
The neural network has learned to age and rejuvenate people from photos
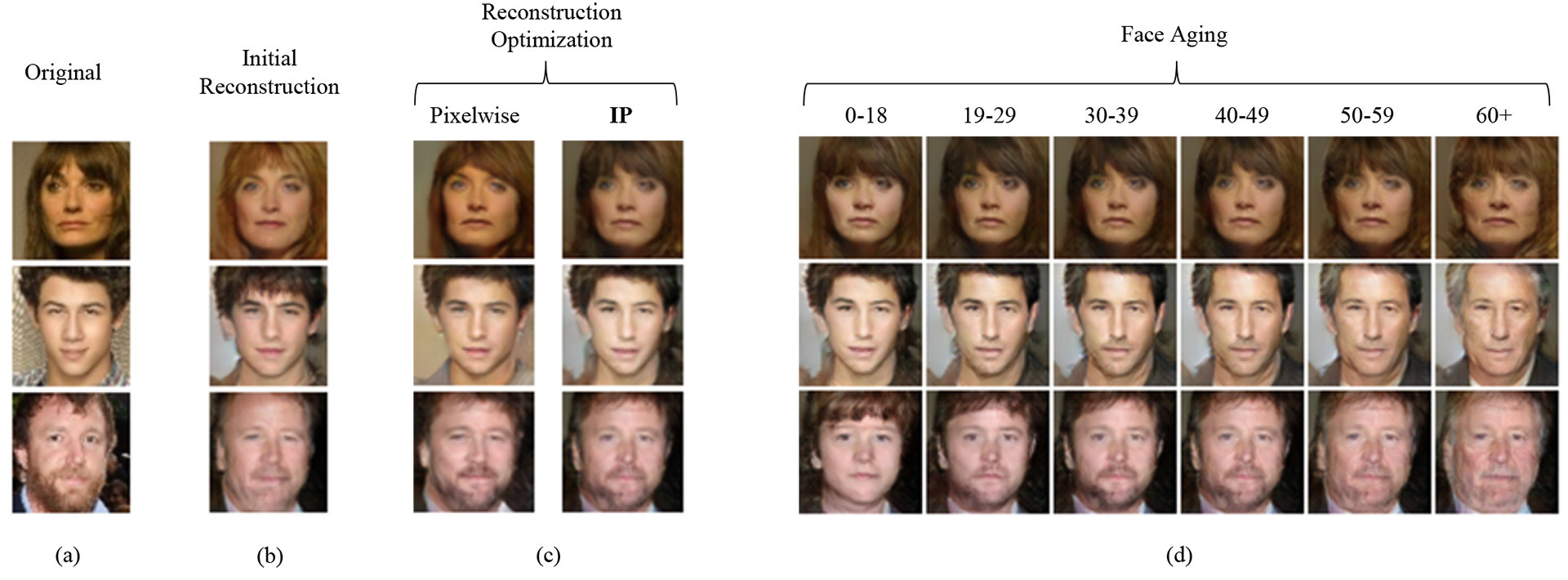
Original photo (a), initial reconstruction (b), two options for optimizing reconstruction (c) and the result of aging / rejuvenating faces with the second option for optimizing IP, that is, with better recognition of the face (d)
Aging of the face (age synthesis) with morphing photos - an important task that has many practical applications. Such aging is necessary for the correct operation of face recognition systems. It is needed when searching for missing children years or decades after the disappearance. And of course, face morphing is used in the entertainment industry - for example, in cinema. Mobile applications with this feature are likely to become popular. Everyone is interested to see how this old teacher appeared in his youth or what your beautiful classmate will look like in 40-50 years.
Traditionally, facial aging programs are based on one of two approaches: prototyping and modeling . In the first case, the estimate of the average person in each age group is calculated, as well as the difference between the average persons in different age groups. This difference is then used to morph a particular face. Prototyping is a simple and quick approach, but it completely ignores the individual traits of a person. Therefore, the images are not very realistic.
In contrast, modeling involves drawing up a parametric model that simulates the mechanisms of aging on the muscles, skin and skull of a specific individual. This approach also has a drawback. To create a good model, you often need photographs of the same person at different ages. Obtaining such data is not always possible.
