The secrets of impossible computing on the GPU
Our experience of using a computing cluster of 480 AMD RX 480 GPU in solving mathematical problems. As a problem, we took the proof of the theorem from the article by Professor A. Chudnov. “ Cyclic decompositions of sets, separating digraphs and cyclic classes of games with guaranteed winnings “. The task is to find the minimum number of participants of one coalition in coalition games of Him-type, which guarantees the winning of one of the parties.
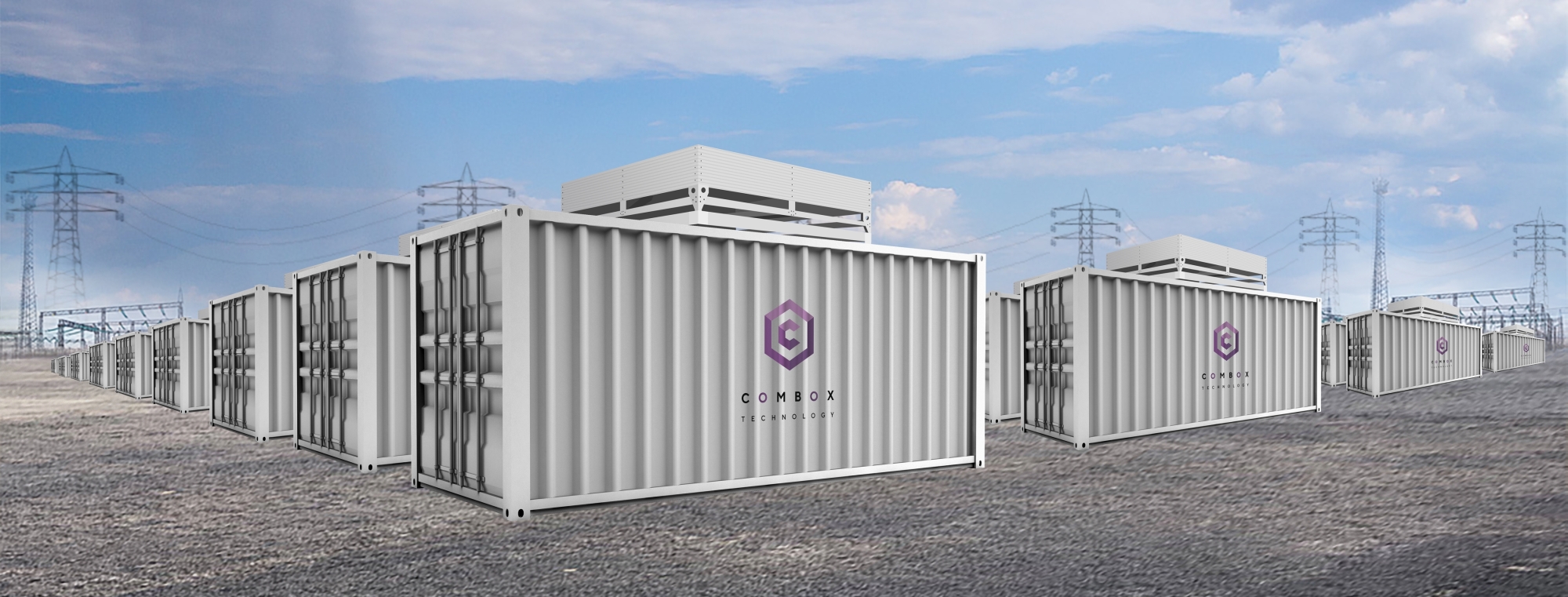
The first processor that received a truly massive distribution is the 8086 from Intel, which was developed in 1978. The 8086 clock frequency was only 8 MHz. A few years later, the first processors appeared inside which there were 2, 4 and even 8 cores. Each kernel allowed to execute the code independently of others. For comparison - a modern Intel Core i9-7980XE processor clocked at 2.6 GHz and contains 18 cores. As you can see - progress does not stand still!
