
Profession Data Scientist: how not to make a mistake with the choice
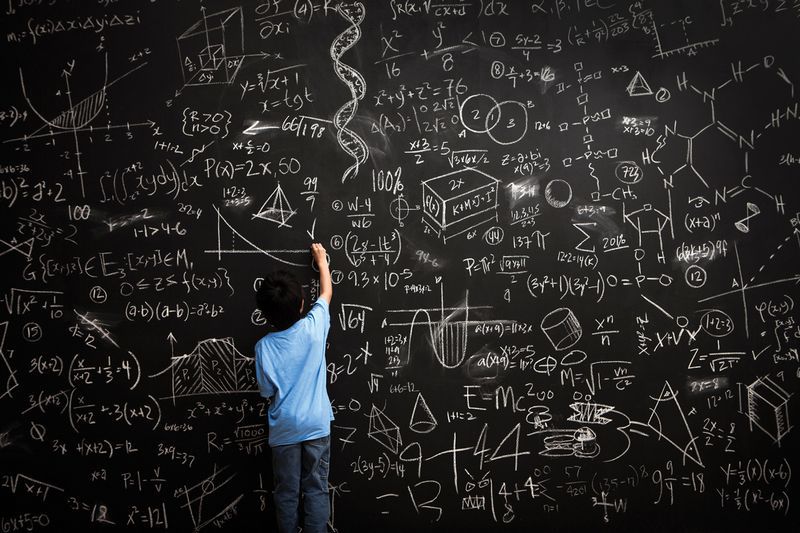
Does a person like to play with numbers or numbers with a person? There is a funny paradox in classical secondary education: schoolchildren are trained to memorize the rules and cases of their application, but the more the student knows the rules and exceptions, the more often he has the opportunity to make a mistake. In the dictation, woven from the texts of classical Russian literature, the abundance of commas of a clarifying nature leads to the idea that it is the unset comma that is a mistake. Therefore, competent work is an essay with a large number of commas. The problem of cause and effect, isn’t it? Maybe if you are a good writer, you use a lot of commas of a clarifying nature, but this is not the case when the number of commas makes you a good writer ...
The interpretation of commas in classical Russian literature is an example of poor data analysis built on the lack of curiosity and understanding of mathematical statistics. These factors + a passionate desire to develop in the field of information technology are key in understanding the specialty of a “data scientist”. The post is based on a presentation by an Airbnb employee, a data science specialist.

