
BDSL-2017: Tanya Bibikova on data visualization
This summer, the Data Laboratory made a sortie to Baikal, where, among other things, I conducted a master class on data visualization in the Baikal design special camp . By the way, on Baikal - stunning!
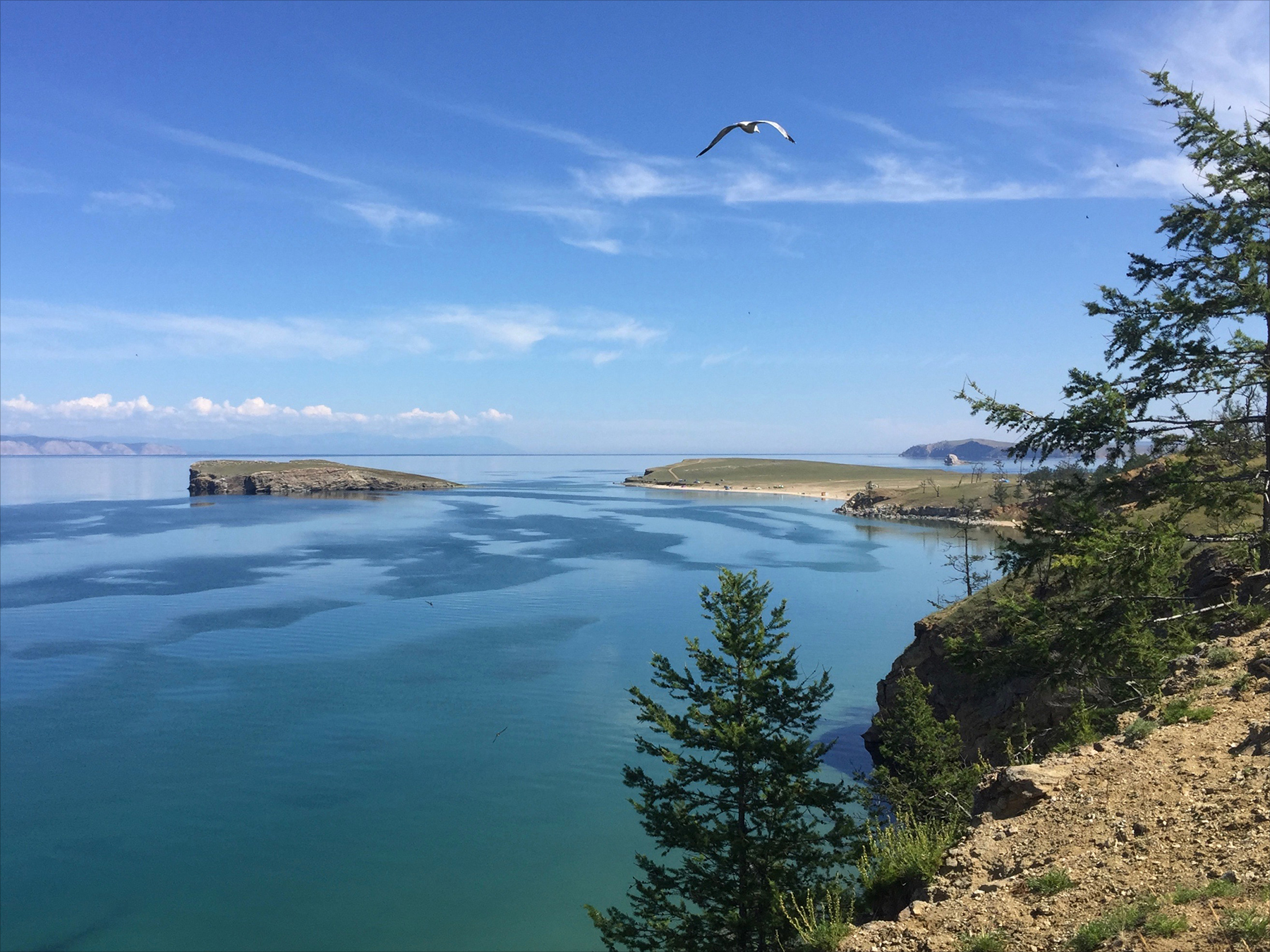
The video of the master class is promised, but will appear soon. In the meantime, one of the camp participants decrypted a recording of my speech. I give here the first part of the decryption of the lecture with minimal cosmetic changes and the synopsis of the second part, devoted to the Δλ algorithm, which we study in the course on data visualization.
Vela Tanya Bibikova from the Data Lab
Decrypted by Misha Novikov
Today we will talk about how to create cool visualizations - visualizations that reveal the nature of data and reveal patterns hidden in them. But to understand how to create good visualizations, let's first figure out which visualizations we will consider good. I will show you some examples.
Earthquake History All earthquakes from 1898 shown on the map. We took a regular table with dry data, where there is a year, the date of the earthquake, place and strength. Just placing this table on the map, we showed a picture that no one has ever seen and cannot see with their own eyes: the outlines of tectonic plates appear behind the history of earthquakes
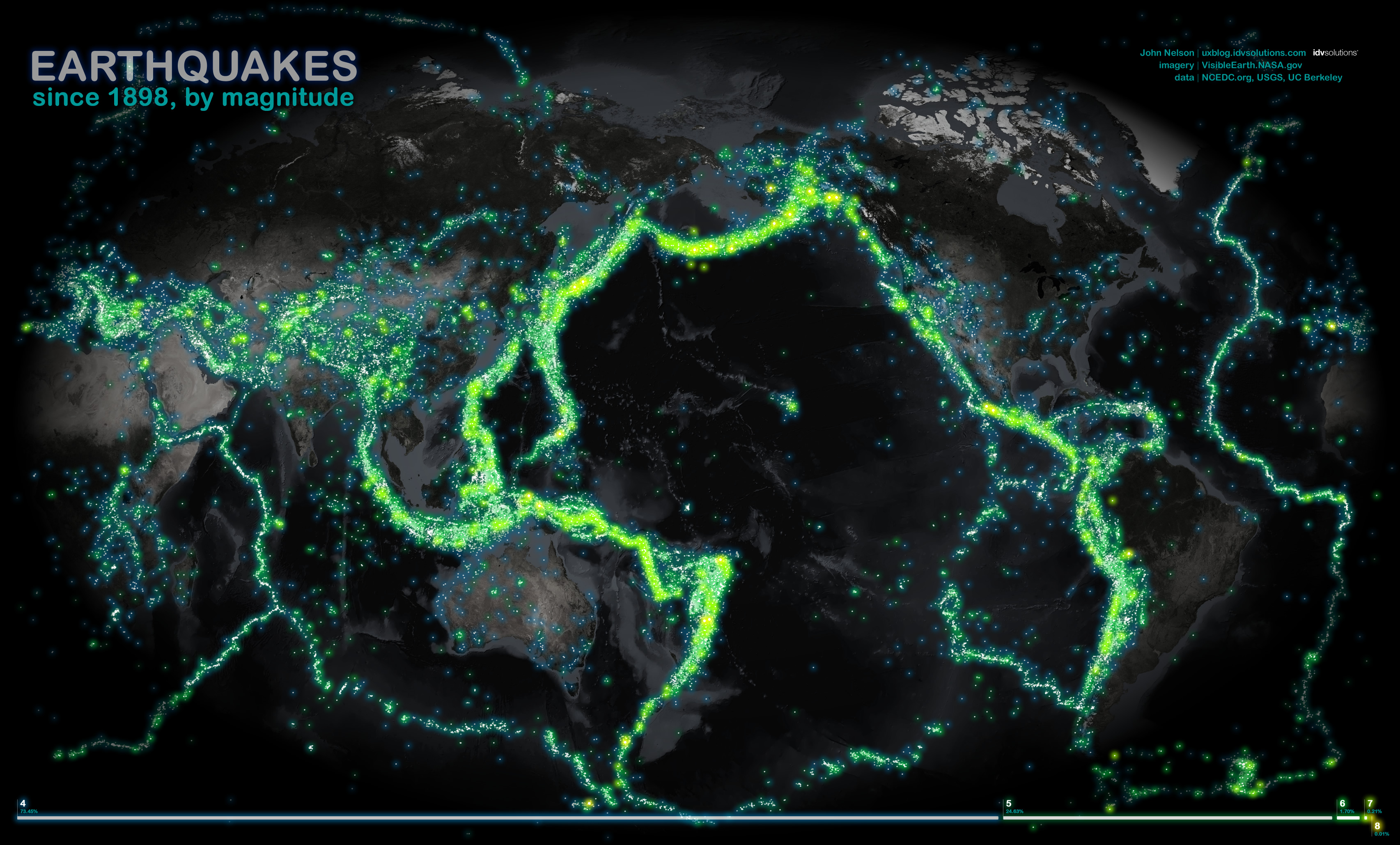
Results of the Moscow marathon The next work is our pride, we were given bronze for it at Malofiej (a prestigious competition in interactive graphics). Given: marathon results that look like a table with fields - last name, first name, age, country.
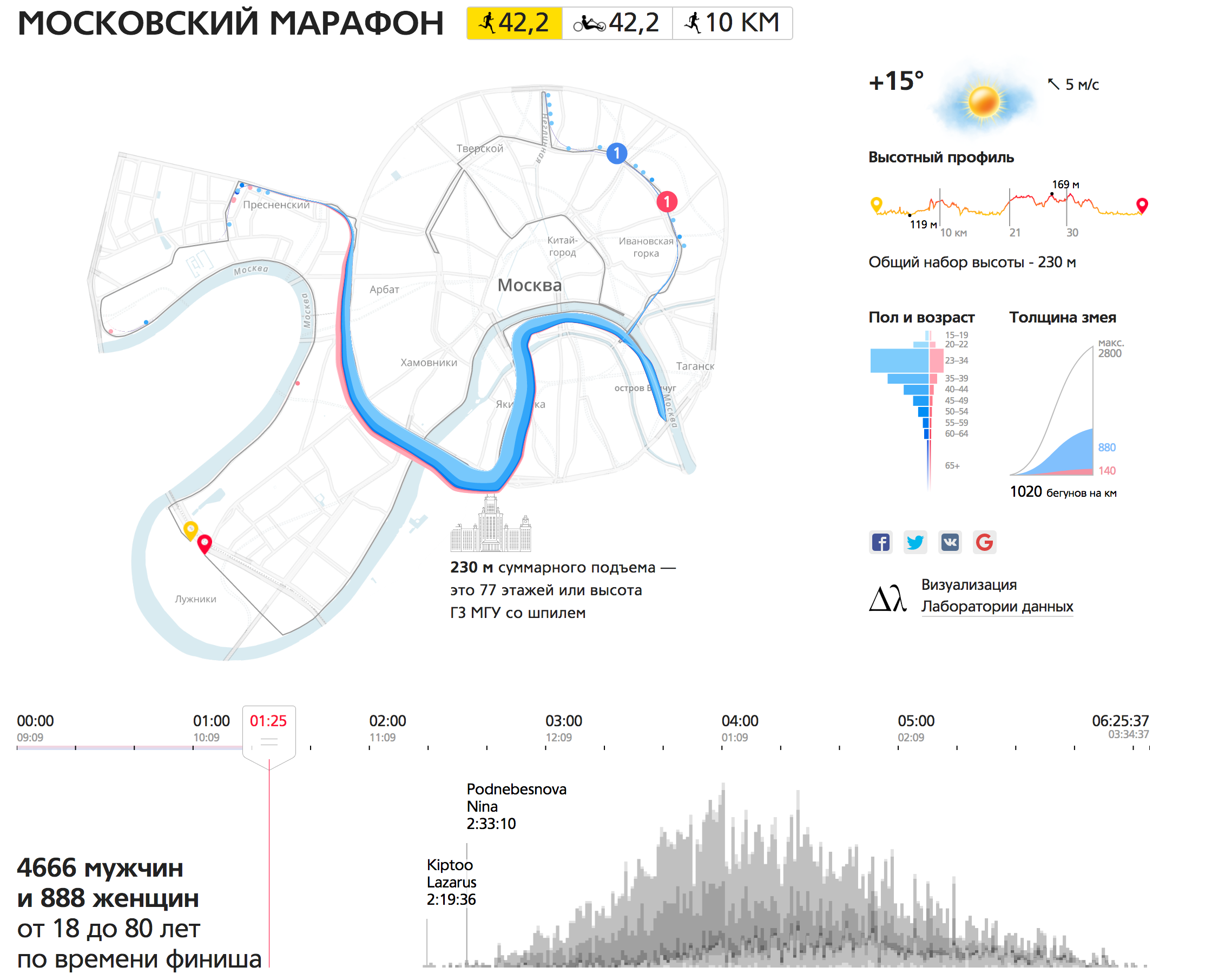
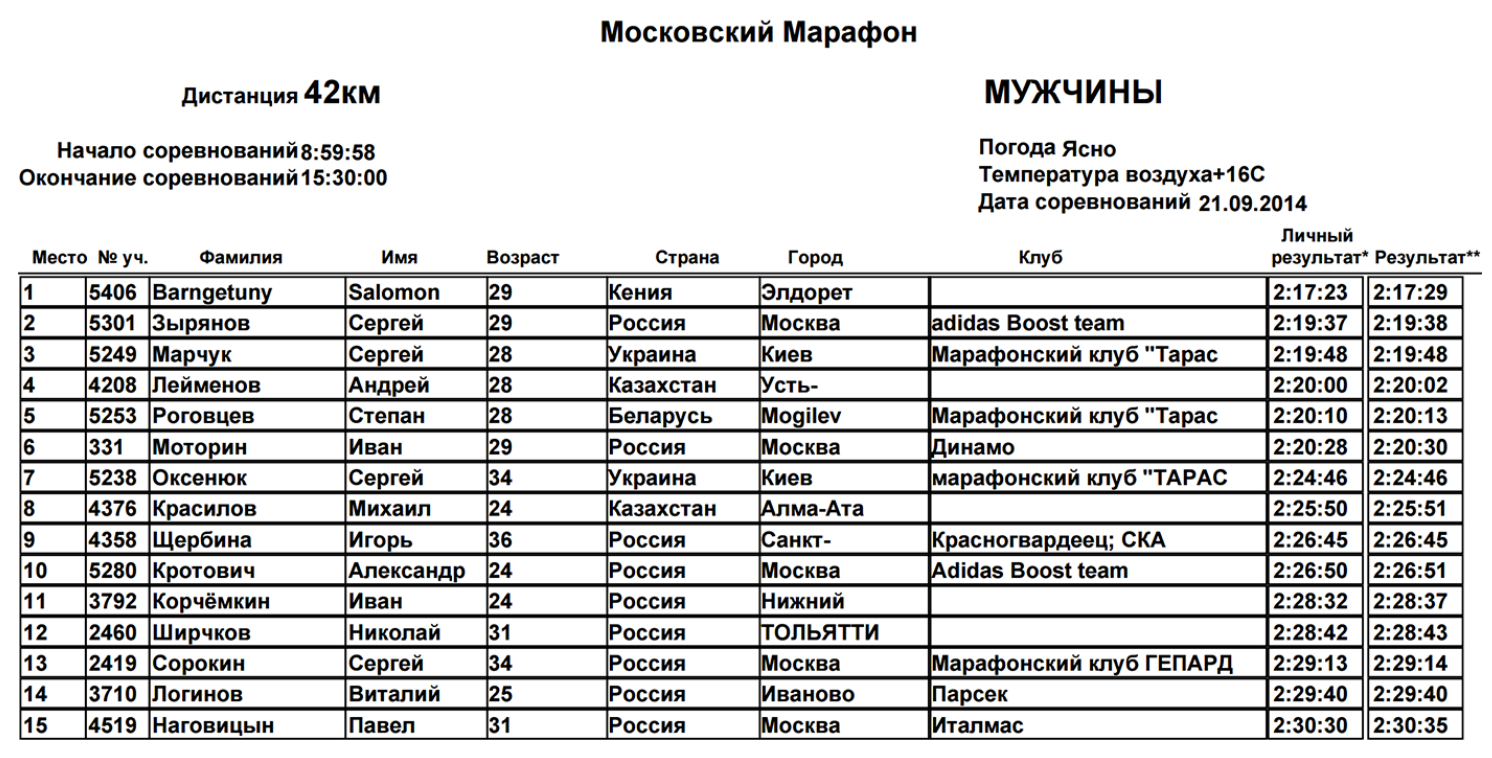
Why did you want to do something with this data? In 2013, I myself was preparing for the marathon and participated in the intermediate races. My first run inspired me a lot because it was a huge crowd of runners, of different ages, running communities, teams. The most interesting thing happens during the race, because it is a huge crowd that runs along the route, forms groups. Someone is trying hard, he is giving all his best, someone is doing it for himself, at a relaxed pace. When, after my first official race, we were given a dry table that did not reflect all that was happening, I wanted to make a visualization.
