
Machine translation for pros
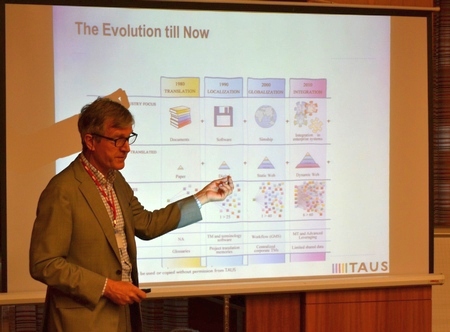
A little help about TAUS
TAUS is a reputable international organization that has been dealing with translation automation since 2004. Among its members are not only us, but also Google, eBay, Cisco, Intel, Adobe, Siemens and many other corporations. The founder of the organization is Jap van der Meer (pictured), an almost living industry legend. You can learn more about TAUS on our corporate blog or on the organization’s website .
The report that we will focus on was devoted to the topic of machine translation (MT). In general, many participants spoke about machine translation. For example, that its popularity is not declining, and many ordinary users and companies have begun to use it more actively in their work - only about 100 GB of information passes through Yandex.Translation daily.
Our innovation director Anton Voronov decided to talk about what is necessary for the productive professional use of machine translation.
We already wrotethat in the West they managed to evaluate the benefits of automation tools, and many organizations and linguistic service providers use different technologies in real work on orders: dictionaries, glossaries, translation memory databases, crowdsourcing and machine translation. It's simple: industry representatives understood - despite the fact that the volume of content around the world doubles almost annually, the pace of translation remains the same. Obviously, you need to increase productivity.
It has been proven in practice that machine translation should be used if at least two or three points of the following requirements are met:
- You have large projects with short deadlines.
- It is necessary to translate the so-called “less visible” content: technical documentation, user-generated content, knowledge bases. They are intended for a large number of users, but, as a rule, are very large in volume, and each section of such a text array (knowledge base, for example) carefully reads only a small number of users.
- As sources, you have texts with a clear structure and a high level of repetition;
- Your team has debugged combined production processes with flexible quality requirements - different projects may require a different level of quality: some texts must be translated as accurately as possible, for others it’s enough to catch the general meaning. And the team should be ready to adapt the translation process to such differences. It is assumed that in this case, experts are familiar with how post-editing, crowdsourcing and other operations that differ from the traditional TEP model work .

What does it look like in practice? Imagine that you need to translate many technical instructions to specific software. Firstly, it is worth stocking up Translation Memory databases, which were compiled during previous translations for this software or remained after similar projects - the more the better. Then it makes sense to decide on a suitable machine translation system - perhaps in some of the past projects some of them showed their best - and conjure with its configuration: feed existing databases and parallel texts. In the process of translation, be prepared to monitor the operation of the machine: so that you can quickly make adjustments if something goes wrong.
In our practice, the following production process scheme has proved its effectiveness:
- Start with the terminology - extract the terms from the source text and immediately translate them, this will make your life easier;
- Do not forget about linguistic resources - parallel texts, translation memory databases: they are important both for tuning the engine and for the translation itself;
- Choose the appropriate “engines” for each project - for this, of course, you need to constantly monitor the performance of all used MT systems;
- Train machine translation systems using results that meet the required level of quality;
- Speaking of quality, constantly monitor the quality of the translation: how much the results are in line with expectations;
- Fix what helps to make the translation of a particular segment better: metrics and verification of compliance with terminology will help;
- Measure all parameters to assess how much you need to finalize the text at the post-editing stage, and adjust the process accordingly;
- Get a platform that will automatically deal with all this.
As experience has shown, to maximize the automation of the translation process in any company, you need to take care of the online CAT tool. It needs to integrate the terminology management module and MT systems. It also makes sense to provide for a flexible production model (in case you have to change something on the go), real-time teamwork of artists, automatic recording of all post-editor actions (this will allow you to find bottlenecks) and built-in quality control.
In our case, this full automation cycle is based on SmartCAT , which we wrote about earlier and which we continue to actively develop.
We also touched on how to train the “translation engine” engines. In order to meet expectations from MT results, it is important to reuse linguistic resources when setting up the system. Retrieve the terminology, keep track of its uniformity and give the resulting glossaries to the “engines”. Take the segments that have already been translated and gone through the post-editing stage, and share them with your MT systems: the last options are important here, because they are more relevant.
Quality control throughout the whole process of working with machine translation will help to avoid unpleasant surprises. The history of the text changes, the time spent and the results of the automatic quality check will help you choose the segments that require close attention during the final quality assessment. In general, anything can happen, so be prepared for changes in the quality control process when translating MT.
We talked a bit about the plans. The fact is that we managed to dive so deeply into the process, since we ourselves have long and actively tested various automation systems and working methods in search of high performance and flexible quality level management. It became clear to us that to work more efficiently with MT, the integrated module is really not enough for extracting terminology, hints when searching in already loaded databases, data on the context of certain terms. And, of course, more quality checks and more metrics. We continue to integrate this into our products and our own processes.
Of course, linguistic technology continues to evolve. But the volume of content is growing even faster, and existing solutions still require the participation of professional translators in the process. In general, the near future of the industry lies in the joint work of people and machines.