
Antifraud systems in popular mobile trackers: AppsFlyer, Adjust, AppMetrica, TMC, Kochava
At the end of last year, I wrote on Habré what solutions exist in the antifraud market, and conducted a comparative analysis of some of them. Then it was about systems aimed specifically at the task of detecting fraud in traffic. Today I want to share my little research on how client trackers themselves struggle with fraud. Consider the main ones: Appsflyer, Adjust, Kochava, TMC Attribution Analytics (ex. MAT), AppMetrica.
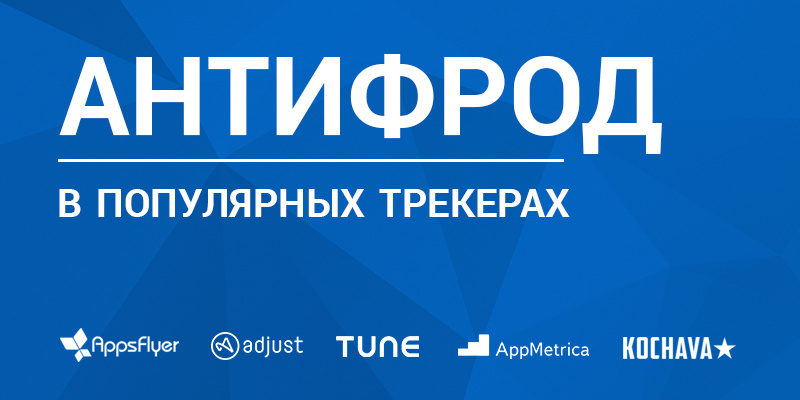
I will tell you what types of fraud the tracker determines (or does not determine), how the analysis takes place, how effective it is, and I will also provide links for the trackers with a description of the anti-fraud system.
I’ll immediately make a reservation that in this article I do not compare the convenience of the trackers themselves and their quality - we will focus exclusively on the antifraud solutions built into them.
We often work with Appsflyer, their new products do not pass us by. Not so long ago, colleagues released their own system of protection against fraud , with which you can detect low-quality traffic and examine each of the sources.
The main thesis of Appsflyer is that they have a huge base of IP and devices, each of which has its own quality label. That is, if a previously defined device was noticed in suspicious activity, then the installation on it will be marked as fraud. Also, the presence of the base itself allows you to find out the number of new devices for each of the sources. If there are too many of them, then Appsflyer considers this an occasion to suspect the source of "creating" new devices - in fact, the use of emulators.
Each device is assigned its own rating: fraudulent devices are rated “C”, suspicious “B”, new devices “N”, etc., depending on the uniqueness of the interaction of a real living user with a device that receives a rating of “A”, “ AA "or" AAA ". Devices with a "C" rating are automatically excluded from the lists of attributable installations and Analytics AppsFlyer, that is, there are no postbacks per se.

In the screenshot you can see a breakdown by traffic sources and antifraud metrics — the percentage of new devices, loyal users, installations from devices of type B and net conversions .
In fact, everything is not so simple. The loud numbers in 98% of well-known Appsflyer devices, including the Russian market, may not be thorough. According to Gartner andIDC every quarter there are about 350 thousand new devices. I do not think that Appsflyer is able to keep track of each of them. Therefore, relying entirely on their database is not too reasonable.
However, they themselves say that the fraud mark is just a reason for additional examination. Also, it is recommended to pay attention to the metric "Loyal Users". If it is much lower in other sources, combined with a large percentage of new devices, then you should study the source of traffic.
I'll prove it in practice. I came across a case when a client using Appsflyer started to worry about the number of new devices in one of the partners. However, when questioning the partner, thoroughly studying the source of his traffic and analyzing with the help of an additional antifraud system, it turned out that the partner distributes offer-ox on new Chinese devices, having concluded an agreement with a store selling these devices. That is, the traffic was absolutely normal and clean, but Appsflyer found it to be suspicious. On the other hand, this traffic could be just fraudulent, therefore the AppsFlyer suspicious label helps to study complex cases and identify partners with unusual traffic. Or discover the real fraud. Therefore, using this system is useful, but you should be extremely careful.
Conclusion:4 out of 5 . You can focus on their metrics with your own thorough analysis, in addition, the advanced antifraud is already included in the advanced AF account and does not require payment.
