"You're one ugly motherf ** ker": hate speech algorithms and methods for circumventing them

Each of the technologies created from the very moment a person picked up a stone is obliged to improve a person’s life by fulfilling its basic functions. However, any technology can have “side effects”, that is, to influence a person and the world around him in a way that no one thought about or did not want to think about at the time of this technology. A vivid example: cars were created, and a person was able to travel long distances at a greater speed than before. But at the same time the pollution began.
Today we will talk about the "side effect" of the Internet, which affects not the atmosphere of the Earth, but the minds and souls of the people themselves. The fact is that the world wide web has become an excellent tool for the dissemination and exchange of information, for communication between people who are physically distant from each other and for many other things. The Internet helps in various areas of society, from medicine to the banal preparation for the control work on history. However, the place in which a great multitude gathers, sometimes nameless, voices and opinions, sadly enough, is filled with the fact that it is so inherent in man - with hatred.
In today's study, scientists dismantled several algorithms, the main task of which is to identify abusive, rude and hostile messages. They managed to break all these algorithms, thereby demonstrating their low level of efficiency and pointing out the errors that should be corrected. How scientists broke what supposedly worked, why they did it, and what conclusions all of us should draw - we will look for answers to these and other questions in the report of the researchers. Go.
Background to the study
Social networks and other forms of Internet interaction between people have become an integral part of our life. Unfortunately, many of the users of such services too literally understand this phenomenon as “freedom of speech, thought and expression”, covering this law with their indecent, familiar, and rude behavior on the web. Each of us in one way or another came across the “activity” of such individuals. Many even became the object of such speeches. Of course, one cannot deny that a person has every right to say what he thinks. However, expressing one's thoughts is one thing, and offending someone is another. In addition to the freedom of speech, anonymity is also exploited, because you can say anything to anyone, while remaining incognito. As a result, you will not incur any penalty for your inappropriate behavior.
It is not necessary to explain that the phrases “I did not like it” and “this is a complete de ** mo, the author kill the wall” (this is a more or less decent option) have completely different emotional colors, although they carry a common essence - the commentator I do not like what he saw / read / heard, etc. But if you prohibit a person from expressing his dissatisfaction in this way, is this considered a violation of his rights? Many will say yes. On the other hand, is it worth it to continue turning a blind eye to the exponentially growing hatred on the Internet, which in most cases is not justified. Hate, as such, is the place to be. Of course, this is a very strong and incredibly negative emotion. However, if a person hates someone who has done something terrible (murder, rape and other inhuman acts), it can still be somehow justified.
Now, many companies and research groups have decided to create their own algorithms that can analyze any text and report where hate speech is present * , and to what degree of power it is expressed. Our today's heroes decided to check these algorithms, in particular, the very Google Acpective API, which defines the “acidity” of the phrase, i.e. how much this phrase can be regarded as an insult.
The language of enmity * - as is clear from the very name of this term, it is a combination of linguistic means aimed at expressing bright hostility between interlocutors. The most common forms of hate speech are: racism, sexism, xenophobia, homophobia, and other forms of hostility towards something else.The main tasks that researchers have set themselves are to study the most popular algorithms for detecting hate speech, understand their methods of work, and try to circumvent them.
Algorithms participating in the study
Scientists have chosen several algorithms whose databases differ from each other, which allows us to determine the best base as well. Some algorithms rely more on the identification of sexual connotations * , others rely on religious ones. Common to all algorithms is the source of their knowledge - Twitter. According to the researchers, this is far from perfect, since this service has certain limitations (for example, the number of characters in one message). Consequently, the base of the effective algorithm should be filled from different social networks and services.
Connotation * - a method of coloring a word or phrase with additional semantic or emotional hues. It may differ depending on language, cultural or other forms of social separation. Example: windy - “the day was windy” (the direct meaning of the word), “he was always a windy man” (in this case means inconstancy and frivolity).The list of algorithms and their functionality:
Detox : a Wikipedia project for identifying inappropriate language in editorial comments. It works on the basis of logistic regression * and multilayer perceptron * , using N-gram * models at the level of letters and words. The size of an N-gram word varies from 1 to 3, and the letters from 1 to 5.
Logistic regression * - a model predicting the probability of an event by fitting data to a logistic curve.
Multi-layer perceptron * - information perception model consisting of three main layers: S - sensors (receiving a signal), A - associative elements (processing) and R-reacting elements (response to a signal), as well as an additional layer A.
N-gram * is a sequence of n elements.The data for the algorithm base was collected by third parties, and each of the comments was evaluated by ten evaluators.
