Pizza ala-semi-supervised
In this article I would like to tell you about some of the techniques for working with data when teaching a model. In particular, how to stretch the segmentation of objects on the boxes, as well as how to train the model and get the markup of the dataset, having marked only a few samples.
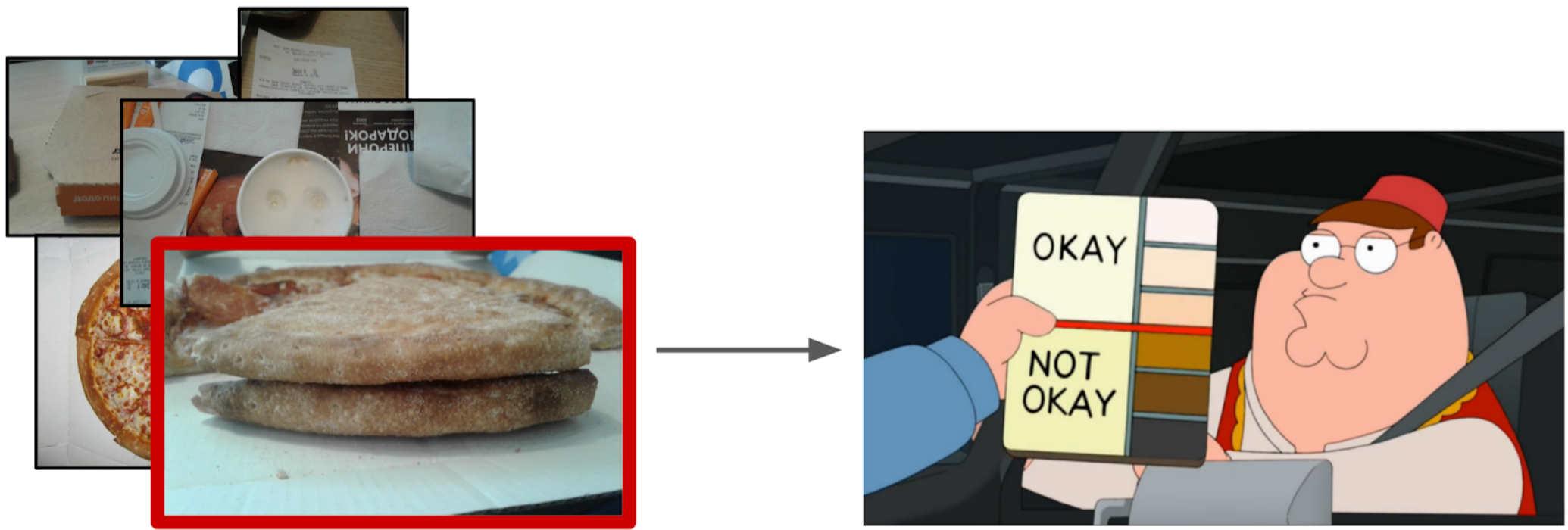
There is a certain process of cooking pizza and photos from its different stages (including not only pizza). It is known that if the dough recipe is spoiled, then there will be white bumps on the cake. There is also a binary markup of the quality of the dough of each pizza made by experts. It is necessary to develop an algorithm that will determine the quality of the test on the photo.
Dataset consists of photos taken from different phones, in different conditions, different angles. Copies of pizza - 17k. Total photos - 60k.
In my opinion, the task is quite typical and well suited to show different approaches to handling data. To solve it you need to:
1. Select photos where there is a pizza shortbread;
2. On the selected photos to highlight the cake;
3. Train the neural network in the selected areas.
At first glance, it seems that the easiest thing would be to give this task to the markers, and then to train on clean data. However, I decided that it would be easier for me to mark out a small part myself, than to explain with a marker, which angle was the right one. Moreover, I did not have a hard criterion for the correct angle.
Therefore, this is how I did:
1. Mark out 100 pictures of the edge;
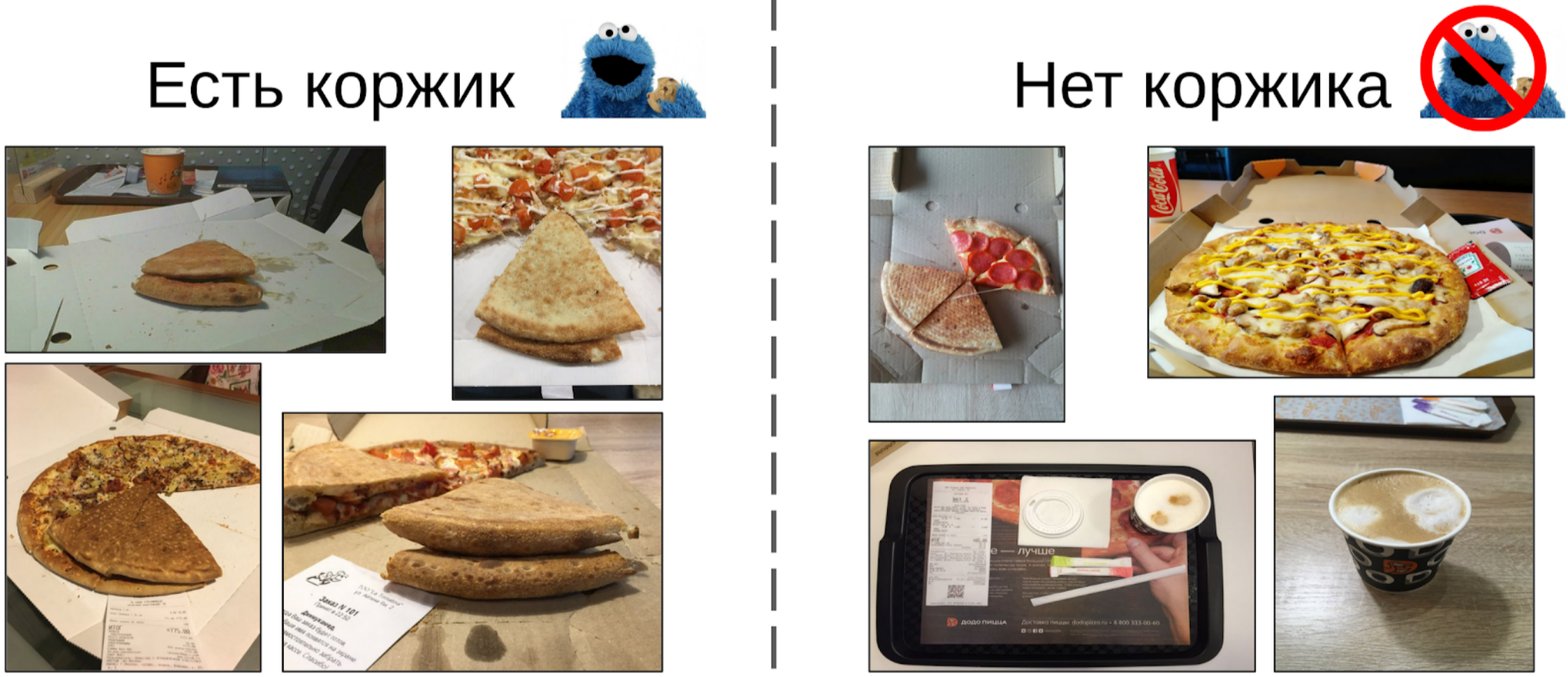
2. I counted features after a global pulling from the resnet-152 grid with weights from imagenet11k_places365;
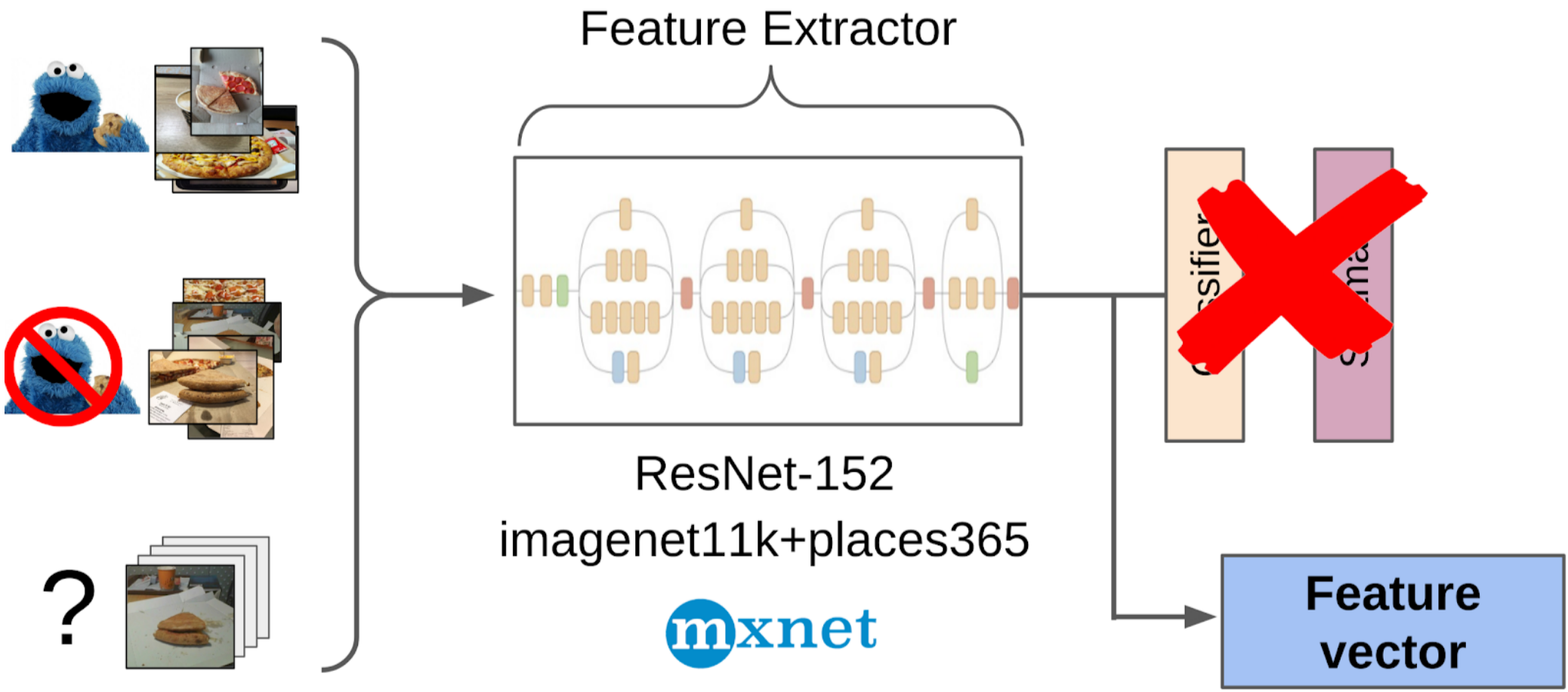
3. I took the average of the features of each class, getting two anchors;
4. I calculated the distance from each anchor to all features of the remaining 50k photos;
5. Top 300 in proximity to one anchor are relevant to the positive class, top 500 closest to the other anchor - negative class;
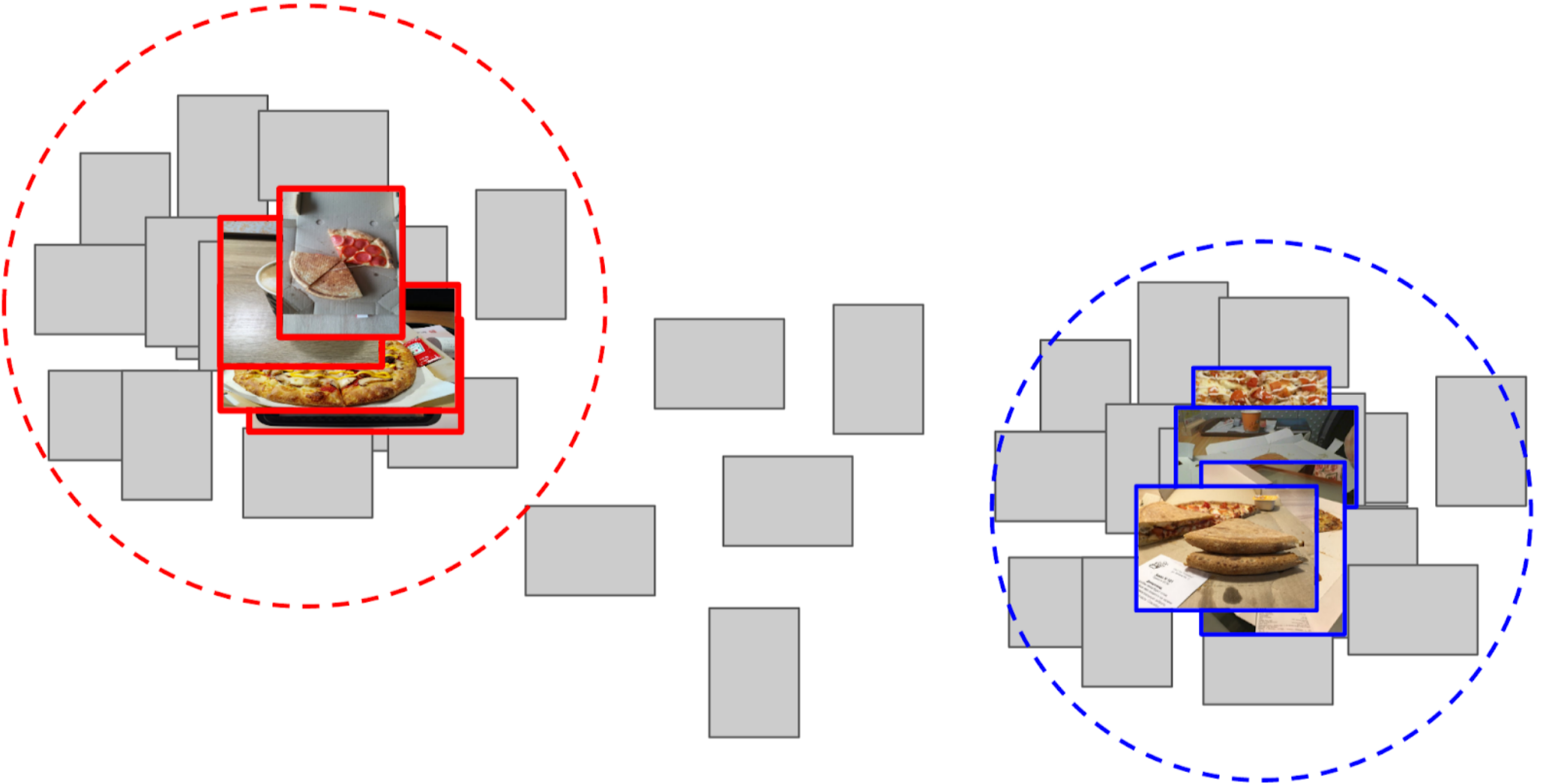
6. In these samples I taught LightGBM on the same features (XGboost is shown in the picture, because it has a logo and is more recognizable, but LightGBM does not have a logo);
7. With the help of this model I got the markup of the whole dataset.

Approximately the same approach I used in kaggle competitions as a baseline .