Adjust provides two fraud control tools in its product. The first, fairly standard, checks for the presence of IP in the VPN database and marks conversions with a hidden IP. It’s quite suitable as a proxy protection, it does its job. But if the proxy did not get into the database initially, or the fraudster uses new servers, there is a risk of missing a fraudulent conversion. Unfortunately, such cases are far from uncommon.
The second tool is a click spam analyzer. Adjust are the only ones who are engaged in such an analysis, so there is nothing special to compare. The method of verification is to study the number of identical clicks from one source and compare the difference between the time of a click and conversion with a conditional average. Let's just say that the idea of analyzing traffic in this way is very interesting, but choosing the same "rate" in time is not so simple. There is a likelihood of a false positive if some deviation occurs, for example, a person simply forgot to launch the downloaded application or deliberately delayed its launch until the moment he needs it.
In general, again, it is required to study each case of such a response in order to achieve maximum accuracy, otherwise you risk losing an adequate source that is falsely accused of fraud.
There is another third tool, the purchase validator, which checks the validity of a purchase by comparing the data with information from the store, but we, as an agency, cannot evaluate it.

In the antifraud statistics, you can immediately see exactly what the conversion is suspected of: AIP indicates a suspicious or hidden IP, TME - about too many identical clicks, and DO - about a strange time between the click and the installation. In the screenshot - one of the "suspects"
Rating: 4.5 out of 5 . While no one else is engaged in such an analysis, therefore, you can use the Adjust tool as recommendations for studying traffic anomalies. However, you need to understand that the built-in tool will not do all the work for you, so you should not rely on it entirely.
Also, the advantages include the use of antifraud to retargeting campaigns. From our experience, Adjust copes very well, so for such purposes it can be definitely positively noted among the rest.
There are no built-in antifraud tools in AppMetrica, it's that simple. But it is worth mentioning that colleagues decided not to reinvent their own bike, but integrated with the FraudScore product, a third-party traffic inspector, which I described in detail in a previous article about mobile fraud .
The solution is worthy of respect: why try to do something that others do much better, it’s wiser to delegate it to well-deserved leaders.
Rating for Appmetrica: no rating .
Score for FraudScore : 5 out of 5 - I already wrote about this system, but you can safely connect it without using AppMetrica.
There are various anti-fraud analytics tools in the TMC system .
The first automatically blocks all conversions for which there are anomalies (settings from one Device ID are duplicated, in-app purchases are emulated and do not match the store data). There are also 2 criteria that can be arbitrarily disabled - Jailbroken devices and maximum IP for one fingerprint. In fact, the system is fair - most of the criteria are unambiguous for the fraud and it makes no sense to disable them, and what can depend on the wishes of the customers can be turned off.
In addition, there is a time report between the click and the installation. It does not block sending postbacks, but it is convenient to search for anomalies on its own. Purchases in the application can also be checked for fraud, but we can’t test this functionality on the part of the agency, so I won’t include it in the evaluation.
Finally, the system provides the opportunity to create your own “black lists” - set a suitable attribution window (how many days can elapse from the time of a click), block sub-partners, device models, countries.
This does not draw on a full-fledged antifraud, however, to be able to customize tracking at this level is definitely nice.
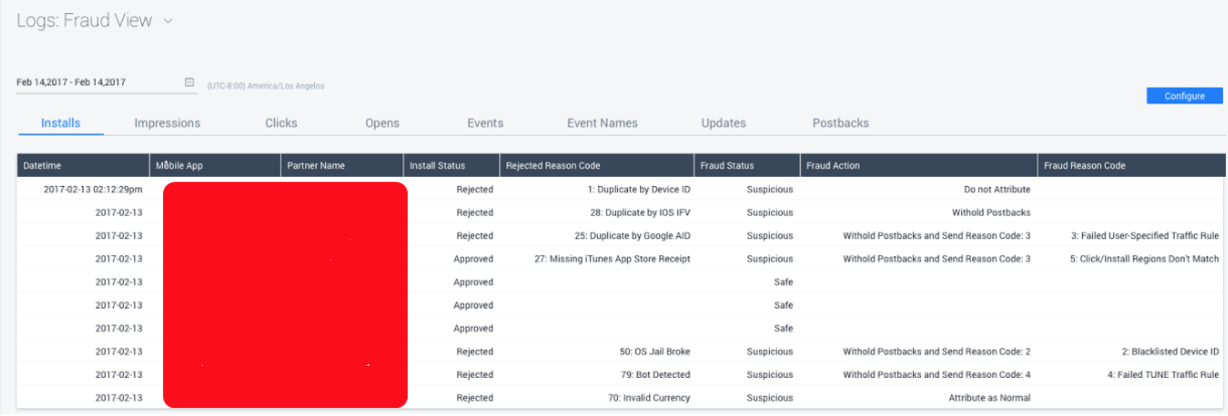
Breakdown of conversions that did not pass the quality assessment - indicated partner, time and reason for suspicion
Conclusion: 3.5 out of 5 . There are no too strict criteria; there are customization and analysis tools. You can recommend it as an adequate solution if you have your own analysts on your side.