We have a crowd of runners running along an interesting route through the whole center of Moscow. At the beginning, the crowd is big and thick, over time it stretches very much along the highway. When the runners are almost at the finish, the tail just runs out of the garden ring.
There are many data dimensions on this visualization. The crowd is divided by color - men and women, and by age, which is shown by a shade of color - the darker the older. From this visualization, you can extract interesting mini-stories. For example, pay attention to the winner. Here, when he runs out to the embankment, he is somewhere in the 7-8th place, already on the embankment he makes a jerk, overtakes everyone and finishes first.
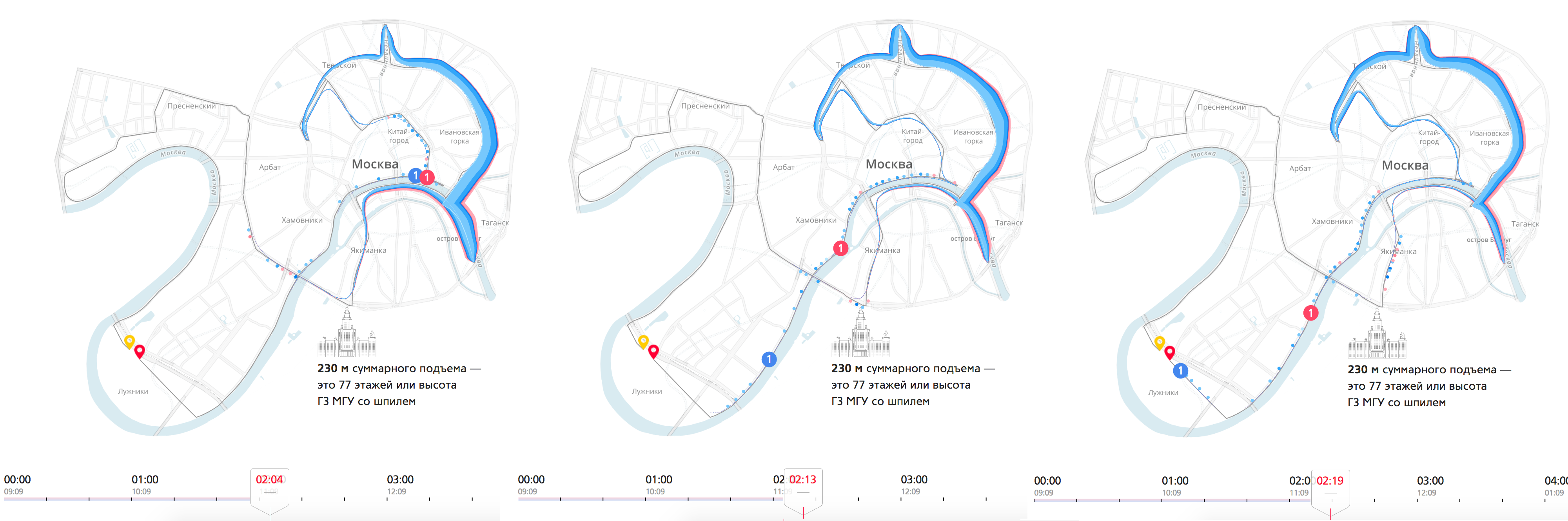
Look: we took a dry table, added intermediate results (10, 15, 21 km, 30 and 35 km) and got a huge layer of new information that it would be difficult for us to extract from the table. We got a complete and holistic picture of what was happening.
In addition to reflecting the positions of the runners, we added small charts, distributed the runners by gender and age. On the histogram of the finishes, you can see how the participants distributed according to the finish time, find those with whom you ran together. There is also a list of participants with the ability to filter (filters affect the main visualization). And the dynamics of the weather throughout the race, the weather for marathon runners - this is very important, directly affects the result.
Question to the audience: Why is this visualization cool?
Answers:
- You can watch in dynamics
- It becomes visible new information that was not in the table. For example, a jerk on the embankment
- Map and python, due to visibility, create a feeling of simplicity and integrity of the story, of what really happened. This is not an isolated set of graphs, but a holistic display of reality.
Comparison of tanks World Of Tanks
There are players who move from level to level. At each new level, the properties of tanks are improved, they become more armored and powerful. The main characteristics of the tank are attack, defense and speed.
Here is a diagram devoted to the properties of tanks that was sent to me by one of the game lovers for analysis:
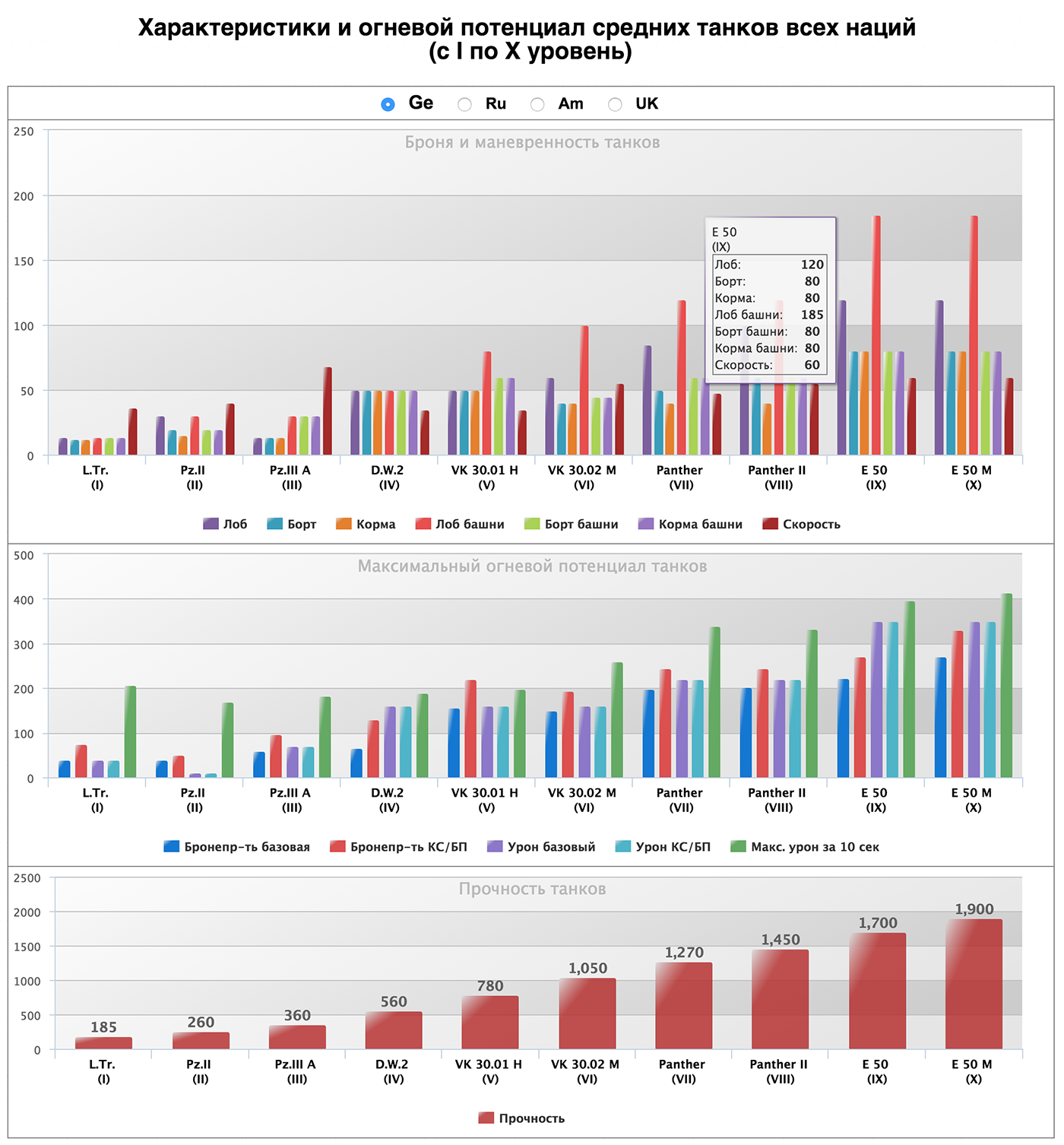
And here is the visualization we made in the laboratory:
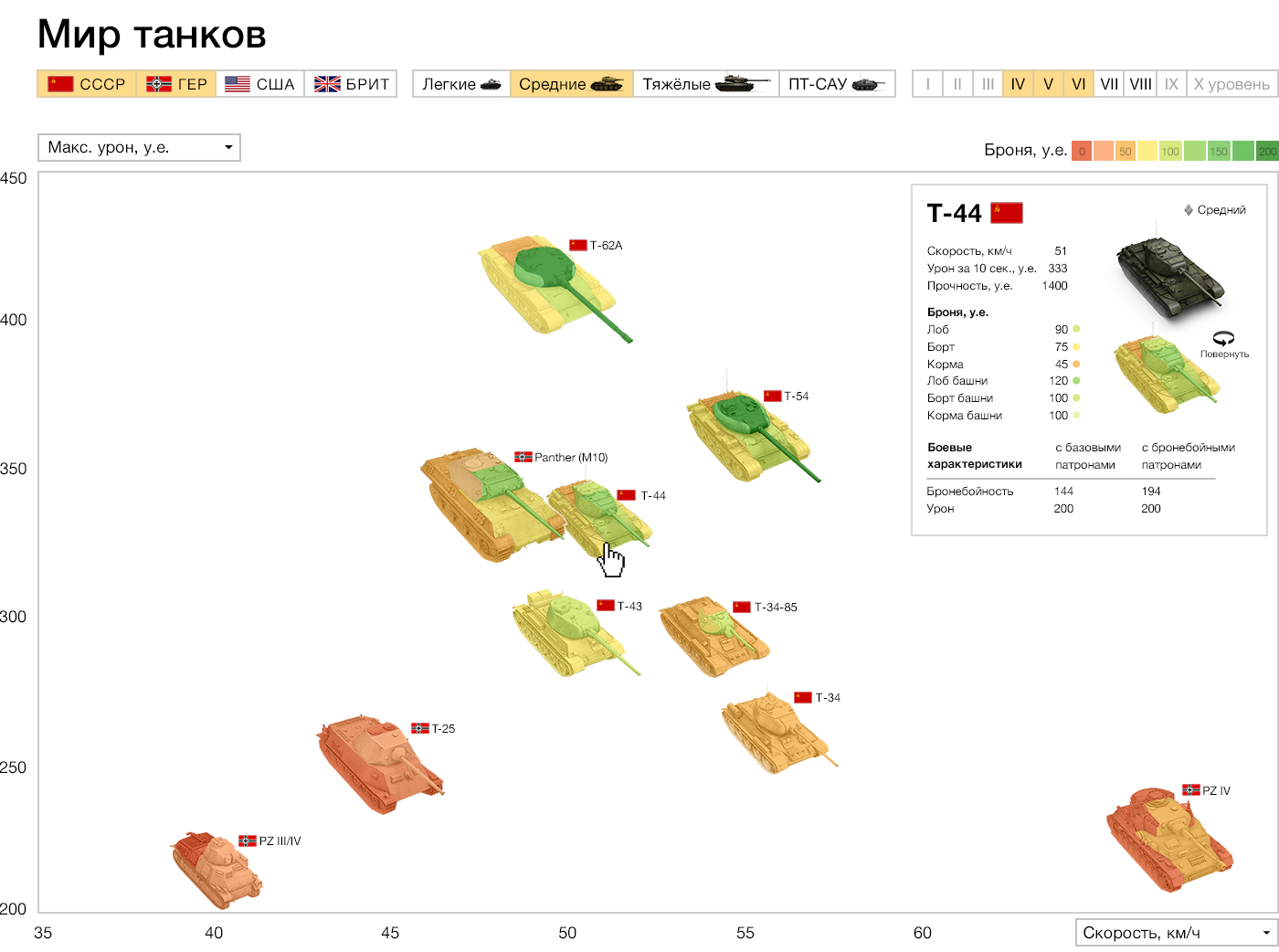
Horizontal speed, attack in 10 seconds of vertical, armor is shown in colors directly on the image of the tank. More detailed specifications and a real click-through image. Above are filters by country and tank class. Instead of dry diagrams, we look at the size, shape of the tanks.
Question from the audience: But at the same time, not all parameters are always visible. On your version, unlike the first one, you can’t find out which tank is cooler at first sight
If desired, you can turn on all tanks of all levels. But we intentionally cut the standard look so as not to clog the screen, and not to impair perception. By the way, by the way, not all of them were also the initial version, but only ten in some unknown way selected tanks - one for each level. The main conclusion that can be made there - with increasing levels, indicators are growing, the most noticeable deviation - in one of the tanks, one of the indicators did not increase. On our visualization, you can compare tanks of one and neighboring levels.
Comment by Ilya Ruderman, WOT player:
- It takes three to four days to pump the first three levels. But to pump from the fourth to the tenth, you need to spend a lot of time. You value your time, so you need to know as much as possible about the next tank in the development tree. The game has a large audience, and the slightest changes in the parameters and characteristics of tanks greatly affect the gameplay. Therefore, players pay a lot of attention to the characteristics. The work is cool. She has great potential, but lacks several critical parameters.
Question to the audience: How good is this visualization?
Answers:
- Filters and parameters do not pile on a huge pile of switches, but are neatly spaced and built into the visualization. There is little visual noise, and each element helps perception, but does not interfere
- On the first chart about tanks, we compared all the capacities of all tanks. This is not very useful since if the tank is weak, what is the point of comparing it with the strong? In your version, you can easily filter them and receive only important information.
- A motivated player will study the first schedule, but your visualization has the potential to attract an ordinary player who is just interested.
