
AI, Big Data and Technology Disinformation
- Transfer
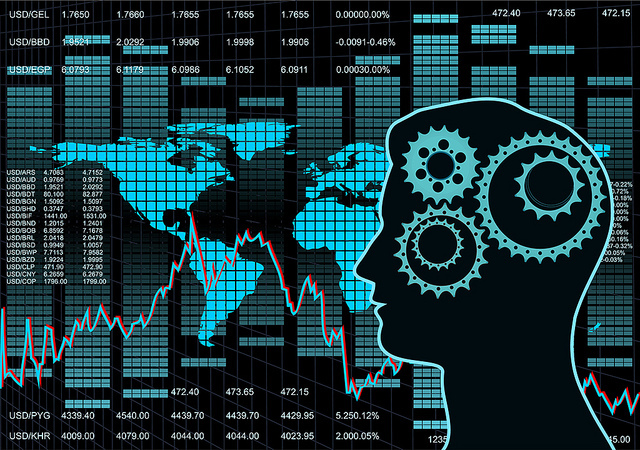
/ photo KamiPhuc CC
Usually in our blog we talk about cloud services , hosting and related technologies. Today we’ll talk about the challenges of technology development in general, artificial intelligence, big data, and Michael Jordan (not a basketball player).
Michael Jordan , Professor Emeritus at the University of California, Berkeley, and IEEE Member. Jordan is one of the most respected and respected people, a world expert on the topic of machine learning. He is confident that the overuse of big data will not produce the expected effect and lead to disasters such as massive collapse of bridges.
Let's try to understand this topic better. Let's look at the definition of the term “artificial intelligence” by the creator of the Lisp language, John McCarthy. In an article of the same name (“What is Artificial Intelligence?”), He emphasized that AI is related to the task of using computers to understand the work of human intelligence, but is not limited to using methods observed in biology.
Of course, such an interpretation is clearly far from our ideas about the futuristic image of AI. Journalist Gomez and Jordan in their conversation confirm this idea and emphasize the existence of a kind of misinformation that is beneficial for various media working on the wave of the growing popularity of this topic.
Michael appeals to the experience of researching the neural networks that have been talked about at every corner since the 1980s, while repeating what was known back in the 1960s. Today, the main idea is a convolutional neural network, but this is not about neurology at all. People are convinced of the need to understand how the human brain processes information, learns and makes decisions, but in reality science is developing in a slightly different direction.
Jordan says that it will take tens or even hundreds of years for neuroscience to understand the underlying principles of brain function. Today we are only approaching the beginning of the study of the principles of presentation, storage and processing of information by neurons. We have virtually no understanding of how learning actually takes place in our brains. Although for similar analogies its place. So, people began to search for metaphors related to the parallel work of the brain, which turned out to be useful for developing algorithms, but almost did not go beyond the level of searching for fresh solutions and ideas.
If we continue to consider the terms, we will see that the “neurons” involved in deep learning are a metaphor (or, in the language of Jordan, a “caricature” of the brain in general), which is used only for brevity and convenience. In reality, the work of the mechanisms of the same deep learning is much closer to the procedure of constructing a statistical model of logistic regression than to the work of real neurons.
John McCarthy, in turn, emphasized: the problem is not only in creating a system in the image and likeness of human intelligence, but in the fact that scientists themselves do not agree on what it (the intellect) is and for what specific processes are responsible. To say that we can “exactly recreate” this architecture and make it work is extremely unlikely in the near future.
Big data may be another media ploy that thousands of researchers around the world have pecked at. The modern obsession with big data can lead to the uncontrolled use of conclusions drawn from data with controversial statistical strength.
For any given database, you can find a combination of columns that is completely random, but will accurately answer any hypothesis that needs to be considered to solve a particular problem. Given the presence of millions of attributes for a particular object and an almost infinite number of combinations of these attributes, all this begins to resemble a joke about Shakespeare, a typewriter and a million monkeys.
Of course, there are many ideas for monitoring research, allowing you to find out with what frequency errors occurred in such hypotheses. But using mathematical and technical tools takes a long time, and we are still learning how to handle big data.
In science and new fields of knowledge, the boundaries and framework for research are one of the elements necessary for progress. This statement is supported by both the story with the first systems of technical vision (face recognition) and the example with speech technologies (recognition of individual words).
PS We are trying to share not only our own experience in working on the service for providing 1cloud virtual infrastructure , but also to talk about various studies and researchers who are involved in related fields of knowledge.
Do not forget to subscribe to our blog on Habré, friends!