Top 5 spheres of application of object recognition systems
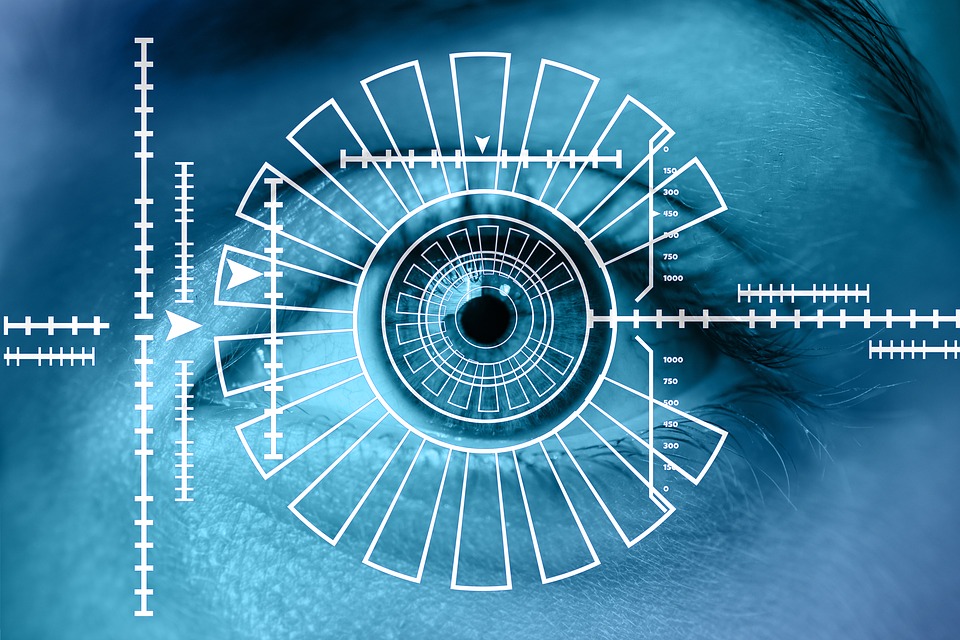
Attempts to teach various systems to see and understand the world in the same way as a person does, began several decades ago, but now these technologies have become so perfect that they are actively used in many areas of our life. Habré already has detailed articles on machine vision, neural networks and recognition algorithms, so we will not go deep and re-describe these complex technologies, we will talk about the practical use of these systems in the real world.
How it works? Briefly
What is a photograph for us, for the pattern recognition system, is just a collection of pixels with different color parameters. To teach the system to recognize individual objects in an image, it is necessary to provide it with a dataset - a set of thousands of images that indicate exactly where the desired object is located. For example, if we want the system to learn to recognize people in pictures, we need to show her many photos of people of different ages, in different poses and clothes, in different conditions. After such a workout, the system will be able to accurately recognize the person in the photos. However, another question arises: if for a system a photo is just a collection of pixels, then how does a neural network understand what exactly is depicted in the photo?
For the recognition of objects in the image using various methods, but one of the most promising is the method of oriented gradient histograms (HOG). The image becomes discolored, and then in blocks of 16x16 pixels, the system finds the direction of color change (gradient vector), builds a map of these vectors throughout the image, and thus a “snapshot” of object features that do not change depending on the position / position and lighting. The improved version of the algorithm is called CoHOG - it takes into account the boundaries of objects, that is, it recognizes the shape, not just the gradient vectors.
Toshiba has improved the CoHOG method, significantly improving recognition in low light - the traditional CoHOG, for example, does not do well with fast recognition in the dark when pedestrians are hardly visible in the light of headlights. The ECoHOG method (technology of histograms of co-presence of oriented gradients) determines a person due to additional analysis of the directions and sizes of his outlines, finding the head, legs, arms, shoulders. If the CoHOG simply isolates anthropometric outlines on the image (analysis “object boundary - boundary vectors”), then for ECoHOG the dimensions of the object's borders relative to each other are important.
Five key applications
Marketing
Pattern recognition is a promising direction in advertising and marketing. Neural networks are allowed to find out in a matter of hours things that, in search of which in other cases, a large team of professionals and weeks or even months of research are needed. For example, the Russian service YouScan, a social media monitoring system, tracks the mention of brands in social networks. And it does it not only in the text of posts, but also in photos, and also helps to draw certain conclusions about the product. With the help of pattern recognition in the photo, we found an interesting pattern , the search for which no one would have thought of: among animals, cats are more often found with Apple technology, and dogs - with the Adidas brand. This unusual information can be useful for advertising targeting.