The rhythm of life in big cities, Jawbone
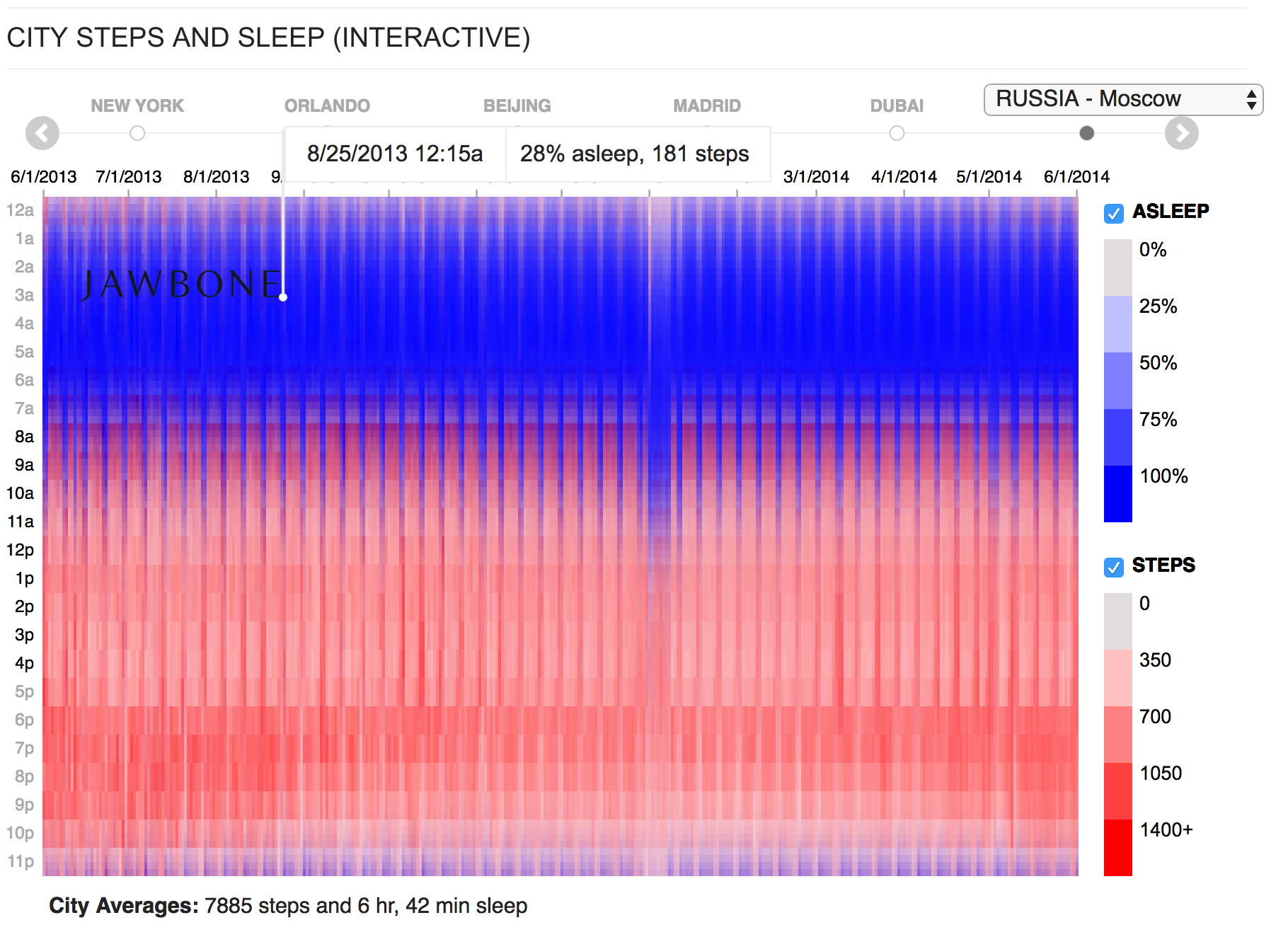
The diagram shows user activity in different cities. Horizontal - 365 days of the year, vertical - a day with a breakdown of 15 minutes. This is ... 365 x 24 x 4 = ~ 35 thousand data cells x 2 parameters: sleep and steps. It turns out ~ 70 thousand numbers, which are reflected in one picture, 45 such pictures for 45 cities. These are averaged numbers for all users, imagine how much data was in the input!
What catches your eye? Chopsticks - the weekend, everybody sleeps. Noticeable subsidence January 1 - celebrate the New Year. On the original visualization, a few seconds are loaded every year, this eliminates the possibility of quick comparison. We took the original graphics and made it so that the data is downloaded immediately and it is convenient to compare.
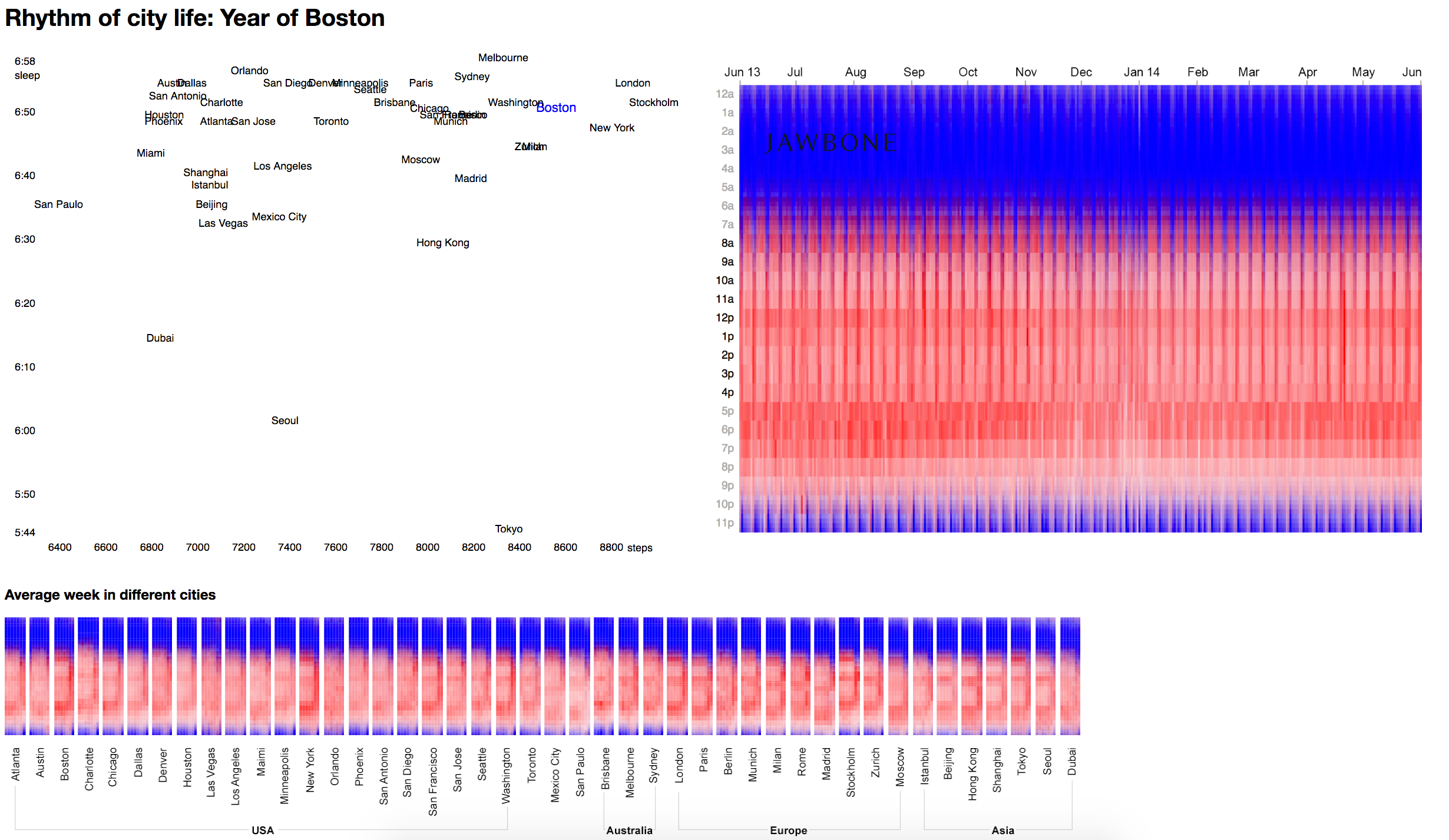
Try our version. See what happens in the summer in Stockholm and London, how much more regular London is. They only have changes for the New Year. Now let's compare London and New York: there are long weekends and weekends in New York. Now look at Madrid, how their siesta after an hour of the day changes the whole picture. And now Miami or Phoenix, where everything is very even and there is not much difference between weekends and working days. In Dubai, for some reason, everyone sleeps in June. Perhaps this is the influence of some kind of religious fasting.
The bar below is the average week of one city. It shows, for example, that Charlotte in the United States is strongly knocked out at the start of the day. And the evening in Tokyo smoothly turns into night.
You can see that New York, Washington, Boston have an actively expressed week and weekend with a lot of activity, and on weekdays everything is pretty sluggish.
An additional level of instant comparison works well with the ability of our eyes to notice changes in similar pictures (Tufty writes about this). Visualizations are cool on their own, you can combine them with other wireframes, connect interactively and get a more powerful communicative effect.
NYT Basketball Visualization
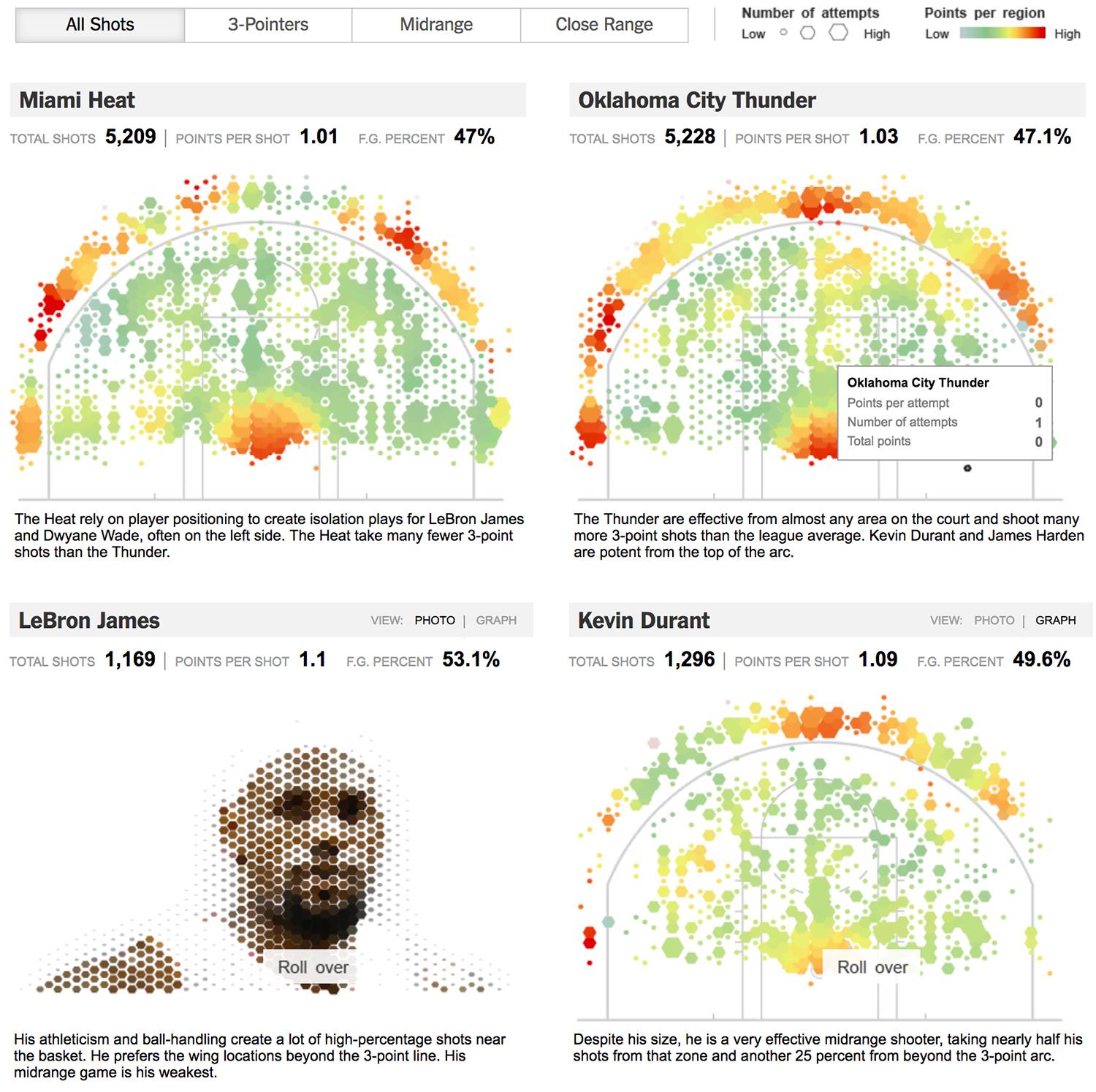
The basketball court and the ring are shown here, and on top is the number of shots from different points of the court and the effectiveness of the shots. The size of the hexagon is the number of throws from a position, the color - the redder, the more hits from here. Statistics are collected for two different teams, there are also summary data: total number of shots, points per shot, percentage of hits.
When two pictures are nearby, we can quite easily compare two teams. We can notice some patterns: Oklahoma City Thunder throw three-pointer from the center, but Miami Heat - no. Their performance there is very low. But the most interesting part begins when we turn to the statistics on players. [When you hover over the images of the players, they scatter and turn into diagrams, enthusiastic sighs from the hall.]
The comparison by players is more interesting, here the wow effect does not interfere with perception and fits very naturally into the graphics. Kevin Durant is a three-pointer special, and LeBron James throws out from under the ring. You can compare and see the technique of different players and get an understanding of the style of the game. You can still see who is left-handed and who is right-handed
Question from the audience: How do they record all this?
Now more and more sports analysts and television channels are beginning to understand the power of visualization and are spending resources on collecting and aggregating such data. As a last resort, you can find records of all goals, and create a dataset manually.
Cash flow visualization
Next boring table, finances:

On the table are the constituent entities of the Russian Federation and their cash turnover by individuals / legal entities, residents / non-residents, cash disbursement / deposit and the amount of turnover. Central Bank analysts are not interested in operations at any given moment in time, but are interested in anomalies and outliers. For example, a sharp jump in cash withdrawals in some entity is a reason for suspicion of fraudulent schemes. We did color coding by anomaly. The internal algorithm calculates the percentage of anomalies and colors the area according to the index.

In the Novgorod region, some values deviate from the average, and we see this anomaly on the map. When we saw the anomaly, we can try to figure it out and fall deeper. The anomaly here turned out to be an extradition. These were large seizures from residents in March, May and January. This visualization does not allow you to dig even deeper, but already here we can watch the temporal dynamics of the season. It is unlikely that this is tourist activity, tourists will not rake nine billion rubles. Perhaps this is an occasion for trial. This is not real data, the institute generated a conditional set for test work.
Here's a classic example of a combination of formats. When we work with a map, the chart comes to life in dynamics. And when we work with the schedule, the map changes, we can see that in April 2013 there was an anomaly in the Tomsk region.
At the customer, all this information is stored on 30 sheets in Excel, and to see such a picture they travel through these 30 sheets. There is a special person who in this exel is guided and digs out the necessary information. The advantage of visualization is that you can pack a huge amount of data into a concise form.
Map of Venice A great example of the relationship between data and color on a map. Color fill areas are areas that are flooded at different frequencies. Some areas are flooded more often. An interesting combination of two data types. On the one hand, this is the water level, and on the other, on the same scale, the frequency of flooding. In addition to filling areas, there is another semantic unit - at home. Light shows buildings that are weakly affected by flooding, dark, which are destroyed very much
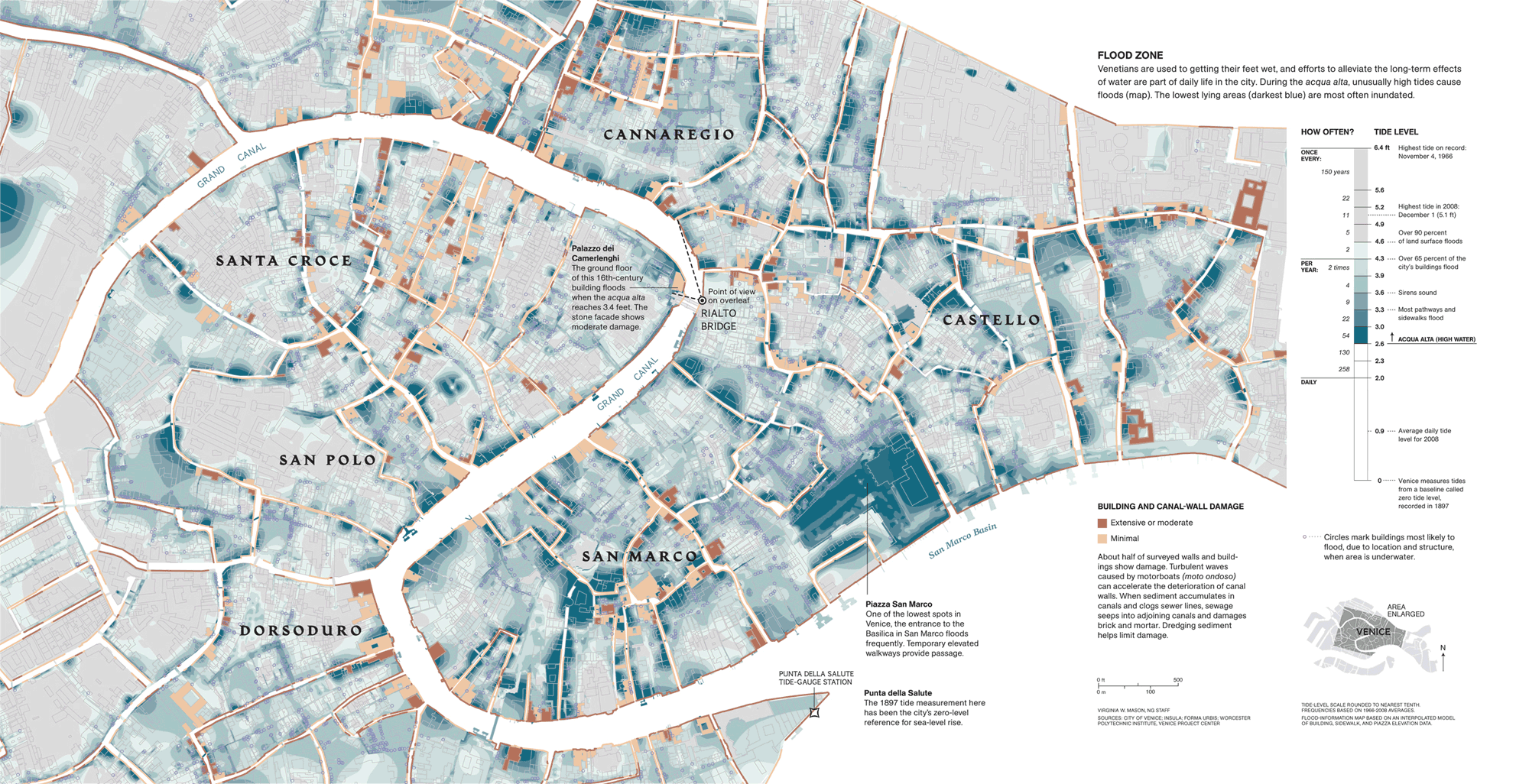
Who will marry whom? The grid on the background is made up of different professions. On the left are more masculine professions, on the right are more female professions. When hovering over a profession, we see communication lines of different thicknesses that show how often people from this profession marry people from another profession. Circles mean that people get married inside one profession. The color of the gradient shows the floor inside the pair. Firefighters marry kindergarten teachers. Designers marry sales managers. Such graphics are in no way tied to the classical formats of information representations. But it takes into account the features of the data that underlie visualization to the maximum and makes super-visual what we want to show - the frequency of the marriage. It turns out very minimalist. And this joke is really interesting to study.