In ODS recently complained that no one writes about their Fails. Correcting the situation. About a year ago, I participated in the Kaggle Sea Lions competition with Yevgeny Nizhibitsky . The task was to calculate the fur seals in the pictures from the drone. The markup was given simply in the form of the coordinates of the carcasses, but at some point Vladimir Iglovikov marked them with boxes and generously shared this with the community. At that time, I considered myself the father of semantic segmentation (after Kaggle Dstl ) and decided that Unet would greatly facilitate the task of counting if I learned how to distinguish the seals coolly.
Accordingly, I began to teach segmentation, having as a target in the first stage only box seals. After the first stage of training, I predicted the train and looked at what the predictions look like. With the help of heuristics, it was possible to choose from an abstract confidence mask (confidence of predictions) and conditionally divide the predictions into two groups: where everything is good and where everything is bad.
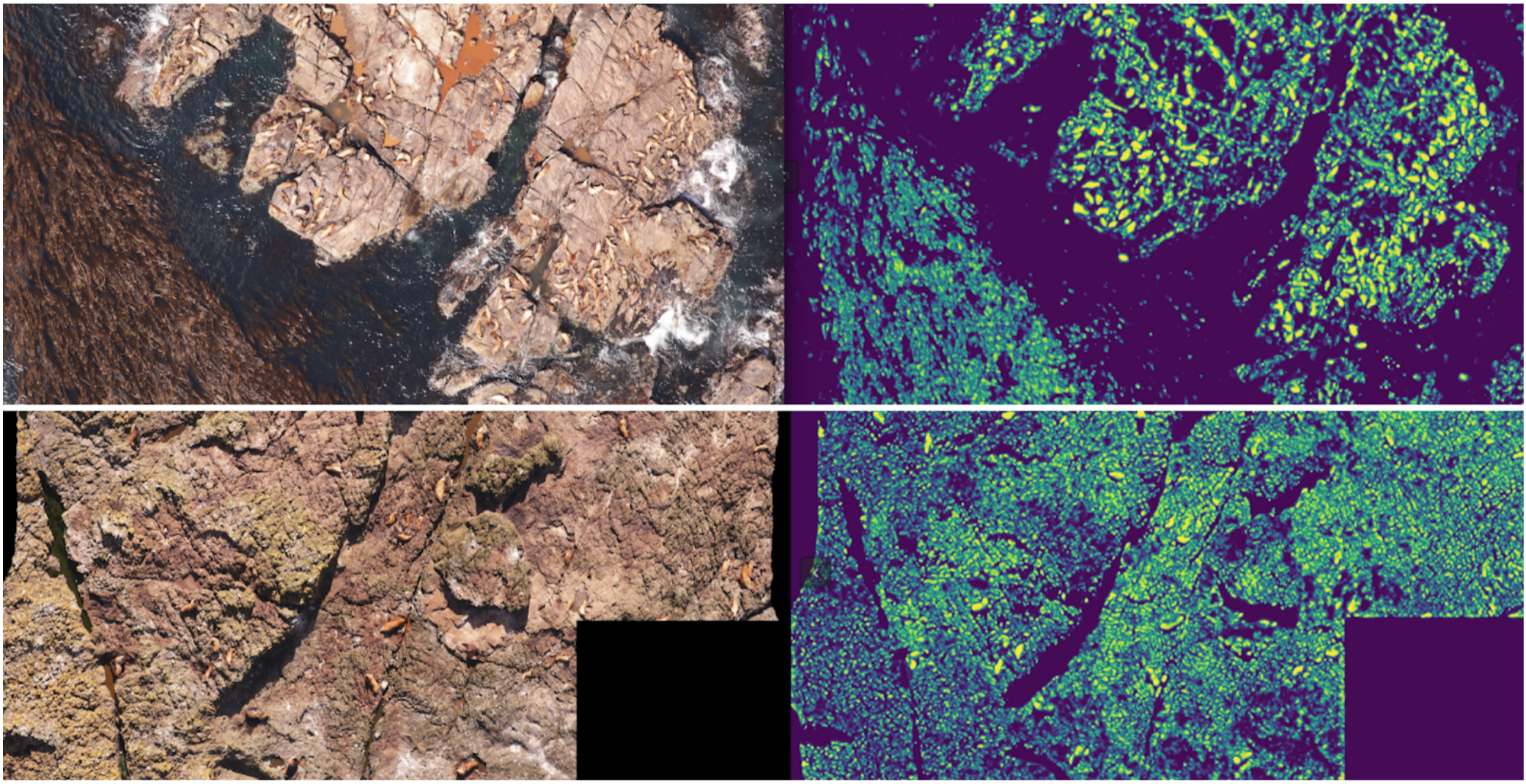
Predictions where everything is good could be used to train the next iteration of the model. Predicts, where everything is bad, it was possible to choose with large areas without seals, to manually mask the masks and also to sink into the train. And so iteratively, we with Eugene taught a model that has learned to even segment the seals of sea lions for large individuals.
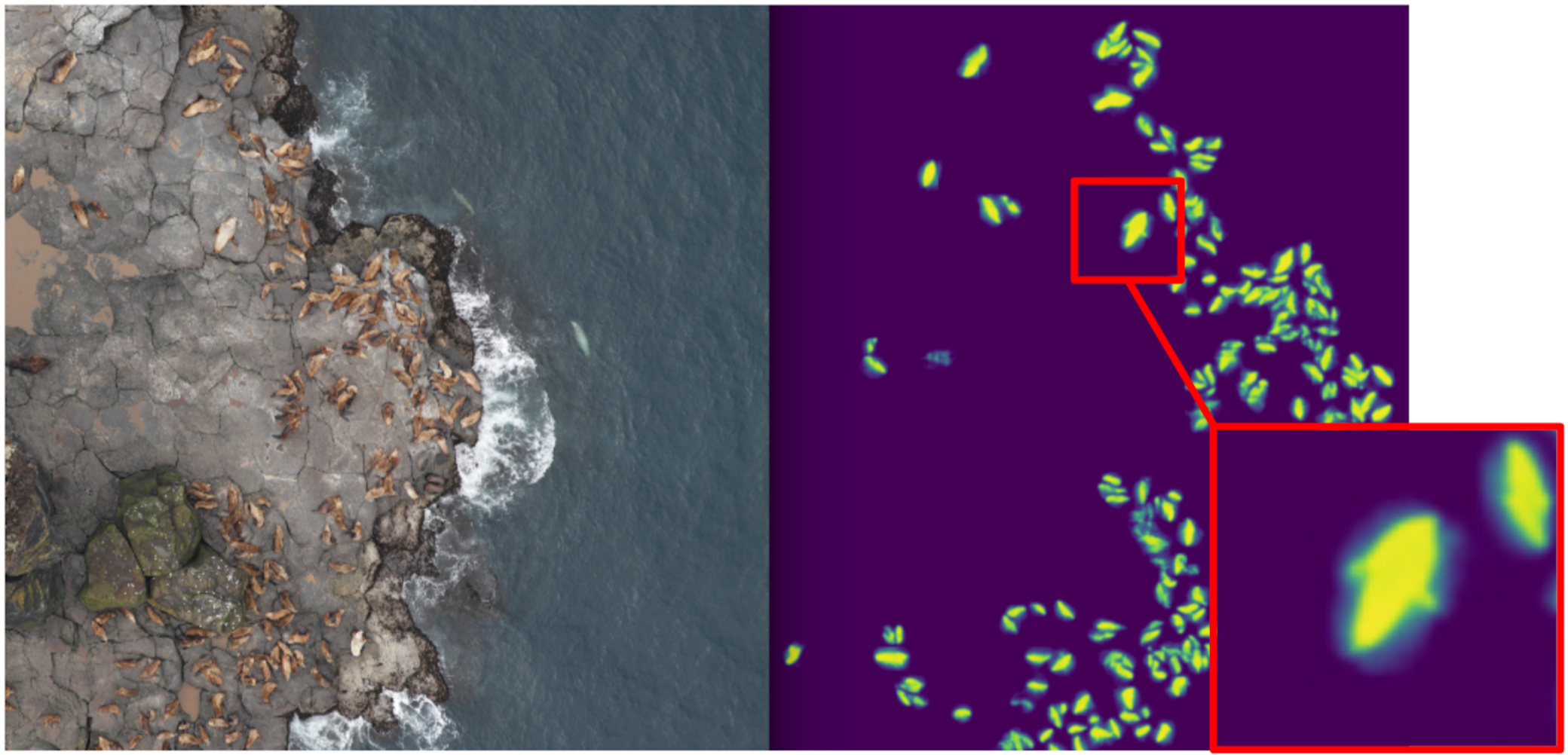
But it was a fierce fayl: we spent a lot of time trying to learn how to segmented the seals abruptly and ... It almost did not help in their calculation. The assumption that the density of seals (the number of individuals per unit area of the mask) is constant did not work, because the drone flew at different heights, and the pictures had a different scale. And at the same time, the segmentation still did not single out individual individuals, if they lay tight - which happened quite often. And before the innovative approach to the division of objects of the Tocoder team on the DSB2018, there was still a year. As a result, we stayed at the broken trough and finished in 40th place of 600 teams.
However, I made two conclusions: semantic segmentation is a convenient approach for visualizing and analyzing the operation of the algorithm, and you can weld masks out of the boxes with some effort.
But back to the pizza. In order to select a cake in the selected and filtered photos, the best option would be to give the task to the markers. At that time, we had already implemented the boxes and the consensus algorithm for them. So I just threw a couple of examples and put it on the markup. In the end, I got 500 samples with exactly selected area of the cake.