Simultaneously with the development of central processors, video cards also developed. Basically, their characteristics are important for computer games, where new technologies appear especially colorfully and the rendering of 3D images is gradually approaching photographic quality. At the beginning of the development of computer games, the picture was calculated on the CPU, but soon the limit of ingenuity of the developers of 3D graphics, who managed to optimize even obvious things, was reached (a good example of this is InvSqrt () ). So, in video cards began to appear coprocessors with a special set of commands for performing 3D calculations. Over time, the number of such teams grew, which, on the one hand, made it more flexible and efficient to work with the image, and on the other, it complicated the development process.
Since 1996, graphics accelerators S3 ViRGE, 3dfx Voodoo, Diamond Monster and others began to be produced. In 1999, nVidia released the GeForce 256 processor, introducing the term GPU - a graphics processor. It is already universal, it can be engaged in geometrical calculations, coordinate transformation, placement of lighting points and work with polygons. The difference between the GPU and other graphics chips was that inside, besides the specialized commands, there was a set of standard commands with which you could implement your rendering algorithm. This gave a significant advantage, as it allowed to add any special effects, and not just those that are already programmed into the video card. Starting with the GeForce 8000/9000, stream processors have appeared in the GPU - already full-fledged solvers. Their number varied depending on the model from 16 to 128. In modern terminology, they are called unified shader units, or simply shader units. The AMD Vega 64 GPU manufactured today contains 4096 shader units, and the clock frequency can reach 1536 MHz!
The GPU architecture differs from the CPU in a large number of cores and a minimalist set of commands aimed mainly at vector computing. At the architecture level, the issues of parallel operation of a large number of cores and simultaneous access to memory are resolved. Modern GPUs contain from 2 to 4 thousand shader units, which are combined into computing units (Compute Unit). With parallel computing, the problem of simultaneous access to memory is particularly acute. If each of the stream processors tries to write to the memory cell, these commands will be locked into the lock and they will have to be queued, which will greatly reduce performance. Therefore, stream processors execute commands in small groups: while one group performs calculations, another loads registers, and so on. You can also combine cores into working groups,
Another important feature of the GPU is the presence of vector registers and vector ALUs that can perform operations simultaneously for several components of the vector. This is primarily needed for 3D graphics, but since our world is three-dimensional, nothing prevents us from using it for many physical calculations. If there are free vector ALUs, they can be used to calculate scalar values.
For the full operation of the computing system, both types of devices are important. For example, we run a step-by-step program, a kind of sequential algorithm. There is no possibility to perform the fifth step of the algorithm, since the data for it is calculated in step four. In this case, it is more efficient to use a CPU with a large cache and a high clock frequency. But there are whole classes of tasks that can be well parallelized. In this case, the effectiveness of the GPU is obvious. The most common example is the calculation of pixels from a rendered image. The procedure for each pixel is almost the same, the data on 3D objects and textures are in the RAM of the video card, and each stream processor can independently calculate the part of the image.
Here is an example of a modern task - learning a neural network. A large number of identical neurons need to be trained, that is, to change the weights of each neuron. After such changes, you need to pass test sequences for training through the neural network and get error vectors. Such calculations are well suited for the GPU. Each stream processor can behave like a neuron and the calculation will not have to build a solution in a sequential manner, all of our calculations will occur simultaneously. Another example is aerodynamic flow calculation. It is necessary to find out the possible behavior of the designed bridge under the influence of the wind, to model its aerodynamic stability, to find the optimal installation sites for the fairings to adjust the air flow or to calculate the resistance to wind resonance. Remember the famous “dancing bridge” in Volgograd? I think that no one would like to be at that moment on the bridge ...
The behavior of the air flow at each point can be described by the same mathematical equations and solve these equations in parallel on a large number of nuclei.
To perform calculations on a GPU, a special language and compiler are used. There are several frameworks for performing general GPU computations: OpenCL, CUDA, C ++ AMP, OpenACC. The first two became widespread, but the use of CUDA is limited only to the GPU from the company nVidia.
OpenCL was released in 2009 by Apple. Later, Intel, IBM, AMD, Google and nVidia joined the Khronos Group consortium and expressed support for a common standard. Since then, the new version of the standard appears every one and a half to two years and each brings more and more serious improvements.
