Neural networks do not understand what optical illusions are
- Transfer
Machine vision systems can recognize faces on a par with people and even create realistic artificial faces. But researchers have found that these systems cannot recognize optical illusions, and therefore create new ones.

Human vision is an amazing device. Although it has evolved in a specific environment for millions of years, it is capable of tasks that never came across in early visual systems. A good example would be reading, or defining artificial objects - cars, airplanes, road signs, etc.
But the visual system has a well-known set of flaws that we perceive as optical illusions. Researchers have already identified many options in which these illusions make people misjudge the color, size, relative position and movement.
By themselves, illusions are interesting in that they give an idea of the nature of the visual system and perception. Therefore, it will be very useful to come up with a way to find new illusions that will help to learn the limitations of this system.
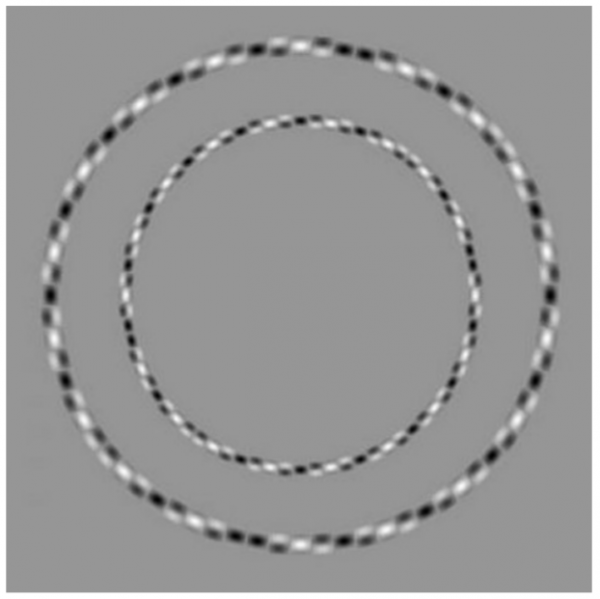
Concentric circles?
Here we should benefit from deep learning. In recent years, machines have learned to recognize objects and faces in images, and then create similar images. It is easy to imagine that a computer vision system must be able to recognize illusions and create its own.
This is where Robert Williams and Roman Yampolsky from the University of Louisville in Kentucky come on the scene. These guys tried to crank up such a thing , but found that everything is not so simple. Existing machine learning systems are not capable of producing their own optical illusions - at least for now. Why so?
First general information. Recent advances in depth learning are based on two breakthroughs. The first is the availability of powerful neural networks and a couple of software tricks that allow them to study well.
The second is the creation of a huge volume of labeled databases on the basis of which machines are capable of learning. For example, to teach a machine to recognize faces, tens of thousands of images containing clearly marked faces are required. With such information, a neural network can learn to recognize the characteristic patterns of faces — two eyes, a nose, a mouth. What is even more impressive, a couple of networks - the so-called. Generative-adversary network (GSS) - able to teach each other to create realistic and completely artificial face images.
Williams and Yampolsky conceived to teach the neural network to define optical illusions. There is enough computing power, and there are not enough suitable databases. Therefore, their first task was to create a database of optical illusions for training.
It turned out to be difficult to do. “There are only a few thousand static optical illusions, and the number of unique types of illusions is very small — perhaps a couple of dozen,” they say.
And this is a serious obstacle for modern machine learning systems. “Creating a model that can learn from such a small and limited set of data will be a huge leap forward for generative models and an understanding of human vision,” they say.
Therefore, Williams and Yampolsky collected a database of more than 6000 images of optical illusions, and trained the neural network to recognize them. Then they created the GSS, which should independently create optical illusions.
The results disappointed them. "After seven hours of training on the Nvidia Tesla K80, nothing of value was created," say the researchers who opened the database for use by everyone.
The result, however, is interesting. “The only optical illusions known to us were created by evolution (for example, eye drawings on butterfly wings) or human artists,” they point out. And in both cases, people played a crucial role in providing feedback - people can see the illusion.
And computer vision systems cannot. “It is unlikely that the GSS will be able to learn to deceive the sight without understanding the principles underlying illusions,” say Williams and Yampolsky.
This can be challenging because there are critical differences between the human and machine visual systems. Many researchers create neural networks that even more closely resemble the human visual system. Perhaps one of the interesting checks for these systems will be whether they can see the illusion.
In the meantime, Williams and Yampolsky are not optimistic: "Apparently, the data set with illusions may not be enough to create new illusions," they say. So, while optical illusions remain bastion of human perception, not subject to machines.