The classic math problem manifests itself in the real world.
- Transfer
A hundred years ago, the great mathematician David Hilbert asked a research question from the field of pure mathematics. Recent developments in optimization theory carry Hilbert's work to the world of robots
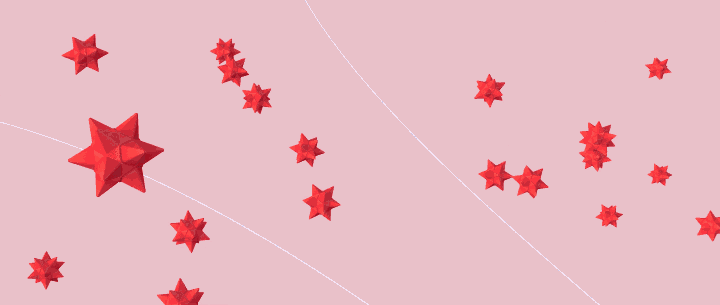
Long before the robots could run and the cars drive themselves, mathematicians pondered a simple mathematical question. Finally, they sorted out with him and laid aside - not being able to know that the object of their mathematical curiosity would manifest itself in the machines of the distant future.
The future has come. As a result of the new work of Amir Ali Ahmadi and Aniruda Majumar from Princeton University, the classical problem of pure mathematics is ready to provide iron proof that automatic drones and robomobils will not crash into trees or taxi into the oncoming lane.
"A complete, 100% provable guarantee is given that your system can" avoid collisions, said Georgina Hall , a Princeton graduate student who collaborated with Ahmadi on this work.
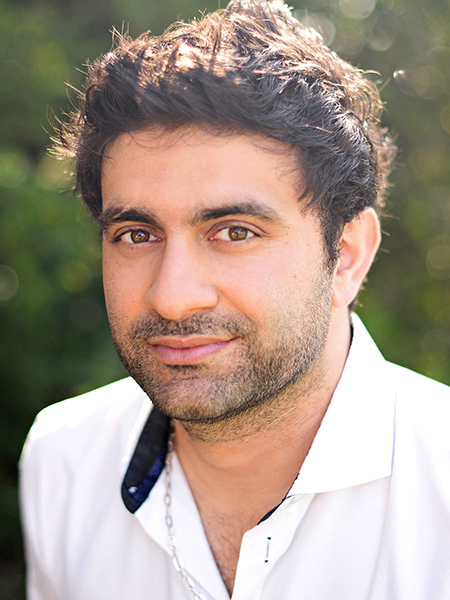
guarantee is taken in an unexpected place - in a mathematical problem known as the " sum of squares ". She put in the 1900th year, the great mathematician David Gilbert. He asked whether certain equations can always be expressed as the sum of two separate terms, each of which is squared.
Mathematicians answered Hilbert's question a few decades later. Then, almost 90 years later, computer scientists and engineers discovered that this mathematical property — expressibility of the equation in terms of a sum of squares — helps solve many real-world problems that they would like to solve.
“A lot of classical mathematics of the XIX century is used in what I do, along with very modern computational mathematics,” said Ahmadi.
However, as soon as the researchers realized that the sum of the squares could help answer many types of questions, they were faced with the problems of applying this approach. The new work eliminates one of the largest such problems, forcing the old mathematical question to solve some of the most important technological issues of our time.
Positively guaranteed
What does it mean that a certain value is the sum of squares? Take the number 13. This is the sum of two squares - 2 2 and 3 2 . The number 34 is equal to the sum of 3 2 and 5 2 .
Instead of numbers, the Hilbert question — the 17th of the 23 problems that he proposed at the dawn of the 20th century — deals with polynomials, such as 5x 2 + 16x + 13. Such polynomials can also sometimes be represented as a sum of squares. For example, 5x 2 + 16x + 13 can be rewritten as (x + 2) 2 + (2x + 3) 2 .
When an expression is a sum of squares, you know that it is always positive (all [real] numbers squared give a positive number or zero, and the sum of positive numbers is positive). Hilbert wanted to know if it works the other way: can all non-negative polynomials be expressed as the sum of squares of rational functions. In 1927 mathematician Emil Artin proved that Hilbert's hypothesis was correct.
This relationship is quite helpful. If you are given a complex polynomial, with dozens of variables raised to a higher degree, it is rather difficult to immediately determine whether it is always non-negative. “Some polynomials are obviously non-negative, others are not. It is difficult to test them for non-negativity, ”said Ahmadi.