Today, the OpenCL C ++ version 2.2 language conforms to the C ++ 14 standard, supports simultaneous execution of several programs inside the device, interaction between them through internal queues and pipelines, and allows you to flexibly manage buffers and virtual memory.
An interesting problem from game theory, in the solution of which we took part, is the proof of a theorem from the article of Professor A.M. Chudnov. “ Cyclic decompositions of sets, separating digraphs and cyclic classes of games with guaranteed winnings “. The task is to find the minimum number of participants of one coalition in coalition games of Him-type, which guarantees the winning of one of the parties.
From a mathematical point of view, this is a search for a reference cyclic sequence. If we represent a sequence in the form of a list of zeros and ones, then the reference test can be implemented by logical bitwise operations. From the point of view of programming, such a sequence is a long register, for example, 256 bits. The most reliable way to solve this problem is to go through all the options except for the impossible ones for obvious reasons.
The objectives of the task solution are the issues of efficient signal processing (detection, synchronization, coordinate measurement, coding, etc.).
The difficulty of solving this problem in the search for a huge number of options. For example, if we are looking for a solution for n = 25, then this is 25 bits, and if n = 100, then this is already 100 bits. If we take the number of all possible combinations, then for n = 25, this is 2 ^ 25 = 33,554,432, and for n = 100, this is already 2 ^ 100 = 1,267,650,626,228,429,401,596,705,205,376 combinations. The increase in complexity is simply enormous!
Such a task is well parallelized, which means that it is ideally suited for our GPU cluster.
Initially, mathematicians solved this problem in Visual Basic in Excel, so they managed to get primary solutions, but the low performance of scripting languages did not allow to go far ahead. The decision to n = 80 took one and a half months ... We bow our heads in front of these patient people.
The first step was to implement the C task algorithm and run it on the CPU. In the process, it turned out that much can be optimized when working with bit sequences.
Next, we optimized the search area and eliminated duplication. Also a good result was given by the analysis of the assembler code generated by the compiler and the optimization of the code for the features of the compiler. All this allowed to achieve a significant increase in the speed of calculations.
The next stage of optimization was profiling. Measurement of the execution time of different sections of the code showed that in some branches of the algorithm the load on the memory was greatly increased, and the excessive branching of the program was also revealed. Because of this “small” flaw, almost a third of the CPU power was not used.
A very important aspect of solving such problems is the accuracy of writing code. Nobody knows the correct answers to this problem and there are no test vectors, respectively. There is only the first part of the range of solutions that were found by mathematicians. The reliability of new solutions can be guaranteed only by the accuracy of writing code.
So the stage of preparing the program for solving on the GPU has come and the code has been modified to work in several threads. The control program is now engaged in dispatching tasks between threads. In a multithreaded environment, the computation speed increased by 5 times! This was achieved by the simultaneous operation of 4 threads and the combination of functions.
At this stage, the solution produced correct calculations up to n = 80 in 10 minutes, whereas at Excel it took six weeks to calculate! Little victory!
It was decided to use OpenCL version 1.2 to ensure maximum compatibility between different platforms. Primary debugging was done on a CPU from Intel, then on a GPU from Intel. Already then switched to a GPU from AMD.
The version of the OpenCL 1.2 standard supports integer variables of 64 bits. The dimension of 128 bits is limited to supported by AMD, but compiled into two 64-bit numbers. For compatibility reasons and to optimize performance, it was decided to represent a 256-bit number as a group of 32-bit numbers, the logical bitwise operations on which are performed on the internal ALU GPU as quickly as possible.
An OpenCL program contains a kernel — a function that is the entry point of a program. Data for processing is loaded from the CPU into the RAM of the video card and transferred to the kernel as buffers - pointers to the input and output data arrays. Why an array? We perform high-performance computing, we need a lot of tasks performed at the same time. The kernel runs on the device in multiple instances. Each core knows its identifier and takes exactly its own piece of input data from the common buffer. The case when the simplest solution is the most effective. OpenCL is not only a language, but also a comprehensive framework in which all the details of scientific and game calculations are thoroughly thought out. This makes life easier for the developer. For example, you can run many threads, task manager will place them on the device itself. Those tasks who did not get up for immediate execution, will be put in a waiting queue and run as soon as the computing blocks are released. Each kernel instance has its own space in the output buffer, where it places the answer upon completion of the work.