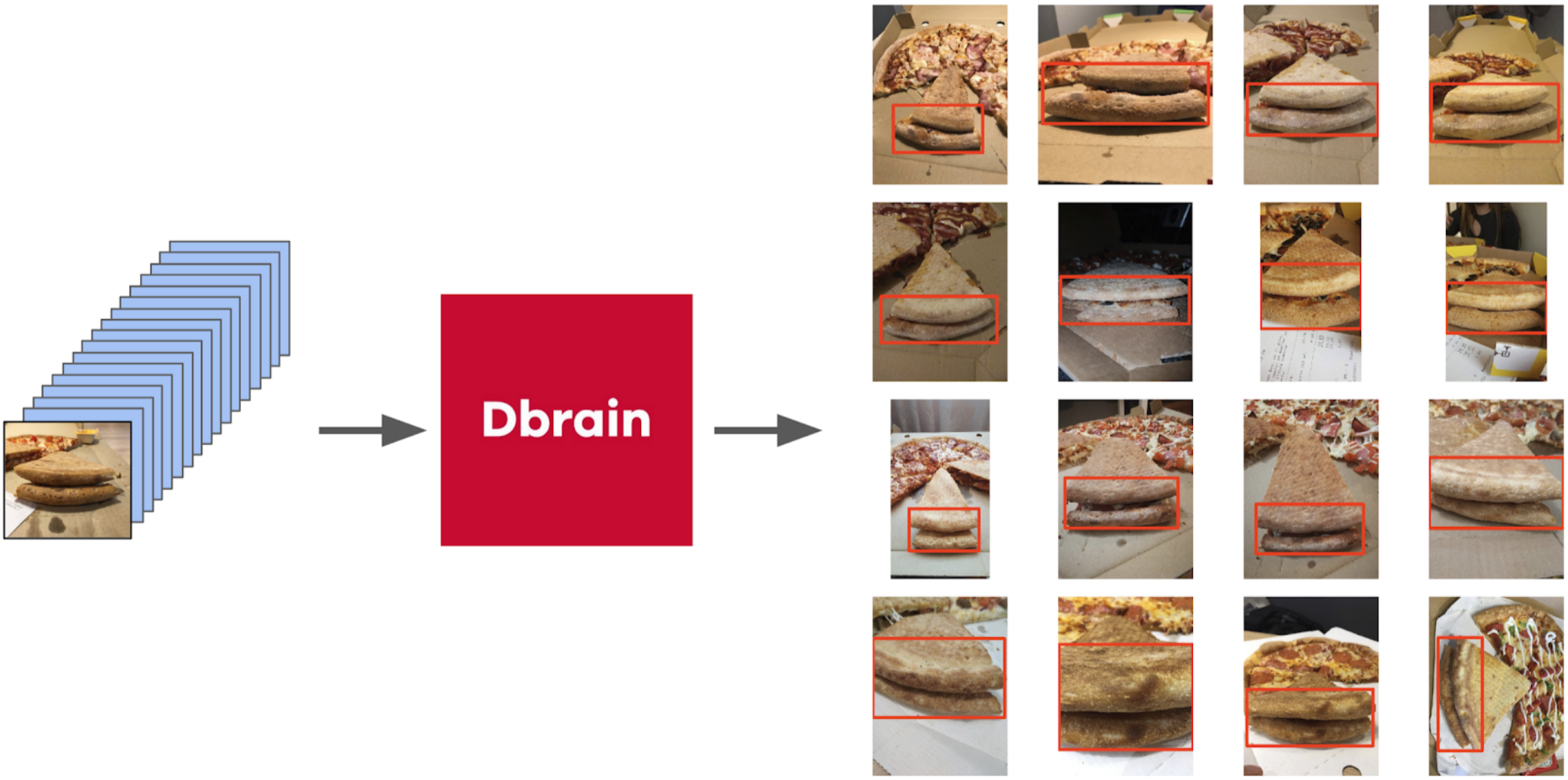
Then I dug out my code from the seals and more formally approached the current procedure. After the first iteration of the training, it was just as well seen where the model is mistaken. And the confidence of predictions can be defined as:
1 - (area of the gray area) / (area of the mask) # there will be a formula, I promise
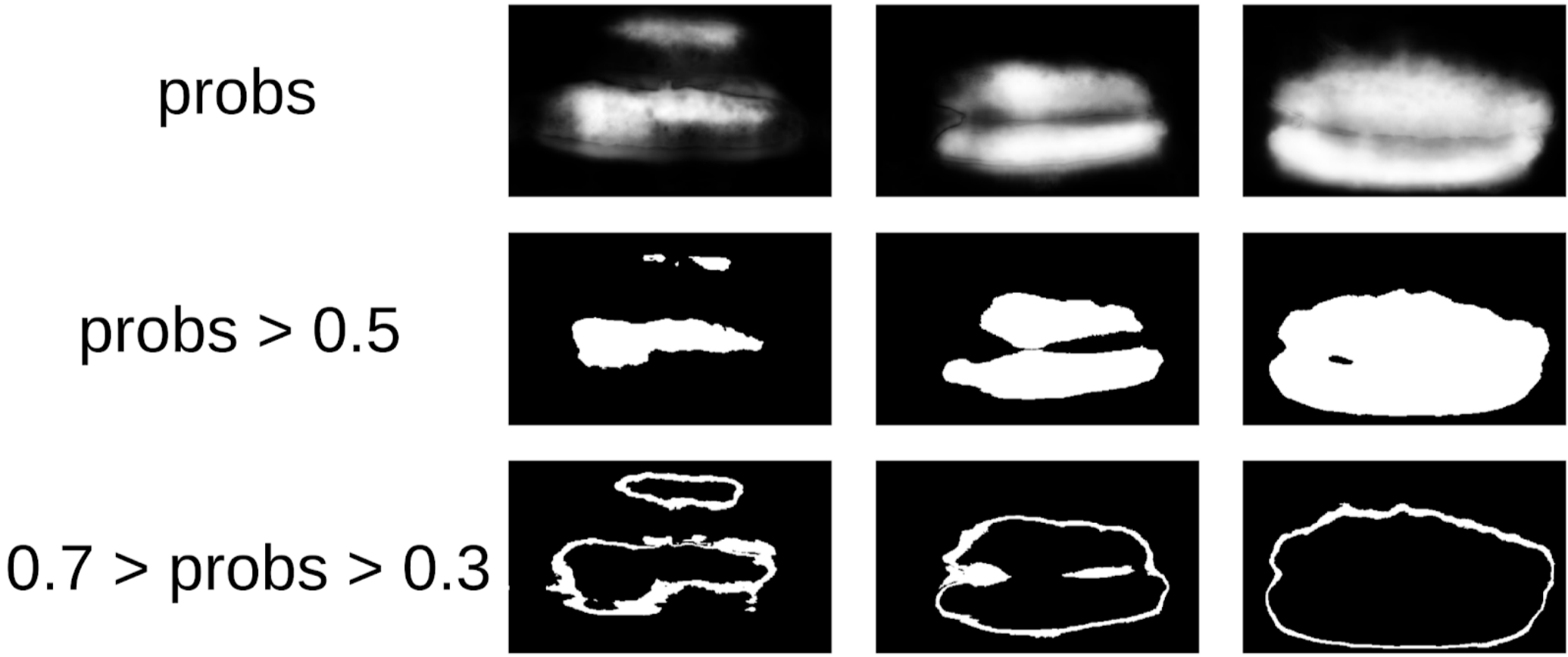
Now, in order to do the next iteration of tensioning the boxes on the masks, we will predict a small ensemble of a train with a TTA. This can be considered to some extent WAAAAGH knowledge distillation, but it is more correct to call it Pseudo Labeling.
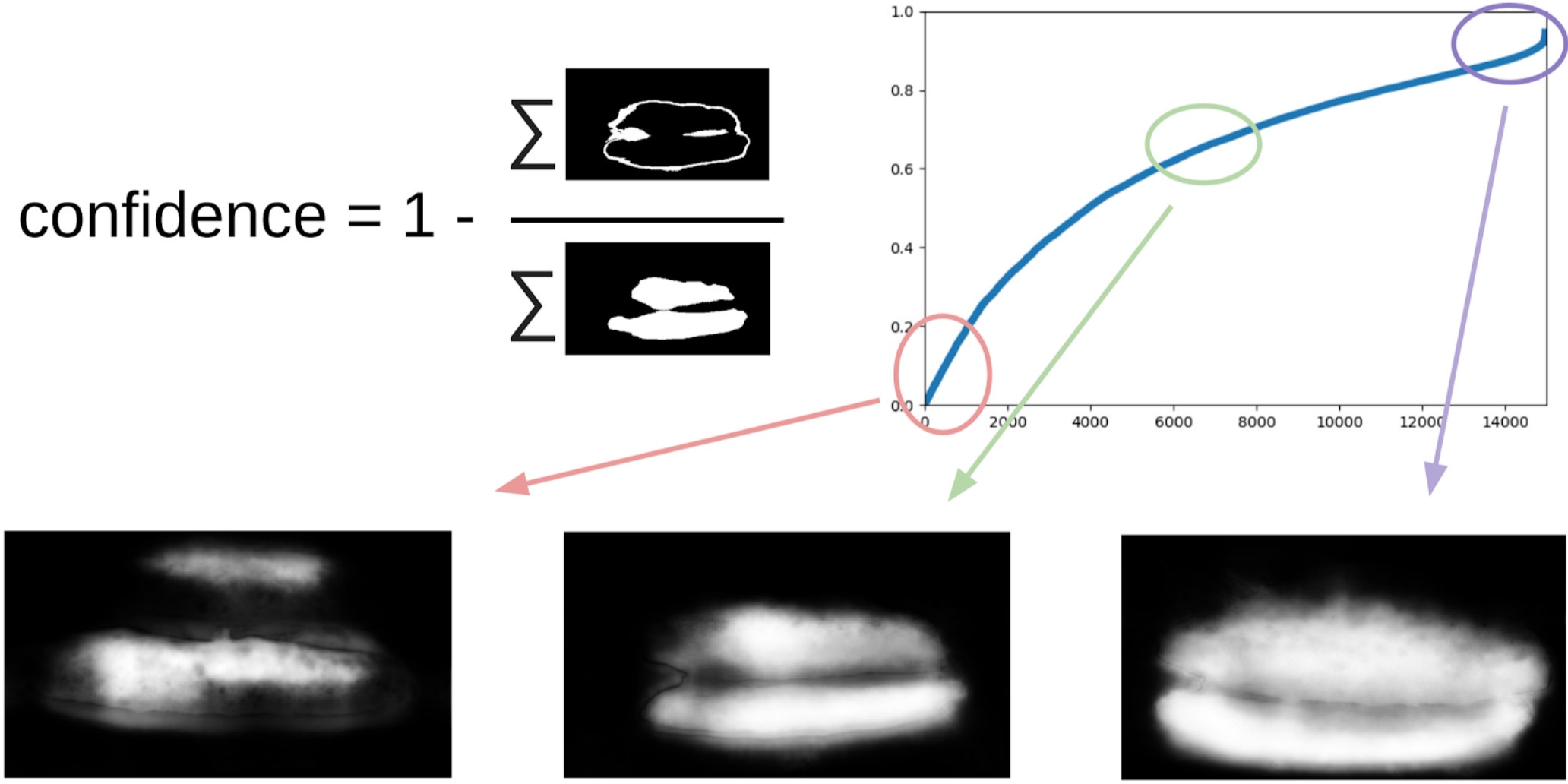
Next you need to use your eyes to choose a certain threshold of confidence, starting from which we form a new train. And optionally you can mark the most difficult samples with which the ensemble failed. I decided that it would be useful, and painted about 20 pictures somewhere while digesting dinner.
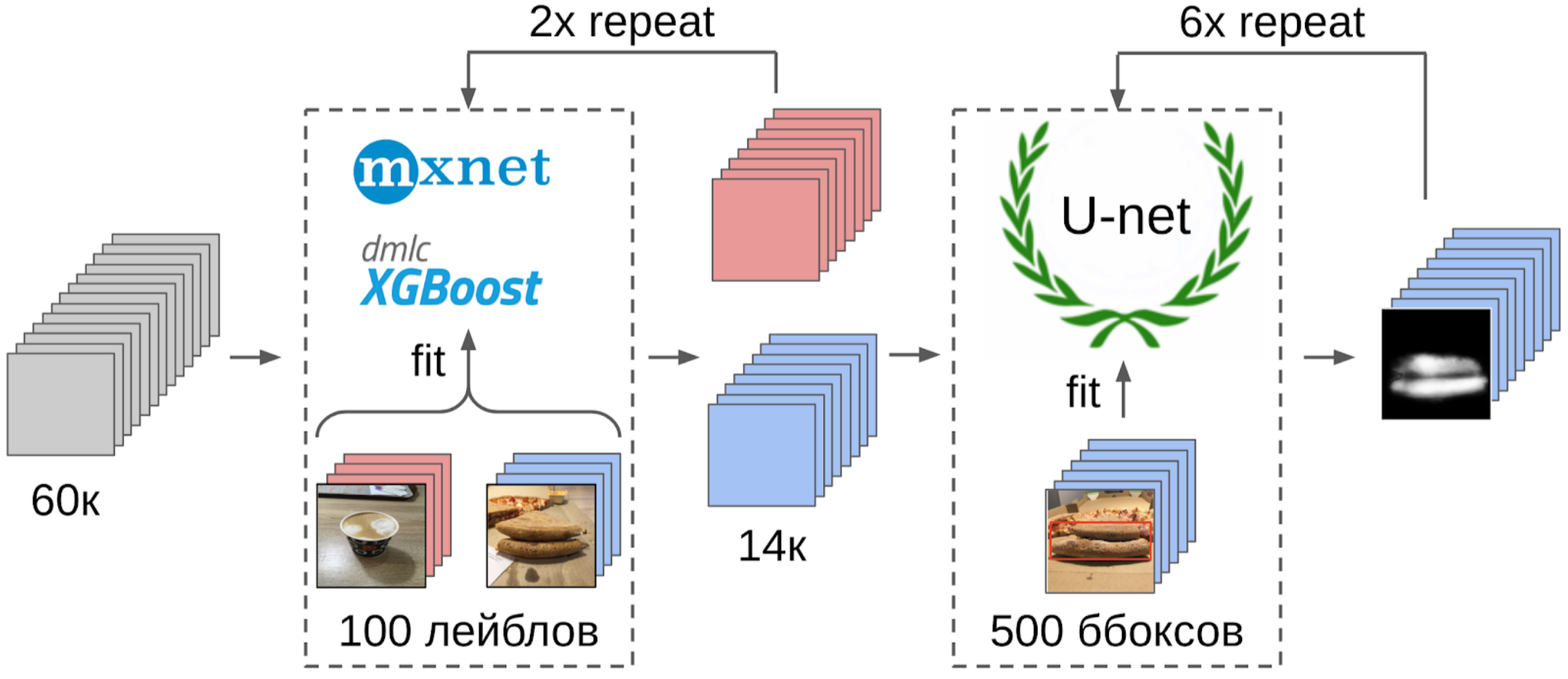
And now the final part of the pipeline: learning the model. To prepare the samples, I extracted the area of the cake on the mask. I also fanned the mask a little and applied it to the image to remove the background, since there should not be any information about the quality of the test. And then I just refilled some models from the Imagenet Zoo. In total, I managed to collect about 12k confident samples. Therefore, I did not teach the entire neural network, but only the last group of convolutions, so that the model would not retrain.
