Can Big Data and AI solve the global water crisis?
- Transfer
There is nothing more important for life on Earth than water.
Throughout the year, almost 663 million people around the world do not have safe access to clean water. The problem of climate change is likely to only worsen the situation, and finding solutions to less economically developed countries is a priority. New technologies such as Big Data (Big Data) and AI can help find a way out ...
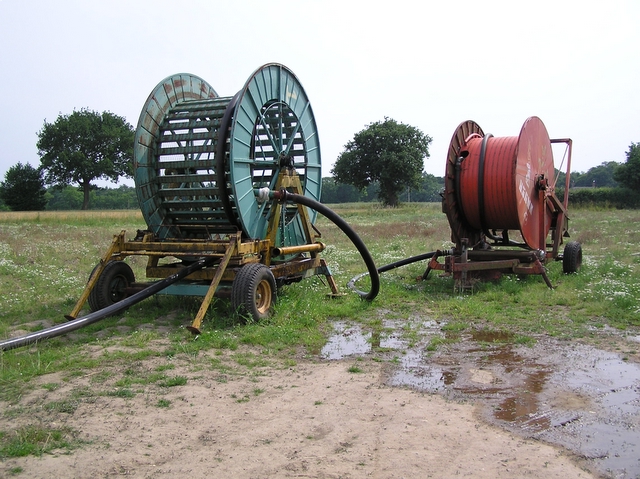
Katy Walters / Water, water everywhere / [CC BY-SA 2.0 ]
Big data is the analysis of a vast array of information with tools that can process them much faster than people can do it without technical support.
The acquisition and accumulation of data has increased in volume in recent years due to cheap sensors and increased application of geospatial analysis. These new technologies have improved our ability to find and control water supplies. Moreover, the infrastructure provided by modern sensors creates opportunities for cloud computing and the increased availability of data on all systems.
Agriculture
Agriculture is unambiguously the largest user (and waster) of water in the world. Farmers use 70% of the world's freshwater supply, but 60% of it is lost as a result of leaks in irrigation plants and unsustainable uses.
Big data analysis can continue to search for optimal solutions to balance performance and reliability when it comes to agriculture. It can also prevent human-induced accidents, such as a sudden drop in water quality, which may remain hidden until the consequences are manifested.
This can help water supply companies understand land use and climate trends that will affect key decisions when planning adaptive and regulated water systems.
Big data and modeling help to work together with water supply companies and land surveyors in assessing how much water will be needed and available for various development options.
Water rastrum
In the twentieth century, the world's population has tripled, while human use of water has increased sixfold.
To this day, water supply companies were in a deadlock with regard to time and resources. Their water and wastewater infrastructure is deteriorating, the pumps are broken, the pipes are leaking, and other parts have expired, but the enterprises that are constrained in funds have no money or knowledge of the infrastructure to make the necessary improvements.
Immediate data issue
In fact, big data means having a huge amount of data. Water supply companies obtain data through supervisory control and data collection systems (SCADA), including flow statistics, online monitoring, etc.
Dispatcher Management and Data Acquisition (SCADA) is software that uses computers, local data transmission networks and a graphical user interface for organizing high-level monitoring and control.
Businesses are already using SCADA systems , which allows them to collect huge amounts of data. However, it often turns out that they do not know or don’t care how to make this data bring concrete benefits.
Their SCADA systems can be old, produce peculiar data formats and do not necessarily have to be created for collaboration (separation).
In addition, data collected in wastewater treatment plants are often fragmentary. There is disunity in these computer systems, which are not always in contact with each other. Developing in big data and new data management tools allow us to turn all this data into understandable, useful information that helps us become more prudent and make better business decisions.
Moreover, employees of enterprises who have this type of information in their hands are more likely to be able to identify in advance potential problems before they happen, and not rush to repair something like a broken pump . SCADA systems are capable of displaying the current situation in real time and immediately signaling problems. The ability to predict probable problems using smart platforms for processing and analyzing data fundamentally changes the state of affairs.
The next step - combining data and using analytical processing tools to predict where we should send our view to become more far-sighted - is extremely important for water management.
Focus on quality, not quantity.
Even the most subtly organized analytical data processing cannot avoid measurement errors. If you are not sure about your main sensors and analyzers, you will have a huge amount of incorrect data that is useless.
How it works
Data mining (note of a translator: there are several translations of this term, this article will use “data extraction”) - this is how a specialist working with big data discovers information in the raw data stream. Incentives and benefits on both sides — utility providers and consumers — can then be synchronized using mathematical models, such as models based on Bayesian inference and game theory. Communication knowledge derived from big data finally spreads so that operators, engineers, and managers can use them.
There is no shortage of raw data. Nearly 60% of water supply companies have remote data collection systems at all pumping stations, and 43% have data collection at all tanks.
The advantages of big data:
- Advanced trend analysis
High-performance big data (enormously huge datasets) have the potential to create smart management of water infrastructure resources, providing managers with the ability to correctly, accurately, evaluate, predict, and distribute their resources. Water companies can be helped by trend analysis, which, when making predictions for the future, is based on analytical methods to determine hidden patterns and trends underlying the old data.
- Forecast of demand
Advanced big data analysis makes forecasting the system workload feasible for high-level executives by recognizing patterns and modeling a number of scenarios using a dynamic modeling system and advanced machine learning algorithms. Improved system load forecasting for predicting behavior with water consumption using large data in several data sets, such as demographic factors (population density, etc.), patterns of consumption over past periods, climate (temperature, humidity, etc.). ), infrastructure (technologies used, age, productivity, etc.), political, economic and other criteria. These components are input variables for the development of a predictive model,
- Automated control.
