Machine control. First business experience
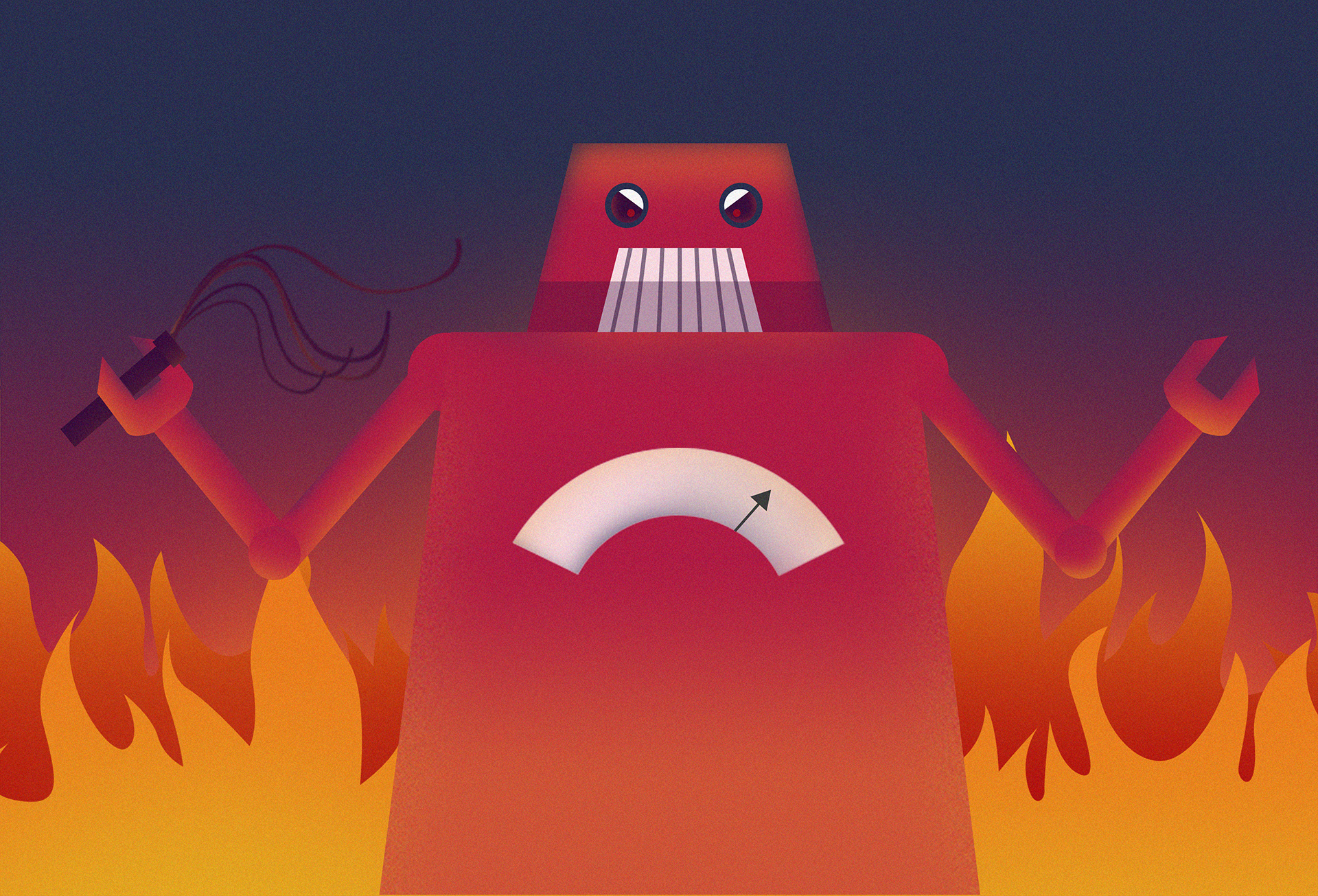
The outgoing 2016 will be remembered by the abundance of bright news about the applied applications of machine learning almost everywhere. Like parents watching the first awkward steps of the children, you and I had the opportunity to witness the first timid attempts at weak artificial intelligence to read, write novels and ... even make trailers for films! Summing up this busy year, Phobos is pleased to add an application to project management to the piggy bank of unusual machine learning applications.
When the world watched with enchantment for the victories of artificial intelligence systems in logic games and the first cognitive successes of Google’s networks in the year 15, our team was already interested in the application of existing technologies for winning management games. Now the car is checkmate to the grandmaster. So, one day, checkmate the ineffectiveness of team management. More than a year ago we decided that we were ready to work in order to bring this moment closer. Then we still did not understand where this would lead.
This material will tell about milestones in solving this problem practically from scratch:
- Formulation of the problem;
- Model formalization;
- Definition of input and output data;
- Training and validation;
- Testing.
Formulation of the problem. The birth of "skyneta"
The purpose of the article is the presentation of the overall picture of the solution, including its architecture, the main software used, and also the description of the most interesting non-standard solutions without losing the overall picture of the system.
