Four main differences of consumer artificial intelligence from production
- Transfer
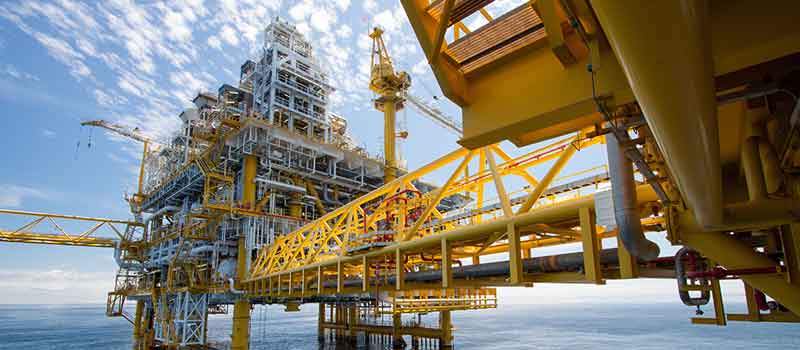
If you are asked to submit artificial intelligence for production and industry, you will most likely first think about robots. Many innovative companies, such as Rethink Robotics , have developed friendly-looking robots for factories, working alongside human colleagues. Industrial robots have historically been designed to perform specific tasks, but modern robots can be taught new tricks, and they are able to make decisions in real time.
And let the robots look sexy and brilliant - but for the most part, AI in production is valued for its ability to take data from sensors and use conventional hardware to turn them into intelligent predictions aimed at improving and accelerating decision-making. Today, about 15 billion machines are connected to the Internet. By 2020, Cisco predicts an increase of this amount to 50 billion. Combining these machines into intelligent automatic cloud systems is the next important step in the evolution of production and industry.

In 2015, General Electric launched the GE Digital program to introduce software innovations in all divisions. Harel Kodesh, Technical Director of the program, told us about the unique difficulties in applying AI to industry, which distinguish this area from the consumer.
1. Industrial data is often inaccurate
“For machine learning to work properly, you need a huge amount of data. Consumer data is harder to misunderstand - if you, for example, buy pizza or click on ads, says Kodesh. “But on the industrial Internet, 40% of the data is false and useless.”
Suppose you need to calculate the depth of drilling, and you stick a humidity sensor into the ground to make important measurements. They can be distorted by extreme temperatures, careless actions of workers, equipment malfunctions, or even a worm accidentally mounted on a device. “Our data does not come from a comfortable and secure computer located in your rest room,” states Kodesh.
2. AI does not work in the cloud, but on the border
User data is processed in the clouds on computing clusters with an apparent infinite capacity. Amazon can quietly rework your browsing and shopping history and make new recommendations. “In user predictions, the cost of false positive and false negative recommendations is low. You will quickly forget that Amazon has recommended a bad book to you, ”Kodesh notes.
And in a deep-water drilling rig, a riser column transfers oil from wells on the seabed to the surface. If a problem arises, several clips blocking the valve should instantly work. Sophisticated software that maintains the actuators of these clips, tracks the minimum changes in temperature and pressure. Any mistake can be a disaster .
The stakes and responsiveness of the system are great in industrial applications when millions of dollars and human lives are at stake. In these cases, you can not trust the work of AI in the clouds, it must be implemented on the ground - sometimes it is called "on the border".
Industrial AI is built as an end-to-end system; Kodesh describes it as a “round trip ticket”. The data appears on the sensors located "on the border", the algorithms process them, then the task is modeled in the cloud, and then transferred back to the border for implementation. Between the border and the cloud are the control nodes and a lot of cumulative nodes, since the system must be able to work under the right load in the right places.
In a factory that processes ore into platinum ingots, it is necessary to instantly monitor the appearance of ingots of irregular density in order to adjust the pressure at the beginning of the chain. Any delay means a loss of material. Similarly, a windmill constantly processes data to control work. Kodesh gives an example of one of many probable problems: “the millionth byte may contain information about the torque of the blade, but if it is too large, the blade will break off. Such critical information needs to be processed first, even if it is located on the millionth place in the queue. ”
Delivering correct, real-time data is so difficult that GE has to rely on customized solutions developed internally. “Spark is fast,” admits Kodesh, “but when you need to make decisions in 10 milliseconds, you need other systems.”
3. The only prediction can cost you $ 1000.
Despite the large share of spurious data and limited processing power at the border, industrial AI needs to be extremely accurate. If the analytical system of the aircraft determines that a problem has appeared in the engine, it is necessary to call technicians and engineers so that they remove and repair the faulty part. Airlines need to provide a temporary replacement so that it can continue flights. And this whole undertaking can easily cost $ 200,000.
“We will not inform you about the existence of a problem if there is none, and we definitely will not inform you about the absence of problems if there is a problem,” Kodesh says. “We want to make sure the system has high accuracy.”
According to Kodesh, the only way to ensure high accuracy and speed of the system is to run thousands of algorithms at the same time. A consumer company like Amazon can earn from $ 1 to $ 9 per book, so they may be willing to spend $ 0.001 on a prediction. And when thousands of dollars are at stake, industrial and industrial giants spend from $ 40 to $ 1000 to predict the amount.
“For $ 1,000, I can run multiple algorithms in parallel, collect results, and run a genetic algorithm to generate a prediction,” Kodesh says. “This will create the survival effect of the strongest, when the most adjusted predictions are used and the less appropriate are discarded.”
4. Complex models must be interpretable.
Users rarely think about why Amazon gives a specific recommendation. When the stakes are high, people start asking questions. Technicians working in this field for 45 years will not trust machines that cannot explain their predictions.
To achieve the level of high interpretability of GE, it is required to invent completely new technologies. Unfortunately, the required talent is extremely small. “I admire educational institutions trying to satisfy market demands with new data processing specialists, but their mathematics is not deep enough,” complains Kodesh.
“Real professionals need to go deeper. They need to possess outstanding analytical skills, and know how to filter and normalize millions of measuring points in real time. ”