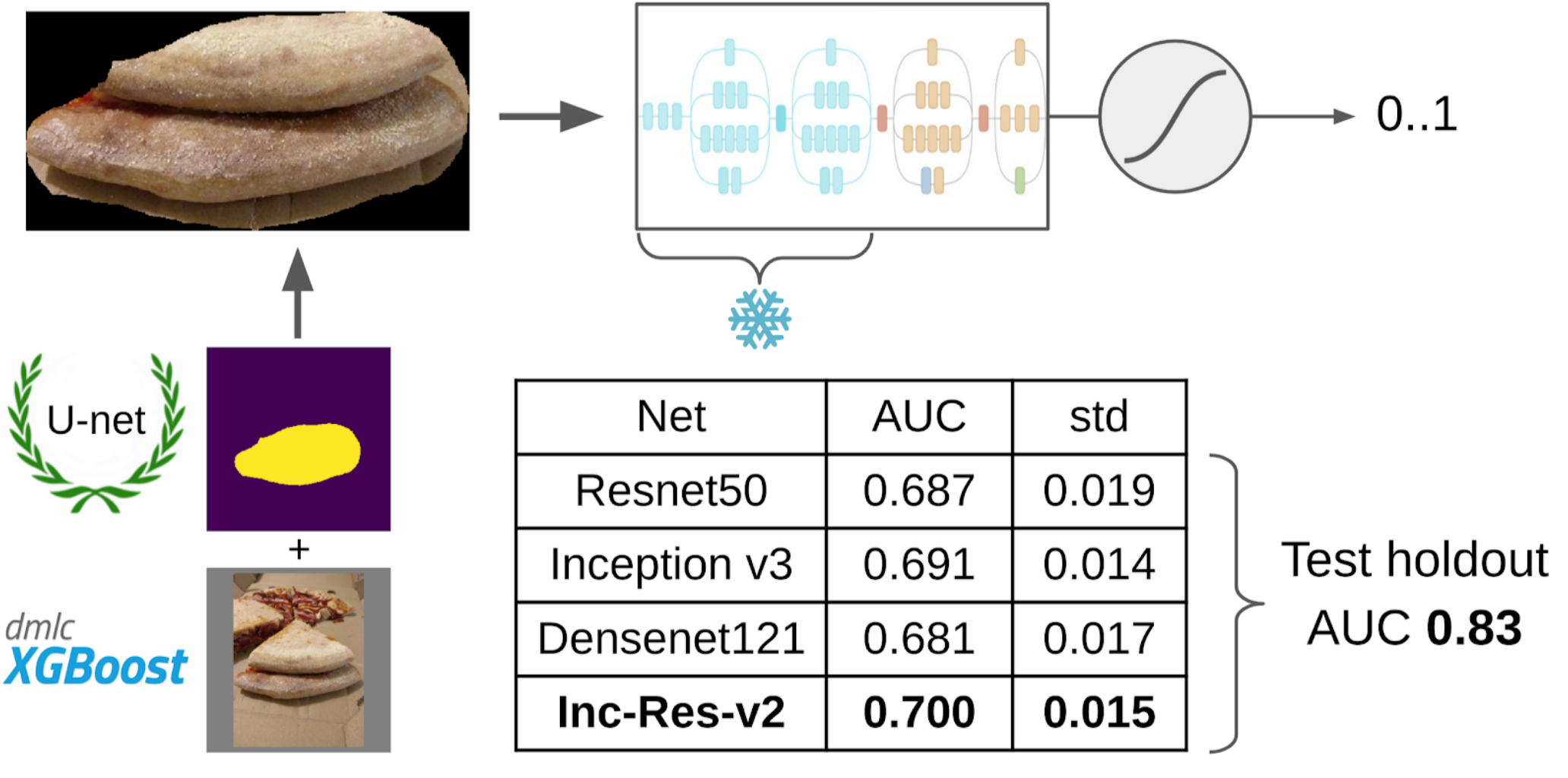
Inception-Resnet-v2 turned out to be the best single model, and for it the ROC-AUC on one fold was 0.700. If you don’t select anything and feed raw images as they are, then ROC-AUC will be 0.58. While I was developing a solution, DODO pizza cooked the next batch of data, and it was possible to test the entire pipeline on an honest holdout. We checked the entire pipeline on it and got a ROC-AUC 0.83.
Let's now look at the errors:
Top False Negative
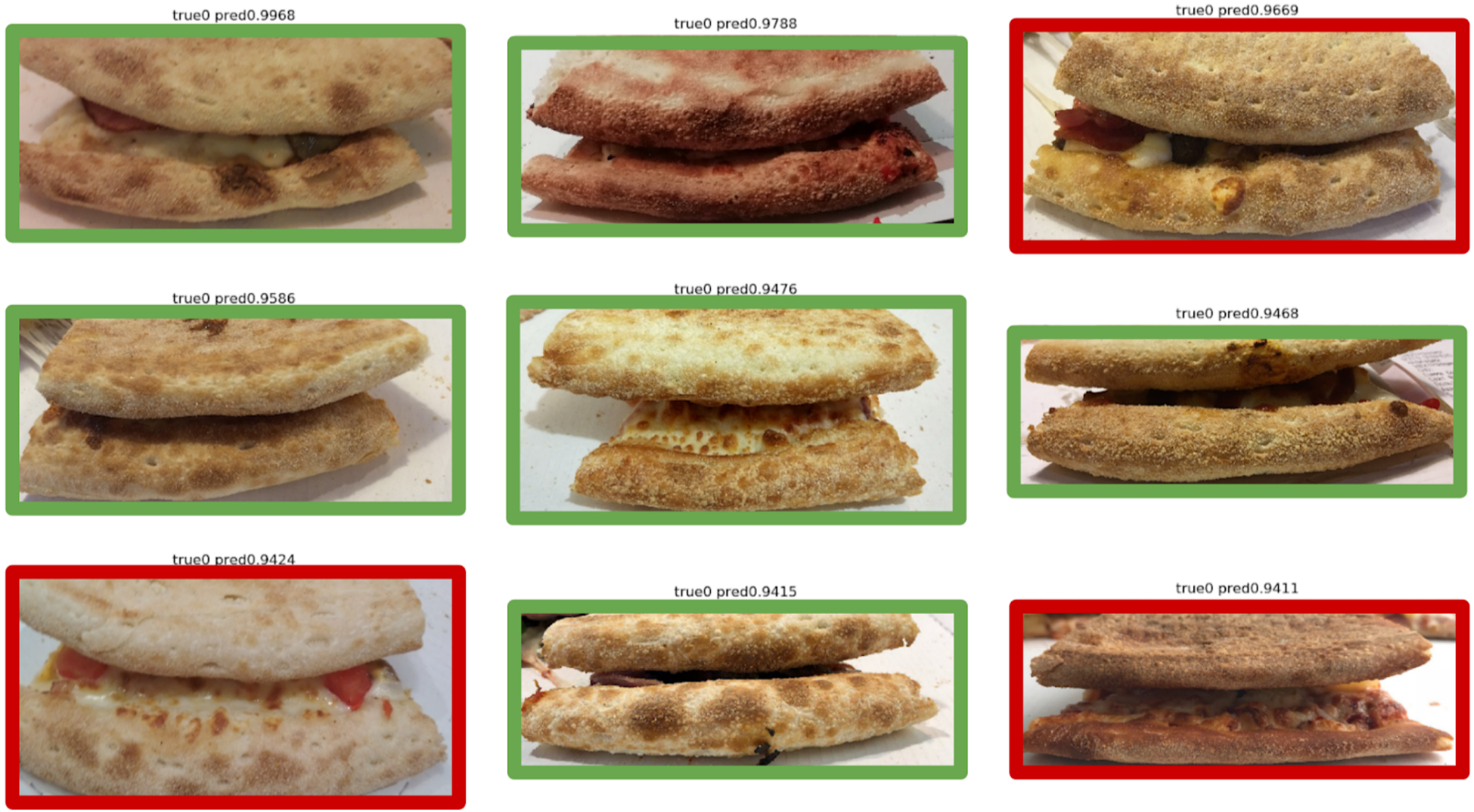
Here you can see that they are associated with the error markup shortcake, since there are clearly signs of a broken test.
Top False Positive
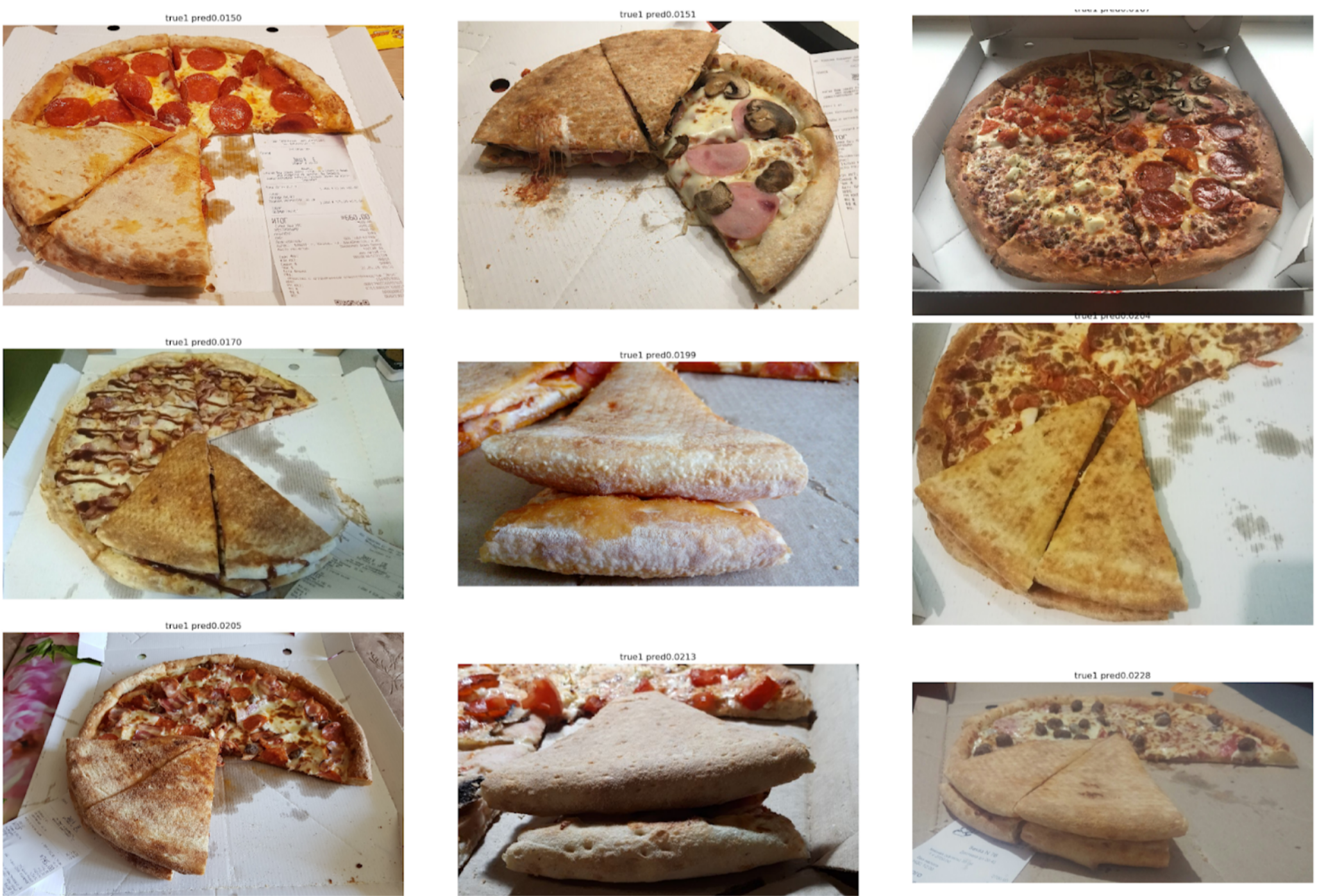
Here the errors are due to the fact that the first model selected a not very good angle, which is difficult to find the key signs of the quality of the test.
Colleagues sometimes tease me that I solve many problems by segmentation using Unet. However, in my opinion, this is quite a powerful and convenient approach. It allows you to visualize model errors and the confidence of its predictions. In addition, the entire pipeline looks very simple and now there are a lot of repositories for any framework.
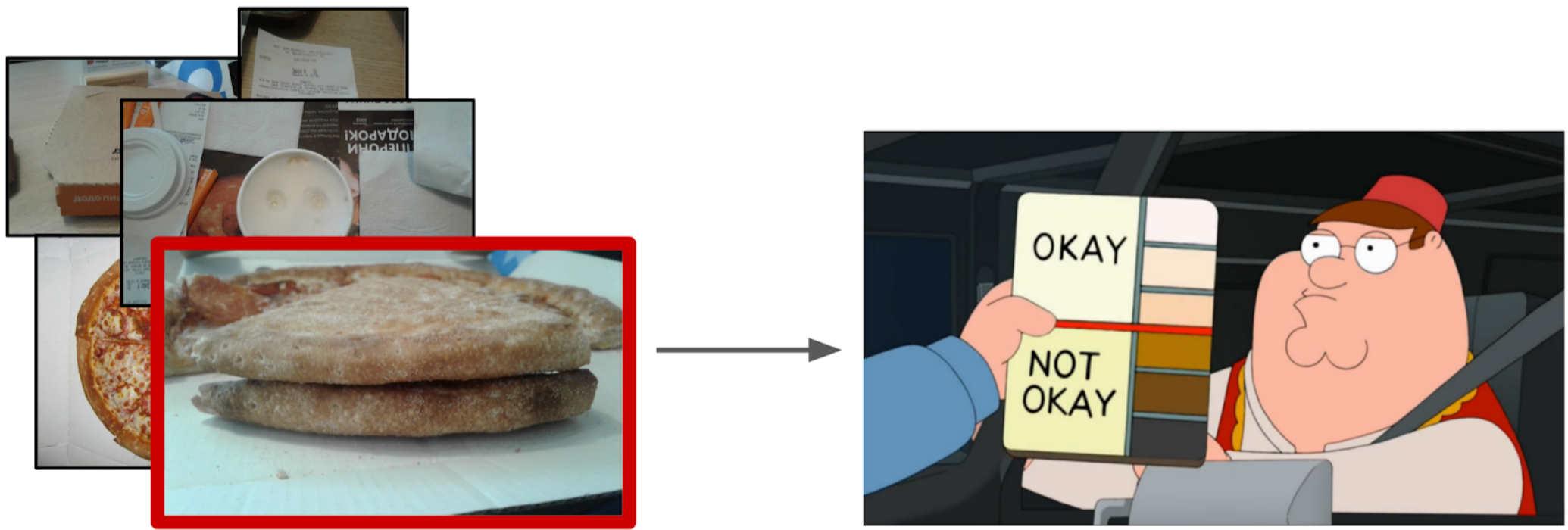
Task
There is a certain process of cooking pizza and photos from its different stages (including not only pizza). It is known that if the dough recipe is spoiled, then there will be white bumps on the cake. There is also a binary markup of the quality of the dough of each pizza made by experts. It is necessary to develop an algorithm that will determine the quality of the test on the photo.
