Computer Vision and the Internet of Things
- Transfer
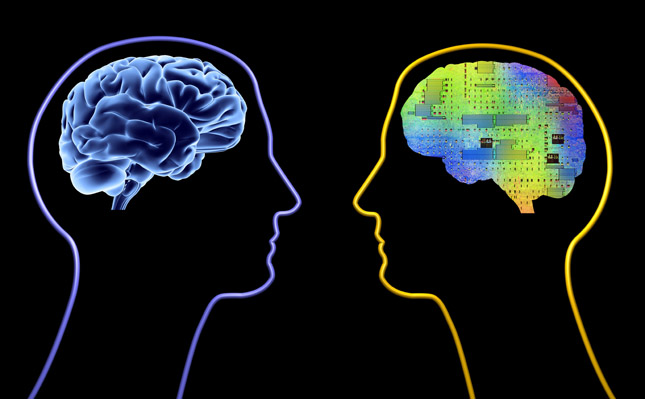
Computers, robots, artificial intelligence ... The basis of many advanced technologies was the need to reproduce or simulate human thinking, feelings and behavior.
Various sensors, for example, acoustic and video sensors, as well as pressure sensors, were created after we figured out how our own hearing and vision are arranged, how we perceive pressure.
Undoubtedly, one of the main sensory organ for a person is vision. Thanks to him, we can see the environment in which we are located, interpret and analyze the situation, take adequate actions.
Human vision is an incredibly complex intellectual “machine” that engages a significant portion of the brain. Neurons designed to process visual information occupy about 30% of the cortex.
For several years now, scientists and engineers have been working on creating devices, objects and things that can “see” the environment, as well as analyze and interpret what they see.
Technological complexity, high resource consumption and prohibitively high costs previously limited the scope of computer vision and related analytics tools, and therefore they were used only as part of security systems and video surveillance. But today the situation has changed dramatically, as the market for video sensors is experiencing rapid growth. Cameras are built into all kinds of devices, objects and things - both mobile and stationary. In addition, the computing power of end devices and cloud solutions has increased dramatically. And this led to a revolution in computer vision.
The affordable price of sensors and cameras, various advanced technologies, increasing the resolution of video sensors, the dynamic range and the amount of computing power for processing video and images - all this leads to a wider distribution of such systems and the emergence of new options for their application.
In the modern world of connected embedded systems, devices and objects, intellectual analysis of images and videos has become possible using classical processing and in-depth training based on the resources of the device itself, as well as cloud computing.
As a result, we are witnessing a boom in the development of technologies for autonomous cars, unmanned aerial vehicles, robots, automated systems for industry, retail, transport, security and video surveillance systems, household appliances, medical devices and solutions for healthcare, sports and entertainment, augmented and virtual reality consumer-level and, of course, the ubiquitous mobile phones. Computer vision technologies and related analytics tools as part of the Internet of things are undergoing rapid development, and this is only the beginning.
In fact, the video sensor made a real revolution, and no other sensor can be compared with it. Video has become part of our daily lives, and most people already take it for granted. Streaming video, providing video on demand, video calls - taking all of this into account, it's easy to forget about the significant impact sensors have in the world of Internet-connected environments and devices; therefore, the video sensor is the most underrated hero in the world of the Internet of things. And in tandem with video and image mining technologies, video sensors create a new dimension for the entire market.
One of the main factors in the rapid development of computer vision has become an increasingly widespread distribution of mobile phones with integrated cameras. Before the revolution in the field of mobile phones, video sensors and cameras, as well as corresponding analytic tools, were mainly used in security and video surveillance systems. But then mobile phones with integrated cameras appeared, which was also accompanied by an active growth in the computing power of end devices and cloud systems, available for video analytics and intelligent analysis systems. This explosive combination has become a catalyst for the rapid development and spread of video sensors, which began to be used everywhere, from robots and drones to automobiles, industrial equipment, household appliances, etc.
There are various types of video sensors, but the complementary elements metal-oxide-semiconductor or CMOS-sensors, of course, had the greatest influence and led to the explosive development of these technologies and the integration of video sensors in various systems and smartphones.
Sensors are everywhere and they are plentiful. Autonomous cars today use more than 10 video cameras, drones - from three to four, surveillance cameras are installed almost everywhere, mobile phones already know how to broadcast video in real time. Video information from these sources is transmitted to the cloud for further analysis, and real-time processing is performed on the devices themselves.
Resolution and dynamic range of video sensors, as well as their number, continue to increase, and in the foreseeable future this trend will only gain momentum. To process, transfer and store large amounts of video information, more and more significant resources are required.
At first, everyone tried to transfer streaming video to the clouds for analysis in real time or ex-post. The clouds provided tremendous processing power, but video transmission, even after compression, required very high bandwidth channels. The need to store huge amounts of data, significant delays and possible security and privacy issues are forcing users to rethink cloud computing approaches. Now many people analyze video information at the device or object level, and then perform offline video processing in the cloud.
And with the advent of the new high-speed 5G connection, which provides minimal delays, the idea arose of distributing the tasks of real-time video processing between end devices and cloud environments. Nevertheless, it remains to be seen how much this is possible (if possible in principle) and whether it makes sense to transfer compressed video from millions of end points to the cloud in real time, almost completely downloading communication channels.
