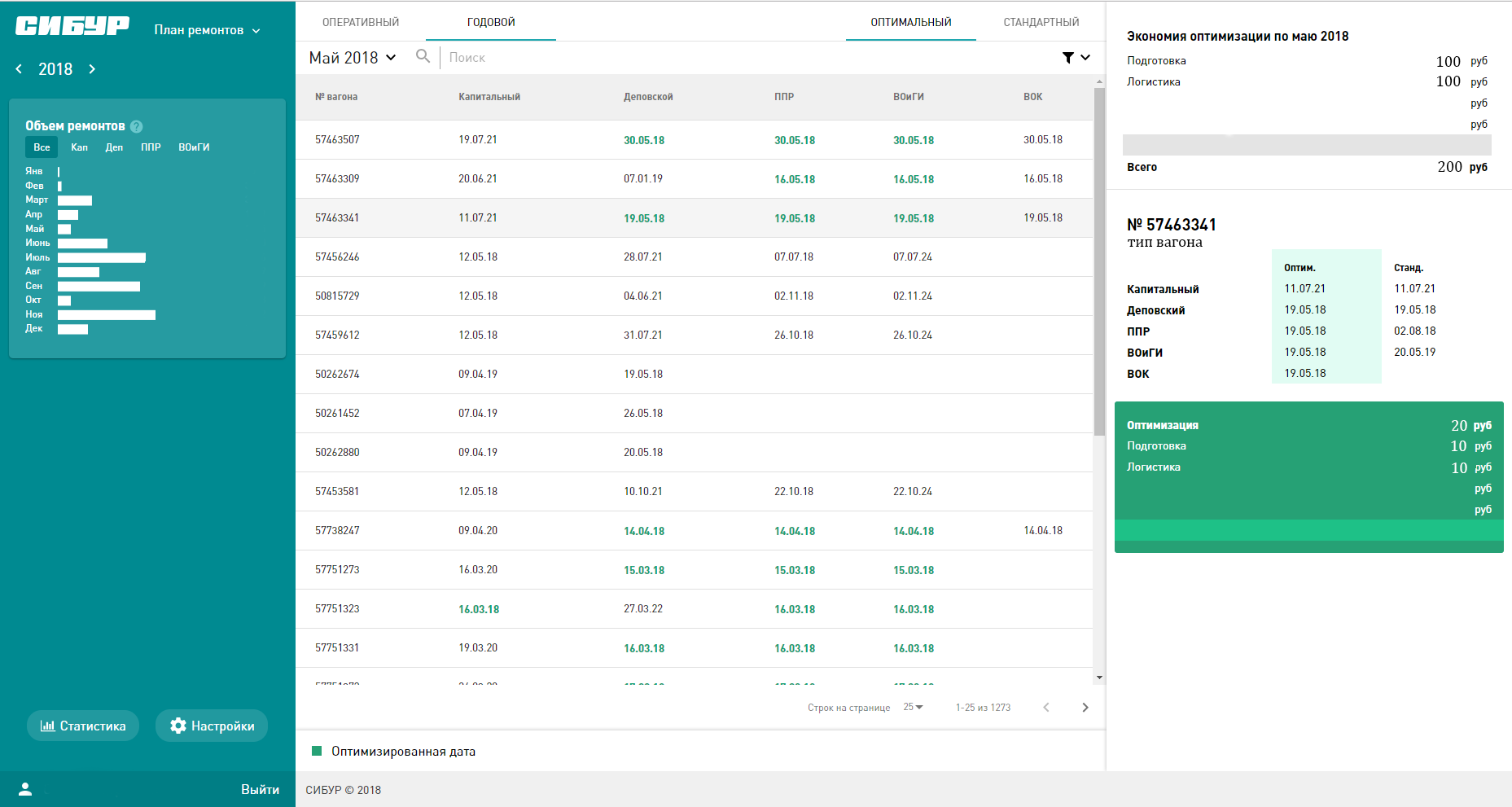
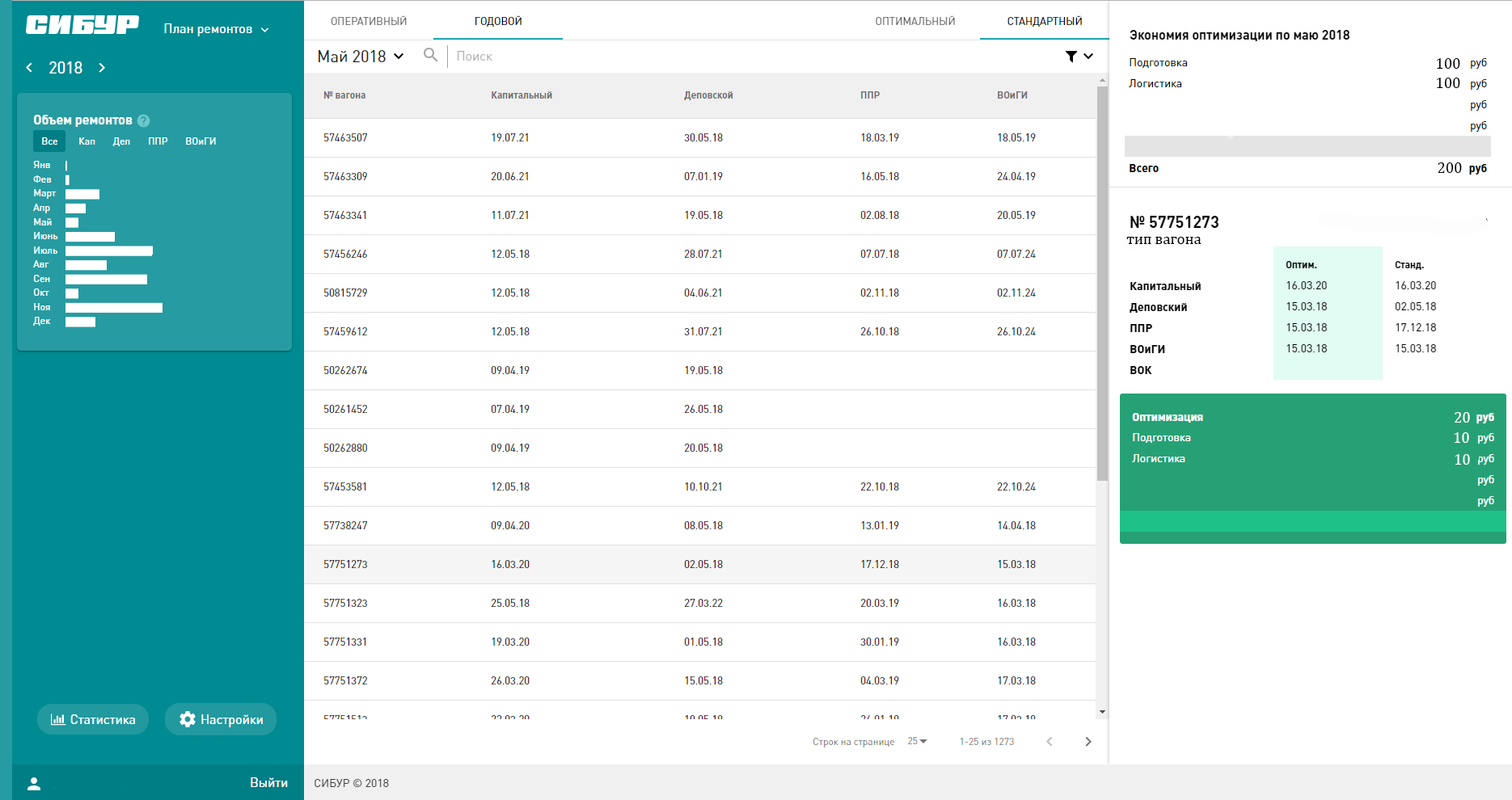
Draft designs sketches The
participants accepted all sketches and wishes and left to think.
A couple of days passed in the format of constant updates - teams came to us, showed approximate sketches, clarified something, received answers, left to finish the decision further.
In fact, from the side of the organizer, this looks very cool - people are creative on the go, adjusting to the new, refined introductory data, finding a few minuses in their own decisions and immediately eliminating them. Moreover, in the format of full-fledged teamwork - while one paints the design of all this stuff, the date-cyntists are already finishing writing the first scripts.
We are now trying to make our digital division work on the daily tasks of the company in the same atmosphere, because it is very exciting.