In practice, I have never been able to deal with the Kochava antifraud , however, their article pretty well describes how to independently find the fraud and what criteria to look at. The tracker practically does not take this work upon itself, however, it tells the client in detail how suspicious traffic can be calculated.
There is, however, Trafic Verifier - a small utility that essentially performs the task of a tracking system - restricts GEO, OS, and device types. An additional function of Frequency Capping acts as a tool that limits the number of clicks from a specific IP and other things for a selected period of time. You can also analyze What-If traffic, which is already in the statistics.
Из других решений — Kochava Blacklist — по сути, глобальный фильтр трафика, помечающий неподходящий по критериям: количества кликов с одного устройства и IP и разницы между кликом и установкой.
Как и было сказано выше — у меня не было возможности проверить его в деле, однако выставлять общие фильтры на все, не учитывая специфики приложения и трафика — идея далеко не рациональная.
Вывод: 2,5 из 5. Инструкция и инструмент хороший, но по факту трекер почти не выполняет функцию антифрода, лишь дает возможность управлять трафиком и пищу для размышлений о его качестве.
From all the above, we can conclude: at the moment, none of the trackers will fully perform the task of determining fraud.
Trackers that implement anti-fraud solutions are definitely well done, since nothing like this had existed before. Now, the tracker can significantly facilitate the task of detecting fraud. However, to make a decision about traffic redirecting based solely on the recommendations of the tracker, without communicating with a partner and without studying all kinds of metrics on their own is a losing strategy in the long run.
We hope that in the future, colleagues from tracking systems will not slow down the pace of development of their own antifraud solutions, making them thoroughly accurate.
In the meantime, choose the tracker that suits you best, train your own analysts to find anomalies, and choose partners who are ready for dialogue and internal analysis - as, for example, we do this in Mobio .
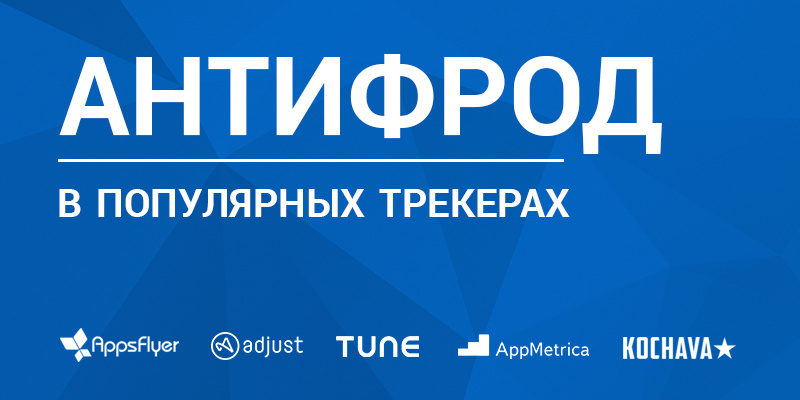
I will tell you what types of fraud the tracker determines (or does not determine), how the analysis takes place, how effective it is, and I will also provide links for the trackers with a description of the anti-fraud system.
I’ll immediately make a reservation that in this article I do not compare the convenience of the trackers themselves and their quality - we will focus exclusively on the antifraud solutions built into them.
Appsflyer
We often work with Appsflyer, their new products do not pass us by. Not so long ago, colleagues released their own system of protection against fraud , with which you can detect low-quality traffic and examine each of the sources.
The main thesis of Appsflyer is that they have a huge base of IP and devices, each of which has its own quality label. That is, if a previously defined device was noticed in suspicious activity, then the installation on it will be marked as fraud. Also, the presence of the base itself allows you to find out the number of new devices for each of the sources. If there are too many of them, then Appsflyer considers this an occasion to suspect the source of "creating" new devices - in fact, the use of emulators.
