
Data Labeling Specialist
Today is a wonderful day (if you know what I mean) to announce our new program - Data Labeling Specialist .
Currently, the situation in the field of artificial intelligence is such that in order to train a strong neural network, several components are needed: hardware, software and, directly, data. Lots of data.
Iron, in general, is accessible to everyone through the clouds. Yes, it can be expensive, but GPUs on EC2 are affordable for most researchers. The software is open source, most frameworks can be downloaded somewhere and work with them. Some are harder, some are easier. But the threshold for entry is quite acceptable. Only the last component remains - this is the data. And here the snag arises.
Deep learning requires really big data: hundreds of thousands – millions of objects. If you want to deal, for example, with the task of classifying images, then, in addition to the data itself, you need to transmit information to the neuron about which class an object belongs to. If your task is also related to image segmentation, then getting a good dataset is already fantastically difficult. Imagine that you need to highlight the boundaries of each object in each image.

In this post, I would like to review those tools (commercial and free) that are trying to make life easier for these wonderful people - data markers.
To begin with, this is a free tool made at MIT . With it, you can mark up your images: it can be just bounding boxes, or pixel-by-pixel segmentation.

In fact, this is a kind of UI in which you can highlight the contours of the image and put dots. It's all. This tool does not know anything smarter. Another feature: LabelMe has a mobile application. You can not waste time in the subway, train, bus, at a boring lecture.
One of the most advanced active learning systems . The idea is that a pre-trained model with minimal training is trying to mark your data, and your task is only to direct it. The target audience is analysts and engineers who need to lay out data qualitatively, and they do not have large resources for external markers. UX, according to the developers, is similar to Tinder.

Tulsa asks to mark only those objects for which she is not sure. It seems like they put more emphasis on working with texts, but they also have computer vision, including working with video. We ourselves did not use it. She's paid. The cost of a license starts at $ 390.
These guys approach the turnkey formatting process. Give us your data, we will give it to our scribblers, we will control the quality, we will give you the result after some time. And all this through the API.
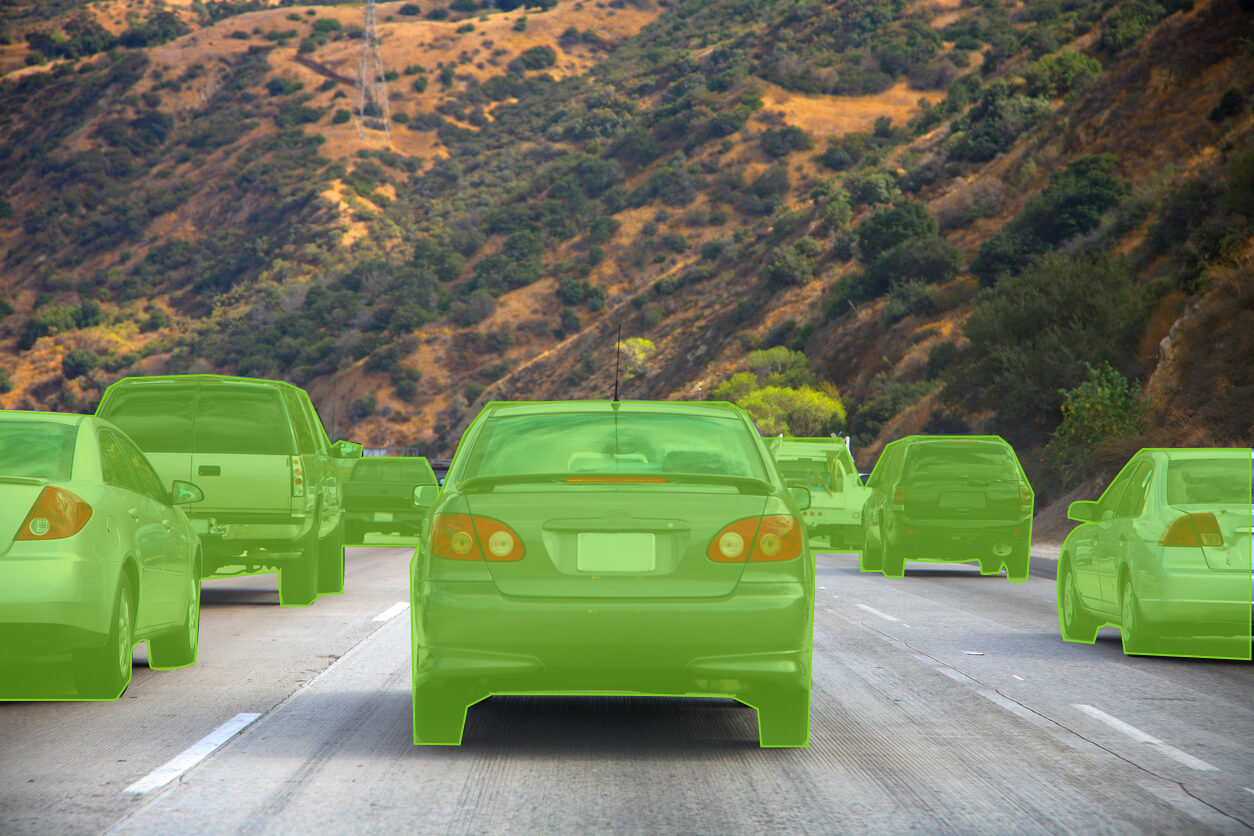
Naturally, this is also not a free tool. For example, marking up one picture for the semantic segmentation task (that is, select objects on the image with contours and say what kind of objects they are) will cost $ 8 if you need it urgently, or $ 6.4 if you are ready to wait.
This tool is intended to simplify markup of type instance segmentation. Under the hood (as it feels) something like the Polygon-RNN works. You select objects with rectangles, and the system itself finds the boundaries of the object inside the rectangle. They have different trained grids for different subject areas.