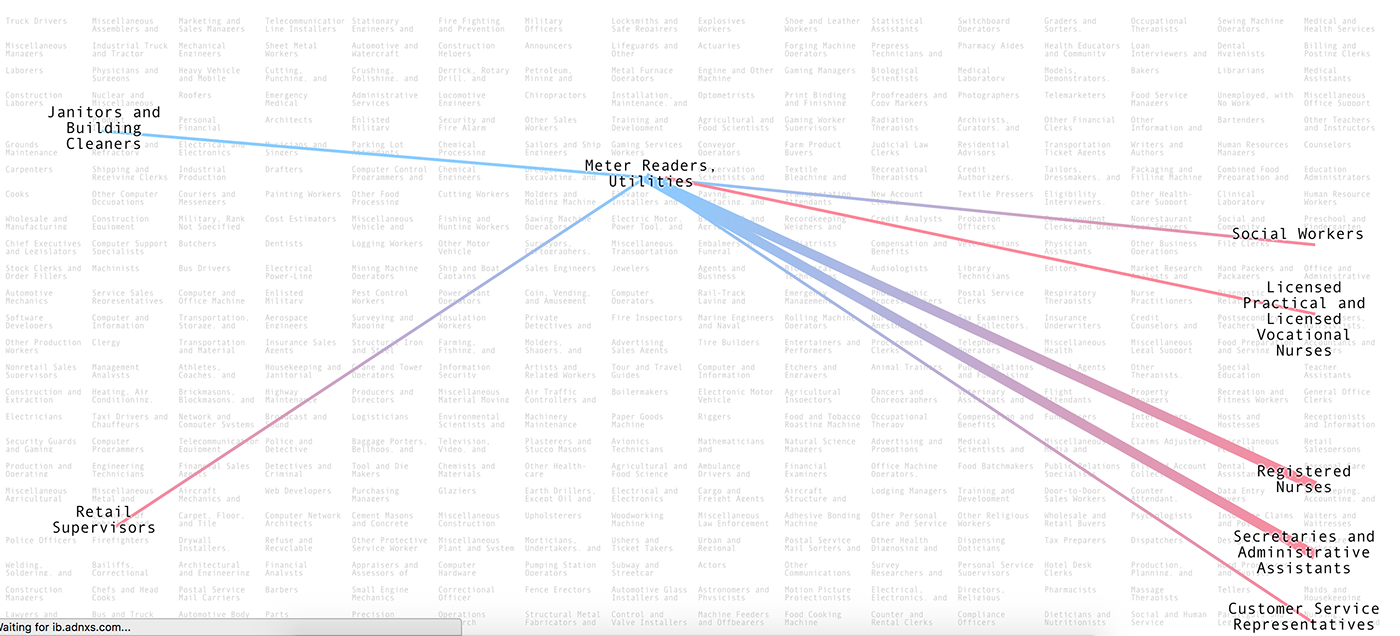
Question from the audience: And in what way are professions scattered horizontally from more masculine to more feminine?
Most likely some tricky algorithm, distributing depending on the proximity of professions, so that the final picture looks good.
Classy visualization, conclusions
Question to the audience: All the examples are very different. What unites them and makes visualization cool?
Answers from the audience:
- The data does not cease to be uninteresting and lively from the fact that the topic is dry and uninteresting.
- When the visualization is done well, I want to explore it and ask questions.
- When multidimensional data is connected, a new meaning is born from their comparison.
...
Here is my understanding.
In an interesting visualization, in which there is a sense of “magic”, there are always many dimensions on a flat screen. We use different expressive means and methods and techniques to make perception convenient.
At the same time, when we show all these measurements, we remain clear. This should not be a mechanical coding "yeah, I have the property height, I will encode it with color, I have the property length, I will encode it with hatching." Try to think what real things are behind the data. If this is the armor of a tank, draw directly on the tank where it is located. Choose coding methods as close to reality as possible, closer to intuitive ideas about colors (boys - blue, girls - pink). The more intuitive coding you use, the easier it will be to perceive the work, even if many data dimensions are sewn into it. There will be no need to make signatures and guides “How to read the schedule”, huge legends will not be needed. Perhaps a minimalistic legend will itself become a diagram. At the same time, the schedule lives well without a legend.
This does not always work. Sometimes a new type of visualization is better suited, which is not immediately clear, but solves the problem very well. You can do that too. But ideally, one should strive for clarity and intuition.
When these principles are followed, a certain general picture is born, we can glance at the data as a whole, without switching over thirty tabs, without switching the cities of the country, we can see a complete picture of what is called a big picture. In the big picture we see patterns, anomalies, communications. It is easy to make comparisons. If we do not have a big picture, but only five scattered graphs, the benefits and effects of visualization will be completely different. After we saw the pattern, we can go deeper and start exploring (cash flow, city jawbone). See the whole picture, notice patterns and go deeper and answer questions about this pattern. This is power, and the key to cool visualization.
We looked at cool examples of visualizations, now let's talk about how our process works inside the laboratory. Most of the results of working with data are completely different from the examples we looked at. The word “visualization” usually immediately presents dashboards, scattered graphs, pie, bar charts, and sometimes sans.
I have a hypothesis of why this happens. Analytics, working with data and visualization is often perceived as a boring and dreary business, because the data is stored in tables or databases. And these data sheets and tables look the same. The same problem with standard types of visualization.
All data is unique. They are alive, something always happens inside them - objects interact, their properties change. If we put this life in the table, we fix it with a dead slice. When we try to visualize this slice, we get moderately informative, but rather boring, inanimate dashboards. Such a straightforward approach, although the most logical, but leads to dry and primitive results.
Although it is not always as simple as it seems. There are a lot of nuances, and analysts get a lot of money to work correctly with the display of data.
To create the “sense of magic” that we talked about in the first part, you need to turn on your imagination and go beyond the tables. You need to imagine what is behind these tables, what generates this data.
Data reality- this is a set of processes that occur in the physical space and generate
data.Here, Katie Ledeki breaks the record and wins Olympic gold in the 800 meters freestyle: This is a great example of how, by connecting the reality of the data, it is possible to visualize what actually happened. How a boring table turns into a thing from which it is difficult to take your eyes off. And another example, but from a different area. Map of Italy's specialties Antoine Corbino: This can hardly be called data visualization, but it is a good example of not being afraid to take real objects, as in the example with tanks or a basketball court. There is no need to be afraid to place everything on a map, to tie it to some real physical space.


We try to use the real “landscape” and transfer elements from our physical reality to visualization. This greatly enhances visibility and brings us closer to the big picture.
Determining the reality of the data, including imagination and imagining what is behind the dry tables, having seen this picture, we take a step to show the essence of the phenomenon, and not a dead and dry cut. The reality of data helps to see the nature of data behind uniform tables.
A data particle is a call from the micro level, it is an elementary data brick that allows you to unravel a tangle of data of any complexity. There may be several, but most often one. It is an analytical work, to find an entity, a unit that will allow you to work with data as flexibly as possible.
Represent the reality of data and isolate a piece of data - The first step of our Δλ algorithm. Introduce the whole picture, and find the smallest brick. Data turns into a collection of particles that we can group in any convenient way.
Next, we move to the two-dimensional plane of the screen and begin to lay out the particles on it as you like. This is the second step of the algorithm - selection of the frame and visual atom.
A wireframe is a way of organizing two dimensions of a screen, which conveys the properties of a data particle due to its position on the screen and changing this position. The framework distributes the data particles on a plane so that they can be compared with each other.
Visual atom- a visual embodiment of a data particle on the screen. Visual atoms show data parameters using color coding, size, shape of objects and other visual attributes.
Why are these difficulties with frameworks and atoms? The ability to separate spatial measurements and visual, as it were, intrinsic properties of a particle significantly increases the flexibility of our approach to data display. Any visual atom can appear on any frame. Even if we consider a small set of basic frameworks that are familiar to everyone, in combination with graphic primitives (point, figure, line ...), we get an impressive table of possible visualization formats:

Why don't I start the lecture with this wonderful table? It seems to me that this table encourages you to follow the standard path and choose a visualization from a large set of graphs in order to push our data into it. And when we try to shove the data in a predetermined form, we do not take into account their essence, their features, and we lose a lot. I want us to dance from the data and therefore I pay so much attention to the first part. Data is more complex and interesting than all standard formats. In general, I have a feeling that the data has its own idea of what form it is better to be in :) That for each data set, taking into account their internal features, there is its own most suitable way of presentation. I do not want you to perceive this table as a tool to cram data into some format.
The third step of the algorithm, after we dumped the data on the frame. We are starting to add interface elements, filtering tools. Perhaps we got too many points and they ripple, then we can merge the points together into shapes. After we have distributed the data on the wireframe, we can add controls based on the scenarios of how we want to work with the data.
The algorithm is laid out very cool on the example of visualization of the losses of the Second World War: The action of the algorithm will now seem to occur before our very eyes, in real time (turn on the video).
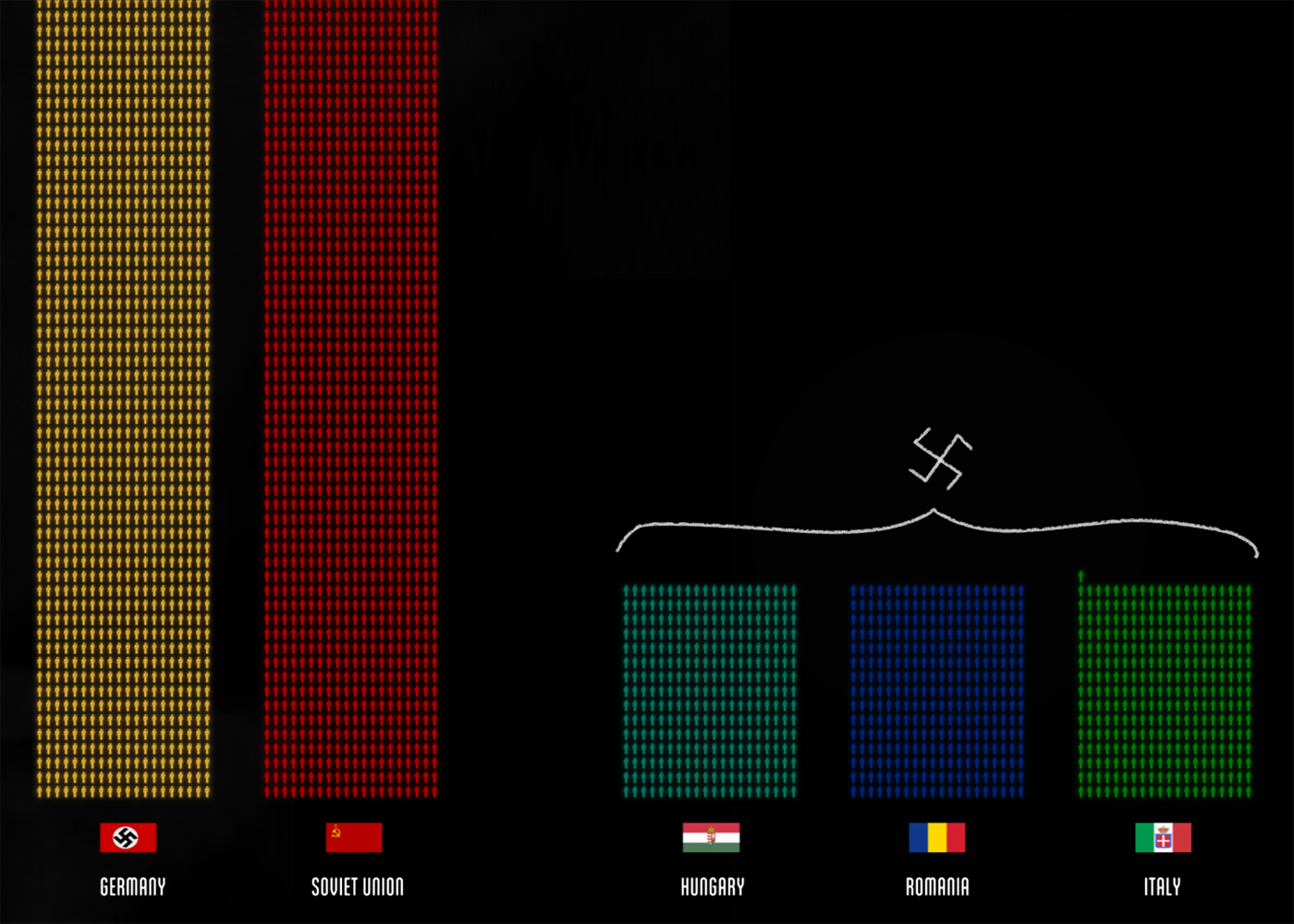
The video begins with an introductory word - this is the reality of the data. Now they have chosen a piece of data - a civilian and a soldier. The first step of the algorithm has been completed. The visual atom is a human figure in which 1000 lives are encoded. Moreover, two different figures - a soldier with a gun and an unarmed civilian. From individual little men a lot of data is obtained. At some point, all the data is on the time axis. We see how the number of losses increases over time and how it is distributed in different battles. This is the second step, we throw the visual atom into different frames, we see different pictures.
Another grouping method is applied to the same particle - by colors, which mean other meaningful parts. It turns out that the data particles are the same between each other, but we can easily compare them with each other and draw conclusions. Pay attention to the scenario move - the idea is repeated that all these are living people, and not points on the chart.
After that, the points turned into columns (third step), because the scale is too large. In this example, as on the famous Minar map , the power of a holistic picture is manifested, in which we see every point, every death. This resonates much more than the dry figure of 87 million, or bars.
***
Read more about the algorithm in the "Complex Data Visualization Algorithm" .
I invite everyone who has read the note to the end to a course on data visualization . On the course, we further analyze the concepts of reality and data particles, get even closer acquainted with wireframes, visual atoms and the principles of working with them. In addition, the course participants learn visualization tools - the Scoreboard and the d3.js library, and on the last day of the course they create their own visualization under the guidance of teachers. To activate the discount of 5 thousand rubles. , when recording in a comment, make a note "from Habr."