Dataset consists of photos taken from different phones, in different conditions, different angles. Copies of pizza - 17k. Total photos - 60k.
In my opinion, the task is quite typical and well suited to show different approaches to handling data. To solve it you need to:
1. Select photos where there is a pizza shortbread;
2. On the selected photos to highlight the cake;
3. Train the neural network in the selected areas.
Photo Filtering
At first glance, it seems that the easiest thing would be to give this task to the markers, and then to train on clean data. However, I decided that it would be easier for me to mark out a small part myself, than to explain with a marker, which angle was the right one. Moreover, I did not have a hard criterion for the correct angle.
Therefore, this is how I did:
1. Mark out 100 pictures of the edge;
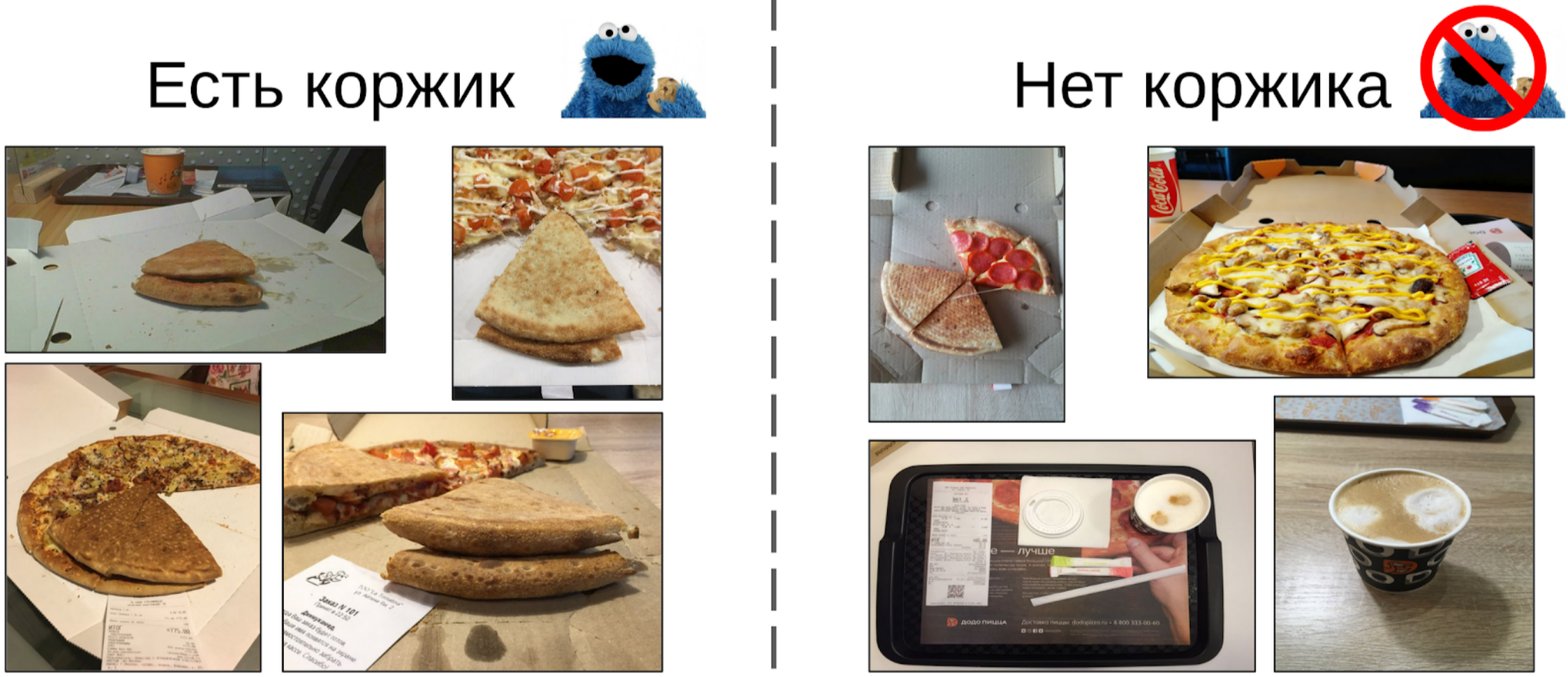
2. I counted features after a global pulling from the resnet-152 grid with weights from imagenet11k_places365;
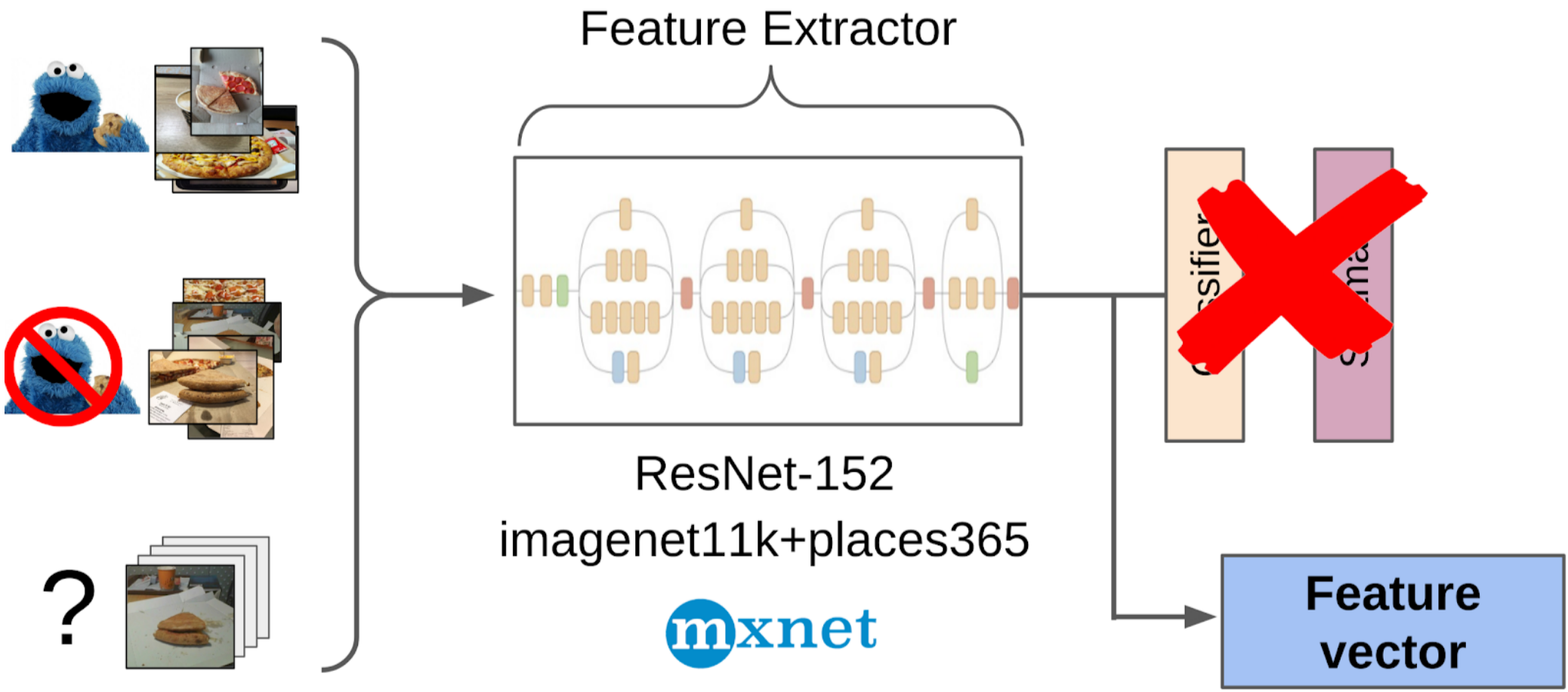
3. I took the average of the features of each class, getting two anchors;
4. I calculated the distance from each anchor to all features of the remaining 50k photos;
5. Top 300 in proximity to one anchor are relevant to the positive class, top 500 closest to the other anchor - negative class;
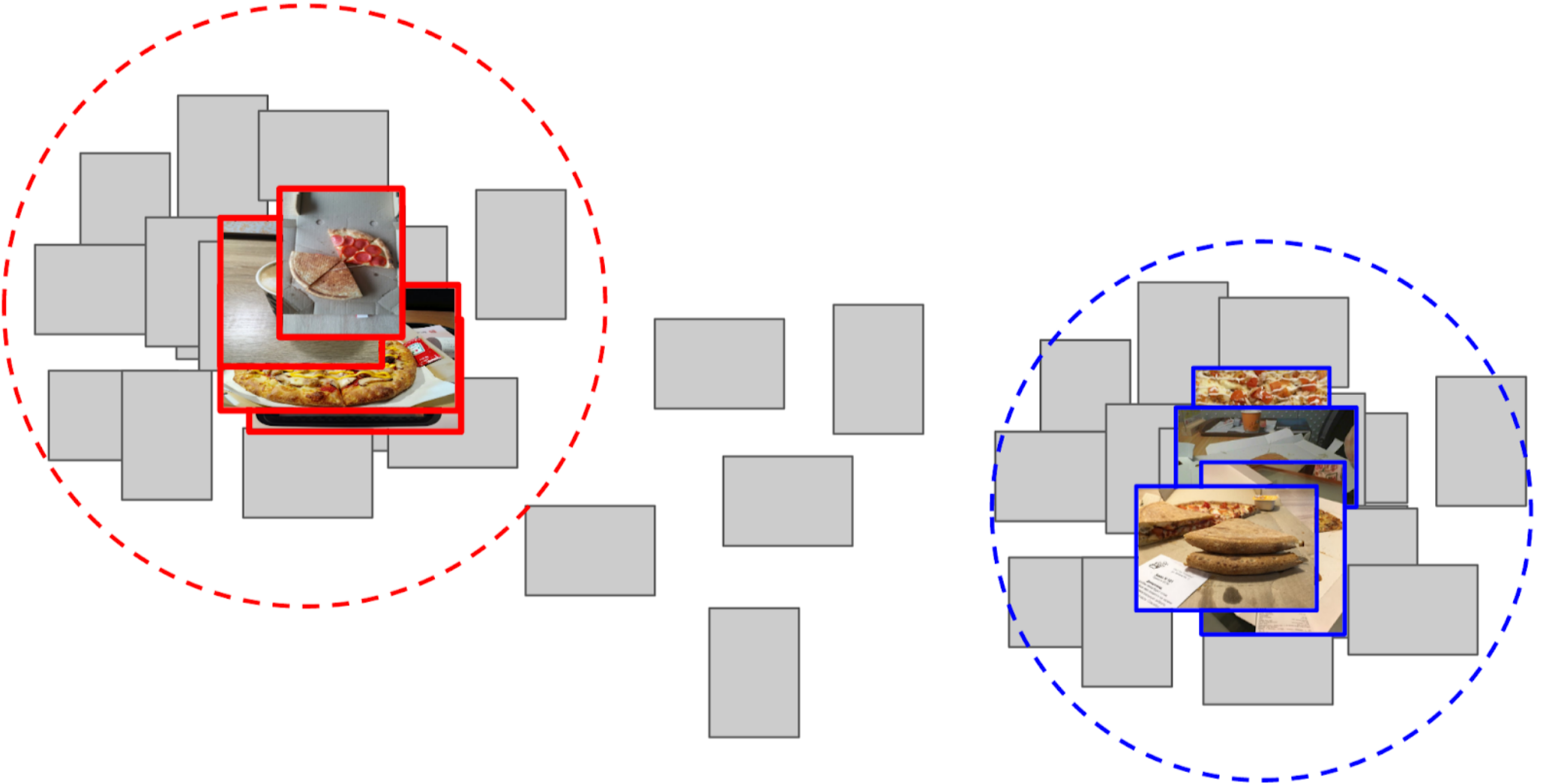
6. In these samples I taught LightGBM on the same features (XGboost is shown in the picture, because it has a logo and is more recognizable, but LightGBM does not have a logo);
7. With the help of this model I got the markup of the whole dataset.

Approximately the same approach I used in kaggle competitions as a baseline .