The guys still know how to generate synthetic data from games and dilute real ones with them, if real ones are hard to get. Plus, they can get their entire system inside your enterprise, so that the data does not go away from you. In general, it feels like it can accelerate the work of the scribbler well. But it is not exactly.
The power of Hindu marking at your fingertips. Expensive for you, a penny for them, poor quality, incomprehensible quality control, but everyone uses it. There is an analogue in Russia - Yandex.Toloka .

Someday we will interview users of these platforms and find out how their work day is going, and what difficulties arise.
This tool is the de facto standard for markup. They also use living people, but provide them with more advanced tools than Toloka or MTurk, to make marking easier.

In addition to standard bounding boxes, semantic segmentation, polygons, they also mark points, for example, for warehouses or shelves in stores.
As you can see, the market for such solutions is still very narrow, but the potential is quite large, because the AI bottleneck now is precisely the well-marked data. And in addition to jokes, this is really the future.
If you know other tools, write in the comments.
Currently, the situation in the field of artificial intelligence is such that in order to train a strong neural network, several components are needed: hardware, software and, directly, data. Lots of data.
Iron, in general, is accessible to everyone through the clouds. Yes, it can be expensive, but GPUs on EC2 are affordable for most researchers. The software is open source, most frameworks can be downloaded somewhere and work with them. Some are harder, some are easier. But the threshold for entry is quite acceptable. Only the last component remains - this is the data. And here the snag arises.
Deep learning requires really big data: hundreds of thousands – millions of objects. If you want to deal, for example, with the task of classifying images, then, in addition to the data itself, you need to transmit information to the neuron about which class an object belongs to. If your task is also related to image segmentation, then getting a good dataset is already fantastically difficult. Imagine that you need to highlight the boundaries of each object in each image.

In this post, I would like to review those tools (commercial and free) that are trying to make life easier for these wonderful people - data markers.
Labelme
To begin with, this is a free tool made at MIT . With it, you can mark up your images: it can be just bounding boxes, or pixel-by-pixel segmentation.

In fact, this is a kind of UI in which you can highlight the contours of the image and put dots. It's all. This tool does not know anything smarter. Another feature: LabelMe has a mobile application. You can not waste time in the subway, train, bus, at a boring lecture.
Prodi.gy
One of the most advanced active learning systems . The idea is that a pre-trained model with minimal training is trying to mark your data, and your task is only to direct it. The target audience is analysts and engineers who need to lay out data qualitatively, and they do not have large resources for external markers. UX, according to the developers, is similar to Tinder.