The main task of the OpenCL dispatcher is to ensure parallel execution of multiple kernel instances. Here applied the accumulated decades of scientific and practical experience. While some cores load data into registers, another part is currently working with memory or performing calculations - as a result, the GPU core is always fully loaded.
The OpenCL compiler does a good job of optimizing, but it's easier for a developer to influence performance. Optimization for the GPU goes in two directions - speeding up code execution and the possibility of its parallelization. How well the code is parallelized by the compiler depends on several things: the number of scratch registers (which are located in the slowest GPU memory - global), the size of the compiled code (you need to fit in 32 kb cache), the number of vector and scalar registers used.
This is the most interesting part of the project when we switched from Excel to a computing cluster consisting of 480 AMD RX 480 video cards. Large, fast, efficient. Fully prepared to perform the task and obtain the results that the world has never seen.
It should be noted that at all stages of improving and optimizing the code, we launched a search for a solution from the very beginning and compared the answers of the new version with the previous ones. This made it possible to be sure that optimization of the code and improvements did not introduce errors into the solutions. Here you need to understand that there are no correct answers at the end of the textbook, and no one in the world knows them.
The launch on the cluster confirmed our assumptions about the speed of solutions: the search for sequences for n> 100 took about an hour. It was amazing to see how on the ComBox A-480 cluster the new solutions were in minutes, while on the CPU it took many hours.
In just two hours of the computing cluster, we got all the solutions up to n = 127. Verification of the decisions showed that the received answers are reliable and correspond to the theorems of Professor A. Chudnov set forth in the article.
If you look at the performance gains in the course of solving the problem, the results were approximately as follows:
Many tasks standing at the intersection of science and practice are awaiting their solution in order to make our life better. The rental market for computing power is only being formed, and the need for parallel computing continues to grow.
Possible uses for parallel computing:
Separate direction - cloud computing on the GPU. For example, such giants as Amazon, IBM and Google lease their computing power on the GPU. Today it is safe to say that the future of high-performance parallel computing will belong to the GPU clusters.
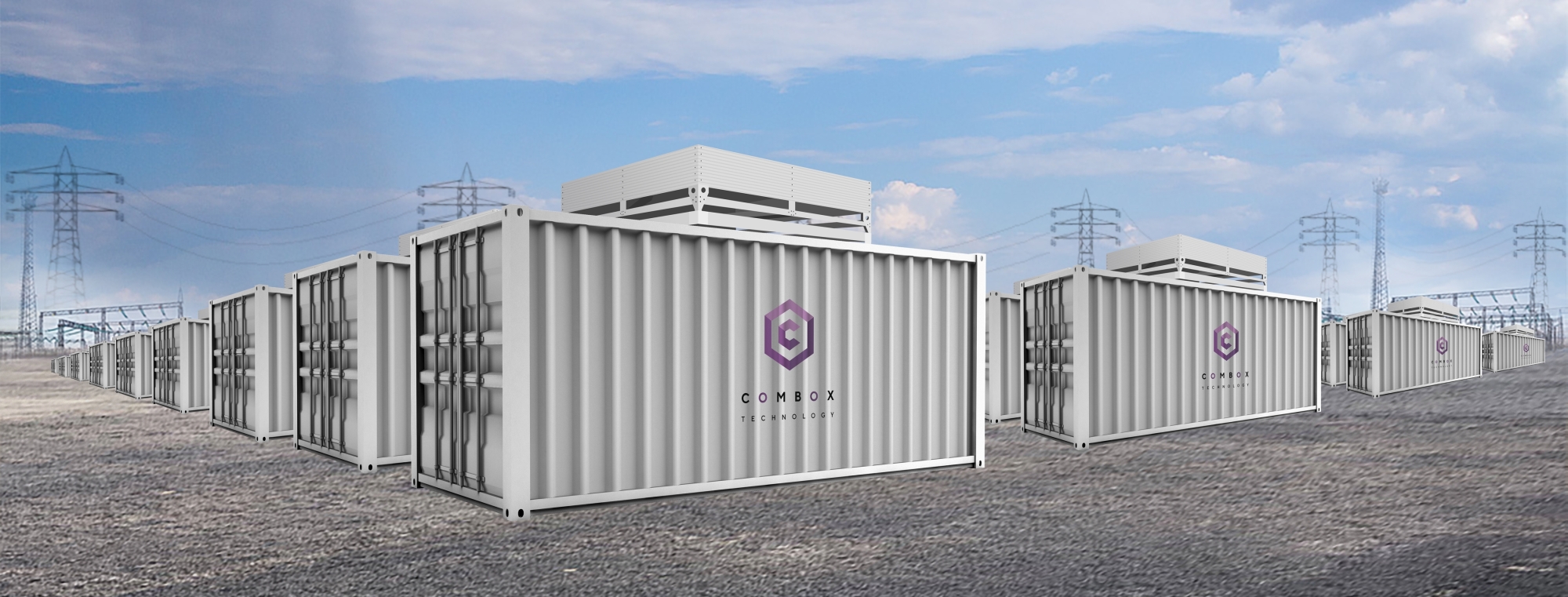
CPU development
The first processor that received a truly massive distribution is the 8086 from Intel, which was developed in 1978. The 8086 clock frequency was only 8 MHz. A few years later, the first processors appeared inside which there were 2, 4 and even 8 cores. Each kernel allowed to execute the code independently of others. For comparison - a modern Intel Core i9-7980XE processor clocked at 2.6 GHz and contains 18 cores. As you can see - progress does not stand still!