In case of demand, we will be happy to publish more detailed descriptions of the stages of interest in separate articles.
The task of the machine learning department by our manager, Alexey Spassky, was a specific one - to implement a system for automatically maximizing the efficiency of the team. The guys called her "skyline".
It was necessary to implement a “virtual manager” who knows how to distribute tasks in our YouTrack tracking system and respond to their status, type, priority and time. Yes, so that the efficiency of employees increased.
The main solution we chose Q-learning is related to the class of learning algorithms with reinforcement. With this approach, an intelligent agent (in this case, our manager, "SkyNet"), interacting with an unfamiliar environment, will learn to perform actions that will bring the greatest reward with feedback from the environment. It is by this method that intelligent agents are trained to play simple games better than humans. The agent performs actions: at first chaotic, at each step in response to actions receives a new state of the environment and a numerical assessment of actions - a whip, if the points are less than zero, and a carrot, if more. By making numerical conclusions from rewards and punishments, the agent learns to choose the actions most likely leading to the most rewarding states of the environment.
A model of a generating prediction of agent's actions (his brain) was chosen as a multi-layer perceptron or fully connected artificially-forward artificial neural network. Why choose Q-learning based on deep learning? This is the best that we could find for solving a multiparameter problem in a game setting.
Formalization of the model. The first virtual manager
The sophisticated reader has probably already noticed that much of what was said is intentionally oversimplified. Let's simplify even more to understand our model.
So, to the input of the “brain” of artificial neurons of our manager “skyNet” we submit statistics from the tracking system according to his project and performers. He fights in the ecstasy of awareness and speaks at the output as if he were in this situation with the project task. Now we need to give a numerical evaluation of his actions, so that the method of back-propagating the error across all layers of his multilayer perceptron he can draw conclusions where he was a little wrong. In the case of a game, the numerical evaluation of the actions or chains of the agent’s actions are game points, which are calculated by goals, coins, or hits on a target. In our case, for evaluation it is necessary to have a formalized business logic. In other words, it would be nice to have an “absolute formula of perfect control” for machine learning of the winner of the control games. We did not have it then. But there was and is the wisdom of our leaders, which we formalized in a semi-empirical model. The formalization of business processes and the development of appropriate metrics took months of discussions. In it, we wanted to express precisely the objective approach to management, devoid of any bias and emotional distortions typical of all people without exception. Please note that when formalizing business logic, psychological management models were not taken into account.
Discarding the philosophy, we summarize that we would like our algorithm to be able to find the minimum of inefficiency in the space of control actions for each of the employees. But it’s not enough to create an inefficiency formula, you need to find its minimum.
Training models implemented through mini-batch gradient descent. It is not uncommon that in machine learning, the loss function (in our case, the inefficiency function) can have many local minima. What kind of gradient descent in the search for the bottom will ultimately determine the “management style” of our SkyNet manager, or the specifics of the interaction between an intelligent agent and the environment.
Some logic was laid into the model in a more explicit form. For example, thanks to our manager Lyoshe, the Eisenhower method was used to prioritize tasks. The importance and urgency of the task we were taught to determine another module of the system based on a multilayer perceptron trained with a teacher. Training took place on the data marked by managers. Urgent and important tasks have the first priority, non-urgent and unimportant - the last. We set a certain threshold of priority, after which the system writes messages to the chat. Using telegram api, it informs about critical tasks.
Definition of input and output data. Cash game
The main implementation language is python. The framework for the implementation of machine learning - TensorFlow, which eventually began to support not only the implementation of networks, but also Q-learning and serving. We received and sent input data via the YouTrack REST API, youtrack-rest-python-library, as well as api messengers and mailers for which there were also libraries in python.
As a result, at the entrance:
- Was installed tracking system YouTrack. Discipline on the reports on the tasks done has been tightened - now it was necessary to report on every task, every little thing. We needed complete statistics. Also responsible for the execution of tasks now had to put marks performers. Was considered every minute of overdue tasks or tasks ahead of the curve. Task execution time is a very influential parameter in evaluating performance;
- Received data on the creation of sprints for the week;
- The work time of employees was measured by the activity of working sessions on machines.
At the exit:
- Control signals to the task setting system: assignment and reassignment of tasks from those already created to the performer; appointment / reassignment of the responsible; setting deadlines; creating sprints for the week.
One of the important management actions was the coefficient of salary adjustment by which at the end of the month the base salary is multiplied. The fall of the coefficient below the predetermined threshold meant dismissal. In this case, the notification was sent; - Messages of special importance with the help of api telegram were broadcast in a general chat. The "importance" of tasks is one of the key parameters associated with efficiency.

Training and validation. Intelligent Undercover Agent
Each project is a separate game environment for interaction with other agents (live project performers). In order to train an intelligent agent to play the game, it is necessary to understand what kind of new game state will transfer us agent actions. In case we train a robot, we can simulate its mechanics and train it on a mat model. But, we want to learn to play with the team, and a person, not that the team, has been successfully simulated to anyone, otherwise it would be ... Therefore, in our case, it was possible to learn only through real interaction with a non-virtual environment. But, in order to exclude “quantum effects” - a change in the observable under the influence of the observer, the agent received random tasks for training and no one knew in advance that artificial or natural intelligence was now leading his task. A kind of secret Santa.