T1 : algorithm with a base broken into three types of comments from Twitter (hate speech, hate speech insults and neutral). Researchers say that this is the only base with a similar division into categories. Hate speech was detected through a search on Twitter using predetermined patterns. Further, the results were evaluated by three employees of CrowdFlower (now Figure Eight Inc., a study of machine learning and artificial intelligence). Most of the base (76%) are offensive phrases, while hate speech takes up only 5%.
T2: algorithm using deep neural networks. The main focus was on long-term short-term memory (LSTM). The base of this algorithm is divided into three categories: racism, sexism and nothing. The researchers combined the first two categories into one, forming an integral category of hate speech. The basis of the base served as 16,000 tweets.
T1 * , T3 : a convolutional neural network (CNN) algorithm and controlled recurrent units (GRU) using the T1 knowledge base, complementing it with separate categories aimed at refugees and Muslims (T3).
Efficiency of algorithms
The efficiency of algorithms was tested by two methods. In the first, they worked as originally intended. And in the second, the algorithms were trained through the databases of each of them, a kind of exchange of experience.
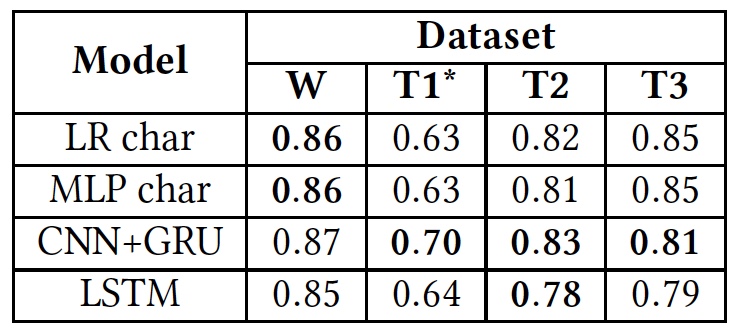
Test results (in bold, the results of using original databases are highlighted).
As can be seen from the table above, all the algorithms showed approximately the same results when applied to different texts (databases). This means that they all studied using the same type of text.
The only significant deviation is seen at T1 *. This is due to the fact that the database of this algorithm is extremely unbalanced, according to scientists. Hate speech takes up only 5%, as we already know. The initial division into three categories of texts was transformed into a division into two, when “insults, but without hate speech” and “neutral” texts were combined into one group, occupying about 80% of the entire base.
Next, the researchers retrained the algorithms. First used the original database. After that, each of the algorithms had to work with the base of another algorithm, instead of its “native” one.
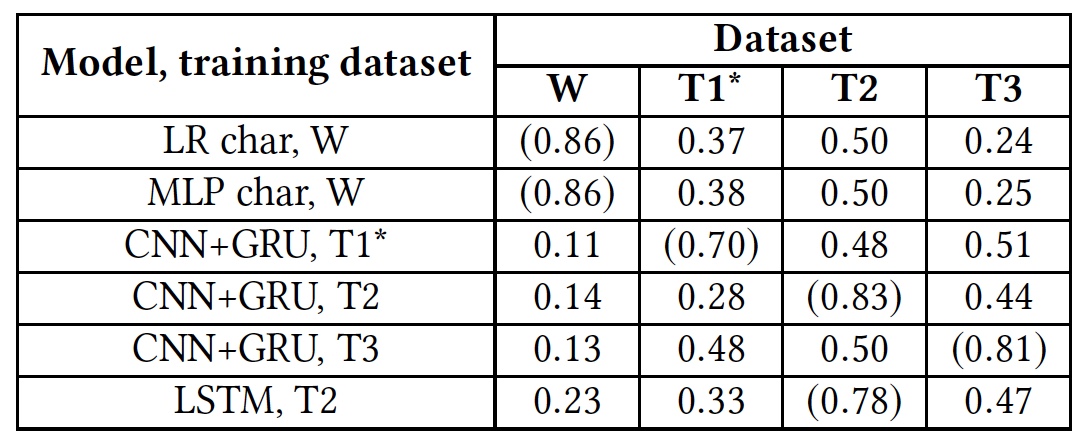
The results of the retraining test (results using native bases are indicated by parentheses).
This test showed the absolute unpreparedness of all algorithms to work with foreign databases. This suggests that the linguistic indicators of hate speech do not overlap in different bases, which may be due to the fact that there are very few matching words in different bases, or because of inaccuracies in the interpretation of certain phrases.
Insults and Hate speech
The researchers decided to pay special attention to two categories of texts: offensive and with hostile language. The bottom line is that some algorithms combine them into one heap, while others try to separate them as independent groups. Of course, insults are obviously a negative phenomenon, and it can be easily attributed to one category with hostility. However, defining insults is a much more complex process than identifying obvious hatred in a text.