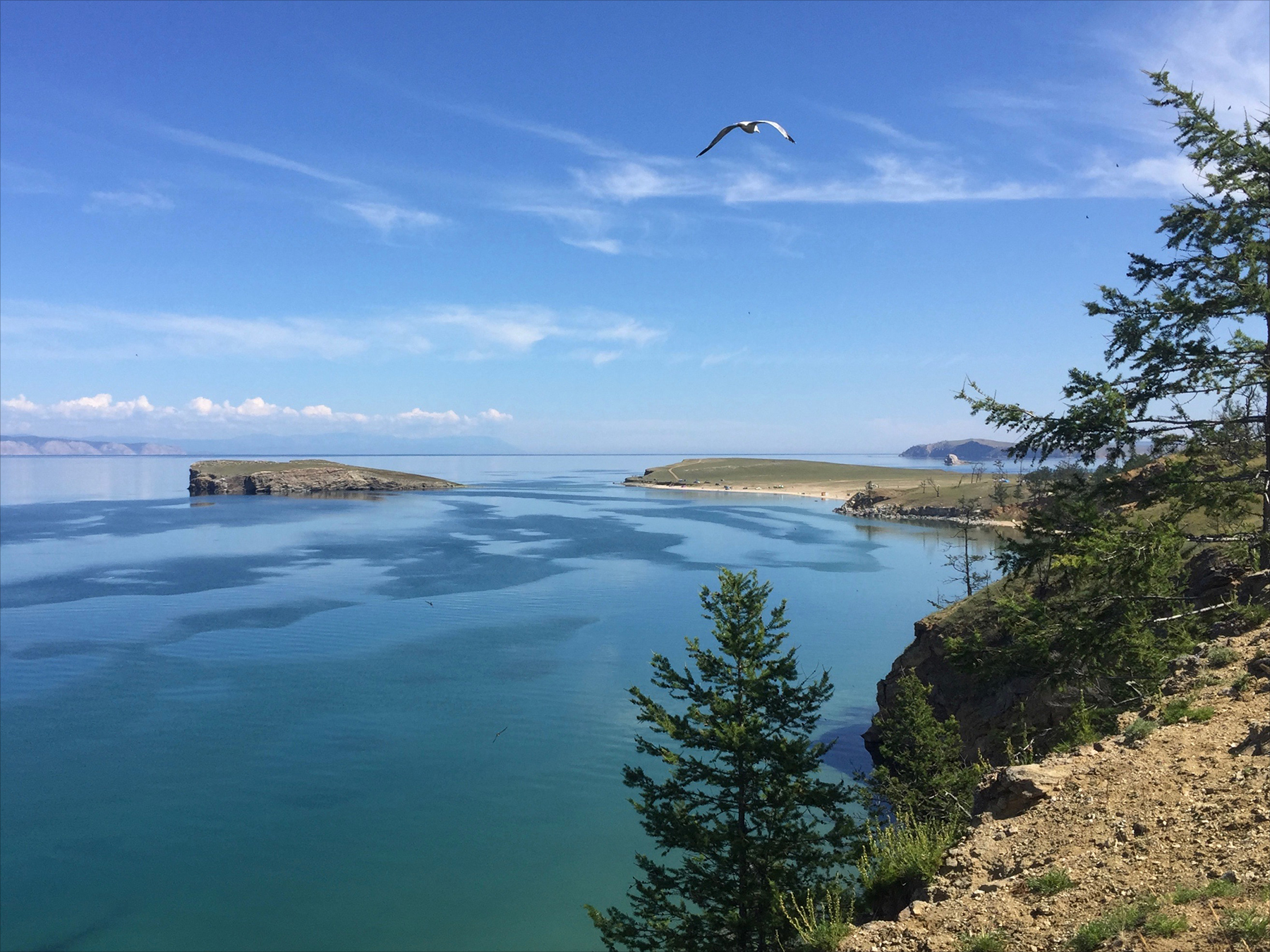
The video of the master class is promised, but will appear soon. In the meantime, one of the camp participants decrypted a recording of my speech. I give here the first part of the decryption of the lecture with minimal cosmetic changes and the synopsis of the second part, devoted to the Δλ algorithm, which we study in the course on data visualization.
Data Visualization Workshop, July 22, 2017, BDSL
Vela Tanya Bibikova from the Data Lab
Decrypted by Misha Novikov
Today we will talk about how to create cool visualizations - visualizations that reveal the nature of data and reveal patterns hidden in them. But to understand how to create good visualizations, let's first figure out which visualizations we will consider good. I will show you some examples.
Earthquake History All earthquakes from 1898 shown on the map. We took a regular table with dry data, where there is a year, the date of the earthquake, place and strength. Just placing this table on the map, we showed a picture that no one has ever seen and cannot see with their own eyes: the outlines of tectonic plates appear behind the history of earthquakes
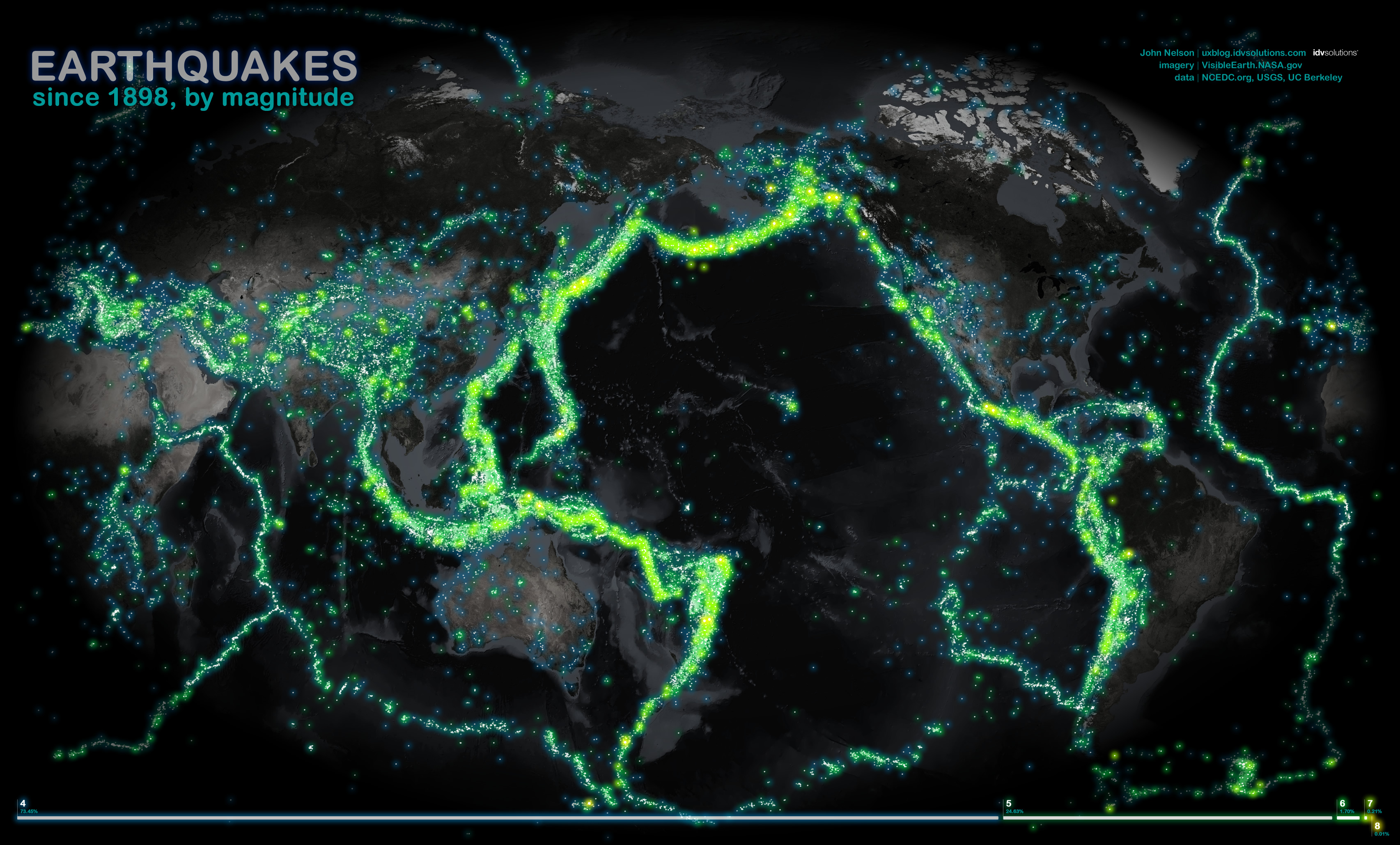
Results of the Moscow marathon The next work is our pride, we were given bronze for it at Malofiej (a prestigious competition in interactive graphics). Given: marathon results that look like a table with fields - last name, first name, age, country.
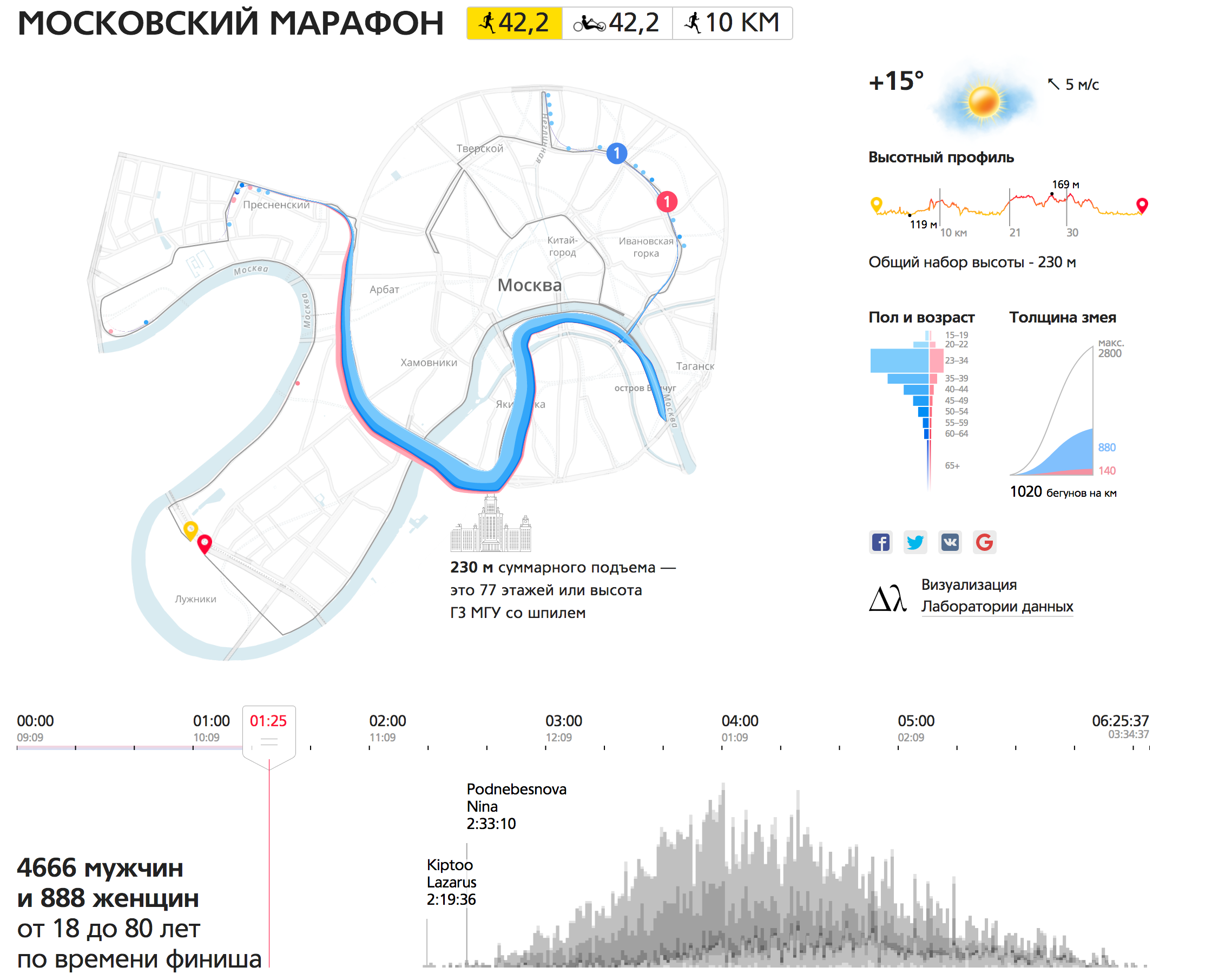
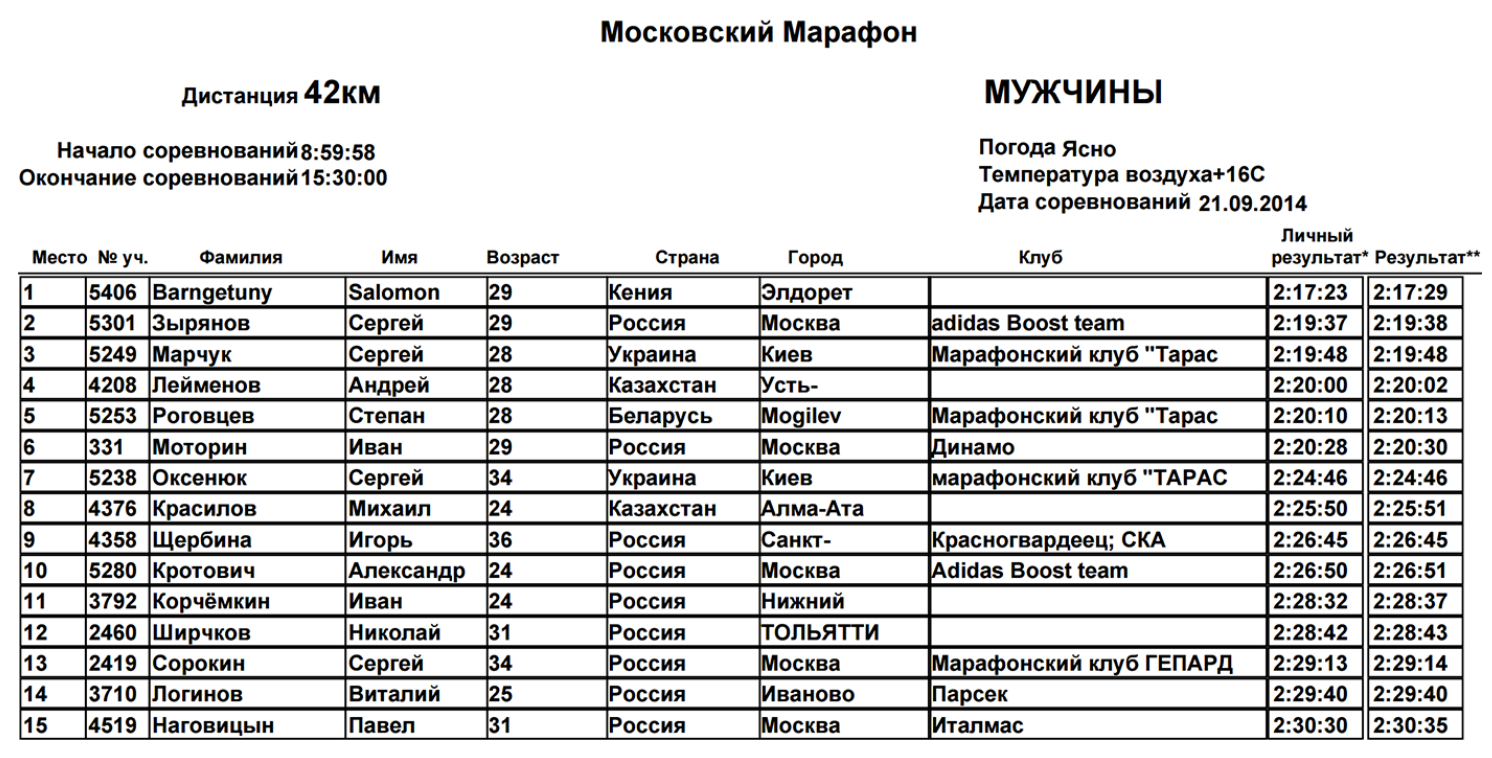
Why did you want to do something with this data? In 2013, I myself was preparing for the marathon and participated in the intermediate races. My first run inspired me a lot because it was a huge crowd of runners, of different ages, running communities, teams. The most interesting thing happens during the race, because it is a huge crowd that runs along the route, forms groups. Someone is trying hard, he is giving all his best, someone is doing it for himself, at a relaxed pace. When, after my first official race, we were given a dry table that did not reflect all that was happening, I wanted to make a visualization.
