4 reasons to become a Data Engineer
Hello, Habr! At the moment, a huge bias has formed in Data Science towards data scientists, even those who are not connected with IT now know about this profession, and new vacancies appear daily. In turn, data engineers do not receive the attention that would correspond to their importance for the company, so in today's post we would like to correct this injustice and explain why developers and administrators should immediately begin to study Kafka and Spark and build their first pipeline.
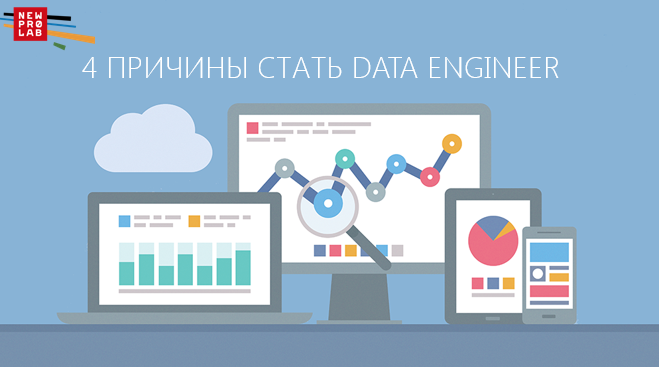
Let's look at a typical working day of a data scientist:
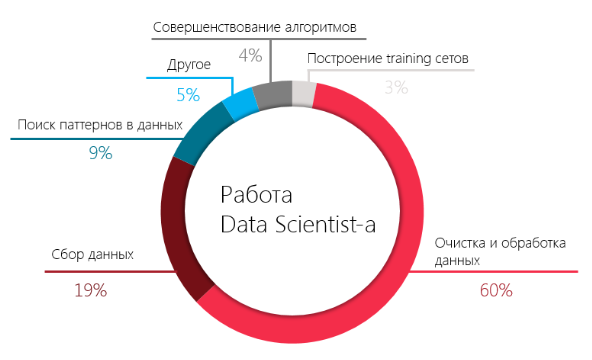
It turns out that about 80% of his time, a data scientist spends on collecting data, preprocessing and cleaning it - processes that are not directly related to his main responsibility: searching for insights and patterns in the data. Of course, the preparation of data requires the highest level of skill, but this is not data science, this is not why thousands of people today are eager to get into this industry.
That is why companies should free data scientists from the least pleasant part of their work and delegate data preprocessing to an engineer, whose presence in the data science team will, firstly, allow data scientists to do what they really love - building models, which in turn will prevent their potential departure from the company and attract the most talented. And secondly, the efficiency of data scientists will increase, since they will spend many times more time looking for valuable insights, which will naturally benefit the business.
Also, do not forget about the principle of garbage in - garbage out: if low-quality data is supplied to the models, then it makes no sense to expect an adequate result from them. Therefore, in order to maximize the effectiveness of the data science department of the company, it is necessary to hire data engineers who, unlike data scientists, specialize in organizing the process of collecting, cleaning and pre-processing data.
Here is what Big Data Engineer at Mail.ru Group thinks about this, Anton Pilipenko: “At the moment, most companies have learned to store a large amount of data and build different types of models on their basis. However, often, issues of efficient storage and processing of accumulated data are not given sufficient attention. As a result, here and there constantly there are questions about sizing, application scaling, streaming and near-realtime processing. As experience shows, the division into Data Science and Data Engineer specialists did not appear from scratch. The Data Engineer is primarily an engineer who understands well what and why he is doing, how it works “under the hood” and which architecture “will not take off”.
It's no secret that today the profession of data scientist is becoming more and more popular, thousands of students around the world want to get a job in this industry, and many mature specialists from other areas are changing their specialization in favor of data science. The reason is simple - high salaries, the solution to analytical problems and the growing unmet demand for data analysts. All this can result in a large number of unskilled personnel who have come to the trend area without having sufficient knowledge of programming and statistics, while it will be difficult for analysts who are really interested in building models to stand out among this mass.
Now, from the same point of view, let's take a look at data engineers who have the opposite situation: at first glance, the duties of a data engineer look less interesting than that of a data scientist (which is naturally not the case), so hundreds of resumes do not fly to the post to employers who are looking for a good data engineer, although the salaries of data engineers and data scientists are at about the same level ( 90 and 91 thousand dollars a year, respectively, in the USA) People need to see the result of their work, and best of all, customer and business satisfaction. The easiest way to enjoy your work is to learn about hundreds of new customers by building a model for creating personalized offers than from cleared data, so it’s hard for most to appreciate the importance of data engineers, who are no less than data scientists who contribute to the final result.