To test the algorithms for the ability to detect insults was used base T1. But the T1 * algorithm did not take part in this test, due to the fact that it is already prepared for such work, which makes the results of its testing non-objective.
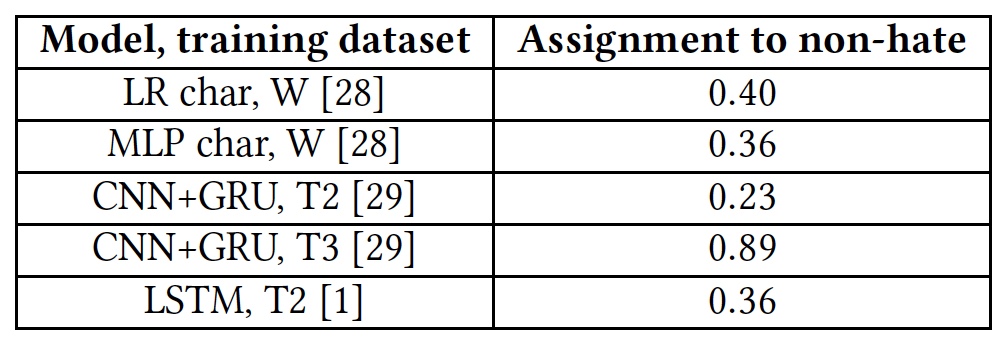
Test results for the ability to identify offensive texts.
All algorithms showed rather mediocre results. The exception was T3, but not at the expense of their talents. The fact is that words that are unfamiliar to the algorithm are marked with the unk tag . Almost 40% of the words in each sentence were marked with this tag, and the algorithm automatically counted them as insults. And this, of course, was far from always correct. In other words, the T3 algorithm also failed to cope with the task in view of its short vocabulary.
Scientists consider one of the main problems of algorithms to be the human factor. Most of the databases of each of the algorithms are collected, analyzed and evaluated by people. And then there may be strong disagreements results. The same phrase may seem offensive to some people or neutral to others.
Also, a negative effect is also played by the lack of understanding of non-standard phrases in algorithms, which can easily contain foul language, but at the same time have no insults or hate language.
To demonstrate this, a test was performed with several phrases. Then the test was repeated, but in each of the phrases they added a very common in the English language abusive word " f * ck " (marked with the letter F in the table).
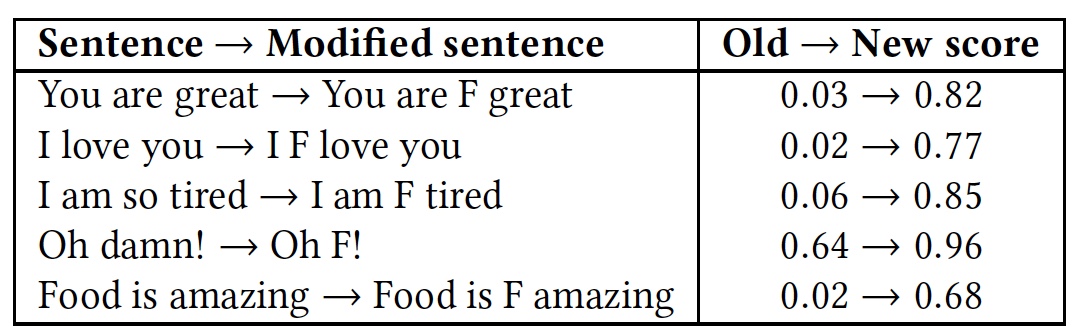
Comparative results of recognizing phrases with and without the word "f * ck".
As can be seen from the table, it was worth adding the word to the letter F, as all the algorithms immediately perceived the phrase as an enmity language. Although the essence of the phrases remained the same, friendly, but the emotional color changed to a more pronounced one.
The above Google Perspective API tests show similar results. This algorithm is also incapable of distinguishing the language of hostility from insults, and an insult from a simple epithet used for emotional embellishment of a phrase.
How to cheat the algorithm?
As it often happens, if someone breaks something, it is not always bad. And all because, having broken, we reveal the lack of a system, its weak point, which should be improved, having prevented the repetition of a breakdown. The above models were no exception, and the researchers decided to see how to disrupt their work. As it turned out, it was not as difficult as the creators of these algorithms thought.
The bypass model of the algorithm is simple: a hacker knows that his texts are being checked, he can change the input data (text) in such a way as to avoid detection. The hacker has no access to the algorithm itself and its structure. Simply put, the intruder breaks the algorithm exclusively at the user level.