But as soon as you show that this polynomial can be expressed in terms of the sum of squares, then non-negativity will simply result from this. “The sum of squares gives you a beautiful certificate of positivity,” said Pablo Parrilo , an information technology specialist and an engineer from the Massachusetts Institute of Technology, who participated in dragging the issue of the sum of squares into the applied world.
Knowing that a certain polynomial is always non-negative can seem like a mathematical triviality. But a hundred years after Hilbert asked his question, the non-negativity of polynomials turned out to be the answer to applied problems affecting all of us.
The best way
The sum of squares meets with the real world in the field of optimization. Optimization theory is concerned with finding the best way to do something while within limits — for example, finding the best route to get to work, given the state of the road and the necessary stop you need to make along the way. Such scenarios can often be reduced to polynomials. In such cases, the scenario can be solved or “optimized” by finding the minimum value that the polynomial takes.
Finding the minimum of a polynomial with many variables is a difficult task. There is no simple algorithm from the textbook for calculating the minimum value of complex polynomials, moreover, it is difficult to construct them on a graph.
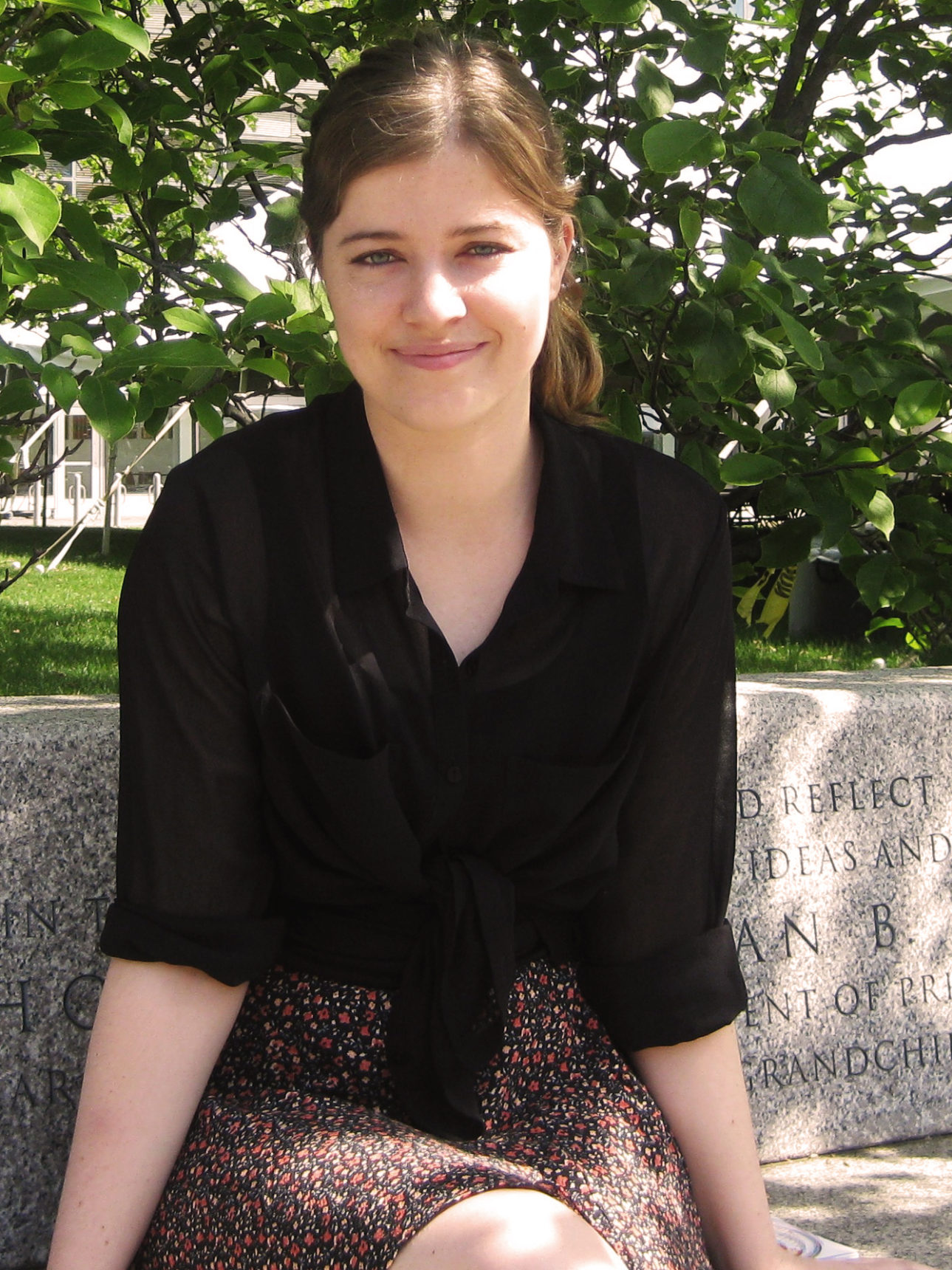
Since the minimum value of a polynomial is difficult to calculate directly, researchers make assumptions about this value by other methods. It is here that the non-negativity comes into play, and the question of whether the polynomial is a sum of squares. “Ensuring non-negativity is the essence of all optimization problems,” said Reha Thomas, a mathematician at the University of Washington.