The explanation on the fingers why this approach works at all
Нейросеть можно воспринимать как сильно нелинейное преобразование картинки. В случае классификации картинка преобразуется в вероятности классов, которые были в обучающей выборке. И эти вероятности по сути можно использовать как фичи для Light GBM. Однако это достатоно бедное описание и в случае пиццы мы будет таким образом говорить, что класс коржика это условно 0.3 кошки и 0.7 собаки, а треш это все остальное. Вместо этого можно использовать менее разреженные фичи, после Global Average Pooling. Они обладают таким свойством, что из сэмплов обучающей выборки генерият фичи, которые должны разделяться линейным преобразованием (полносвязным слоем с Softmax'ом). Однако из-за того, что в трейне imagenet'a не было пиццы в явном виде, то чтобы разделить классы новой обучающей выборки, лучше взять нелинейное преобразование в виде деревьев. В принципе, можно пойти еще дальше и взять фичи из каких-то промежуточных слоев нейросети. Они будут лучше в том, что не еще не потеряли локальность объектов. Но они сильно хуже из-за размера вектора фичей. И кроме того менее линейны, чем перед полносвязным слоем.
Small lyrical digression
In ODS recently complained that no one writes about their Fails. Correcting the situation. About a year ago, I participated in the Kaggle Sea Lions competition with Yevgeny Nizhibitsky . The task was to calculate the fur seals in the pictures from the drone. The markup was given simply in the form of the coordinates of the carcasses, but at some point Vladimir Iglovikov marked them with boxes and generously shared this with the community. At that time, I considered myself the father of semantic segmentation (after Kaggle Dstl ) and decided that Unet would greatly facilitate the task of counting if I learned how to distinguish the seals coolly.
Explanation of semantic segmentation
Семантическая сегментация это по сути попиксельная классификация картинки. То есть каждому исходному пикселю картинки нужно поставить в соответствие класс. В случае бинарной сегментации (случай статьи), это будет либо положительный, либо отрицательный класс. В случае многоклассовой сегментации, каждому пикселю вставится в соответствие класс из обучающей выборки (фон, трава, котик, человек и т.д.). В случае бинарной сегментации в то время хорошо себя зарекомендовала архитектура нейросети U-net. Эта нейросеть по структуре похожая на обычный энкодер-декодер, но с пробросами фичей из энкодер-части в декодер на соответствующих по размеру стадиях.

В ванильном виде, правда, ее никто уже не использует, а как минимум добавляют Batch Norm. Ну и как правило берут жирный энкодер и раздувают декодер. Еще на смену U-net-like архектурам сейчас пришли новомодные FPN сегментационные сетки, которые показывают хороший перфоманс на некоторых задачах. Однако Unet-like архитектуры и по сей день не потеряли актуальность. Они хорошо работают как бейзлайн, их легко обучать и очень просто варьировать глубину / размер нейрости меняя разные экнкодеры.

В ванильном виде, правда, ее никто уже не использует, а как минимум добавляют Batch Norm. Ну и как правило берут жирный энкодер и раздувают декодер. Еще на смену U-net-like архектурам сейчас пришли новомодные FPN сегментационные сетки, которые показывают хороший перфоманс на некоторых задачах. Однако Unet-like архитектуры и по сей день не потеряли актуальность. Они хорошо работают как бейзлайн, их легко обучать и очень просто варьировать глубину / размер нейрости меняя разные экнкодеры.
Accordingly, I began to teach segmentation, having as a target in the first stage only box seals. After the first stage of training, I predicted the train and looked at what the predictions look like. With the help of heuristics, it was possible to choose from an abstract confidence mask (confidence of predictions) and conditionally divide the predictions into two groups: where everything is good and where everything is bad.
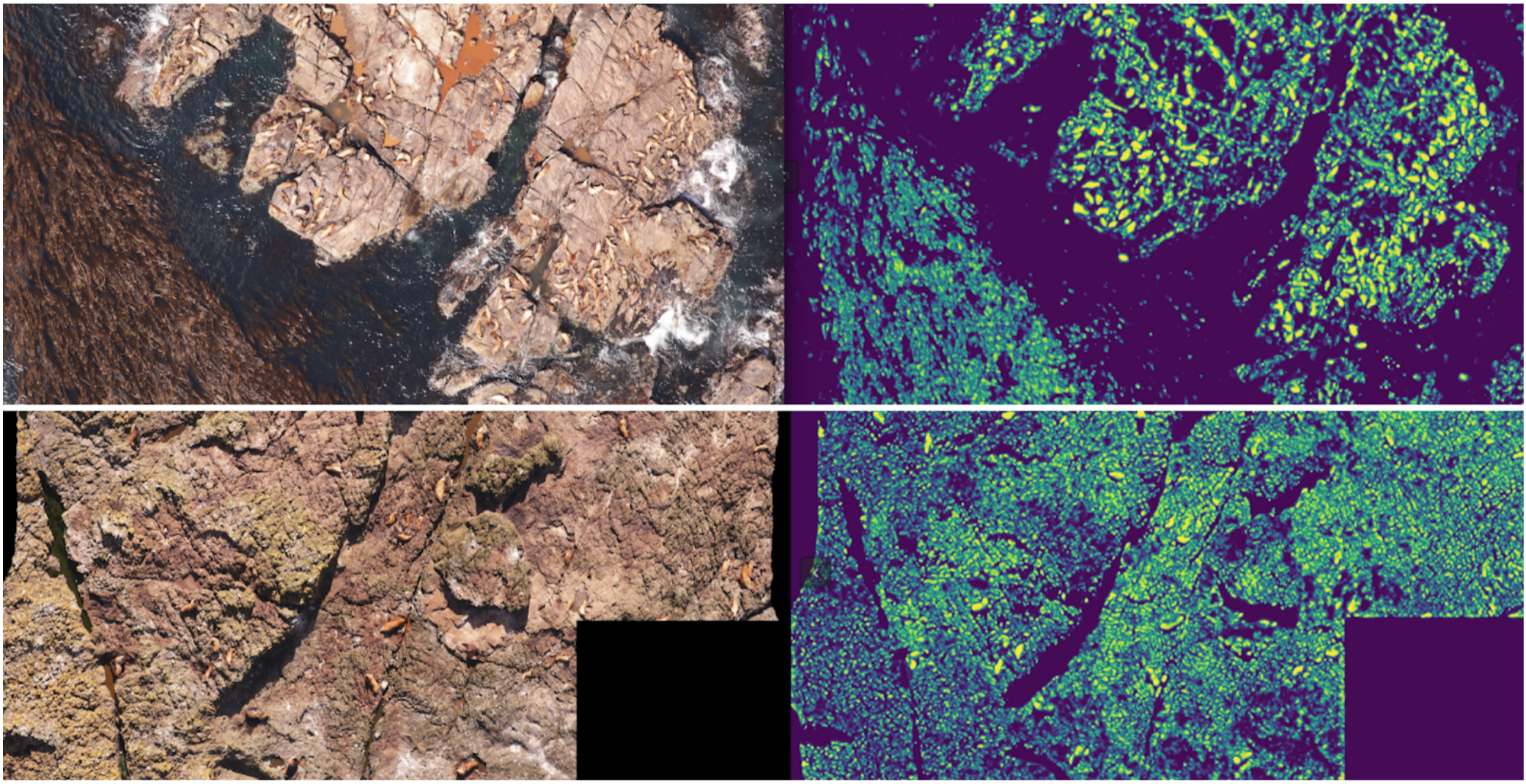
Predictions where everything is good could be used to train the next iteration of the model. Predicts, where everything is bad, it was possible to choose with large areas without seals, to manually mask the masks and also to sink into the train. And so iteratively, we with Eugene taught a model that has learned to even segment the seals of sea lions for large individuals.
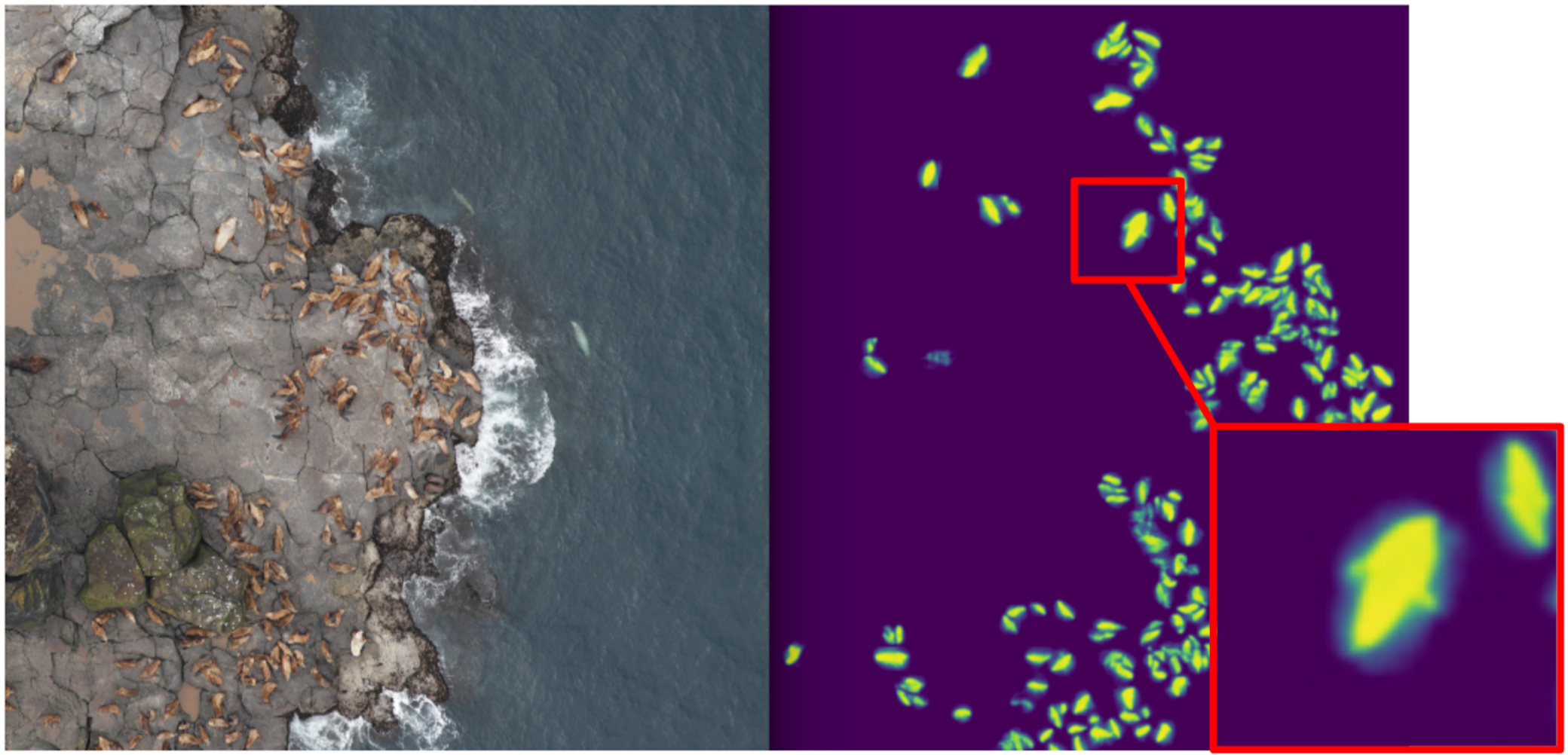
But it was a fierce fayl: we spent a lot of time trying to learn how to segmented the seals abruptly and ... It almost did not help in their calculation. The assumption that the density of seals (the number of individuals per unit area of the mask) is constant did not work, because the drone flew at different heights, and the pictures had a different scale. And at the same time, the segmentation still did not single out individual individuals, if they lay tight - which happened quite often. And before the innovative approach to the division of objects of the Tocoder team on the DSB2018, there was still a year. As a result, we stayed at the broken trough and finished in 40th place of 600 teams.
However, I made two conclusions: semantic segmentation is a convenient approach for visualizing and analyzing the operation of the algorithm, and you can weld masks out of the boxes with some effort.
But back to the pizza. In order to select a cake in the selected and filtered photos, the best option would be to give the task to the markers. At that time, we had already implemented the boxes and the consensus algorithm for them. So I just threw a couple of examples and put it on the markup. In the end, I got 500 samples with exactly selected area of the cake.