The bypass of the algorithm (let's call it the old kind word “hacking”) is divided into three types:
- Change the words: intentional misprints and Leet, that is, replacing some letters with numbers (for example: You look great today! - Y0U 100K 6r347 70D4Y!);
- Change the space between words: add and remove spaces;
- Add words at the end of a phrase.
The first hacking program, word change, must successfully accomplish three tasks: reduce the degree of word recognition by the algorithm, avoid spelling corrections, and preserve the readability of words for a person.
The program swaps two letters in a word. Preference is given to letters closer to the middle of the word and to each other. Only the first and last letters in the word are excluded. Next, the words are changed with an eye to Leet, where some letters are replaced with numbers: a - 4, e - 3, l - 1, o - 0, s - 5.
In order to deal with such tricks, the algorithms were slightly improved by introducing spelling checks and stochastic transformations of the training knowledge base. That is, not only the main words were present in the database, but also their modified forms by rearranging the form letters.
However, the longer the word, the more options for rearranging letters exist, which expands the capabilities of the cracker program.
The method of removing or adding spaces also has its own characteristics. Removing spaces is more suitable for countering algorithms that analyze whole words. But algorithms that analyze each letter can easily cope with the absence of spaces.
Adding spaces may seem like a very inefficient method, but it is still capable of fooling some algorithms. Models that consider words in their entirety conduct a lexical analysis of a phrase, breaking it up into components (lexemes). In this case, the space serves as a word separator, that is, an important element of the phrase analysis. If there are more spaces than necessary, then the words between them become unrecognizable for the algorithm. At the same time, this bypass method retains a high degree of readability of phrases for a person. The method works simply: a random letter is selected in the word, followed by a space. As a result, a word that was previously known to the algorithm ceases to be such. Example: “Hate” - “Hate Whist.” If we remove all the gaps in the text, then the whole phrase will become for the algorithm one unintelligible word. Like in that story where the daughter gave her mother a new phone, and she wrote her a text message with the text: “dear, as it were, put the space on this phone”. We can read this phrase, but the algorithm will take it as one word, which, of course, he does not know.
However, if the algorithm analyzes the letters separately, then it will be able to recognize the phrase, therefore this hacking method is not suitable in such cases.
To counter such attacks, the algorithms were also re-trained. To combat the addition of spaces, the base of algorithms went through a program of random insertion of spaces: a word of n letters can be separated by a space in n-1 ways. However, this led to a combinatorial explosion, when the complexity of the algorithm increases dramatically due to an increase in the size of the input data. As a result, learning the algorithm, based on the well-known method of adding spaces, is extremely difficult and ineffective.
With the removal of spaces, there are also difficulties. If the algorithm base is replenished with phrases that it knows, but already without spaces, then it will work effectively only if such a phrase is applied. It is necessary to replace a couple of letters or a word, and the algorithm does not recognize anything.
In the hacking method by adding words, the main essence is how the recognition algorithm works. He divides words into categories, say "good" and "bad." If the phrase contains more “good” ones, then most likely the algorithm will determine the entire phrase as “good”. And vice versa. If we add a random “good” word to the “bad” meaning of the phrase, then the algorithm can be deceived, and the meaning of the phrase for the person reading it will remain the same. The hacking program generates random numbers (from 10 to 50) or words at the end of each phrase. As a source of random words, a list of the most common common English words provided by Google was chosen.

Table of the results of applying the above described methods of hacking and the reaction of algorithms to this (A - attack, AT - training based on the principle of an attacking program, SC - spell checking, RW - removing spaces).
To demonstrate the method of circumvention by introducing additional words, the researchers conducted a test using the word "love" (love). This word was added at the end of offensive phrases.

Test results with the addition of the word "love".
The table shows that this method was able to fool all the algorithms that analyze words entirely. Algorithms analyzing the letters separately also suffered, but not so significantly.
Previously, the Google Perspective API has already been tested, which showed its ineffective ability to recognize neutral and offensive texts.
As test phrases then used (I will not translate for obvious reasons):
S1: Climate change is happening and it’s not changing in our favor. If you think differently you’re an idiot.
S2: They’re stupid, it’s getting warmer, we should enjoy it while it lasts
S3: They are liberal idiots who are uneducated
S4: idiots. backward thinking people. nationalists. not accepting facts. susceptible to lies.