Today, almost everywhere, the question arises more often about whether certain professions will soon be replaced by artificial intelligence. With regards to data engineering, many are of the opinion that the process of collecting, processing and cleaning up data is routine and can be easily automated, so the profession is unpromising. However, this opinion is incorrect, since preparing data for analysis is a real art, and the approach that worked with one dataset may not be suitable for another dataset at all. Machines are not yet able to independently adapt to the data; in the near future, a human engineer - data engineer will still be engaged in their configuration.
Moreover, the duties of a data engineer include an even more complex task than data preprocessing, the task of building stable pipelines that make data accessible to all users within the company. Only thanks to the data engineer is the data scientist provided with high-quality datasets in a convenient form and at the right time, this is the indispensability of the data engineer. The way it affects business processes and the success of the company can be seen with the naked eye.
From this point of view, the professionals also agree: Senior Software Engineer at Agoda, Artem Moskvin, says: “A data engineer is the one who makes all the gig you heard about possible. Work with data can be divided into 2 parts: engineering and research. However, in order to make the second possible, you need to work hard on the first “, and according to Data Engineer at E-Contenta, Andrei Sutugin:“ In the world of data analysis, not everything is as rosy and beautiful as it might seem after solving “titanic "On kaggle. In order to proceed directly to the analysis itself, it is necessary to do a titanic work, but in order to “streamline” the collection and transformation of data, even more effort is required. Unfortunately, in the world of "big data" there are no "silver bullets", and an abundance of tools and frameworks can turn your head. "
Many people who want to build a career in IT, after 1-2 courses of technical universities with furious courses in mathematical analysis and probability theory, give up, believing that without an advanced mathematical background they will not be able to find work, even though they write good code . In this regard, data engineering is a great opportunity to start a career in the field of working with data for people who have only a basic understanding of machine learning, but are interested in database development and management. Thus, such work, of course, is more suitable for software engineers, architects and database administrators.
According to Nikolai Markov, Senior Data Science Engineer at Aligned Research Group LLC: “Why do Data Engineering? I believe that this is a logical way into the field of data analysis for people who are able to program and have experience in the development industry. The fact is that people are extremely rarely deeply interested in both of them - at the same time, serious knowledge of mathematics and deep computer science in one person is almost never encountered. Therefore, let us leave to mathematicians what they do best - research, models, and graphs, and we’ll think about what needs to be done in order to get a finished working product from an analytical idea? ”
Newprolab on November 13 launches the Data Engineer program, where participants will create stable data processing pipelines from collection to visualization for 6 weeks, learn and hone their skills with the following tools: Divolte, Kafka, ELK, Spark, Luigi, Sqoop, Druid, ClickHouse, Superset, Storm, which will be combined into one large and stable pipeline. Learn more about Data Engineer .
Soon, no company can do without a Data Engineer
Let's look at a typical working day of a data scientist:
It turns out that about 80% of his time, a data scientist spends on collecting data, preprocessing and cleaning it - processes that are not directly related to his main responsibility: searching for insights and patterns in the data. Of course, the preparation of data requires the highest level of skill, but this is not data science, this is not why thousands of people today are eager to get into this industry.
That is why companies should free data scientists from the least pleasant part of their work and delegate data preprocessing to an engineer, whose presence in the data science team will, firstly, allow data scientists to do what they really love - building models, which in turn will prevent their potential departure from the company and attract the most talented. And secondly, the efficiency of data scientists will increase, since they will spend many times more time looking for valuable insights, which will naturally benefit the business.
Also, do not forget about the principle of garbage in - garbage out: if low-quality data is supplied to the models, then it makes no sense to expect an adequate result from them. Therefore, in order to maximize the effectiveness of the data science department of the company, it is necessary to hire data engineers who, unlike data scientists, specialize in organizing the process of collecting, cleaning and pre-processing data.