What if instead of sending signals to a team of engineers, could these SCADA systems send self-tuning commands? Let's imagine something like self-regulating technologies that help us regulate water.
- Open data
Some other areas in which data integration drives innovation are open data and civic sciences. The flip side of the fact that utilities do not work in a competitive environment is the opportunity to create the conditions for innovation for others. The data sets collected by enterprises may become, and in some cases already become, for the third party, as open data.
How to apply AI
AI is a highly scalable and cost-effective solution for a large number of water pipes owned by utility companies. In addition to data integration, AI also improves decision-making by providing recommendations based on this data.
Software with AI elements based on machine learning for pipe condition assessment is a better development strategy than just robotization. The AI can analyze thousands of miles [tubes] in a matter of hours, becoming an extremely cost effective solution.
Machine learning is the best way to find meaningful relationships within the data, and then derive a functional relationship that can be used to make decisions.
For example, forecasting models were designed to allow utilities to predict demand with an accuracy of up to 98%. These models use the collected data, combine with other data, such as the weather forecast, which are then transmitted to the machine learning model in external applications.
While other industries make extensive use of trend analysis and forecasting, their key importance remains a mystery for highly fragmented water management.
Service providers and utilities should invest in the organization of appropriate data collection systems for collecting, grouping, and analyzing trends in micro- and macro-data as a first step towards optimizing infrastructure management and decision-making in water management.
Some startups develop water management solutions based on deep learning. Companies promise "to provide an opportunity to prevent water leaks in water supply systems, to predict the overall state of the system and minimize operating costs." They can offer data with time stamps from sensors and counters, thanks to the use of the most advanced deep learning algorithm for analyzing them.
In India, two INS models were developed to determine the quality of water in the Gomti River. Water quality parameters such as acidity (pH), total solids, chemical oxygen consumption were taken as a data set, and a preliminary calculation of oxygen dissolved in water and biological oxygen demand was made.
Artificial Neural Network (ANN) is a computational model based on the structure and functioning of biological neural networks.
A prototype of the neural network was developed using data that contained observations over three years. The input datasets were calculated using the correlation coefficient with dissolved oxygen. The INS prototype calculations were compared using the correlation coefficient, the mean square error and the efficiency coefficient. Estimated values of oxygen dissolved in water and biological oxygen demand coincided.
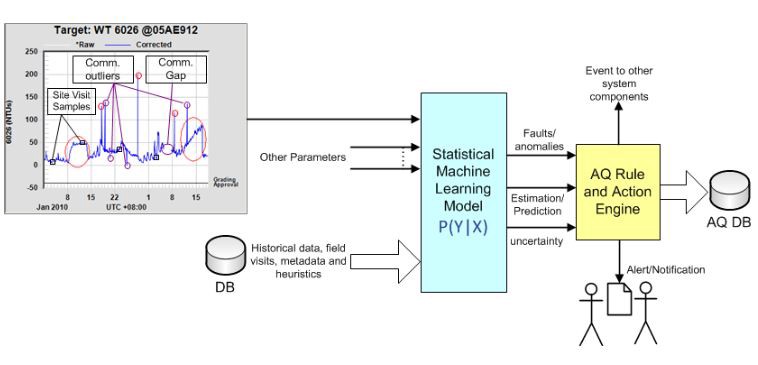
An example of the processing of data from the pipeline
Specific examples
In Bangalore, water companies can measure consumption at any time and make access to water as equitable as possible. Watching a single control panel, it is possible to monitor the work of more than 250 water meters, as well as pay more attention to individual blocks.
In the state of Kerala [India], companies rely on IBM's water meters and sensors to monitor water consumption, including irregularities that may indicate individual incidents of unauthorized use. The advantage of big data processing and analysis platforms is that they can look for deviations in patterns that might otherwise remain undetected.
Finally, Google agreed with several countries to develop an AI model for flood forecasting.

ИИ показывает, что доступ к чистой воде вновь является ведущим приоритетом в мире. Группа SXSW (South by Southwest — создание фильмов и организация конференций) сформировала «коллективный разум» с хэштегом @единодушиеИИ, чтобы представить оптимальные первоочередные задачи для глобальных целей ООН.
The future of data analysis
Since we are entering the era of big data, water supply companies will be able to use advanced sensors that will capture previously undetected changes in infrastructure performance. These forecasting technologies will help companies anticipate equipment problems and leaks.
Smart technologies can help water supply companies improve their customer service. For example, an information and analytical system with a self-service function using an advanced method of recording and analyzing water quality data could allow users to monitor and optimize their own water consumption.
The new wave of technically advanced analytics tools offers water supply companies the opportunity to meet these immediate needs and transform raw data into practical information. Data analysis can quickly identify a malfunction in the infrastructure, reduce water loss, prevent overflow in drains and assess the status of the system. Moreover, data can reveal performance, provide information on proactive maintenance cases, and provide guidance in long-term planning.
While most people talk about big data as replacing physical assets with digital technologies, a more significant and influential trend is the use of online tools to improve the efficiency of the use of physical assets in “offline” enterprises, such as water management. In this context, the role of the data does not make the manager smart to talk. Their task is to help make the best decisions. And you can’t do it only with technology or with data analysis, no matter how cool you are.
Data and water are truly compatible.
About the author of the article . Alexandre Gonfalonieri writes in her blog about the use of artificial intelligence in everyday life and solving immediate problems.