S5: They are stupid and ignorant with no class
S6: It’s stupid and wrong
S7: If they voted for Hilary they are idiots
S8: Anyone who voted for Trump is a moron
S9: Screw you trump supporters
S2: They’re stupid, it’s getting warmer, we should enjoy it while it lasts
S3: They are liberal idiots who are uneducated
S4: idiots. backward thinking people. nationalists. not accepting facts. susceptible to lies.
S5: They are stupid and ignorant with no class
S6: It’s stupid and wrong
S7: If they voted for Hilary they are idiots
S8: Anyone who voted for Trump is a moron
S9: Screw you trump supporters
Researchers used the same phrases in their research. The result was significantly better, which suggests that the Google Perspective API has been improved. However, using the method of removing spaces in combination with the introduction of the word “love” on each of the above phrases showed a completely different result.
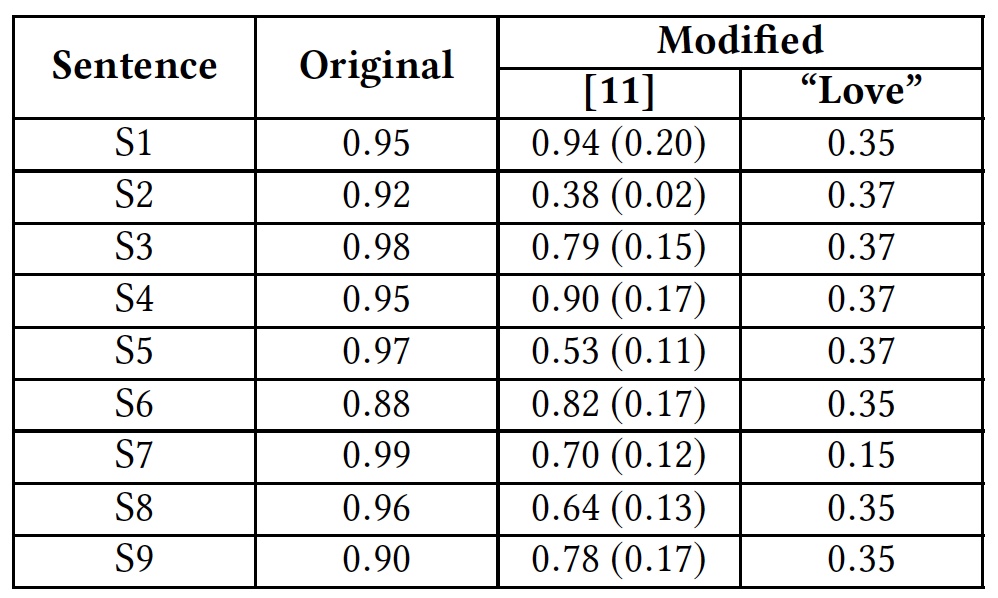
Google Perspective API: degree of "toxicity" (offensiveness) of phrases.
In parentheses are the current results in comparison with those that were before the improvement of the Google Perspective API.
For a more detailed acquaintance with this study, you can use the report of scientists available here .
Epilogue
The researchers deliberately used the simplest methods of cheating algorithms to demonstrate their low degree of efficiency, as well as to indicate to the developers that they are not weak points that require their attention.
Programs that can track offensive phrases online are certainly a great idea. But what next? Should this program block such phrases? Or should she offer an alternative version of the phrase that will not contain insults? There are a lot of questions on the ethics side: freedom of speech, censorship, culture of behavior, equality of people and so on. Does the program have the right to decide what person has the right to say and what not? Maybe. However, the implementation of such a program must be flawless, devoid of flaws that can be used against it. And while scientists continue to puzzle over this issue, society itself must decide for itself - is the total freedom of speech so beautiful, or can it be sometimes limited?
Thank you for staying with us. Do you like our articles? Want to see more interesting materials? Support us by placing an order or recommending to friends, 30% discount for Habr's users on a unique analogue of the entry-level servers that we invented for you: The whole truth about VPS (KVM) E5-2650 v4 (6 Cores) 10GB DDR4 240GB SSD 1Gbps from $ 20 or how to share the server? (Options are available with RAID1 and RAID10, up to 24 cores and up to 40GB DDR4).
VPS (KVM) E5-2650 v4 (6 Cores) 10GB DDR4 240GB SSD 1Gbps until December for free if you pay for a period of six months, you can order here .
Dell R730xd 2 times cheaper? Only we have 2 x Intel Dodeca-Core Xeon E5-2650v4 128GB DDR4 6x480GB SSD 1Gbps 100 TV from $ 249in the Netherlands and the USA! Read about How to build an infrastructure building. class c using servers Dell R730xd E5-2650 v4 worth 9000 euros for a penny?
Only registered users can participate in the survey. Sign in , please.