GPU development
Simultaneously with the development of central processors, video cards also developed. Basically, their characteristics are important for computer games, where new technologies appear especially colorfully and the rendering of 3D images is gradually approaching photographic quality. At the beginning of the development of computer games, the picture was calculated on the CPU, but soon the limit of ingenuity of the developers of 3D graphics, who managed to optimize even obvious things, was reached (a good example of this is InvSqrt () ). So, in video cards began to appear coprocessors with a special set of commands for performing 3D calculations. Over time, the number of such teams grew, which, on the one hand, made it more flexible and efficient to work with the image, and on the other, it complicated the development process.
Since 1996, graphics accelerators S3 ViRGE, 3dfx Voodoo, Diamond Monster and others began to be produced. In 1999, nVidia released the GeForce 256 processor, introducing the term GPU - a graphics processor. It is already universal, it can be engaged in geometrical calculations, coordinate transformation, placement of lighting points and work with polygons. The difference between the GPU and other graphics chips was that inside, besides the specialized commands, there was a set of standard commands with which you could implement your rendering algorithm. This gave a significant advantage, as it allowed to add any special effects, and not just those that are already programmed into the video card. Starting with the GeForce 8000/9000, stream processors have appeared in the GPU - already full-fledged solvers. Their number varied depending on the model from 16 to 128. In modern terminology, they are called unified shader units, or simply shader units. The AMD Vega 64 GPU manufactured today contains 4096 shader units, and the clock frequency can reach 1536 MHz!
Что содержит в себе GPU

Another important feature of the GPU is the presence of vector registers and vector ALUs that can perform operations simultaneously for several components of the vector. This is primarily needed for 3D graphics, but since our world is three-dimensional, nothing prevents us from using it for many physical calculations. If there are free vector ALUs, they can be used to calculate scalar values.
They are so different, CPU and GPU
For the full operation of the computing system, both types of devices are important. For example, we run a step-by-step program, a kind of sequential algorithm. There is no possibility to perform the fifth step of the algorithm, since the data for it is calculated in step four. In this case, it is more efficient to use a CPU with a large cache and a high clock frequency. But there are whole classes of tasks that can be well parallelized. In this case, the effectiveness of the GPU is obvious. The most common example is the calculation of pixels from a rendered image. The procedure for each pixel is almost the same, the data on 3D objects and textures are in the RAM of the video card, and each stream processor can independently calculate the part of the image.
