
A few words about “linear” regression

Some formulas
In the simplest case, the linear model can be represented as follows:
y i = a 0 + a 1 x i + ε i
where a 0 is the mathematical expectation of the dependent variable y i when the variable x i is zero; a 1 - the expected change in the dependent variable y i when x i changes by one (this coefficient is selected so that ½Σ (y i - i i ) 2 is minimal - this is the so-called “residual function” ); ε i is a random error.
In this case, the coefficients a 1 and a 0 can be expressed in
â 1 = cor (y, x) σ y / σ x
â 0 = ȳ - â 1 x̄
Diagnostics and model errors
For the model to be correct, it is necessary to fulfill the Gauss-Markov conditions , i.e. errors should be homoskedastic with zero expectation. The residual plot e i = y i - ŷ i helps to determine how adequate the constructed model is (e i can be considered an estimate of ε i ).
Let us look at the graph of residuals in the case of a simple linear dependence y 1 ~ x (hereinafter all examples are given in the language R ):
Hidden text
set.seed(1)
n <- 100
x <- runif(n)
y1 <- x + rnorm(n, sd=.1)
fit1 <- lm(y1 ~ x)
par(mfrow=c(1, 2))
plot(x, y1, pch=21, col="black", bg="lightblue", cex=.9)
abline(fit1)
plot(x, resid(fit1), pch=21, col="black", bg="lightblue", cex=.9)
abline(h=0)
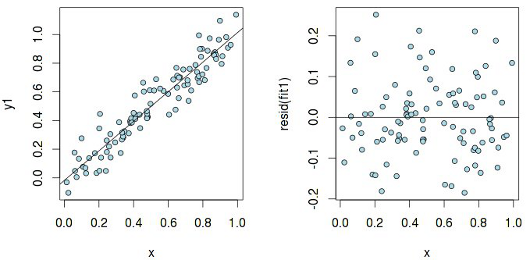
The residues are more or less evenly distributed relative to the horizontal axis, which indicates "the absence of a systematic relationship between the values of the random term in any two observations." Now, let's examine the same graph, but built for a linear model, which is actually not linear:
Hidden text
y2 <- log(x) + rnorm(n, sd=.1)
fit2 <- lm(y2 ~ x)
plot(x, y2, pch=21, col="black", bg="lightblue", cex=.9)
abline(fit2)
plot(x, resid(fit2), pch=21, col="black", bg="lightblue", cex=.9)
abline(h=0)
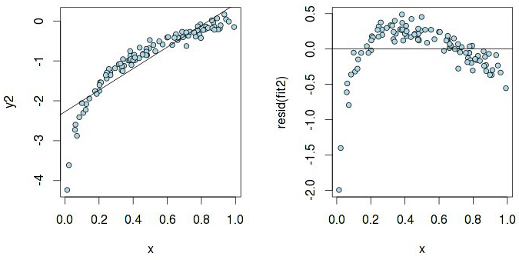
According to the graph y 2 ~ x, it seems that a linear dependence can be assumed, but the residuals have a pattern, which means that pure linear regression will not work here . And here is what heteroskedasticity really means :
Hidden text
y3 <- x + rnorm(n, sd=.001*x)
fit3 <- lm(y3 ~ x)
plot(x, y3, pch=21, col="black", bg="lightblue", cex=.9)
abline(fit3)
plot(x, resid(fit3), pch=21, col="black", bg="lightblue", cex=.9)
abline(h=0)

A linear model with such "swelling" residues is not correct. It is sometimes still useful to plot the quantiles of residues versus quantiles, which could be expected provided that the residues are normally distributed:
Hidden text
qqnorm(resid(fit1))
qqline(resid(fit1))
qqnorm(resid(fit2))
qqline(resid(fit2))

The second graph clearly shows that the assumption of the normality of the residues can be rejected (which again indicates the incorrectness of the model). And there are such situations:
Hidden text
x4 <- c(9, x)
y4 <- c(3, x + rnorm(n, sd=.1))
fit4 <- lm(y4 ~ x4)
par(mfrow=c(1, 1))
plot(x4, y4, pch=21, col="black", bg="lightblue", cex=.9)
abline(fit4)