Here is what Big Data Engineer at Mail.ru Group thinks about this, Anton Pilipenko: “At the moment, most companies have learned to store a large amount of data and build different types of models on their basis. However, often, issues of efficient storage and processing of accumulated data are not given sufficient attention. As a result, here and there constantly there are questions about sizing, application scaling, streaming and near-realtime processing. As experience shows, the division into Data Science and Data Engineer specialists did not appear from scratch. The Data Engineer is primarily an engineer who understands well what and why he is doing, how it works “under the hood” and which architecture “will not take off”.
Data Engineer is easier to attract the attention of the employer
It's no secret that today the profession of data scientist is becoming more and more popular, thousands of students around the world want to get a job in this industry, and many mature specialists from other areas are changing their specialization in favor of data science. The reason is simple - high salaries, the solution to analytical problems and the growing unmet demand for data analysts. All this can result in a large number of unskilled personnel who have come to the trend area without having sufficient knowledge of programming and statistics, while it will be difficult for analysts who are really interested in building models to stand out among this mass.
Now, from the same point of view, let's take a look at data engineers who have the opposite situation: at first glance, the duties of a data engineer look less interesting than that of a data scientist (which is naturally not the case), so hundreds of resumes do not fly to the post to employers who are looking for a good data engineer, although the salaries of data engineers and data scientists are at about the same level ( 90 and 91 thousand dollars a year, respectively, in the USA) People need to see the result of their work, and best of all, customer and business satisfaction. The easiest way to enjoy your work is to learn about hundreds of new customers by building a model for creating personalized offers than from cleared data, so it’s hard for most to appreciate the importance of data engineers, who are no less than data scientists who contribute to the final result.
Data Engineers are virtually indispensable in the company
Today, almost everywhere, the question arises more often about whether certain professions will soon be replaced by artificial intelligence. With regards to data engineering, many are of the opinion that the process of collecting, processing and cleaning up data is routine and can be easily automated, so the profession is unpromising. However, this opinion is incorrect, since preparing data for analysis is a real art, and the approach that worked with one dataset may not be suitable for another dataset at all. Machines are not yet able to independently adapt to the data; in the near future, a human engineer - data engineer will still be engaged in their configuration.
Moreover, the duties of a data engineer include an even more complex task than data preprocessing, the task of building stable pipelines that make data accessible to all users within the company. Only thanks to the data engineer is the data scientist provided with high-quality datasets in a convenient form and at the right time, this is the indispensability of the data engineer. The way it affects business processes and the success of the company can be seen with the naked eye.
From this point of view, the professionals also agree: Senior Software Engineer at Agoda, Artem Moskvin, says: “A data engineer is the one who makes all the gig you heard about possible. Work with data can be divided into 2 parts: engineering and research. However, in order to make the second possible, you need to work hard on the first “, and according to Data Engineer at E-Contenta, Andrei Sutugin:“ In the world of data analysis, not everything is as rosy and beautiful as it might seem after solving “titanic "On kaggle. In order to proceed directly to the analysis itself, it is necessary to do a titanic work, but in order to “streamline” the collection and transformation of data, even more effort is required. Unfortunately, in the world of "big data" there are no "silver bullets", and an abundance of tools and frameworks can turn your head. "
Data Engineering does not require in-depth knowledge of statistics and probability theory
Many people who want to build a career in IT, after 1-2 courses of technical universities with furious courses in mathematical analysis and probability theory, give up, believing that without an advanced mathematical background they will not be able to find work, even though they write good code . In this regard, data engineering is a great opportunity to start a career in the field of working with data for people who have only a basic understanding of machine learning, but are interested in database development and management. Thus, such work, of course, is more suitable for software engineers, architects and database administrators.
According to Nikolai Markov, Senior Data Science Engineer at Aligned Research Group LLC: “Why do Data Engineering? I believe that this is a logical way into the field of data analysis for people who are able to program and have experience in the development industry. The fact is that people are extremely rarely deeply interested in both of them - at the same time, serious knowledge of mathematics and deep computer science in one person is almost never encountered. Therefore, let us leave to mathematicians what they do best - research, models, and graphs, and we’ll think about what needs to be done in order to get a finished working product from an analytical idea? ”
Newprolab on November 13 launches the Data Engineer program, where participants will create stable data processing pipelines from collection to visualization for 6 weeks, learn and hone their skills with the following tools: Divolte, Kafka, ELK, Spark, Luigi, Sqoop, Druid, ClickHouse, Superset, Storm, which will be combined into one large and stable pipeline. Learn more about Data Engineer .