Here is an example of a modern task - learning a neural network. A large number of identical neurons need to be trained, that is, to change the weights of each neuron. After such changes, you need to pass test sequences for training through the neural network and get error vectors. Such calculations are well suited for the GPU. Each stream processor can behave like a neuron and the calculation will not have to build a solution in a sequential manner, all of our calculations will occur simultaneously. Another example is aerodynamic flow calculation. It is necessary to find out the possible behavior of the designed bridge under the influence of the wind, to model its aerodynamic stability, to find the optimal installation sites for the fairings to adjust the air flow or to calculate the resistance to wind resonance. Remember the famous “dancing bridge” in Volgograd? I think that no one would like to be at that moment on the bridge ...
The behavior of the air flow at each point can be described by the same mathematical equations and solve these equations in parallel on a large number of nuclei.
GPU in the hands of programmers
To perform calculations on a GPU, a special language and compiler are used. There are several frameworks for performing general GPU computations: OpenCL, CUDA, C ++ AMP, OpenACC. The first two became widespread, but the use of CUDA is limited only to the GPU from the company nVidia.
OpenCL was released in 2009 by Apple. Later, Intel, IBM, AMD, Google and nVidia joined the Khronos Group consortium and expressed support for a common standard. Since then, the new version of the standard appears every one and a half to two years and each brings more and more serious improvements.
Today, the OpenCL C ++ version 2.2 language conforms to the C ++ 14 standard, supports simultaneous execution of several programs inside the device, interaction between them through internal queues and pipelines, and allows you to flexibly manage buffers and virtual memory.
Real tasks
An interesting problem from game theory, in the solution of which we took part, is the proof of a theorem from the article of Professor A.M. Chudnov. “ Cyclic decompositions of sets, separating digraphs and cyclic classes of games with guaranteed winnings “. The task is to find the minimum number of participants of one coalition in coalition games of Him-type, which guarantees the winning of one of the parties.
From a mathematical point of view, this is a search for a reference cyclic sequence. If we represent a sequence in the form of a list of zeros and ones, then the reference test can be implemented by logical bitwise operations. From the point of view of programming, such a sequence is a long register, for example, 256 bits. The most reliable way to solve this problem is to go through all the options except for the impossible ones for obvious reasons.
The objectives of the task solution are the issues of efficient signal processing (detection, synchronization, coordinate measurement, coding, etc.).
The difficulty of solving this problem in the search for a huge number of options. For example, if we are looking for a solution for n = 25, then this is 25 bits, and if n = 100, then this is already 100 bits. If we take the number of all possible combinations, then for n = 25, this is 2 ^ 25 = 33,554,432, and for n = 100, this is already 2 ^ 100 = 1,267,650,626,228,429,401,596,705,205,376 combinations. The increase in complexity is simply enormous!
Such a task is well parallelized, which means that it is ideally suited for our GPU cluster.
Programmers vs Math
Initially, mathematicians solved this problem in Visual Basic in Excel, so they managed to get primary solutions, but the low performance of scripting languages did not allow to go far ahead. The decision to n = 80 took one and a half months ... We bow our heads in front of these patient people.
The first step was to implement the C task algorithm and run it on the CPU. In the process, it turned out that much can be optimized when working with bit sequences.
Next, we optimized the search area and eliminated duplication. Also a good result was given by the analysis of the assembler code generated by the compiler and the optimization of the code for the features of the compiler. All this allowed to achieve a significant increase in the speed of calculations.
The next stage of optimization was profiling. Measurement of the execution time of different sections of the code showed that in some branches of the algorithm the load on the memory was greatly increased, and the excessive branching of the program was also revealed. Because of this “small” flaw, almost a third of the CPU power was not used.
A very important aspect of solving such problems is the accuracy of writing code. Nobody knows the correct answers to this problem and there are no test vectors, respectively. There is only the first part of the range of solutions that were found by mathematicians. The reliability of new solutions can be guaranteed only by the accuracy of writing code.
So the stage of preparing the program for solving on the GPU has come and the code has been modified to work in several threads. The control program is now engaged in dispatching tasks between threads. In a multithreaded environment, the computation speed increased by 5 times! This was achieved by the simultaneous operation of 4 threads and the combination of functions.
At this stage, the solution produced correct calculations up to n = 80 in 10 minutes, whereas at Excel it took six weeks to calculate! Little victory!