This is the so-called "outlier" , which can greatly distort the results and lead to erroneous conclusions. R has a means to detect it - using the standardized measure dfbetas and hat values :
> round(dfbetas(fit4), 3)
(Intercept) x4
1 15.987 -26.342
2 -0.131 0.062
3 -0.049 0.017
4 0.083 0.000
5 0.023 0.037
6 -0.245 0.131
7 0.055 0.084
8 0.027 0.055
.....
> round(hatvalues(fit4), 3)
1 2 3 4 5 6 7 8 9 10...
0.810 0.012 0.011 0.010 0.013 0.014 0.013 0.014 0.010 0.010...
As can be seen, the first term of the vector x4 has a noticeably greater influence on the parameters of the regression model than the others, thus being an outlier.
Multiple Regression Model Selection
Naturally, with multiple regression the question arises: is it worth considering all the variables? On the one hand, it would seem that it costs, because any variable potentially carries useful information. In addition, increasing the number of variables, we increase R 2 (by the way, for this reason, this measure cannot be considered reliable in assessing the quality of the model). On the other hand, it’s worth remembering things like AIC and BIC , which introduce penalties for the complexity of the model. The absolute value of the information criterion in itself does not make sense, therefore, it is necessary to compare these values for several models: in our case, with a different number of variables. A model with a minimum value of the information criterion will be the best (although there is something to argue about).
Consider the UScrime dataset from the MASS library:
library(MASS)
data(UScrime)
stepAIC(lm(y~., data=UScrime))
The model with the lowest AIC value has the following parameters:
Call:
lm(formula = y ~ M + Ed + Po1 + M.F + U1 + U2 + Ineq + Prob,
data = UScrime)
Coefficients:
(Intercept) M Ed Po1 M.F U1 U2 Ineq Prob
-6426.101 9.332 18.012 10.265 2.234 -6.087 18.735 6.133 -3796.032
Thus, the optimal model taking into account AIC will be as follows:
fit_aic <- lm(y ~ M + Ed + Po1 + M.F + U1 + U2 + Ineq + Prob, data=UScrime)
summary(fit_aic)
...
Coefficients:
Estimate Std. Error t value Pr(>|t|)
(Intercept) -6426.101 1194.611 -5.379 4.04e-06 ***
M 9.332 3.350 2.786 0.00828 **
Ed 18.012 5.275 3.414 0.00153 **
Po1 10.265 1.552 6.613 8.26e-08 ***
M.F 2.234 1.360 1.642 0.10874
U1 -6.087 3.339 -1.823 0.07622 .
U2 18.735 7.248 2.585 0.01371 *
Ineq 6.133 1.396 4.394 8.63e-05 ***
Prob -3796.032 1490.646 -2.547 0.01505 *
Signif. codes: 0 ‘***’ 0.001 ‘**’ 0.01 ‘*’ 0.05 ‘.’ 0.1 ‘ ’ 1
If you look closely, it turns out that the variables MF and U1 have a rather high p-value, which seems to hint to us that these variables are not so important. But p-value is a rather ambiguous measure when assessing the importance of a variable for a statistical model. This fact is clearly demonstrated by an example:
data <- read.table("http://www4.stat.ncsu.edu/~stefanski/NSF_Supported/Hidden_Images/orly_owl_files/orly_owl_Lin_9p_5_flat.txt")
fit <- lm(V1~. -1, data=data)
summary(fit)$coef
Estimate Std. Error t value Pr(>|t|)
V2 1.1912939 0.1401286 8.501431 3.325404e-17
V3 0.9354776 0.1271192 7.359057 2.568432e-13
V4 0.9311644 0.1240912 7.503873 8.816818e-14
V5 1.1644978 0.1385375 8.405652 7.370156e-17
V6 1.0613459 0.1317248 8.057300 1.242584e-15
V7 1.0092041 0.1287784 7.836752 7.021785e-15
V8 0.9307010 0.1219609 7.631143 3.391212e-14
V9 0.8624487 0.1198499 7.196073 8.362082e-13
V10 0.9763194 0.0879140 11.105393 6.027585e-28
p-values for each variable are almost zero, and we can assume that all variables are important for this linear model. But actually, if you look closely at the remnants, it somehow goes like this:
Hidden text
plot(predict(fit), resid(fit), pch=".")

And yet, an alternative approach is based on analysis of variance , in which p-values play a key role. Compare the model without the MF variable with the model built taking into account only AIС:
fit_aic0 <- update(fit_aic, ~ . - M.F)
anova(fit_aic0, fit_aic)
Analysis of Variance Table
Model 1: y ~ M + Ed + Po1 + U1 + U2 + Ineq + Prob
Model 2: y ~ M + Ed + Po1 + M.F + U1 + U2 + Ineq + Prob
Res.Df RSS Df Sum of Sq F Pr(>F)
1 39 1556227
2 38 1453068 1 103159 2.6978 0.1087
Given a P-value of 0.1087, with a significance level of α = 0.05, we can conclude that there is no statistically significant evidence in favor of an alternative hypothesis, i.e. in favor of a model with an additional variable MF
References:
Only registered users can participate in the survey. Please come in.
Is it necessary to use MathJax on Habr?
- 87.2% Yes 192
- 12.7% No 28