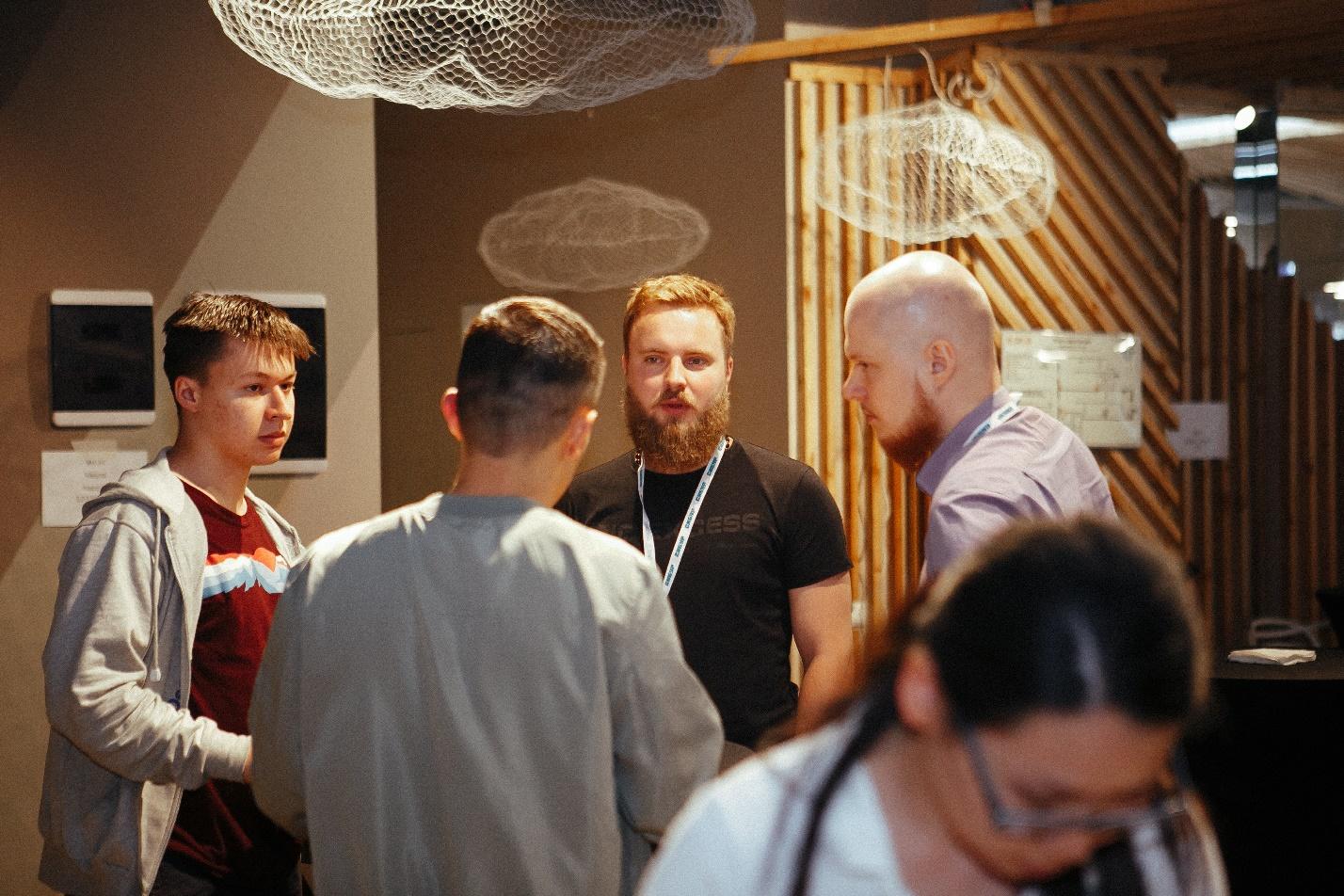
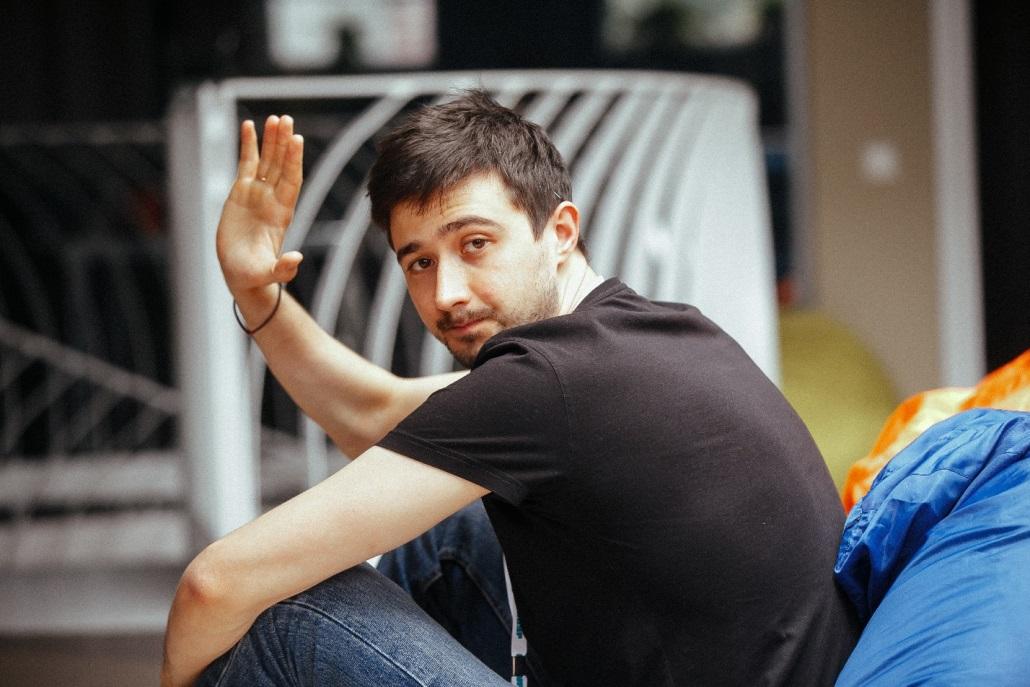
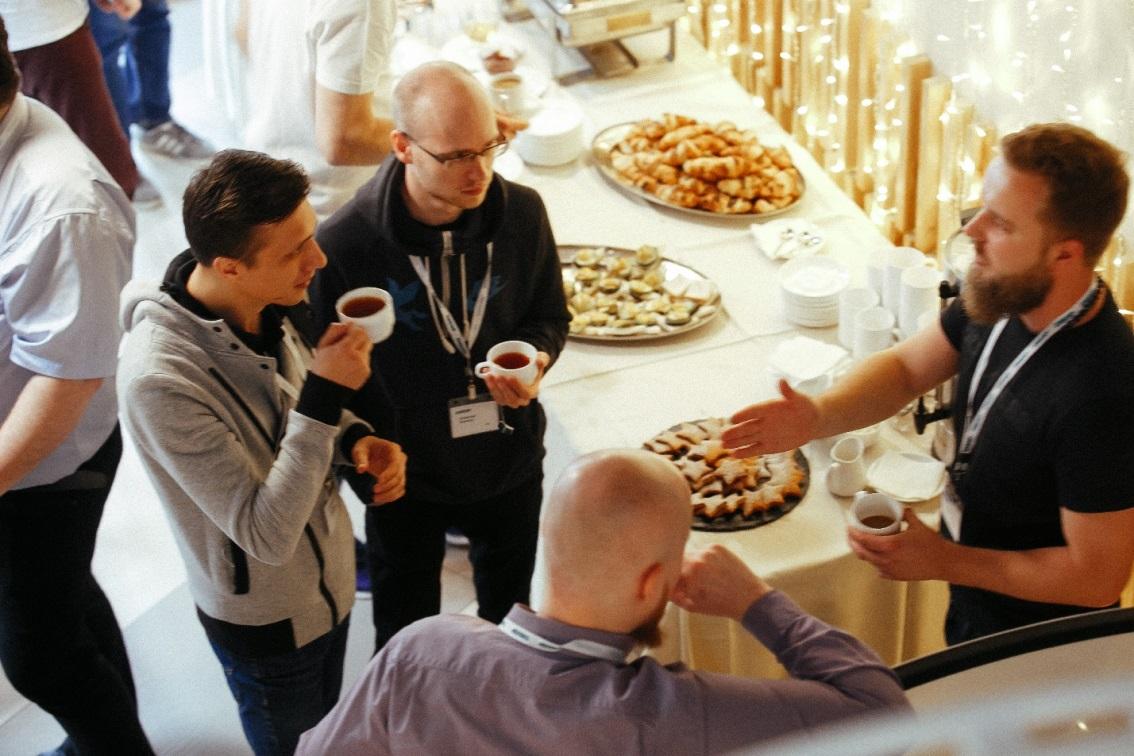
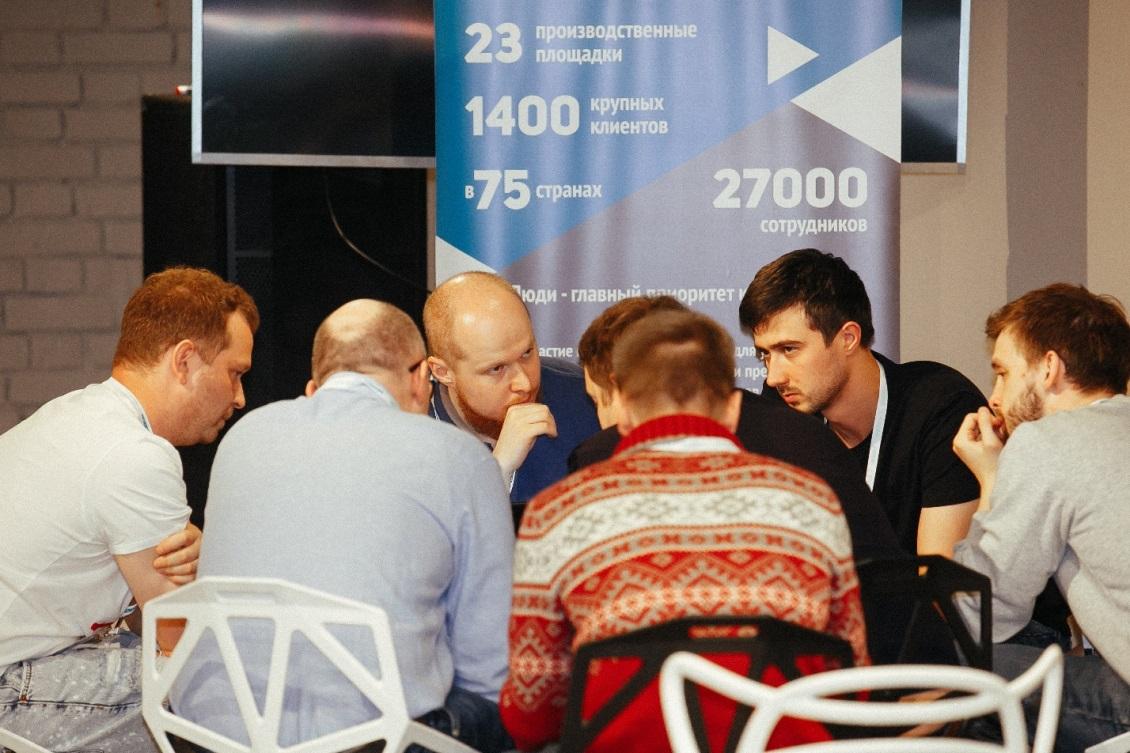
Watch demo and final
Everything was simple and familiar. Each team has 5 minutes to speak, and the organizers have 5 minutes to answer questions. Of course, the framework was not very hard, and sometimes we went out during this time.