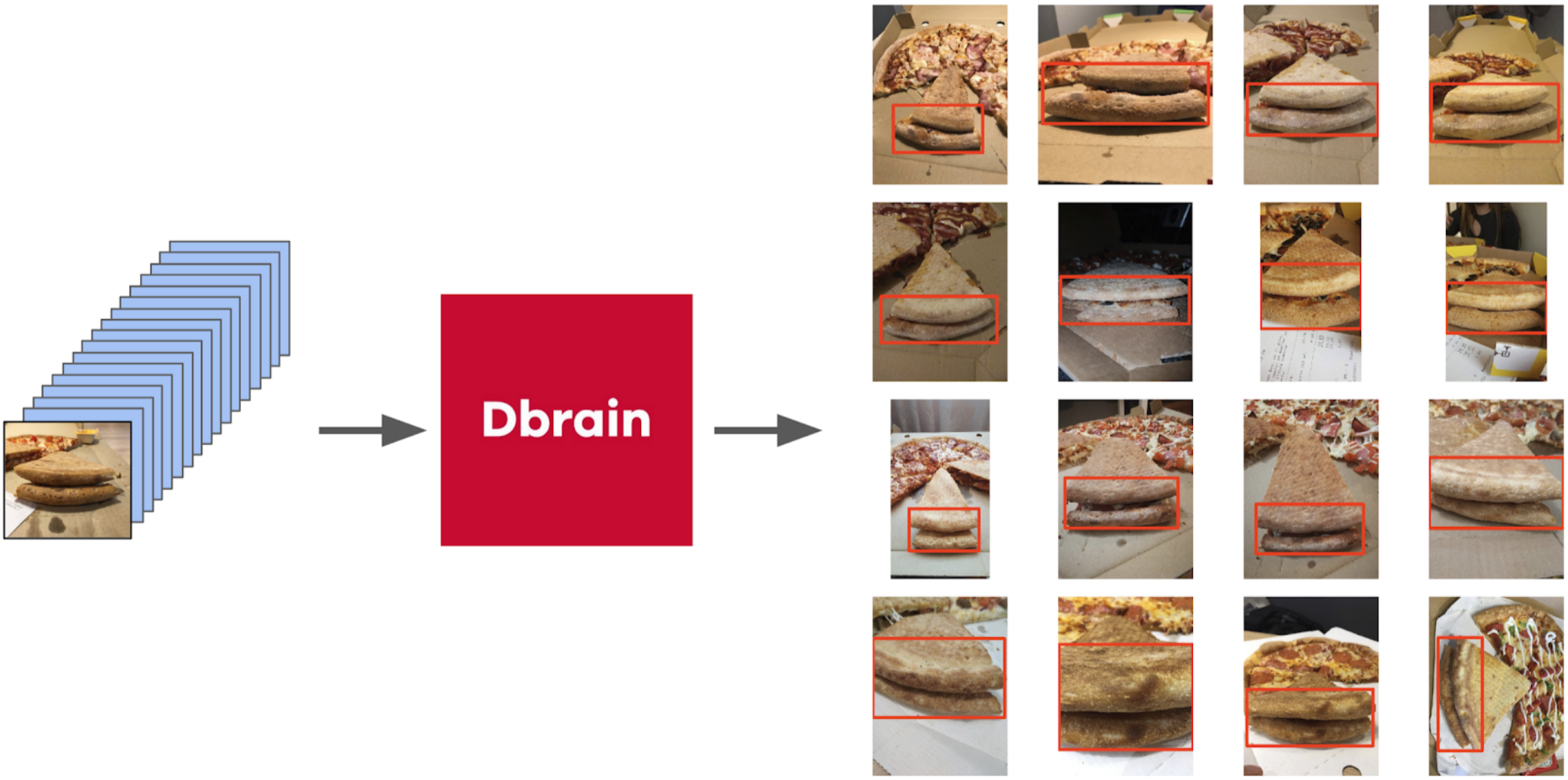
Then I dug out my code from the seals and more formally approached the current procedure. After the first iteration of the training, it was just as well seen where the model is mistaken. And the confidence of predictions can be defined as:
1 - (area of the gray area) / (area of the mask) # there will be a formula, I promise
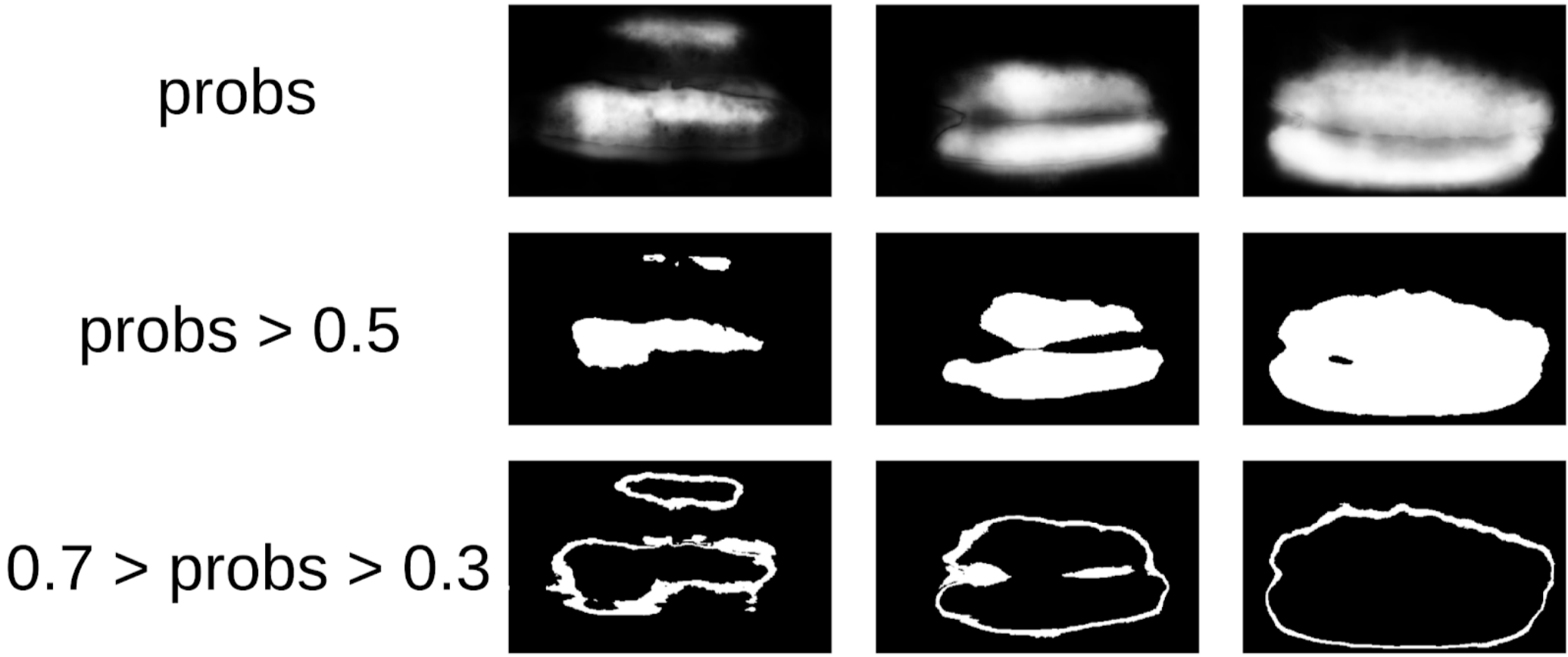
Now, in order to do the next iteration of tensioning the boxes on the masks, we will predict a small ensemble of a train with a TTA. This can be considered to some extent WAAAAGH knowledge distillation, but it is more correct to call it Pseudo Labeling.
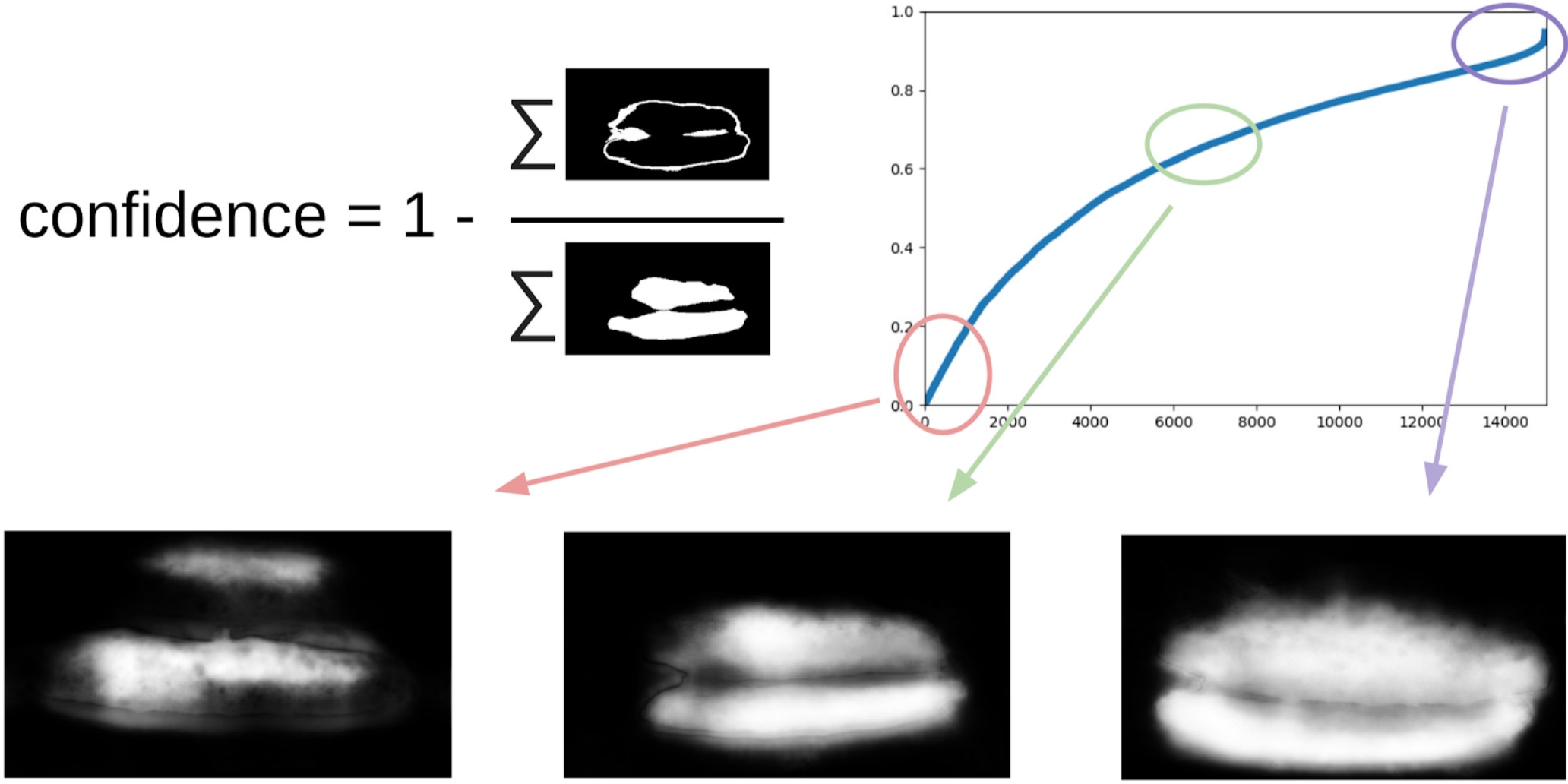
Next you need to use your eyes to choose a certain threshold of confidence, starting from which we form a new train. And optionally you can mark the most difficult samples with which the ensemble failed. I decided that it would be useful, and painted about 20 pictures somewhere while digesting dinner.
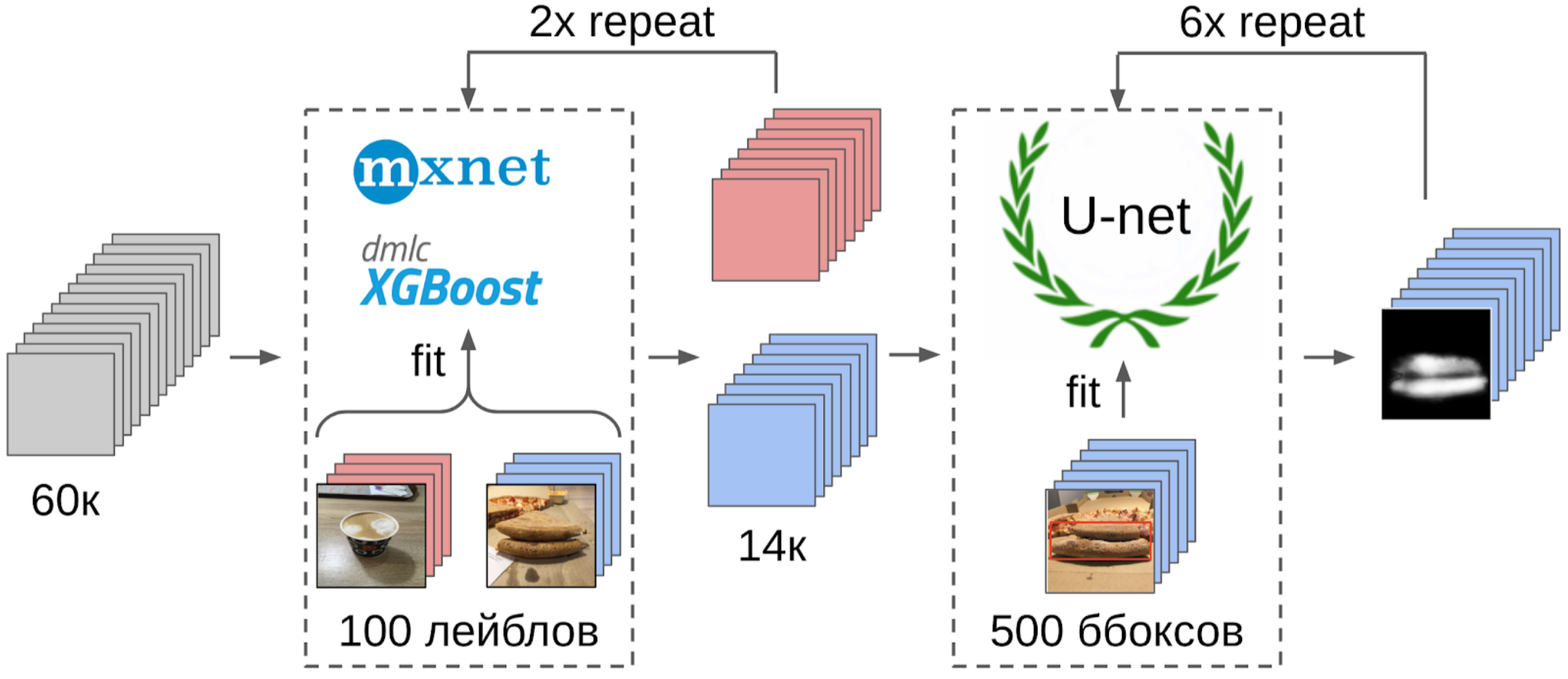
And now the final part of the pipeline: learning the model. To prepare the samples, I extracted the area of the cake on the mask. I also fanned the mask a little and applied it to the image to remove the background, since there should not be any information about the quality of the test. And then I just refilled some models from the Imagenet Zoo. In total, I managed to collect about 12k confident samples. Therefore, I did not teach the entire neural network, but only the last group of convolutions, so that the model would not retrain.