We will not dwell in detail on why the data scientist profession is noted as one of the most attractive and promising in the world. It’s enough to mention that the number of vacancies in this direction is growing exponentially, and according to McKinsey Global Institute estimates, by 2018 in America alone, an additional 190 thousand data specialists with training in statistics and machine learning will be needed. McKinsey noted that additionally, millions of managers will need to be trained in basic data skills.
This is a huge market that is just emerging, however, big data problems and ways to solve them did not arise yesterday. The amount of archived data accumulated over the years of work only in Airbnb is several petabytes of data. Dozens of terabytes of information are processed daily using storage built on the basis of Apache Hadoop and Hive. We already talked about the personalized search system Airbnb - it was created on the Storm distributed processing system in real time. For Airbnb, user data analysis is needed to make almost any decision to develop a company. And we need data scientist professionals.
Today, only a third of the demand for data science professionals can be met. An undersaturated market cannot provide companies with qualified personnel in the field of data mining or predictive analytics, which leads to an increase in demand and salaries. Public and private universities do not cope with the process of training data specialists.
Data Scientist: personality traits
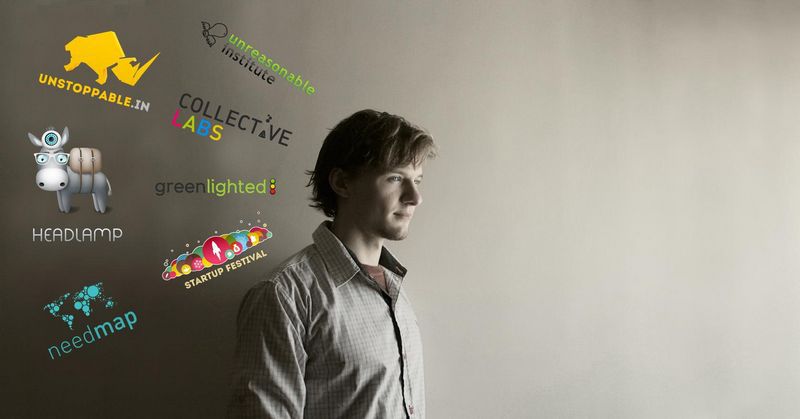
A number of technical universities offer a training program for “Masters of Science in Data Science and Management”. The specialty will require you to have deep knowledge in the field of mathematical statistics, machine learning, and programming. However, no training can be compared with the experience that you get directly from work, faced with real problems. Only work will demonstrate to you that the chosen path is not the easiest in life.
Engaging in data science is as difficult as engaging in science in general. As in ordinary scientific disciplines, most of the methods you use will not work. You can’t just go into the laboratory, click your fingers and get the result. You will come up with a lot of interesting (just great!) Things: how to make the system better, how to configure and optimize the selection, and the like. About two-thirds of your ideas will not work. The vast majority of the time you will fail. And must be prepared for this.
To be a good data scientist is not enough to be a good programmer. You should be better versed in statistics than in software engineering. A competent data scientist is a competent statistician. The specialists around you understand everything else better - and this is normal, you should be able to listen to them, receive from them the data necessary in your work.
Data scientist is a person who loves math. Employers who are looking for a specialist in the field of data should first of all pay attention to mathematical specialties. You have not studied math and are afraid to put an end to your career? There is an alternative way - the study of computer science. And you can succeed in academic science. Mentality is important, understand? You can be a specialist in neuroscience and decide to study data - mathematics will welcome you with open arms.
Immersion in mathematics should not stop you from studying computer systems. Otherwise, it’s easier to become a teacher. This is a big problem in fact, that mathematicians do not understand the scale of the data used, they do not understand the very structure of computer data and, as a result, are not able to simulate the appearance of system problems in the future. There is always a gap between a probabilistic mathematical model, which, as you assume, corresponds to the structure of your problem, and the actual data that you are trying to analyze. Collecting statistics means rushing between the model and the data. It is very important to understand this at a deep level, and not to treat mathematics (and computer systems) as a magic box, where you can put numbers, turn the knob and get the result.
Data Scientist: how to become one

A person acts according to the patterns embedded in the head. When considering a problem, you operate with ready-made behaviors. Data scientist works with random variables and probabilistic models, because his task is to identify the most unexpected patterns. If you want to hire such a specialist, and admit to yourself that you do not know much about statistics, offer the person you are interviewing a test completely out of context. Taken out of context. And you will see how he will handle the problem without knowing how to solve the problem. This is the essence of the work - to think not about pre-obtained statistics, not about computer models of a solution, but about a problem. Such a solution demonstrates the ability of a specialist to operate with probabilistic models with complex data.
So, you are ready to do all these things, you understand statistics, understand the data structure and algorithms, or you are a scientist who understands what lies at the base of modeling. Now you can get a job. But there is still a mass of everything in the world that you do not know, which is difficult to understand, because it is not listed in textbooks. For example, most data analysts do not understand how teams work in software development. This is very scary and unnerving when you come in contact with an environment with strange material. There is nothing demeaning to admit it and start all over again - to become a student of more experienced developers.
Watching a software project develop from scratch is an invaluable experience. Another way to gain experience interacting with a real environment is to participate in the Kaggle project.. The resource is used to solve complex problems in various fields of knowledge (marketing, finance, banking, medicine, insurance, research). Kaggle turns company business tasks into a structured data set that is easy to work with.
Data Scientist: not being who you are not

Do not try to be who you are not. It is not uncommon for a data scientist to be perceived as a data analytics. The analyst can say: "If my data analysis tools cannot answer the question, then the question remains unanswered." Here we ask a question to the database and, if it doesn’t come back in half an hour, we cancel it and move on to the next.
A data scientist reflects as follows: “If my data analysis tools cannot answer the question, then I need better tools and data.” This example explains best of the above how to be a data scientist. The scientist does not say: I can not answer the question, I’ll go do something else. The scientist continues to think about the question and find out ways by which he can answer it.