We spent about 3 hours on everything about everything in such a rhythm. We
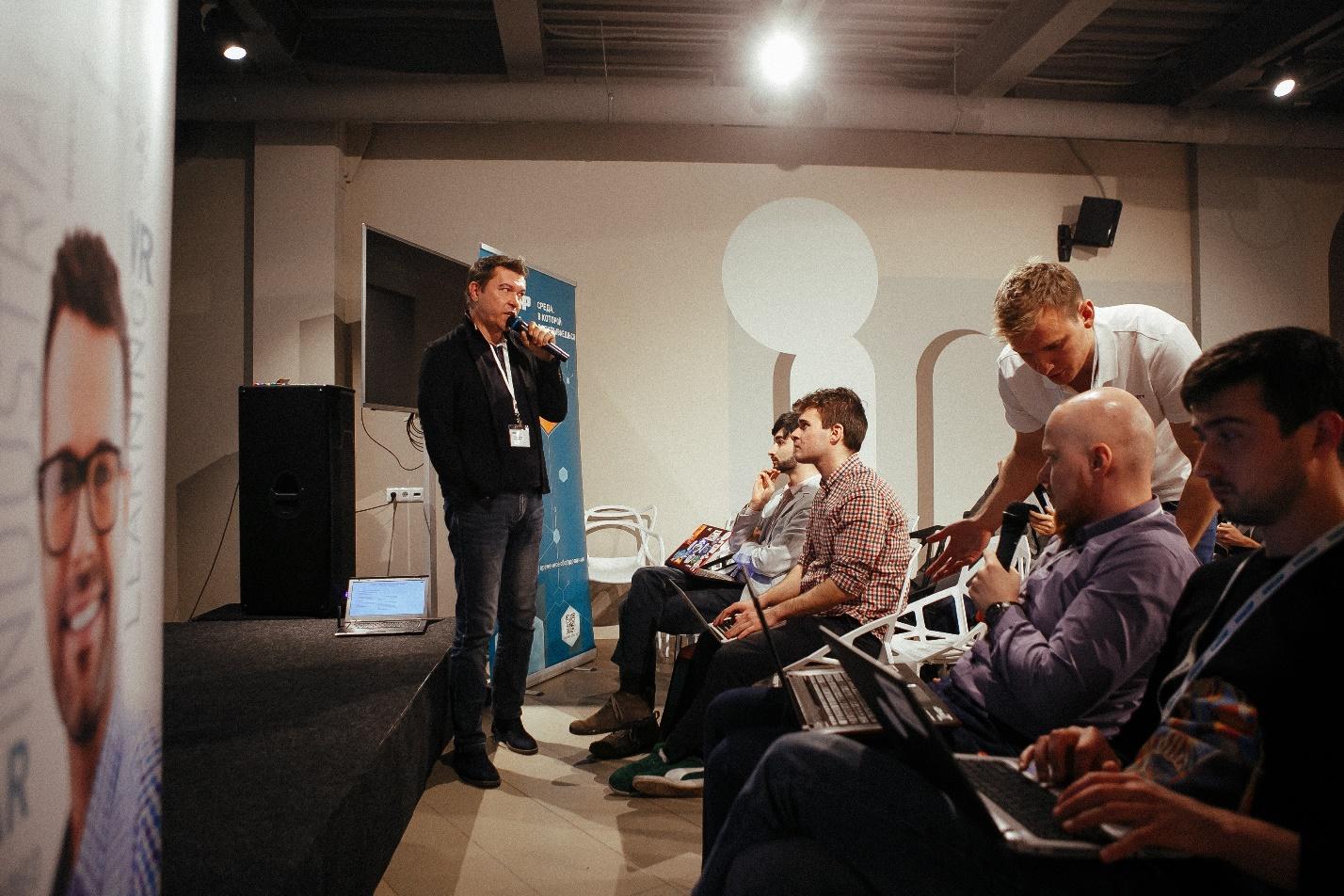
evaluated the solutions obtained comprehensively - approaches to solving the problem in general, visualization, the applicability of the proposals in reality. Here the approach of the AI-community helped, by which the intermediate results of the process were also recorded.