In other words, the agent must know how humans behave in their natural environment, otherwise they will not avoid cheating. First of all, they can say that the task for the day, and the business for 30 minutes. The estimation of the time of the task, in contrast to the “urgency” and “importance” in a separate module, was not carried out. Validation was also carried out on a random sample of tasks with the measurement of efficiency metrics, then the tasks were again chosen randomly and the efficiency value was averaged over all samples.
Testing. Doubtful experiment
One morning we woke up with the knowledge that a big brother, specially trained by us, would manage us for a month. Our work will govern our work. Responses to the subjects and test results can be found in the article "How we put the team under the control of artificial intelligence for a month." Conclusions about the timeliness and success of the experiment as a whole are suggested to be made by readers independently. The graph shows the test results in hours.
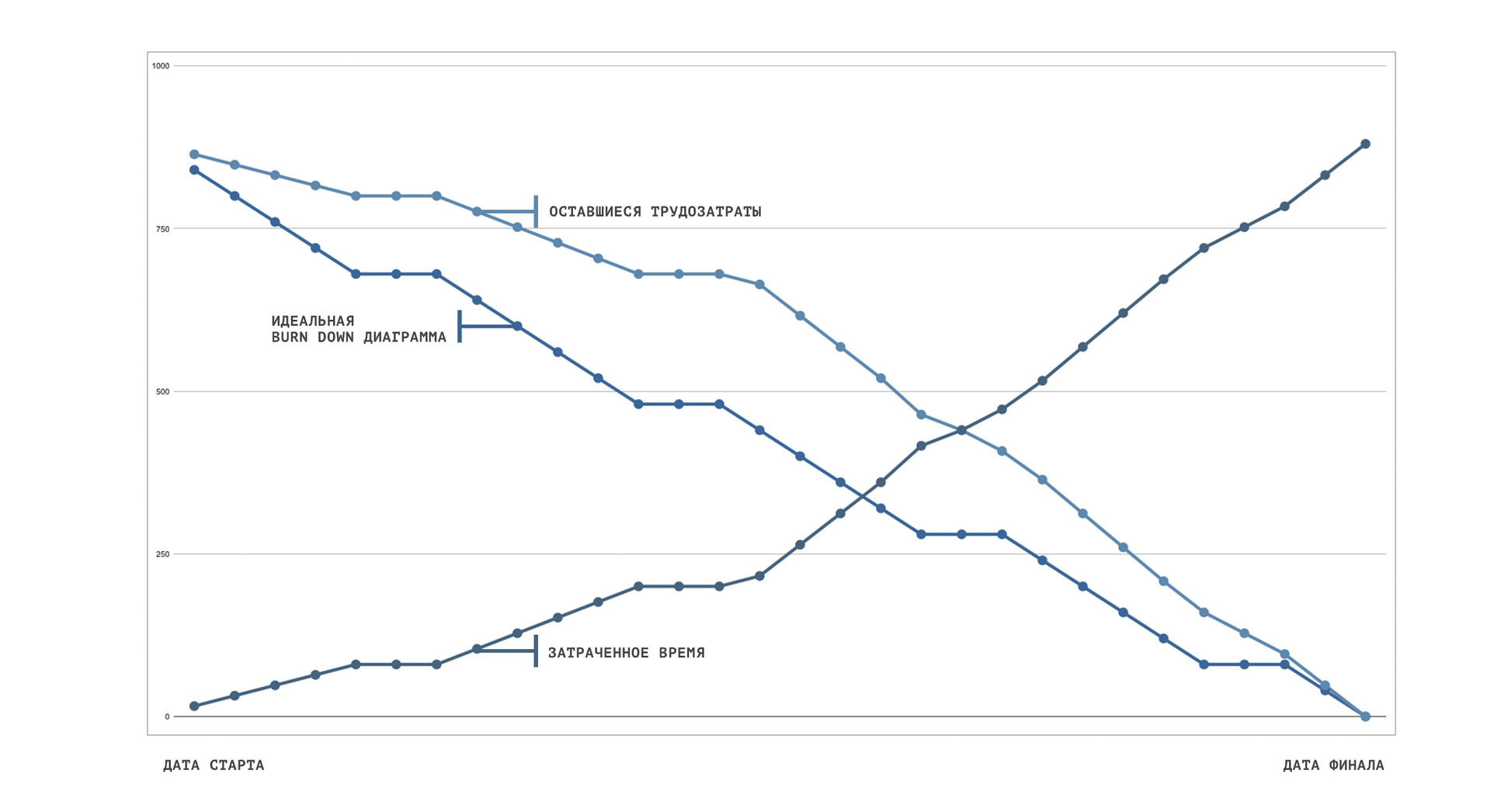