We have a crowd of runners running along an interesting route through the whole center of Moscow. At the beginning, the crowd is big and thick, over time it stretches very much along the highway. When the runners are almost at the finish, the tail just runs out of the garden ring.
There are many data dimensions on this visualization. The crowd is divided by color - men and women, and by age, which is shown by a shade of color - the darker the older. From this visualization, you can extract interesting mini-stories. For example, pay attention to the winner. Here, when he runs out to the embankment, he is somewhere in the 7-8th place, already on the embankment he makes a jerk, overtakes everyone and finishes first.
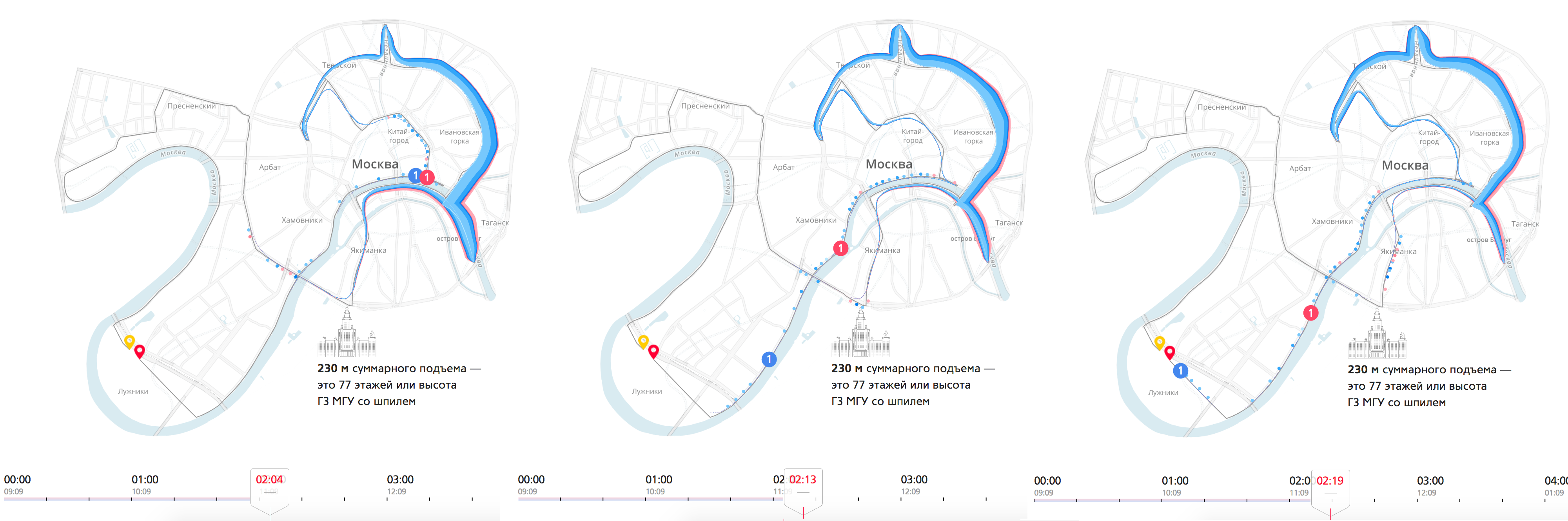
Look: we took a dry table, added intermediate results (10, 15, 21 km, 30 and 35 km) and got a huge layer of new information that it would be difficult for us to extract from the table. We got a complete and holistic picture of what was happening.
In addition to reflecting the positions of the runners, we added small charts, distributed the runners by gender and age. On the histogram of the finishes, you can see how the participants distributed according to the finish time, find those with whom you ran together. There is also a list of participants with the ability to filter (filters affect the main visualization). And the dynamics of the weather throughout the race, the weather for marathon runners - this is very important, directly affects the result.
Question to the audience: Why is this visualization cool?
Answers:
- You can watch in dynamics
- It becomes visible new information that was not in the table. For example, a jerk on the embankment
- Map and python, due to visibility, create a feeling of simplicity and integrity of the story, of what really happened. This is not an isolated set of graphs, but a holistic display of reality.
Comparison of tanks World Of Tanks
There are players who move from level to level. At each new level, the properties of tanks are improved, they become more armored and powerful. The main characteristics of the tank are attack, defense and speed.
Here is a diagram devoted to the properties of tanks that was sent to me by one of the game lovers for analysis:
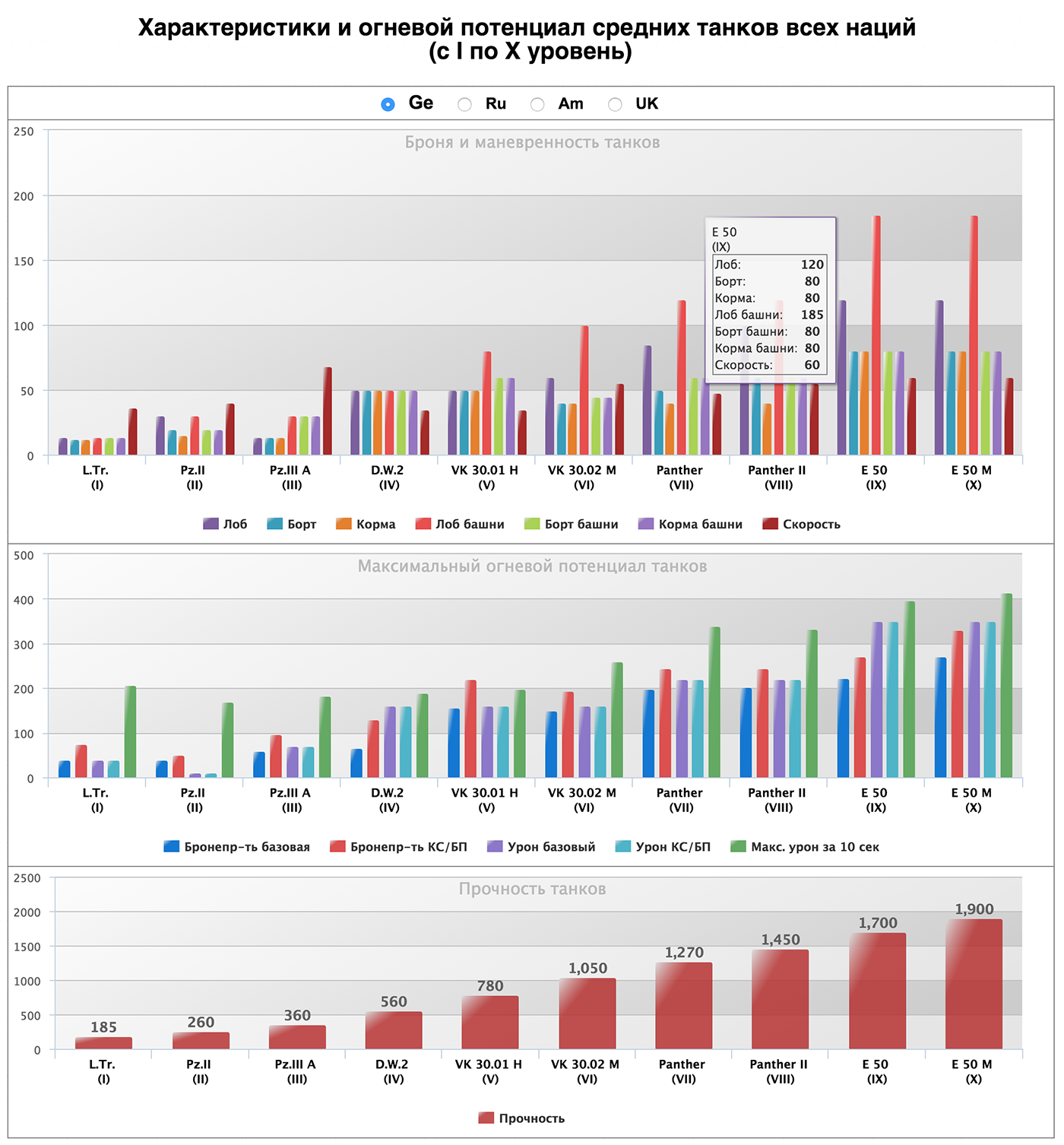
And here is the visualization we made in the laboratory:
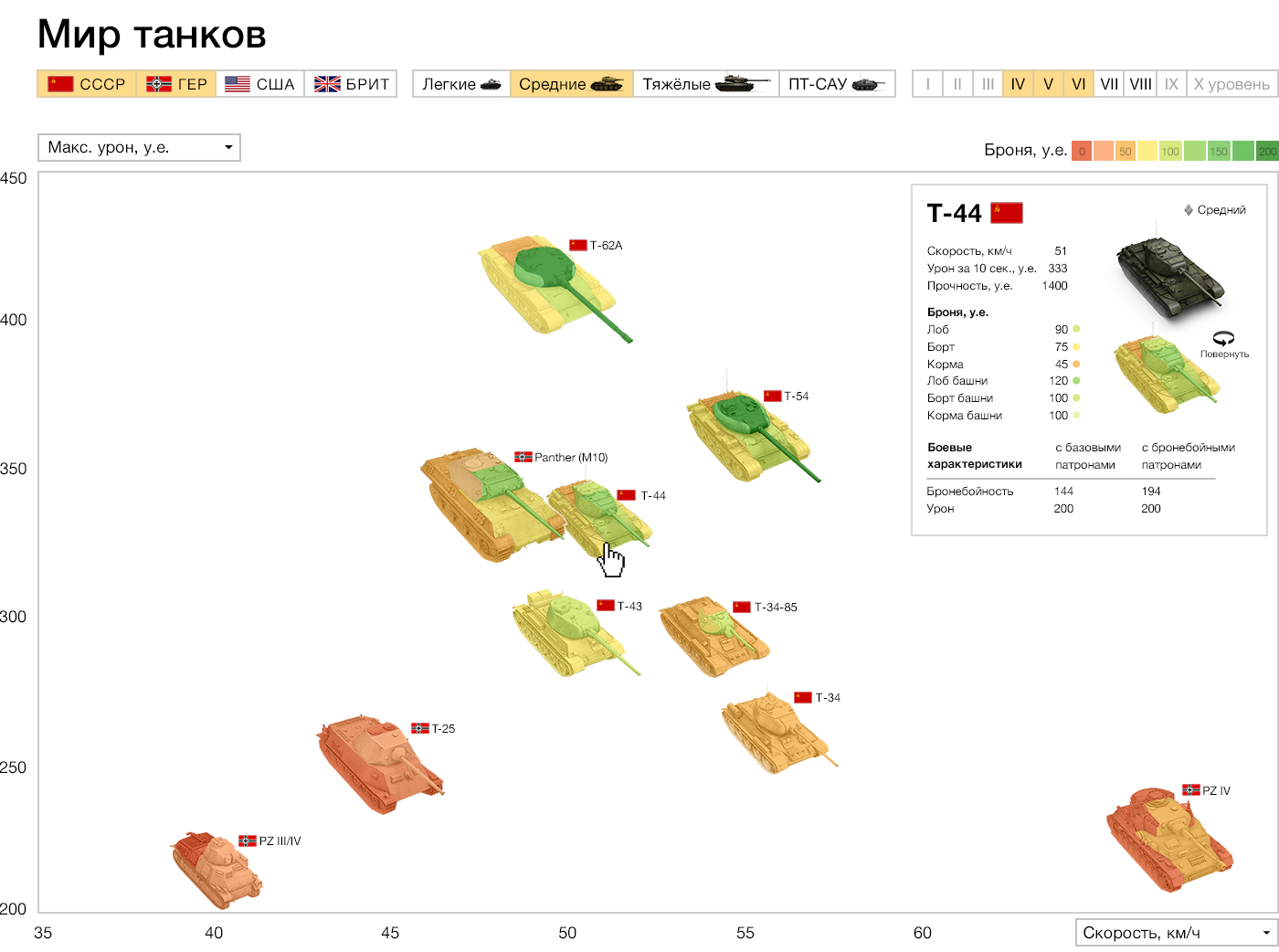
Horizontal speed, attack in 10 seconds of vertical, armor is shown in colors directly on the image of the tank. More detailed specifications and a real click-through image. Above are filters by country and tank class. Instead of dry diagrams, we look at the size, shape of the tanks.
Question from the audience: But at the same time, not all parameters are always visible. On your version, unlike the first one, you can’t find out which tank is cooler at first sight
If desired, you can turn on all tanks of all levels. But we intentionally cut the standard look so as not to clog the screen, and not to impair perception. By the way, by the way, not all of them were also the initial version, but only ten in some unknown way selected tanks - one for each level. The main conclusion that can be made there - with increasing levels, indicators are growing, the most noticeable deviation - in one of the tanks, one of the indicators did not increase. On our visualization, you can compare tanks of one and neighboring levels.
Comment by Ilya Ruderman, WOT player:
- It takes three to four days to pump the first three levels. But to pump from the fourth to the tenth, you need to spend a lot of time. You value your time, so you need to know as much as possible about the next tank in the development tree. The game has a large audience, and the slightest changes in the parameters and characteristics of tanks greatly affect the gameplay. Therefore, players pay a lot of attention to the characteristics. The work is cool. She has great potential, but lacks several critical parameters.
Question to the audience: How good is this visualization?
Answers:
- Filters and parameters do not pile on a huge pile of switches, but are neatly spaced and built into the visualization. There is little visual noise, and each element helps perception, but does not interfere
- On the first chart about tanks, we compared all the capacities of all tanks. This is not very useful since if the tank is weak, what is the point of comparing it with the strong? In your version, you can easily filter them and receive only important information.
- A motivated player will study the first schedule, but your visualization has the potential to attract an ordinary player who is just interested.