GPU and OpenCL
It was decided to use OpenCL version 1.2 to ensure maximum compatibility between different platforms. Primary debugging was done on a CPU from Intel, then on a GPU from Intel. Already then switched to a GPU from AMD.
The version of the OpenCL 1.2 standard supports integer variables of 64 bits. The dimension of 128 bits is limited to supported by AMD, but compiled into two 64-bit numbers. For compatibility reasons and to optimize performance, it was decided to represent a 256-bit number as a group of 32-bit numbers, the logical bitwise operations on which are performed on the internal ALU GPU as quickly as possible.
An OpenCL program contains a kernel — a function that is the entry point of a program. Data for processing is loaded from the CPU into the RAM of the video card and transferred to the kernel as buffers - pointers to the input and output data arrays. Why an array? We perform high-performance computing, we need a lot of tasks performed at the same time. The kernel runs on the device in multiple instances. Each core knows its identifier and takes exactly its own piece of input data from the common buffer. The case when the simplest solution is the most effective. OpenCL is not only a language, but also a comprehensive framework in which all the details of scientific and game calculations are thoroughly thought out. This makes life easier for the developer. For example, you can run many threads, task manager will place them on the device itself. Those tasks who did not get up for immediate execution, will be put in a waiting queue and run as soon as the computing blocks are released. Each kernel instance has its own space in the output buffer, where it places the answer upon completion of the work.
The main task of the OpenCL dispatcher is to ensure parallel execution of multiple kernel instances. Here applied the accumulated decades of scientific and practical experience. While some cores load data into registers, another part is currently working with memory or performing calculations - as a result, the GPU core is always fully loaded.
The OpenCL compiler does a good job of optimizing, but it's easier for a developer to influence performance. Optimization for the GPU goes in two directions - speeding up code execution and the possibility of its parallelization. How well the code is parallelized by the compiler depends on several things: the number of scratch registers (which are located in the slowest GPU memory - global), the size of the compiled code (you need to fit in 32 kb cache), the number of vector and scalar registers used.
ComBox A-480 GPU or one million cores
This is the most interesting part of the project when we switched from Excel to a computing cluster consisting of 480 AMD RX 480 video cards. Large, fast, efficient. Fully prepared to perform the task and obtain the results that the world has never seen.
It should be noted that at all stages of improving and optimizing the code, we launched a search for a solution from the very beginning and compared the answers of the new version with the previous ones. This made it possible to be sure that optimization of the code and improvements did not introduce errors into the solutions. Here you need to understand that there are no correct answers at the end of the textbook, and no one in the world knows them.
The launch on the cluster confirmed our assumptions about the speed of solutions: the search for sequences for n> 100 took about an hour. It was amazing to see how on the ComBox A-480 cluster the new solutions were in minutes, while on the CPU it took many hours.
In just two hours of the computing cluster, we got all the solutions up to n = 127. Verification of the decisions showed that the received answers are reliable and correspond to the theorems of Professor A. Chudnov set forth in the article.
Evolution of speed
If you look at the performance gains in the course of solving the problem, the results were approximately as follows:
- month and a half to n = 80 in Excel;
- hour to n = 80 on Core i5 with an optimized C ++ program;
- 10 minutes to n = 80 on Core i5 using multi-threading;
- 10 minutes to n = 100 on a single AMD RX 480 GPU;
- 120 minutes to n = 127 on ComBox A-480.
Perspectives and Future
Many tasks standing at the intersection of science and practice are awaiting their solution in order to make our life better. The rental market for computing power is only being formed, and the need for parallel computing continues to grow.
Possible uses for parallel computing:
- tasks of automatic control of vehicles and drones;
- calculations of aerodynamic and hydrodynamic characteristics;
- speech recognition and visual imagery;
- neural network training;
- tasks of astronomy and astronautics;
- statistical and correlation data analysis;
- folding protein-protein compounds;
- early diagnosis of diseases using AI.
Separate direction - cloud computing on the GPU. For example, such giants as Amazon, IBM and Google lease their computing power on the GPU. Today it is safe to say that the future of high-performance parallel computing will belong to the GPU clusters.