With the realization of the importance of analytics at the level of end devices, various systems on a chip (SoC) have become more widespread, graphic processors (GPUs) and video accelerators. Cloud resources with GPU acceleration are used to analyze archived video or train neural networks on a large amount of test data, and real-time processing occurs on the end devices themselves with accelerators.
In-depth learning technologies and optimized SoCs, along with video accelerators for traditional image processing, help maintain the tendency to perform analysis on end devices, while additional events, parameters and analytics are transmitted to the clouds for further research and comparison. Cloud resources will continue to be used to analyze video archives, while some systems will still perform real-time analysis.
Computer vision. Real use cases
The market for computer vision technologies and related analytics tools will continue to develop. There are currently some surprising trends in technology, and they should give a new impetus to the development of computer vision systems for years to come. Here are just a few examples:
3D cameras and 3D sensors.3D cameras or, in a more general sense, a sensor technology with 3D support, which allows you to determine the depth in the scene and build 3D maps of the scene. This technology appeared some time ago, and today it is widely used in gaming systems such as Microsoft Kinect, and most recently it was used in the iPhoneX 3D sensor for biometrics. And this market is again waiting for rapid growth, when smartphones can provide the necessary acceleration for a much wider range of applications. In addition, robots, drones and autonomous cars with 3D cameras will be able to recognize the shape and size of objects and will use these technologies to navigate, map and detect obstacles. 3D and stereoscopic cameras are also the basis of augmented, virtual and mixed reality.
In-depth training on end devices and in the cloud.Artificial intelligence systems based on neural networks are becoming more widespread. Again, the deployment of deep learning networks was made possible only thanks to the tremendous computing power available today. There are other factors that have led to the rapid development of neural networks and their practical applications, including the availability of huge amounts of data (video, photos, text) available for training and advanced research and development in universities and first-tier companies, which contribute to the popularization and development of open solutions and systems. The result is a large number of neural networks used to solve specific practical problems. In fact, for robots, autonomous cars and drones, deep learning using GPU / SoC on end devices has already become the norm. Cloud resources will continue to be used within deep learning networks, as well as for processing video from archives. Data processing within the framework of distributed architectures covering end devices and clouds is also possible, since network delays and video stream delays are already considered acceptable.
SLAM in cars, robots, drones. Simultaneous Localization And Mapping (SLAM) is a key component of autonomous cars, robots, and drones equipped with various types of cameras and sensors, including radar, lidar, ultrasonic sensors, etc.
Augmented / virtual reality and perceptual computing. Take Microsoft HoloLens as an example. What is this system based on? Six cameras in combination with depth sensors. Microsoft has even announced the creation of a research center in Cambridge (USA), which specializes in the development of computer vision technology for HoloLens.
Security / CCTV.This article does not address this area of the collection and analysis of video information. This in itself is a very large market.
Biometric authentication in mobile phones and embedded devices. Biometric authentication can give a new impetus to the development of mobile applications, and here again video sensors are used in combination with analytics tools on end devices and in the clouds. As this technology develops, it will be implemented in various embedded devices.
Retail. The Amazon Go store is an example of the use of cameras and advanced video analytics. Soon, customer consultant robots equipped with several cameras with a video analysis system, as well as other sensors, will meet buyers' shelves.
MASS MEDIA.Video analytics is already widely used in the media industry. Video analytics systems allow you to view large video files in search of a specific topic, scene, object or face.
Sport. Real-time 3D video, video analytics and virtual reality will create a new generation of personalized sports and entertainment systems.
Perspectives, Challenges, Motives and Problems
The need to constantly increase the resolution, dynamic range and frame rate of video, as well as the performance of video analytics systems, necessitates a corresponding increase in computing power and the expansion of the capabilities of data transmission and storage systems. And it is not always possible to quickly solve these problems.
Several companies take a different approach to solving this problem. Neural networks are based on the results of research in the field of biology, similarly, developments and commercial products in the field of computer vision begin to appear, which respond to changes in the scene and generate a stream from a small number of events instead of transmitting a sequence of images. This will allow the use of video collection and processing systems with much more modest capabilities.
This approach seems promising; it can radically change the way video is received and processed. As a result of a significant reduction in the required computing power, greater energy savings will also be achieved.
Video sensors will continue to be the main catalysts for the rapid development of the Internet of things. Similarly, endpoint-level video analytics will continue to drive the development of the SoC and semiconductor industries, helping to improve video accelerators using GPUs, specialized integrated circuits (ASICs) , SoC logic inference, user-programmable gate arrays (FPGAs), and digital signal processing algorithms (DSPs) ) All this will also contribute to the improvement of traditional image processing systems and deep learning technologies, and developers will have more opportunities for programming.
Today it is a battlefield where many major players and startups have come together.
Built-in low-power video sensors
Currently, millions of self-powered objects use video sensors and video analytics, so improving the integrated video sensors with low power consumption remains one of the main growth factors for the entire industry in the new era, as well as one of the key problems that need to be solved. The advent of devices and systems with integrated video sensors and video analytics tools necessitates the analysis and elimination of privacy and security problems already at the design stage.
Despite all the problems and challenges, systems that combine computer vision technology and the Internet of things have a great future and huge market opportunities, so companies that can cope with these problems and challenges will be fully rewarded.