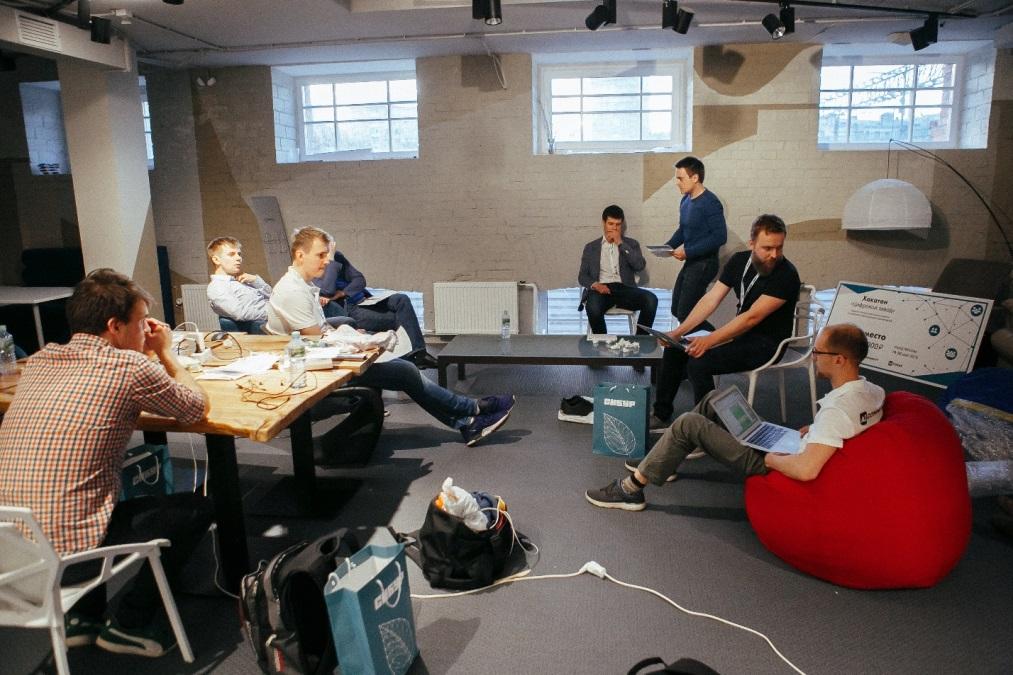
Winners
The main prize (300,000 rubles) went to the Hack.zamAI team.
The guys created a comprehensive solution, not only optimizing financial performance, but also adding a bunch of additional buns there, displaying the finished business process in the product.