One way to find the minimum value is to ask the question: what is the maximum value that can be subtracted from a non-negative polynomial so that it does not become negative at some point? To answer the question, it is necessary to check different values - is it possible to subtract 3 so that it does not become negative? And 4? And 5? Repeating the procedure, at each step you need to know whether the polynomial remains non-negative. This is checked through the possibility of its expression in the form of a sum of squares.
“The question to ask is“ Is the polynomial non-negative? ”The problem is that with a large number of variables it is difficult to answer this question, Ahmadi said. “So we use the sum of squares as a replacement for non-negativity.”
As soon as the researchers know the minimum — the optimal value of the polynomial — they can use other methods to determine the input parameters that lead to this value. However, in order for non-negativity to help solve optimization problems, it is necessary to think of a way to quickly calculate whether a polynomial is expressed in terms of a sum of squares. And the fact that the researchers were able to do this, it took 100 years from the time the question was posed by Gilbert.
Breaking problem
Hilbert's 17th problem passed from the world of pure mathematics to the applied plane around 2000th year. It was then that several researchers came up with an algorithm for checking whether we can represent a polynomial in terms of a sum of squares. They have come to this by solving the square problem through " semi-defined programming ", thanks to which computers are able to cope with such a task. This made it possible for researchers from such fields as computer science and engineering to use the possibilities of non-negativity to direct their searches towards the optimal ways of solving problems.
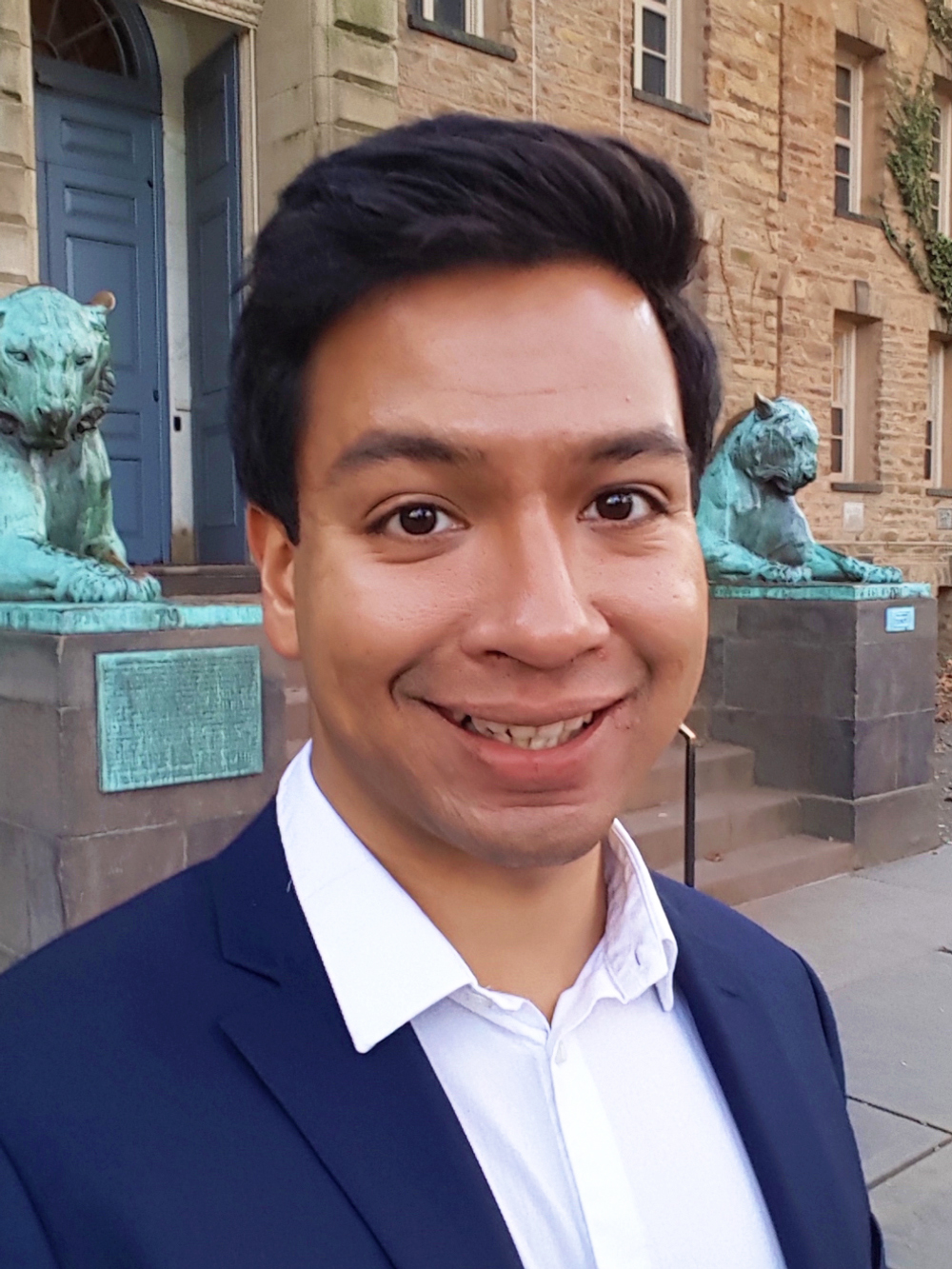
But semi-defined programming has a big limitation: it works slowly on large tasks and is unable to handle some of the most complex polynomials that researchers are particularly interested in. Semi-defined programming can be used to decompose into a sum of squares of such polynomials, which consist of no more than a dozen variables raised to a power of no more than 6. Polynomials describing complex engineering problems — for example, ensuring that the robot remains on its feet — can enter 50 variables, or even more. The program can chew such a polynomial to the end of time, and never give the sum of squares.
In work, published online last June, Ahmadi and Majumdar explain how you can get around the slow work of semi-defined programming. Instead of trying to find the decomposition into a sum of squares with one slow program, they show how you can do this by solving a
sequence of simpler problems that will be much faster to calculate.