The rhythm of life in big cities, Jawbone
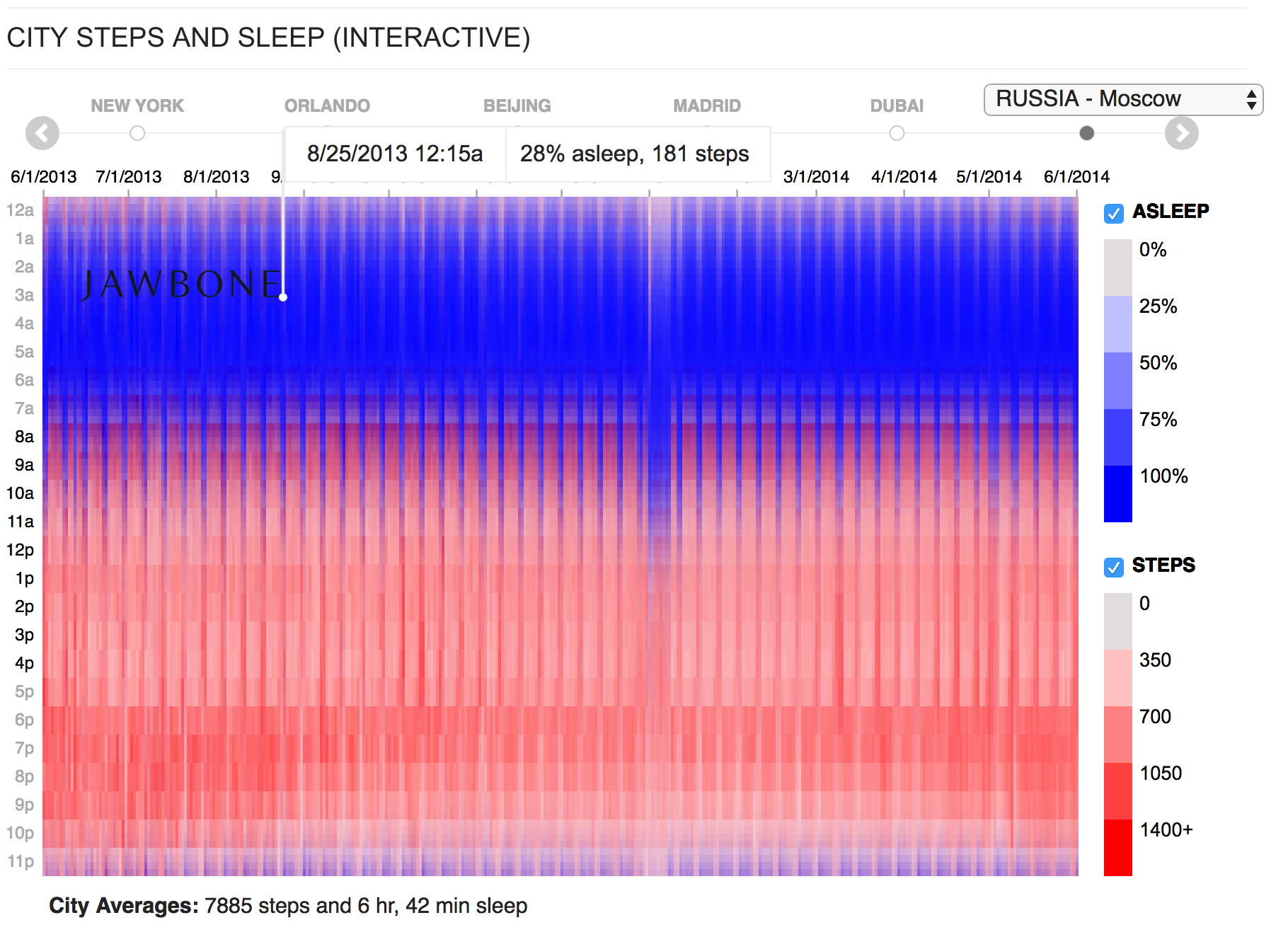
The diagram shows user activity in different cities. Horizontal - 365 days of the year, vertical - a day with a breakdown of 15 minutes. This is ... 365 x 24 x 4 = ~ 35 thousand data cells x 2 parameters: sleep and steps. It turns out ~ 70 thousand numbers, which are reflected in one picture, 45 such pictures for 45 cities. These are averaged numbers for all users, imagine how much data was in the input!
What catches your eye? Chopsticks - the weekend, everybody sleeps. Noticeable subsidence January 1 - celebrate the New Year. On the original visualization, a few seconds are loaded every year, this eliminates the possibility of quick comparison. We took the original graphics and made it so that the data is downloaded immediately and it is convenient to compare.
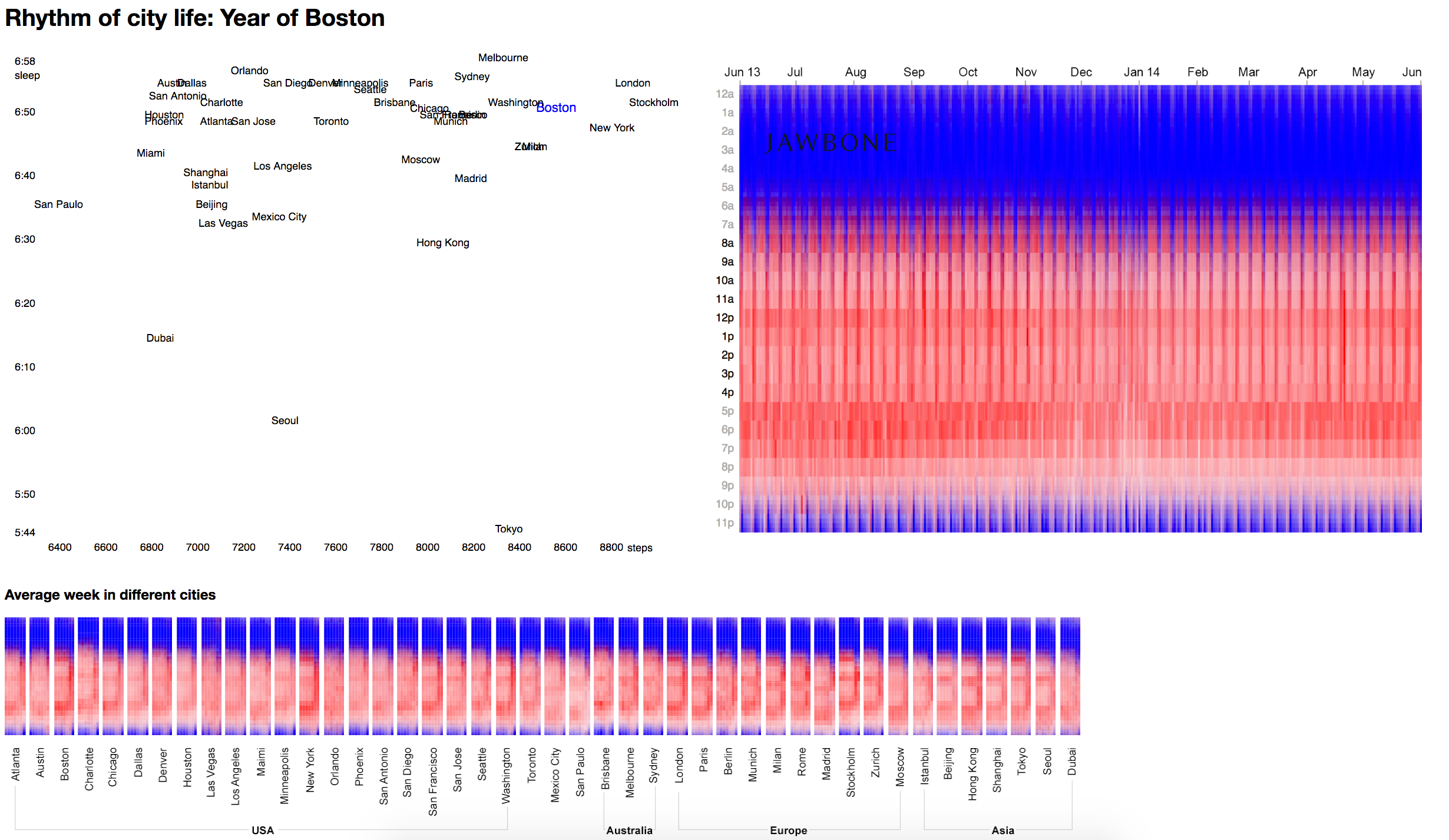
Try our version. See what happens in the summer in Stockholm and London, how much more regular London is. They only have changes for the New Year. Now let's compare London and New York: there are long weekends and weekends in New York. Now look at Madrid, how their siesta after an hour of the day changes the whole picture. And now Miami or Phoenix, where everything is very even and there is not much difference between weekends and working days. In Dubai, for some reason, everyone sleeps in June. Perhaps this is the influence of some kind of religious fasting.
The bar below is the average week of one city. It shows, for example, that Charlotte in the United States is strongly knocked out at the start of the day. And the evening in Tokyo smoothly turns into night.
You can see that New York, Washington, Boston have an actively expressed week and weekend with a lot of activity, and on weekdays everything is pretty sluggish.
An additional level of instant comparison works well with the ability of our eyes to notice changes in similar pictures (Tufty writes about this). Visualizations are cool on their own, you can combine them with other wireframes, connect interactively and get a more powerful communicative effect.
NYT Basketball Visualization
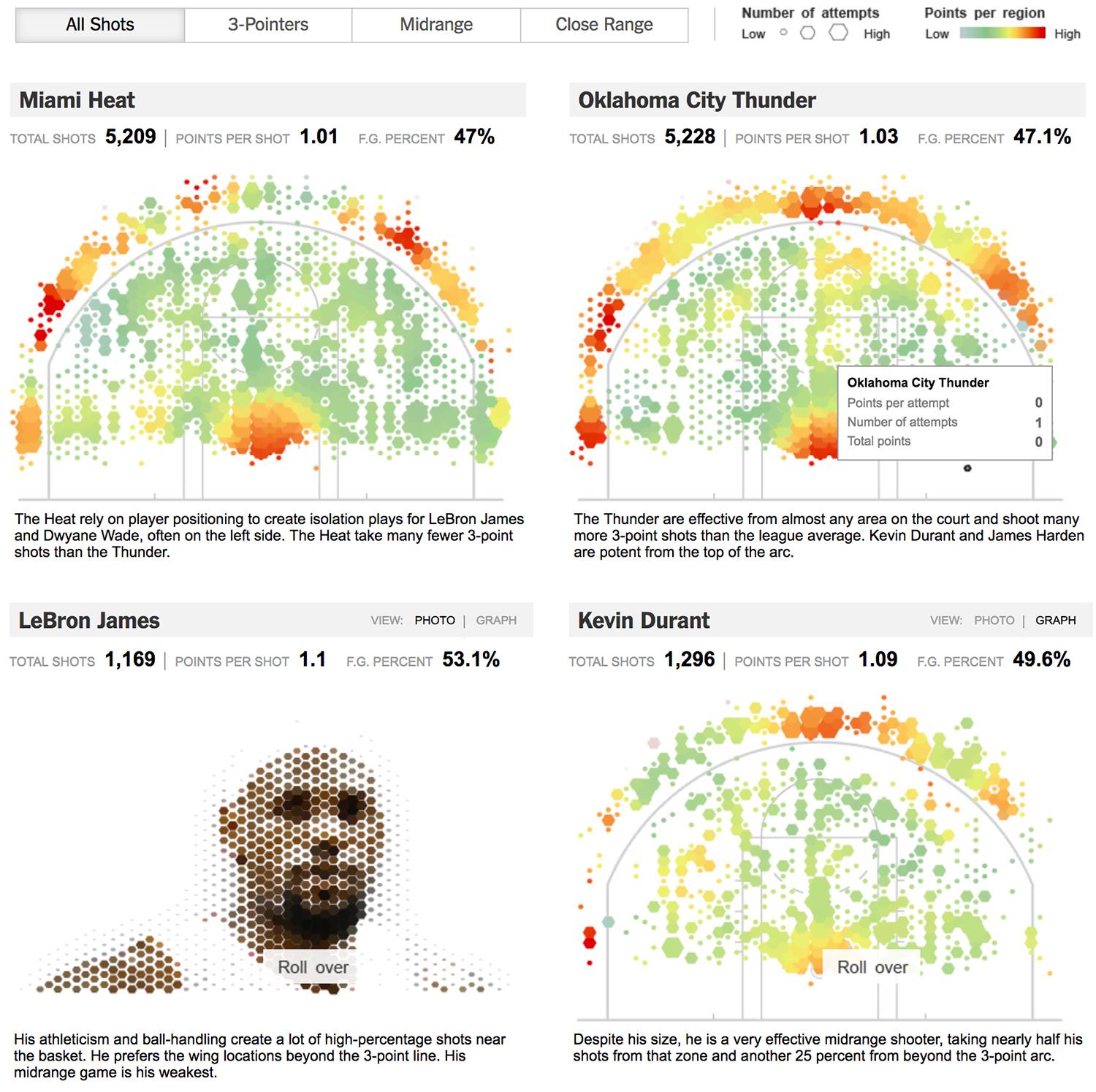
The basketball court and the ring are shown here, and on top is the number of shots from different points of the court and the effectiveness of the shots. The size of the hexagon is the number of throws from a position, the color - the redder, the more hits from here. Statistics are collected for two different teams, there are also summary data: total number of shots, points per shot, percentage of hits.
When two pictures are nearby, we can quite easily compare two teams. We can notice some patterns: Oklahoma City Thunder throw three-pointer from the center, but Miami Heat - no. Their performance there is very low. But the most interesting part begins when we turn to the statistics on players. [When you hover over the images of the players, they scatter and turn into diagrams, enthusiastic sighs from the hall.]
The comparison by players is more interesting, here the wow effect does not interfere with perception and fits very naturally into the graphics. Kevin Durant is a three-pointer special, and LeBron James throws out from under the ring. You can compare and see the technique of different players and get an understanding of the style of the game. You can still see who is left-handed and who is right-handed
Question from the audience: How do they record all this?
Now more and more sports analysts and television channels are beginning to understand the power of visualization and are spending resources on collecting and aggregating such data. As a last resort, you can find records of all goals, and create a dataset manually.
Cash flow visualization
Next boring table, finances:

On the table are the constituent entities of the Russian Federation and their cash turnover by individuals / legal entities, residents / non-residents, cash disbursement / deposit and the amount of turnover. Central Bank analysts are not interested in operations at any given moment in time, but are interested in anomalies and outliers. For example, a sharp jump in cash withdrawals in some entity is a reason for suspicion of fraudulent schemes. We did color coding by anomaly. The internal algorithm calculates the percentage of anomalies and colors the area according to the index.

In the Novgorod region, some values deviate from the average, and we see this anomaly on the map. When we saw the anomaly, we can try to figure it out and fall deeper. The anomaly here turned out to be an extradition. These were large seizures from residents in March, May and January. This visualization does not allow you to dig even deeper, but already here we can watch the temporal dynamics of the season. It is unlikely that this is tourist activity, tourists will not rake nine billion rubles. Perhaps this is an occasion for trial. This is not real data, the institute generated a conditional set for test work.
Here's a classic example of a combination of formats. When we work with a map, the chart comes to life in dynamics. And when we work with the schedule, the map changes, we can see that in April 2013 there was an anomaly in the Tomsk region.
At the customer, all this information is stored on 30 sheets in Excel, and to see such a picture they travel through these 30 sheets. There is a special person who in this exel is guided and digs out the necessary information. The advantage of visualization is that you can pack a huge amount of data into a concise form.
Map of Venice A great example of the relationship between data and color on a map. Color fill areas are areas that are flooded at different frequencies. Some areas are flooded more often. An interesting combination of two data types. On the one hand, this is the water level, and on the other, on the same scale, the frequency of flooding. In addition to filling areas, there is another semantic unit - at home. Light shows buildings that are weakly affected by flooding, dark, which are destroyed very much
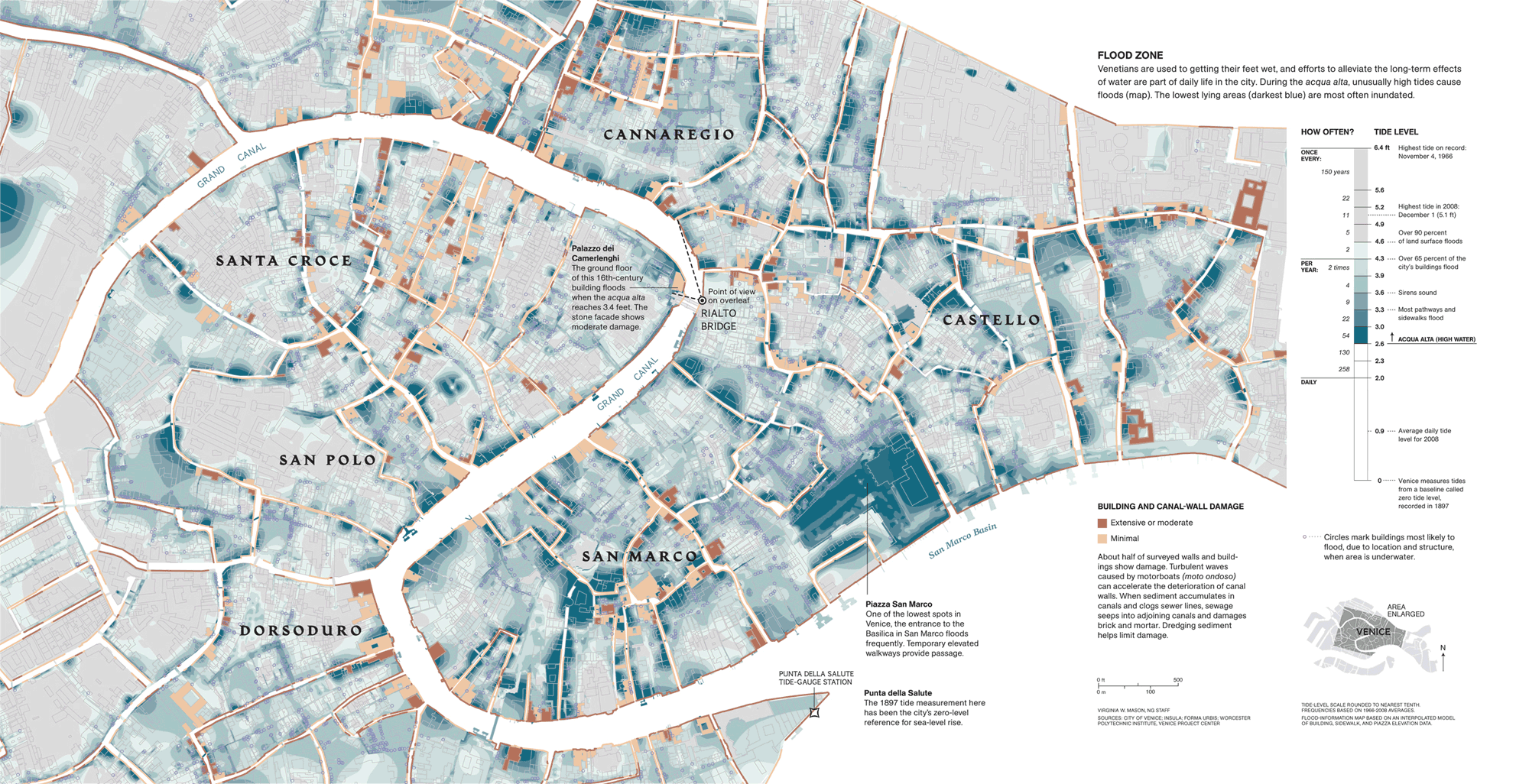
Who will marry whom? The grid on the background is made up of different professions. On the left are more masculine professions, on the right are more female professions. When hovering over a profession, we see communication lines of different thicknesses that show how often people from this profession marry people from another profession. Circles mean that people get married inside one profession. The color of the gradient shows the floor inside the pair. Firefighters marry kindergarten teachers. Designers marry sales managers. Such graphics are in no way tied to the classical formats of information representations. But it takes into account the features of the data that underlie visualization to the maximum and makes super-visual what we want to show - the frequency of the marriage. It turns out very minimalist. And this joke is really interesting to study.