Each device is assigned its own rating: fraudulent devices are rated “C”, suspicious “B”, new devices “N”, etc., depending on the uniqueness of the interaction of a real living user with a device that receives a rating of “A”, “ AA "or" AAA ". Devices with a "C" rating are automatically excluded from the lists of attributable installations and Analytics AppsFlyer, that is, there are no postbacks per se.

In the screenshot you can see a breakdown by traffic sources and antifraud metrics — the percentage of new devices, loyal users, installations from devices of type B and net conversions .
In fact, everything is not so simple. The loud numbers in 98% of well-known Appsflyer devices, including the Russian market, may not be thorough. According to Gartner andIDC every quarter there are about 350 thousand new devices. I do not think that Appsflyer is able to keep track of each of them. Therefore, relying entirely on their database is not too reasonable.
However, they themselves say that the fraud mark is just a reason for additional examination. Also, it is recommended to pay attention to the metric "Loyal Users". If it is much lower in other sources, combined with a large percentage of new devices, then you should study the source of traffic.
I'll prove it in practice. I came across a case when a client using Appsflyer started to worry about the number of new devices in one of the partners. However, when questioning the partner, thoroughly studying the source of his traffic and analyzing with the help of an additional antifraud system, it turned out that the partner distributes offer-ox on new Chinese devices, having concluded an agreement with a store selling these devices. That is, the traffic was absolutely normal and clean, but Appsflyer found it to be suspicious. On the other hand, this traffic could be just fraudulent, therefore the AppsFlyer suspicious label helps to study complex cases and identify partners with unusual traffic. Or discover the real fraud. Therefore, using this system is useful, but you should be extremely careful.
Conclusion:4 out of 5 . You can focus on their metrics with your own thorough analysis, in addition, the advanced antifraud is already included in the advanced AF account and does not require payment.
Adjust
Adjust provides two fraud control tools in its product. The first, fairly standard, checks for the presence of IP in the VPN database and marks conversions with a hidden IP. It’s quite suitable as a proxy protection, it does its job. But if the proxy did not get into the database initially, or the fraudster uses new servers, there is a risk of missing a fraudulent conversion. Unfortunately, such cases are far from uncommon.
The second tool is a click spam analyzer. Adjust are the only ones who are engaged in such an analysis, so there is nothing special to compare. The method of verification is to study the number of identical clicks from one source and compare the difference between the time of a click and conversion with a conditional average. Let's just say that the idea of analyzing traffic in this way is very interesting, but choosing the same "rate" in time is not so simple. There is a likelihood of a false positive if some deviation occurs, for example, a person simply forgot to launch the downloaded application or deliberately delayed its launch until the moment he needs it.
In general, again, it is required to study each case of such a response in order to achieve maximum accuracy, otherwise you risk losing an adequate source that is falsely accused of fraud.
There is another third tool, the purchase validator, which checks the validity of a purchase by comparing the data with information from the store, but we, as an agency, cannot evaluate it.

In the antifraud statistics, you can immediately see exactly what the conversion is suspected of: AIP indicates a suspicious or hidden IP, TME - about too many identical clicks, and DO - about a strange time between the click and the installation. In the screenshot - one of the "suspects"
Rating: 4.5 out of 5 . While no one else is engaged in such an analysis, therefore, you can use the Adjust tool as recommendations for studying traffic anomalies. However, you need to understand that the built-in tool will not do all the work for you, so you should not rely on it entirely.
Also, the advantages include the use of antifraud to retargeting campaigns. From our experience, Adjust copes very well, so for such purposes it can be definitely positively noted among the rest.
Appmetrica
There are no built-in antifraud tools in AppMetrica, it's that simple. But it is worth mentioning that colleagues decided not to reinvent their own bike, but integrated with the FraudScore product, a third-party traffic inspector, which I described in detail in a previous article about mobile fraud .
The solution is worthy of respect: why try to do something that others do much better, it’s wiser to delegate it to well-deserved leaders.
Rating for Appmetrica: no rating .
Score for FraudScore : 5 out of 5 - I already wrote about this system, but you can safely connect it without using AppMetrica.
TMC Attribution Analytics (ex. MAT)
There are various anti-fraud analytics tools in the TMC system .
The first automatically blocks all conversions for which there are anomalies (settings from one Device ID are duplicated, in-app purchases are emulated and do not match the store data). There are also 2 criteria that can be arbitrarily disabled - Jailbroken devices and maximum IP for one fingerprint. In fact, the system is fair - most of the criteria are unambiguous for the fraud and it makes no sense to disable them, and what can depend on the wishes of the customers can be turned off.