These types of tasks are called “linear”, and they were developed in the 1940s to solve optimization problems related to military issues. Linear programs are now well understood and quickly solved. In the new paper, Ahmadi and Majumdar show that many related linear programs can be solved (or, in some cases, a different type of problem, a second-order cone program), and combine the results to get something almost as good as the answer. which a program for semi-defined programming could give. As a result, engineers have a new, practical tool that they can use to test for non-negativity and quickly find a decomposition of the sum of squares.
“We studied several problems of robotics and control theory, and showed that the quality of the solutions obtained is useful for practical use, and that they are much faster to calculate,” said Majumdar.
Safety proof
The speed of the decision is everything if you are in a robility mobile. In such a situation, a polynomial can act as a mathematical barrier installed around obstacles that you don’t want to crash into - if it can be calculated quickly enough.
Imagine a simple example: a robotic car in a giant parking lot. There is nothing in the parking lot except the guard's booth at the far end. Your task is to program the machine so that it never crashes into this booth.
In this case, you can pull the grid on the parking lot. Then make a polynomial, as an input taking points on the grid. Make sure that the values of the polynomial at the location of the car are negative, and the value at the location of the guard’s booth is positive.
On some set of points between your car and the booth the polynomial will go from minus to plus. Since your car can only be where the polynomial is negative, these points will play the role of a wall.
“If we start from a certain place, the robot will not cross the line beyond which there is an obstacle. This gives you a formal proof of collision avoidance, ”said Ahmadi.
Of course, it will be inconvenient if this wall is in the middle of the path between the car and the booth. We need to build a polynomial so that the wall surrounds the obstacle as closely as possible. This option will protect the booth, and give the car a lot of room for movement.
In practice, it is necessary to minimize the value - the distance between the wall and the booth - so you need to shift the graph of the polynomial and see how far you can move it until it goes from minus to plus. This is achieved by checking whether the polynomial remains the sum of squares.
Almost empty parking is one thing. But in realistic scenarios of machine control, its sensors constantly detect new moving obstacles - cars, bicycles, children. Each time a new obstacle appears or a known movement occurs, the machine needs to construct new complex polynomials enclosing these obstacles. This is a lot of checks on the amount of squares that must be performed on the fly.
Seven years ago, another pair of researchers had already imagined that such techniques of working with polynomials might be used to separate robots and those places where they should not go. But at the time, the power of computers made this idea a dream.
The new approach of Ahmadi and Majumdar opens up a new way to carry out such instant calculations. So, if and when robomobils can safely move around the world, we can thank Google and Tesla for this — as well as Hubert.