Tulsa asks to mark only those objects for which she is not sure. It seems like they put more emphasis on working with texts, but they also have computer vision, including working with video. We ourselves did not use it. She's paid. The cost of a license starts at $ 390.
Scale API
These guys approach the turnkey formatting process. Give us your data, we will give it to our scribblers, we will control the quality, we will give you the result after some time. And all this through the API.
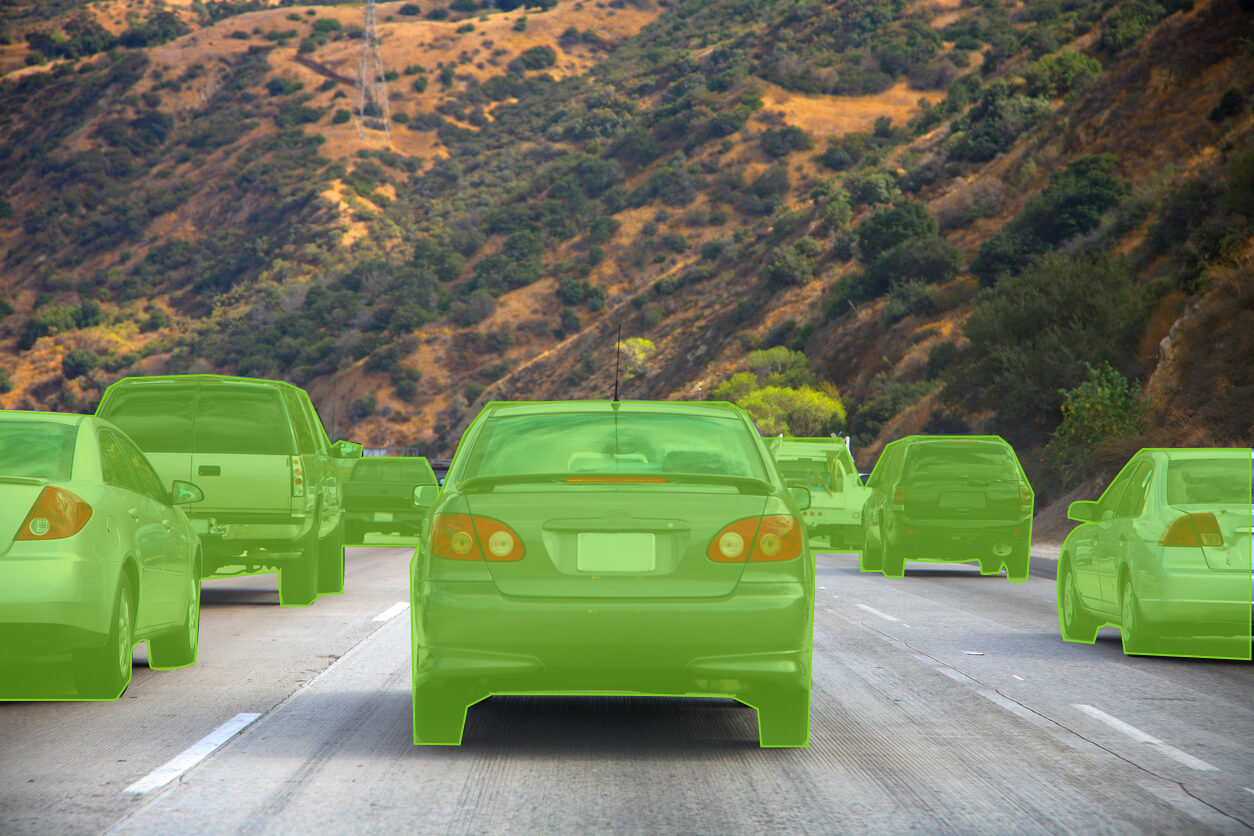
Naturally, this is also not a free tool. For example, marking up one picture for the semantic segmentation task (that is, select objects on the image with contours and say what kind of objects they are) will cost $ 8 if you need it urgently, or $ 6.4 if you are ready to wait.
Supervise.ly
This tool is intended to simplify markup of type instance segmentation. Under the hood (as it feels) something like the Polygon-RNN works. You select objects with rectangles, and the system itself finds the boundaries of the object inside the rectangle. They have different trained grids for different subject areas.

The guys still know how to generate synthetic data from games and dilute real ones with them, if real ones are hard to get. Plus, they can get their entire system inside your enterprise, so that the data does not go away from you. In general, it feels like it can accelerate the work of the scribbler well. But it is not exactly.
Mechanical Turk
The power of Hindu marking at your fingertips. Expensive for you, a penny for them, poor quality, incomprehensible quality control, but everyone uses it. There is an analogue in Russia - Yandex.Toloka .

Someday we will interview users of these platforms and find out how their work day is going, and what difficulties arise.
Crowdflower
This tool is the de facto standard for markup. They also use living people, but provide them with more advanced tools than Toloka or MTurk, to make marking easier.

In addition to standard bounding boxes, semantic segmentation, polygons, they also mark points, for example, for warehouses or shelves in stores.
As you can see, the market for such solutions is still very narrow, but the potential is quite large, because the AI bottleneck now is precisely the well-marked data. And in addition to jokes, this is really the future.
If you know other tools, write in the comments.