In addition, there is a time report between the click and the installation. It does not block sending postbacks, but it is convenient to search for anomalies on its own. Purchases in the application can also be checked for fraud, but we can’t test this functionality on the part of the agency, so I won’t include it in the evaluation.
Finally, the system provides the opportunity to create your own “black lists” - set a suitable attribution window (how many days can elapse from the time of a click), block sub-partners, device models, countries.
This does not draw on a full-fledged antifraud, however, to be able to customize tracking at this level is definitely nice.
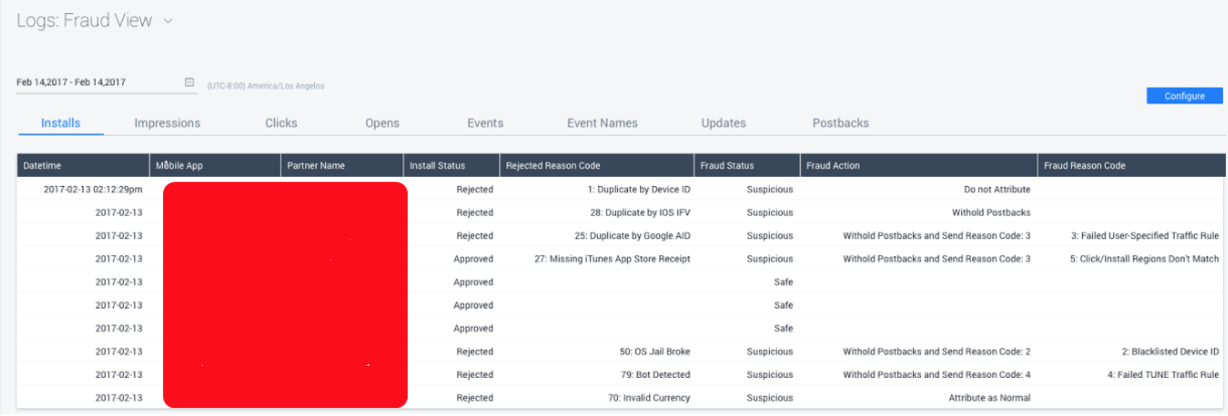
Breakdown of conversions that did not pass the quality assessment - indicated partner, time and reason for suspicion
Conclusion: 3.5 out of 5 . There are no too strict criteria; there are customization and analysis tools. You can recommend it as an adequate solution if you have your own analysts on your side.
Kochava
In practice, I have never been able to deal with the Kochava antifraud , however, their article pretty well describes how to independently find the fraud and what criteria to look at. The tracker practically does not take this work upon itself, however, it tells the client in detail how suspicious traffic can be calculated.
There is, however, Trafic Verifier - a small utility that essentially performs the task of a tracking system - restricts GEO, OS, and device types. An additional function of Frequency Capping acts as a tool that limits the number of clicks from a specific IP and other things for a selected period of time. You can also analyze What-If traffic, which is already in the statistics.
Из других решений — Kochava Blacklist — по сути, глобальный фильтр трафика, помечающий неподходящий по критериям: количества кликов с одного устройства и IP и разницы между кликом и установкой.
Как и было сказано выше — у меня не было возможности проверить его в деле, однако выставлять общие фильтры на все, не учитывая специфики приложения и трафика — идея далеко не рациональная.
Вывод: 2,5 из 5. Инструкция и инструмент хороший, но по факту трекер почти не выполняет функцию антифрода, лишь дает возможность управлять трафиком и пищу для размышлений о его качестве.
Результаты
From all the above, we can conclude: at the moment, none of the trackers will fully perform the task of determining fraud.
Trackers that implement anti-fraud solutions are definitely well done, since nothing like this had existed before. Now, the tracker can significantly facilitate the task of detecting fraud. However, to make a decision about traffic redirecting based solely on the recommendations of the tracker, without communicating with a partner and without studying all kinds of metrics on their own is a losing strategy in the long run.
We hope that in the future, colleagues from tracking systems will not slow down the pace of development of their own antifraud solutions, making them thoroughly accurate.
In the meantime, choose the tracker that suits you best, train your own analysts to find anomalies, and choose partners who are ready for dialogue and internal analysis - as, for example, we do this in Mobio .
Only registered users can participate in the survey. Please come in.
Are you comfortable with the built-in antifraud tool in your tracker?
- 30% Yes 3
- 20% No 2
- 10% I do not use the built-in tool 1
- 40% My tracker does not have a similar tool 4