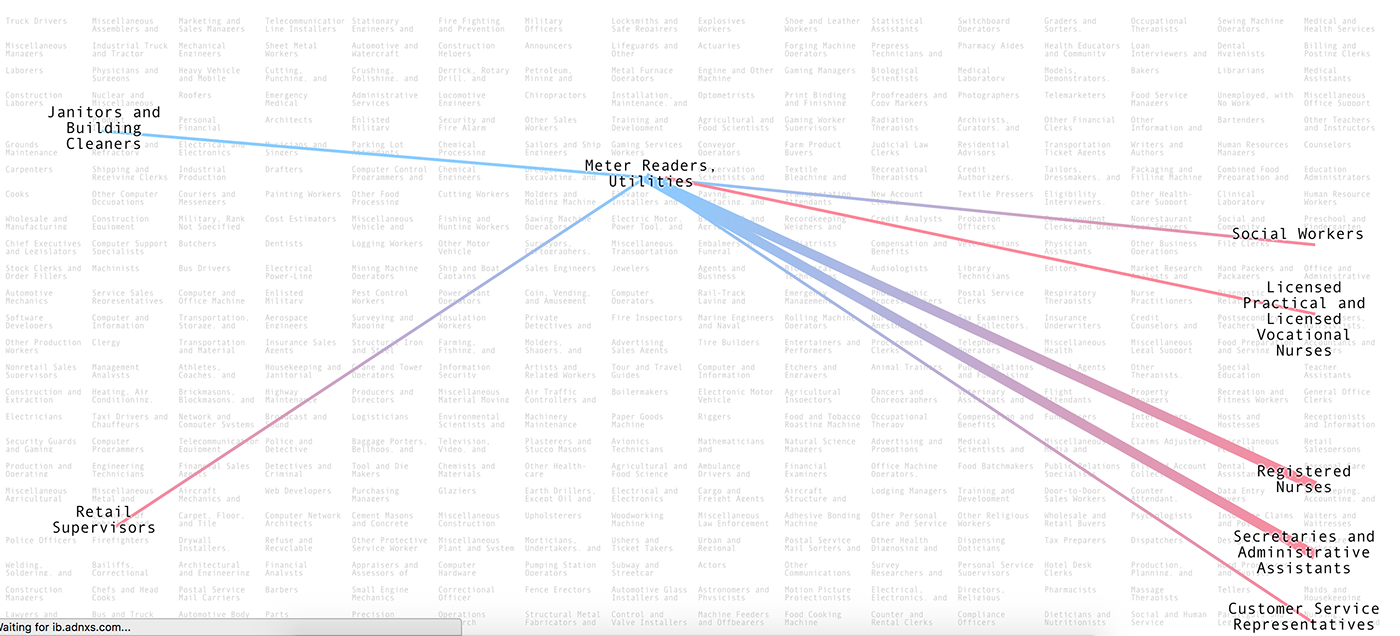
Question from the audience: And in what way are professions scattered horizontally from more masculine to more feminine?
Most likely some tricky algorithm, distributing depending on the proximity of professions, so that the final picture looks good.
Classy visualization, conclusions
Question to the audience: All the examples are very different. What unites them and makes visualization cool?
Answers from the audience:
- The data does not cease to be uninteresting and lively from the fact that the topic is dry and uninteresting.
- When the visualization is done well, I want to explore it and ask questions.
- When multidimensional data is connected, a new meaning is born from their comparison.
...
Here is my understanding.
In an interesting visualization, in which there is a sense of “magic”, there are always many dimensions on a flat screen. We use different expressive means and methods and techniques to make perception convenient.
At the same time, when we show all these measurements, we remain clear. This should not be a mechanical coding "yeah, I have the property height, I will encode it with color, I have the property length, I will encode it with hatching." Try to think what real things are behind the data. If this is the armor of a tank, draw directly on the tank where it is located. Choose coding methods as close to reality as possible, closer to intuitive ideas about colors (boys - blue, girls - pink). The more intuitive coding you use, the easier it will be to perceive the work, even if many data dimensions are sewn into it. There will be no need to make signatures and guides “How to read the schedule”, huge legends will not be needed. Perhaps a minimalistic legend will itself become a diagram. At the same time, the schedule lives well without a legend.
This does not always work. Sometimes a new type of visualization is better suited, which is not immediately clear, but solves the problem very well. You can do that too. But ideally, one should strive for clarity and intuition.
When these principles are followed, a certain general picture is born, we can glance at the data as a whole, without switching over thirty tabs, without switching the cities of the country, we can see a complete picture of what is called a big picture. In the big picture we see patterns, anomalies, communications. It is easy to make comparisons. If we do not have a big picture, but only five scattered graphs, the benefits and effects of visualization will be completely different. After we saw the pattern, we can go deeper and start exploring (cash flow, city jawbone). See the whole picture, notice patterns and go deeper and answer questions about this pattern. This is power, and the key to cool visualization.
Algorithm Δλ
We looked at cool examples of visualizations, now let's talk about how our process works inside the laboratory. Most of the results of working with data are completely different from the examples we looked at. The word “visualization” usually immediately presents dashboards, scattered graphs, pie, bar charts, and sometimes sans.
I have a hypothesis of why this happens. Analytics, working with data and visualization is often perceived as a boring and dreary business, because the data is stored in tables or databases. And these data sheets and tables look the same. The same problem with standard types of visualization.
All data is unique. They are alive, something always happens inside them - objects interact, their properties change. If we put this life in the table, we fix it with a dead slice. When we try to visualize this slice, we get moderately informative, but rather boring, inanimate dashboards. Such a straightforward approach, although the most logical, but leads to dry and primitive results.
Although it is not always as simple as it seems. There are a lot of nuances, and analysts get a lot of money to work correctly with the display of data.
To create the “sense of magic” that we talked about in the first part, you need to turn on your imagination and go beyond the tables. You need to imagine what is behind these tables, what generates this data.
Data reality- this is a set of processes that occur in the physical space and generate
data.Here, Katie Ledeki breaks the record and wins Olympic gold in the 800 meters freestyle: This is a great example of how, by connecting the reality of the data, it is possible to visualize what actually happened. How a boring table turns into a thing from which it is difficult to take your eyes off. And another example, but from a different area. Map of Italy's specialties Antoine Corbino: This can hardly be called data visualization, but it is a good example of not being afraid to take real objects, as in the example with tanks or a basketball court. There is no need to be afraid to place everything on a map, to tie it to some real physical space.


We try to use the real “landscape” and transfer elements from our physical reality to visualization. This greatly enhances visibility and brings us closer to the big picture.
Determining the reality of the data, including imagination and imagining what is behind the dry tables, having seen this picture, we take a step to show the essence of the phenomenon, and not a dead and dry cut. The reality of data helps to see the nature of data behind uniform tables.
A data particle is a call from the micro level, it is an elementary data brick that allows you to unravel a tangle of data of any complexity. There may be several, but most often one. It is an analytical work, to find an entity, a unit that will allow you to work with data as flexibly as possible.
Represent the reality of data and isolate a piece of data - The first step of our Δλ algorithm. Introduce the whole picture, and find the smallest brick. Data turns into a collection of particles that we can group in any convenient way.
Next, we move to the two-dimensional plane of the screen and begin to lay out the particles on it as you like. This is the second step of the algorithm - selection of the frame and visual atom.
A wireframe is a way of organizing two dimensions of a screen, which conveys the properties of a data particle due to its position on the screen and changing this position. The framework distributes the data particles on a plane so that they can be compared with each other.
Visual atom- a visual embodiment of a data particle on the screen. Visual atoms show data parameters using color coding, size, shape of objects and other visual attributes.
Why are these difficulties with frameworks and atoms? The ability to separate spatial measurements and visual, as it were, intrinsic properties of a particle significantly increases the flexibility of our approach to data display. Any visual atom can appear on any frame. Even if we consider a small set of basic frameworks that are familiar to everyone, in combination with graphic primitives (point, figure, line ...), we get an impressive table of possible visualization formats:

Why don't I start the lecture with this wonderful table? It seems to me that this table encourages you to follow the standard path and choose a visualization from a large set of graphs in order to push our data into it. And when we try to shove the data in a predetermined form, we do not take into account their essence, their features, and we lose a lot. I want us to dance from the data and therefore I pay so much attention to the first part. Data is more complex and interesting than all standard formats. In general, I have a feeling that the data has its own idea of what form it is better to be in :) That for each data set, taking into account their internal features, there is its own most suitable way of presentation. I do not want you to perceive this table as a tool to cram data into some format.
The third step of the algorithm, after we dumped the data on the frame. We are starting to add interface elements, filtering tools. Perhaps we got too many points and they ripple, then we can merge the points together into shapes. After we have distributed the data on the wireframe, we can add controls based on the scenarios of how we want to work with the data.
The algorithm is laid out very cool on the example of visualization of the losses of the Second World War: The action of the algorithm will now seem to occur before our very eyes, in real time (turn on the video).
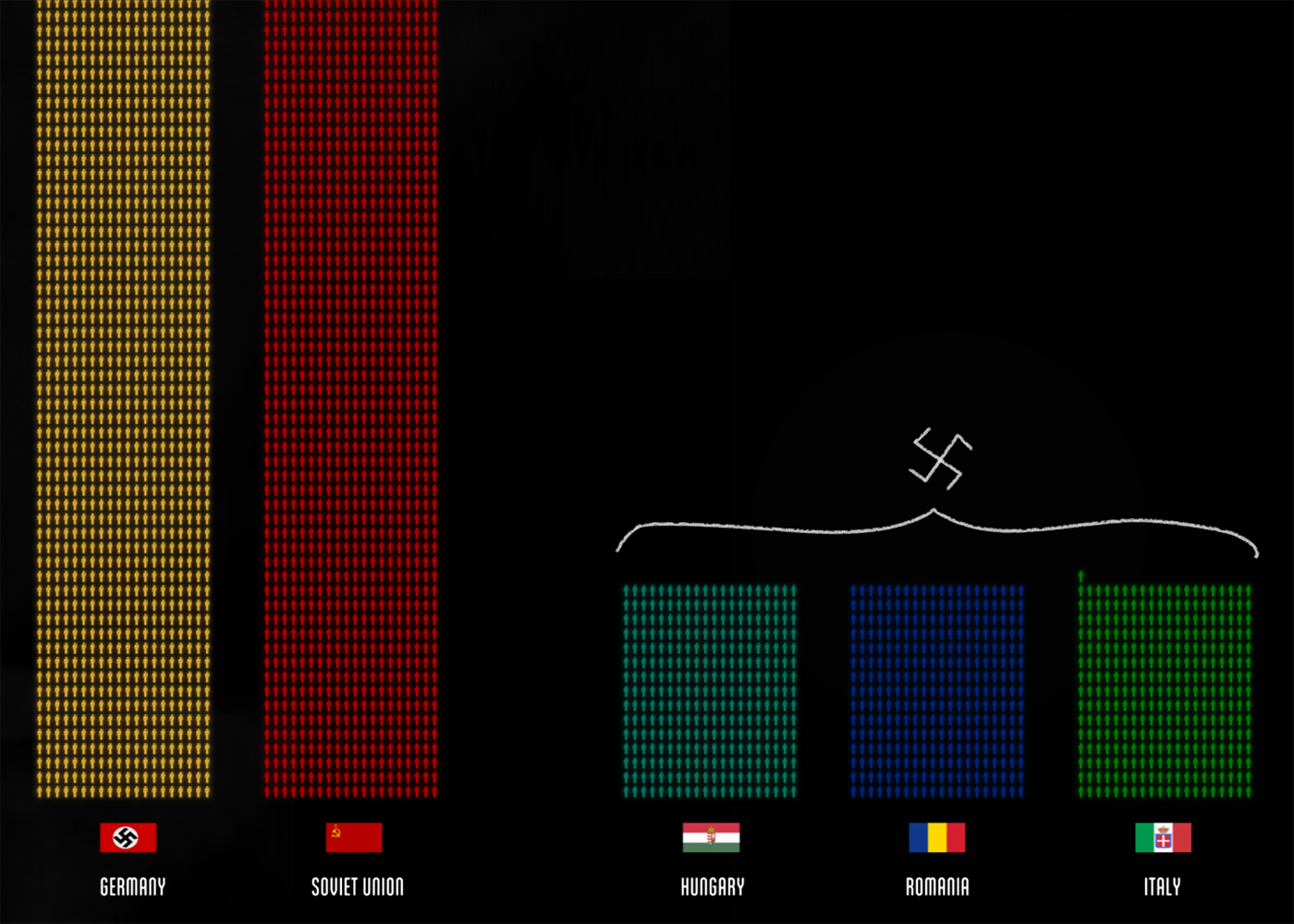
The video begins with an introductory word - this is the reality of the data. Now they have chosen a piece of data - a civilian and a soldier. The first step of the algorithm has been completed. The visual atom is a human figure in which 1000 lives are encoded. Moreover, two different figures - a soldier with a gun and an unarmed civilian. From individual little men a lot of data is obtained. At some point, all the data is on the time axis. We see how the number of losses increases over time and how it is distributed in different battles. This is the second step, we throw the visual atom into different frames, we see different pictures.
Another grouping method is applied to the same particle - by colors, which mean other meaningful parts. It turns out that the data particles are the same between each other, but we can easily compare them with each other and draw conclusions. Pay attention to the scenario move - the idea is repeated that all these are living people, and not points on the chart.
After that, the points turned into columns (third step), because the scale is too large. In this example, as on the famous Minar map , the power of a holistic picture is manifested, in which we see every point, every death. This resonates much more than the dry figure of 87 million, or bars.
***
Read more about the algorithm in the "Complex Data Visualization Algorithm" .
I invite everyone who has read the note to the end to a course on data visualization . On the course, we further analyze the concepts of reality and data particles, get even closer acquainted with wireframes, visual atoms and the principles of working with them. In addition, the course participants learn visualization tools - the Scoreboard and the d3.js library, and on the last day of the course they create their own visualization under the guidance of teachers. To activate the discount of 5 thousand rubles. , when recording in a comment, make a note "from Habr."