Why do you need to freeze layers
От этого есть два профита: 1. Сеть обучается быстрее, поскольку не нужно считать градиенты для замороженных слоев. 2. Сеть не переобучается, поскольку у нее теперь меньше свободных параметров. При этом утверждается, что первые несколько групп сверток в ходе обучения на Imagenet генерят достаточно общие признаки типа резких цветовых переходов и текстур, которые подходят для очень широкого класса объектов на фотографии. А это значит, что их можно не обучать в ходе Transer Learning'a.
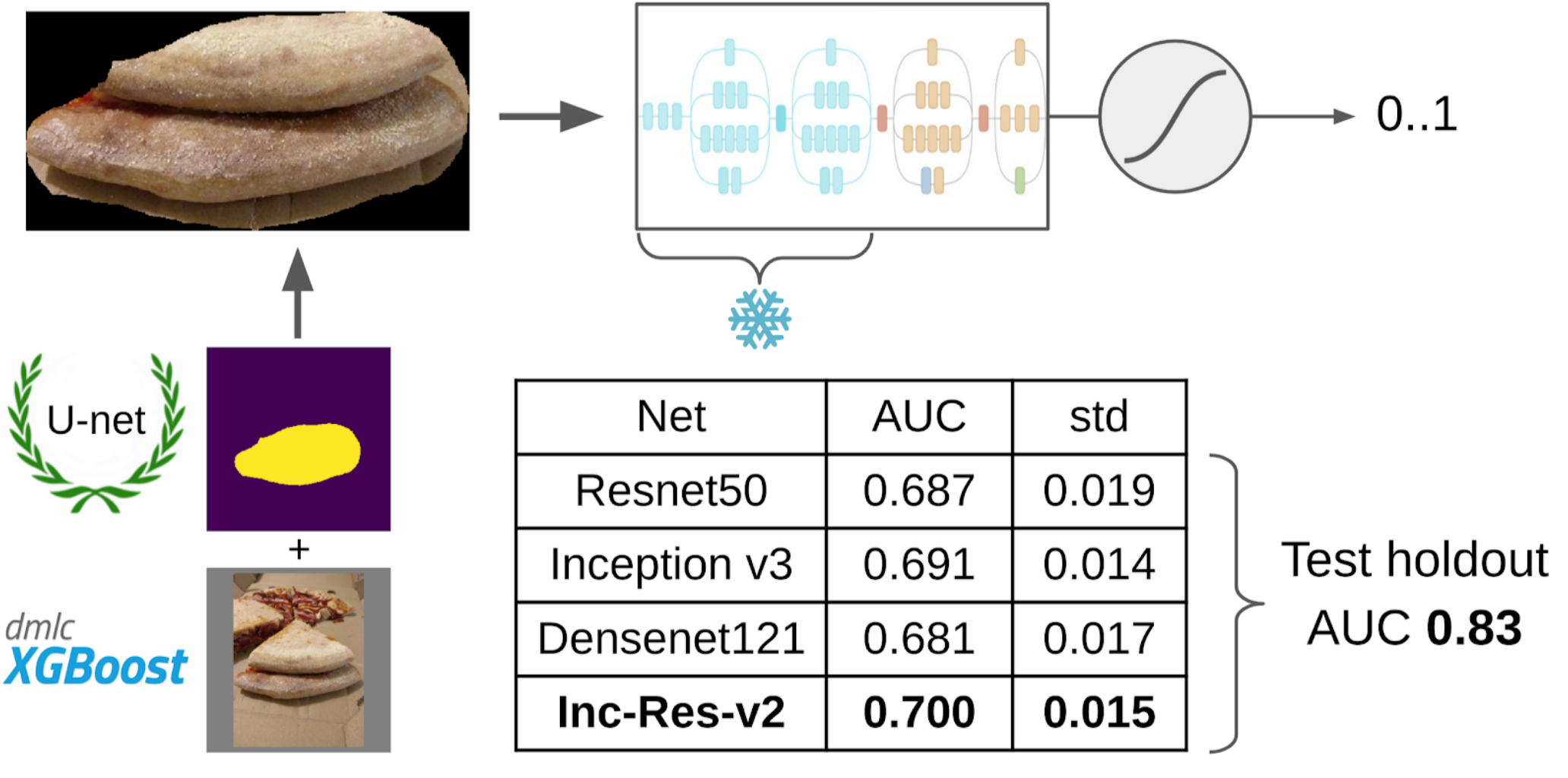
Inception-Resnet-v2 turned out to be the best single model, and for it the ROC-AUC on one fold was 0.700. If you don’t select anything and feed raw images as they are, then ROC-AUC will be 0.58. While I was developing a solution, DODO pizza cooked the next batch of data, and it was possible to test the entire pipeline on an honest holdout. We checked the entire pipeline on it and got a ROC-AUC 0.83.
Let's now look at the errors:
Top False Negative
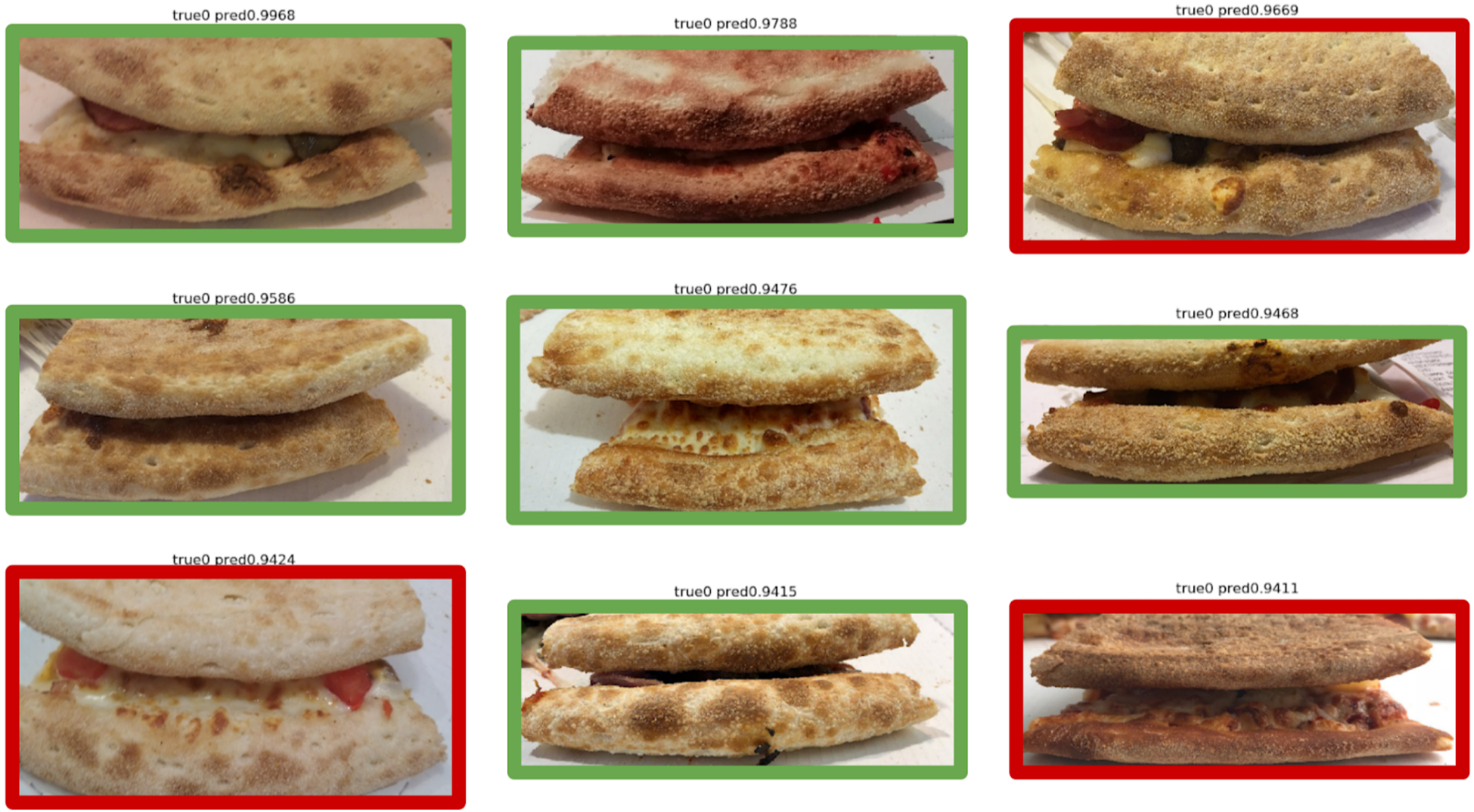
Here you can see that they are associated with the error markup shortcake, since there are clearly signs of a broken test.
Top False Positive
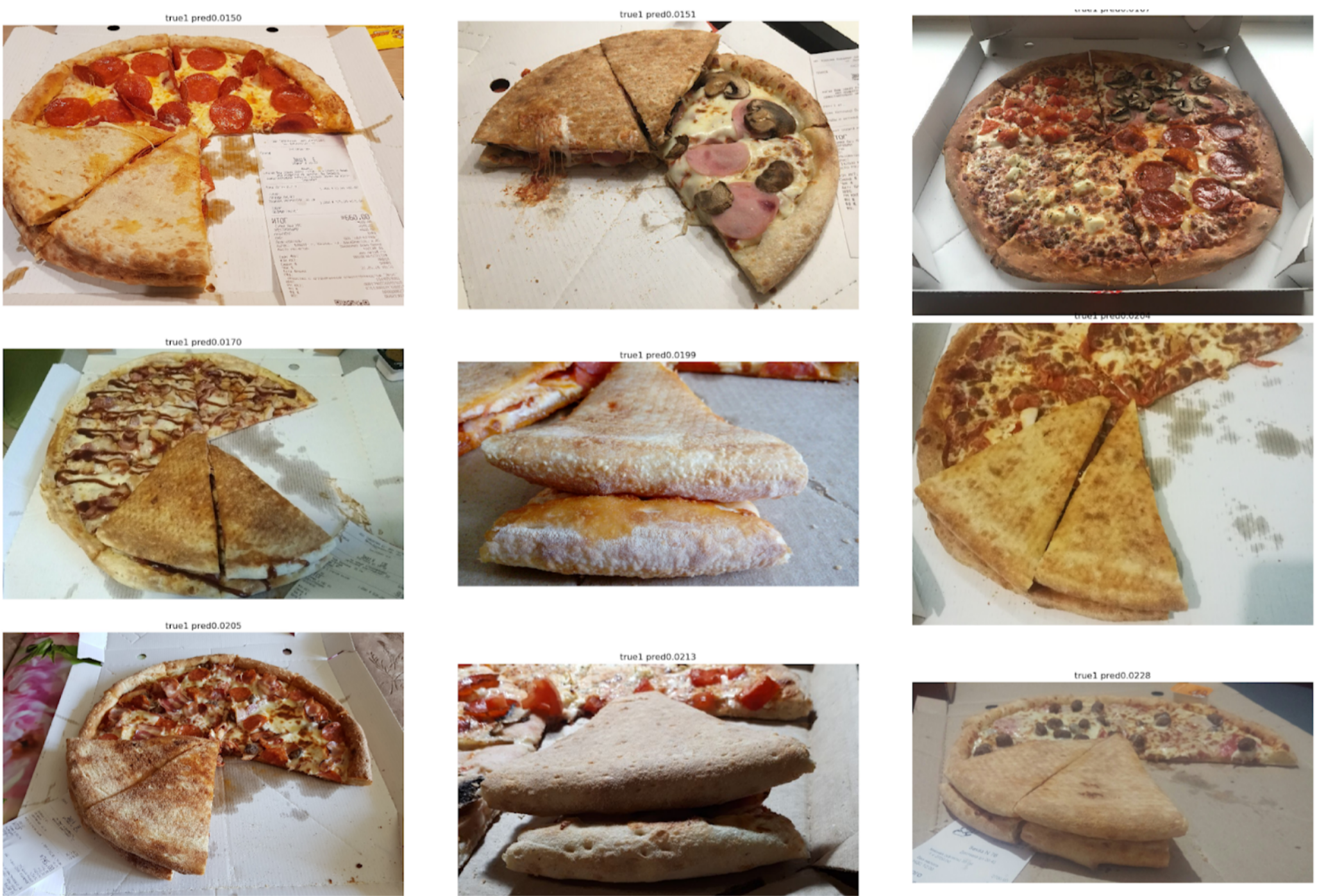
Here the errors are due to the fact that the first model selected a not very good angle, which is difficult to find the key signs of the quality of the test.
Conclusion
Colleagues sometimes tease me that I solve many problems by segmentation using Unet. However, in my opinion, this is quite a powerful and convenient approach. It allows you to visualize model errors and the confidence of its predictions. In addition, the entire pipeline looks very simple and now there are a lot of repositories for any framework.