Both of these approaches do not cope well with additional modifications that occur with an aging face. For example, the appearance of a beard, bald head or glasses.
The methods of natural image generation have been studied for many decades, but they received a significant impetus after 2014, as a result of serious progress in deep learning. Neural networks have learned to generate images of very high quality and resolution. The key technology in this area is Generative Adversarial Network (GAN), which have been repeatedly described on Habré and GT . GAN teaches the loss function, whose task is to classify the image as “real” or “fake”. A generative model is being trained at the same time to minimize this function.
Unlike auto encoders, a GAN cannot generate a blurry image because it will not pass the classification check as “real”. GAN is a kind of “war” of two neural networks, a generator and a discriminator, where the first one tries to trick the second one, to generate a photo that will pass for the real one at the discriminator.
Generative adversarial neural networks proved to be excellent in various programs for changing the face - for example, in applying hairstyles and changing hair color or in a simple change in a person’s age without clear age criteria. But in most cases, these programs had a problem that, along with aging, the characteristic individual features inherent in a particular person disappeared on the face. This problem was tried to solve by the staff of the research team led by Grigory Antipov from Orange Labs (France). Their scientific article was published on February 7, 2017 on the preprint site arXiv.org (arXiv: 1702.01983).
Antipov and his colleagues developed an acGAN (Age Conditional Generative Adversarial Network) generative adversarial neural network, trained to age or rejuvenate a person withrecognition recognition . This is the first neural network that generates high-quality faces in any given age category. In addition, the neural network can restore the original photo while maintaining human recognition.