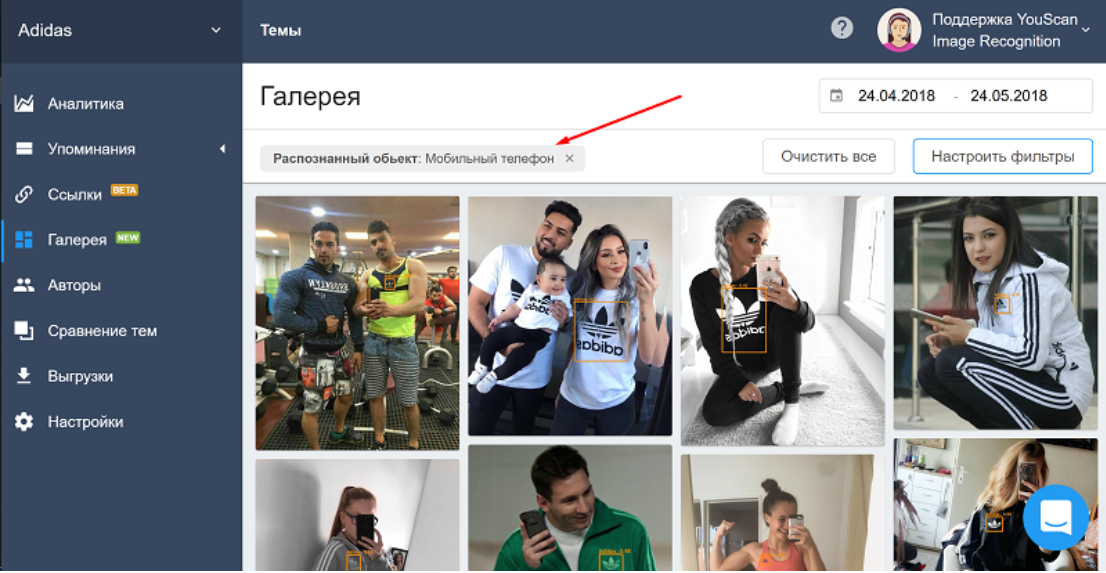
When searching for the Adidas logo, the YouScan service filtered photos with smartphones in the hands of the owners. Copyright: YouScan
CCTV
Pattern recognition on city video surveillance cameras is perhaps the most inevitable prospect of using machine vision. Since 2017, a smart video surveillance system has been tested in Moscow to identify criminals in crowded places. A technology from the Russian company NTechLab is connected to the city network of cameras, which has already helped to detain several dozen offenders. In China, such a video surveillance system is capable of recognizing not only faces, but also car and clothing brands in public, which can later be used by marketers for their research.
The video shows the real work of SenseTime pattern and face recognition.
The medicine
Pattern recognition has already become a real breakthrough in medicine - in many cases computers notice things that even the most experienced doctors miss. They act as original assistants, whose “technical” opinion confirms the hypothesis of the doctor or gives reason for deeper research.
In Russia, software systems are being developed for diagnosing cancers in CT, MRI and PET images. To do this, thousands of tagged pictures are run through the neural network, after which the recognition accuracy of new pictures increases to 95-97%. Among others, the development of such a platform is carried out by the Department of Information Technology of Moscow, using the open library Google TensorFlow.
The Google-created Inception neural network analyzes a microscopic study of a lymph node biopsy in a search for cancer cells in the mammary glands. For a person, this is a very long and laborious process, during which it is easy to make a mistake or to miss something important, because in some cases the image size is 100,000 x 100,000 pixels. Inception neural network provides sensitivity of about 92% versus 72% at the doctor. The neural network will not lose sight of all suspicious areas of images, although false positives are allowed, which the doctor will later filter out.
Cars
Object recognition in cars is a necessary part of ADAS (Advanced driver-assistance systems) security systems. ADAS can be implemented as complex means, like radar and infrared sensors, and with the help of a monocular camera. In the last articlewe have already said that one video camera is enough for a real-time car to recognize pedestrians, signs and traffic lights. However, such recognition "on the fly" is a very resource-intensive task, which requires a specialized processor. Toshiba has been developing a series of such processors for several years. They build a three-dimensional model based on a moving image from a single camera, and thus notice unknown obstacles on the road. After all, if the neural network is trained to recognize only people, markings and signs, then a tire or piece of fencing lying on the asphalt will not be recognized and regarded as a danger.

Visconti processors highlight zones in the image, classify them and help the autopilot or ADAS to make a decision. Source: Toshiba
Drones
In drones, object recognition is used for both entertainment and scientific purposes. In 2015, a lot of noise was made by the Lily copter with automatic engine start when tossing and the owner tracking function. Lily aimed the lens at the owner, regardless of the trajectory and speed of its movement. True, this function of Lily had nothing to do with image recognition, since the drone was not so much watching the image of a person as it was at the control panel that was put on the owner’s hand.
Image recognition drones are also used for more serious things. For example, the Norwegian company eSmart Systems has developed intelligent solutions for power grids. As part of one of their projects, Connected Drone, drones are used to troubleshoot power lines. Trained in recognizing the elements of power grids, they check the integrity of wires, insulators and other parts of power lines. This is especially important for quick localization of the fault, when the power supply of a city or an enterprise depends on the line. Considering that power lines are often built in hard to reach places, it is much more effective to send a brigade of drones to search for malfunction somewhere in the taiga or in the mountains than to send a brigade of people.

Drones eSmart find elements of the energy infrastructure and in the event of damage, mark the object, leaving a warning to the operator. Source: eSmart Systems