At the same time, it still looks decent and friendly.
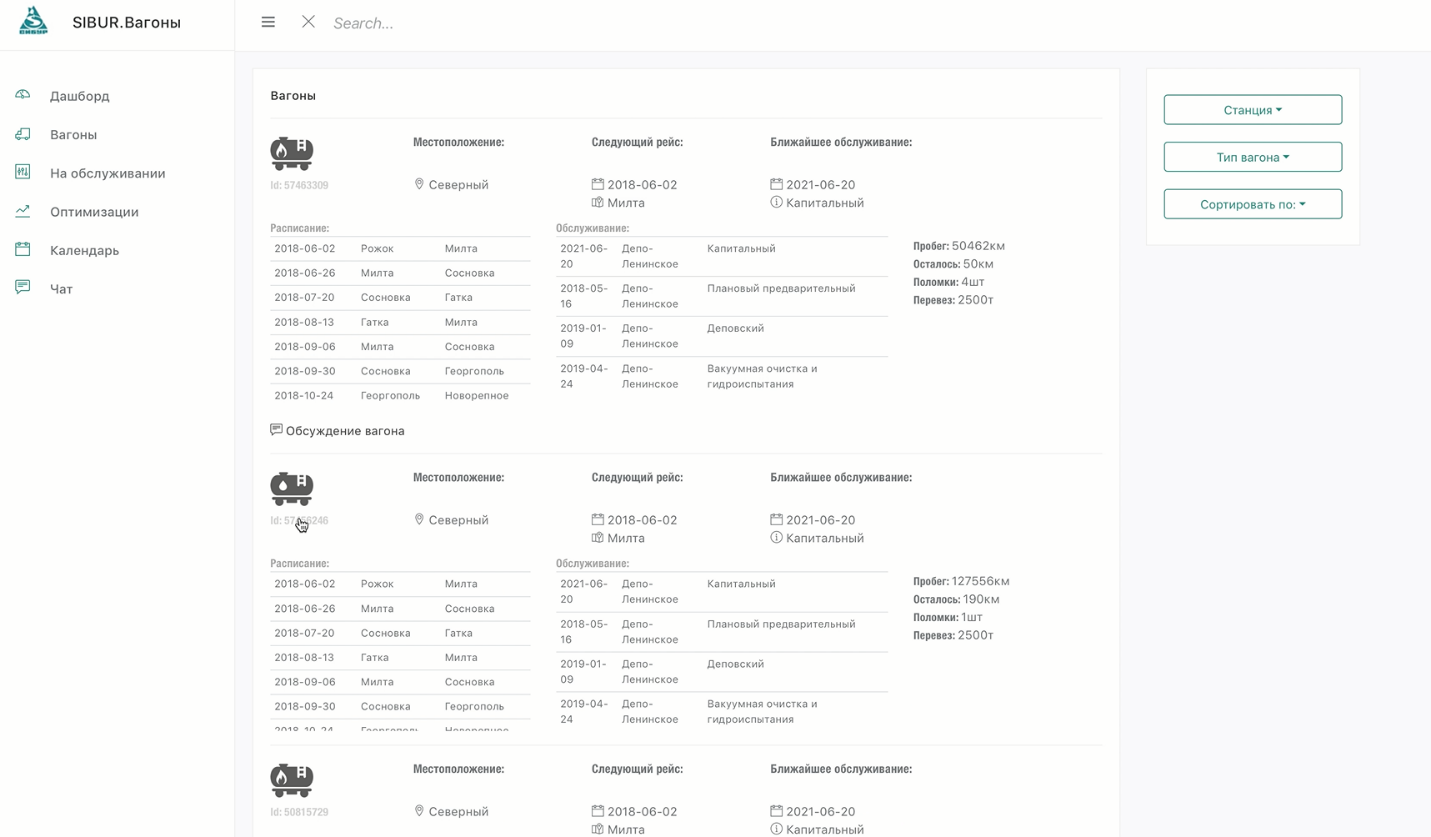
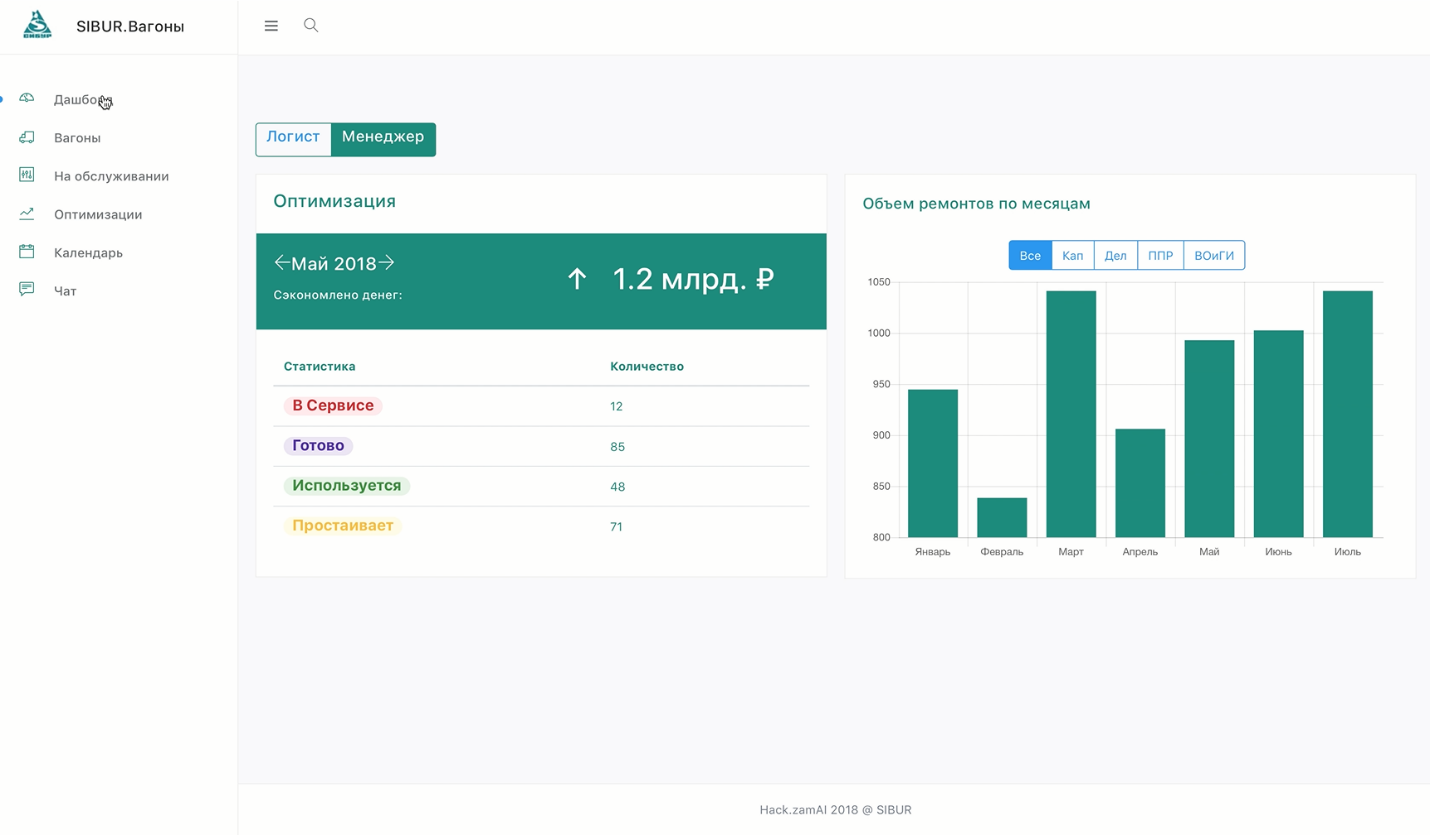
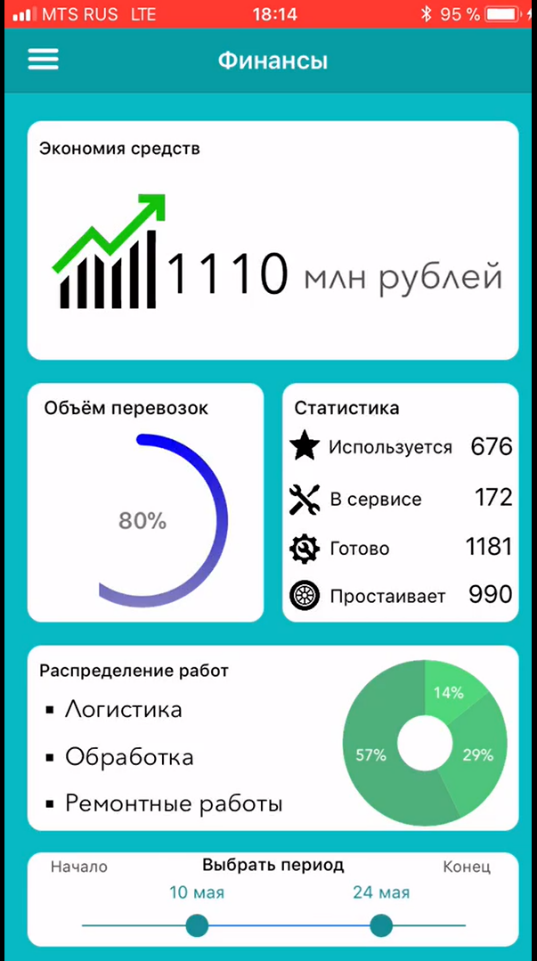
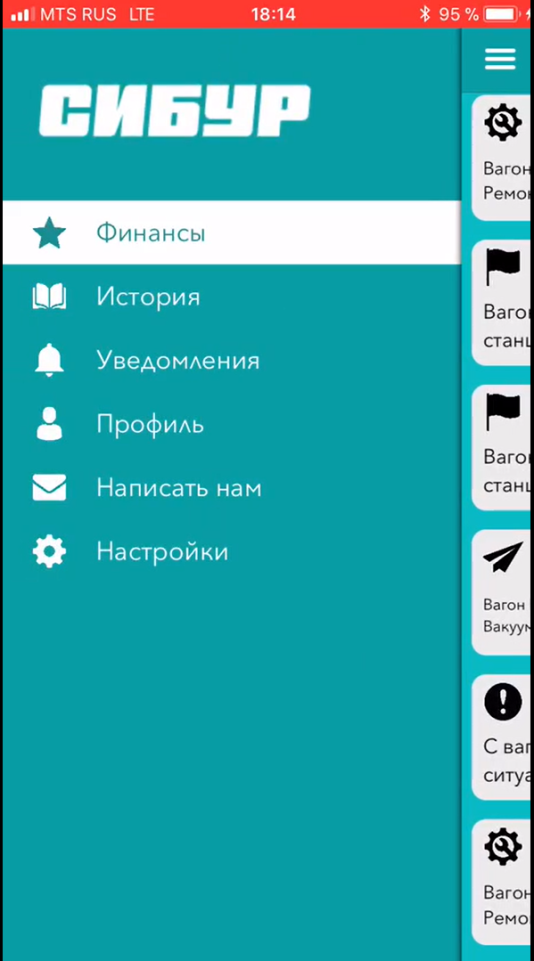
Here you can see a demonstration of their decision.
(video on GoogleDrive)
Of course, this is not our last hackathon.
We want to say thank you to everyone who participated in this. And be sure to post the announcement of the following.
Dmitry Arkhipov, Architect, Digitization of Processes, SIBUR