The authors write that after training the neural network, aging occurs in two steps. At the first step, the optimal eigenvector for reconstruction is calculated. At the second stage, the image generator is turned on, which simply creates a photograph of a specified age, taking into account its own vector.
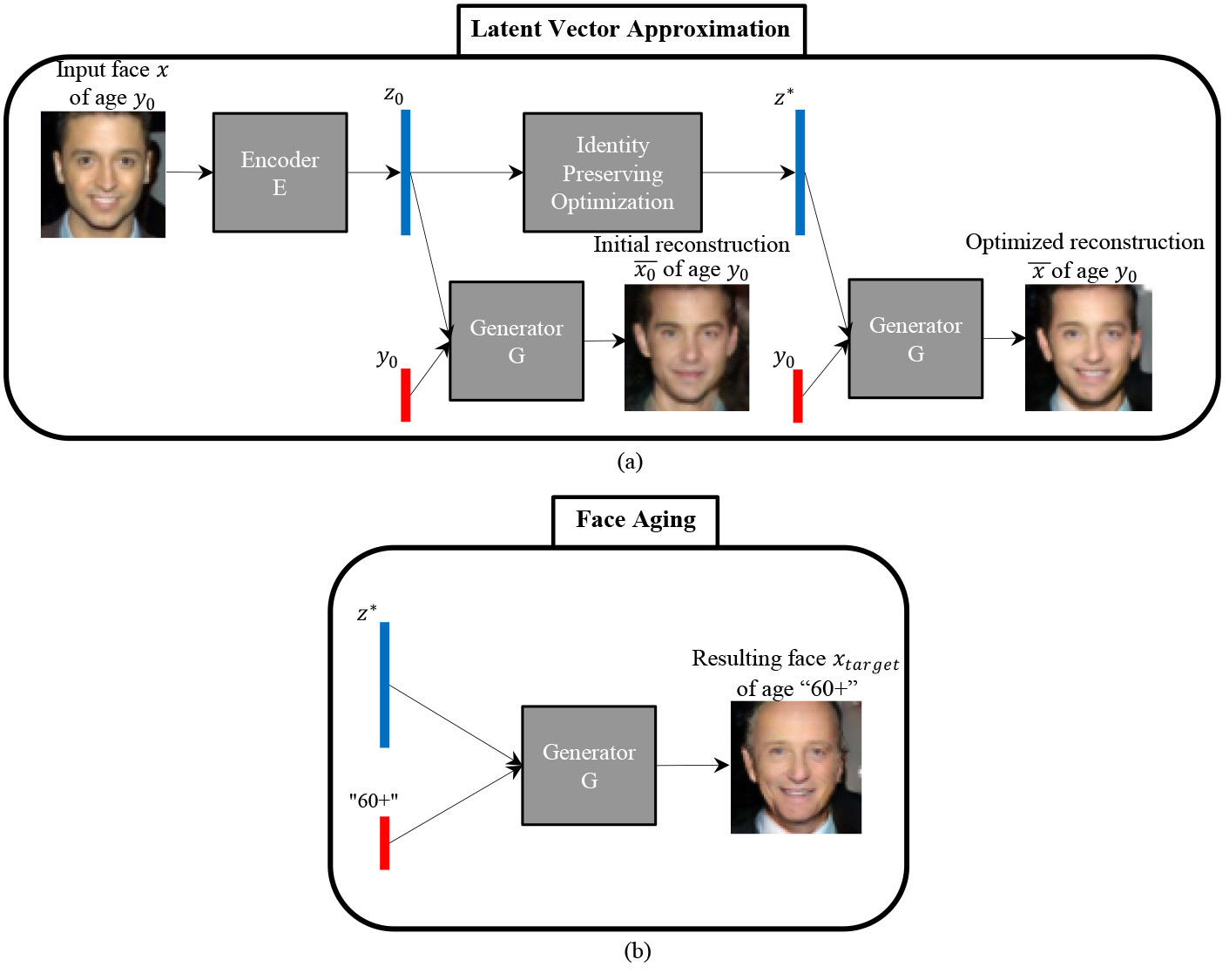
AcGAN Generative Competitive Neural Network Operation Algorithm
Six age categories were established for the conditions of the acGAN generator: 18 years old, 19-29, 30-39, 40-49, 50-59 and more than 60 years. The neural network was trained on the IMDB-Wiki cleaned photo set containing a minimum of 5000 images in each age category. Thus, acGAN conditions are six-dimensional straight vectors.
In total, there were 120 thousand photographs in the training kit. Of these, 110 thousand were used for training, and the remaining 10 thousand were used to test the operation of the neural network using two alternative methods for calculating the optimal eigenvectors Pixelwise and Identity-Preserving .
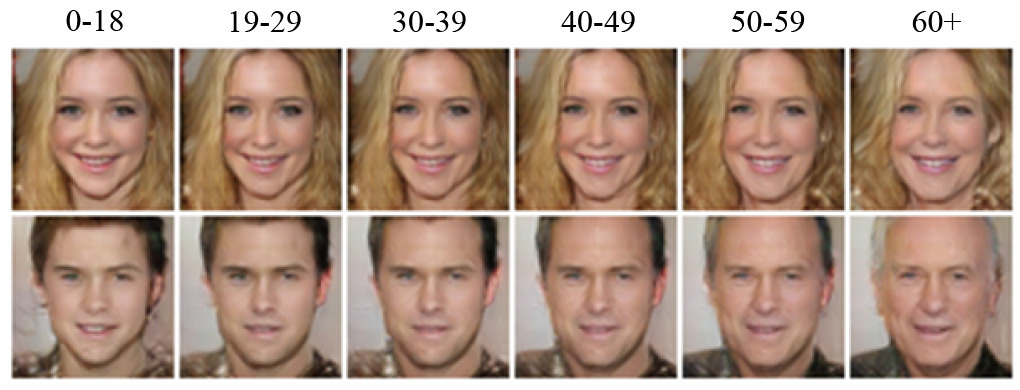
Examples of generating synthetic images in six age categories using two random optimal eigenvectors
A comparison of the results of the Pixelwise and Identity-Preserving methods showed that the second of these methods shows the most optimal results. Face recognition was assessed using OpenFace software , one of the best open source face recognition software.
The authors of the scientific work believe that the innovative Identity-Preserving method is suitable not only for aging people, but also for other face transformations while maintaining recognition. For example, for applying a beard, glasses and other transformations. This technology can be applied in various face recognition systems and entertainment applications.