Artificial neural networks grow navigation cells like in the brain
- Transfer

Having the ability to find a shortcut, the most direct from point "A" to point "B" does not look like an impressive test of reasonableness today. However, according to a new report that was published in Nature magazine some time ago, in which researchers told about their navigation system of artificial intelligence, the ability to explore complex simulated spaces and find the shortest route to a goal puts systems of this kind to the same level and other animals.
Surprisingly, the key to the desired performance was that when training a network spontaneously cultivated equivalent " lattice neurons » (grid cells) - a plurality of cells in the brain, which allows a number of mammalian track their position in space.
For neuroscientists, this work may suggest an important link in understanding how the neurons of the lattice in the living brain allow us to develop navigation abilities. The work also shows how neural networks can have a big impact on future research. Neil Burgess, of University College London, who did not take part in the study, suggested that such systems should "provide a fertile ground for understanding how and why the brain works in this way."
Meanwhile, for researchers in the field of artificial intelligence, the benefits of this work for improving automated navigation systems are obvious. But it can make even greater contribution to the general principles of understanding intelligence and the development of intelligent systems.
According to researchers Andrea Banino from DeepMind and Kaswell Barry from University College London, who were the main authors of an article in Nature, the project evolved from the question of the function of the neurons of the lattice, which they studied. Lattice neurons are often called the brain's GPS because of their important role in the navigation of many animals. Edward Moser and May-Britt Moser received the Nobel Prize in 2014, for their discovery nine years earlier. These clusters of neurons, organized in hexagonal arrays, together give results similar to inertial navigation systems of ships, airplanes and rockets — they give an understanding of body movements in space even in complete darkness. “Relatively speaking, they update your prediction of where you are based on how you move,” added Barry.
For this reason, neuroscientists attribute to the neurons of the lattice the function of “path integration” - a subconscious intuitive form of navigation that does not take into account external signals: for example, “take five steps forward, turn 90 degrees to the left, and again go forward another 15 steps”. But some experiments gave clues that these neurons perform other functions, even beyond navigation. For example, some experiments showed the participation of this type of neurons in such tasks as the measurement of time and distance during movement. As Barry notes, if lattice neurons provide spatial reference of objects and places, then “in principle you can use them to compute direct routes between these points,” that is, what is essentially called “vector navigation”.
To study the role of these neurons in navigation tasks, the researchers decided to use neural networks with deep learning. To understand how pathfinding works, they first created a neural network for an agent that moves in a small, simulated space. “We wanted to understand whether we could create a neural network in such a way that it itself began to develop a semblance of neurons,” said Barry.
The neural network did the job, and according to Barry, “it’s surprising how well it worked.” In the process of work, “grid units” spontaneously emerged, which were strikingly similar to what we see in the brain of animals, up to the format of a hexagonal grid.

These images show the responses of live and artificial neurons. Spontaneously arising in the neural network for navigation tasks, the lattice modules are strikingly similar to the neurons of the lattice in the brain, up to their hexagonal shape.
The researchers then added neural network capabilities to the system that helped simulated agents find the right path to the goal in the virtual maze. The system with the lattice modules exceeded the analogous system by an order of magnitude without them. For example, the system could understand if the passage closed before this gave a shorter path to the goal and chose it. According to Banino, this skill demonstrated that the lattice modules in the neural network provided vector navigation because they detected shorter and more direct paths based on the position of the target.
“I think that thanks to this work we were able to prove how the lattice neurons are used to create the search for the shortest path,” said Banino. Thus, the results confirm the theory that the lattice neurons in the brain are able to perform both the task of integrating the path and the task of vector navigation. Comparable experimental evidence in the case of living things will be an order of magnitude more difficult, he added.
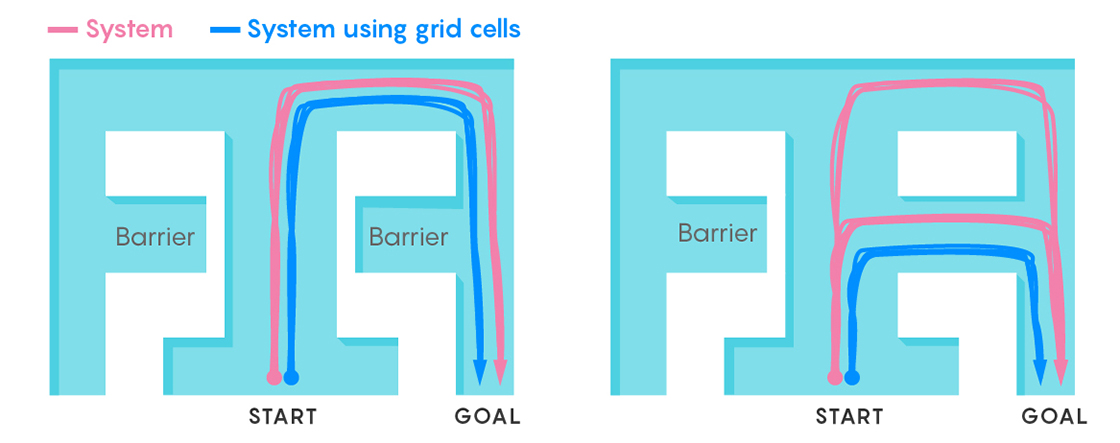
In a series of maze experiments, all tested neural networks were able to arrive at a goal. But if one of the barriers was removed, then only the network with neurons of the lattice recognized this and chose this path as the most preferable. The remaining systems continued to choose any of the available routes.
“An interesting conclusion is that a similar approach can be used for other neuroscience tasks,” said Barry. For example, researchers ponder the task of managing limbs. Neural networks could be taught to control a robotic hand, just as the brain controls a living hand, and then conduct a series of experiments that can give important clues about how these processes take place in living systems. "This approach can potentially become a universal tool in the field of neuroscience."
“This is quite an impressive result,” summarized Stefan Leitgeb, a professor of neuroscience at the University of California at San Diego. “I think they have found a very good argument that vector navigation is a function of the neuron lattice. For a long time this was only an assumption, but I think they got as close as possible to the solution of a possible mechanism. ”
At the same time, he noted that: “Any computational approach has its limitations. And the fact that it can work on a model does not mean that it works in such a way in living organisms. ”
Francesco Savelly, neuroscientists at Johns Hopkins University, who co-authored the commentaries that accompanied the article, have a similar view. He finds it very interesting that “you somehow get the neurons of the lattice without programming them directly, but they still appear as self-organization”. But at the same time, “since it is not an exact biological system, you cannot be completely sure what kind of conclusion it gives.”
“As long as you can’t look into this black box and make the network a little more like biological, at some point you will be at the limit of neurobiological research,” he said.
On the other hand, from a technical point of view, it is encouraging that "these deep learning systems can solve problems that are more and more similar to the higher cognitive functions of the brain," said Savelli. “This is a good demonstration of the fact that deep learning can be extended to include tasks like higher cognitive functions.”
We can assume that researchers from DeepMind will seek to use this navigation network to improve, say, the capabilities of research robots or future drone drones. But according to Banino, their plans are less focused and more ambitious. “We believe that navigation is one of the fundamental properties of intelligence,” he said. "Personally, we are not thinking about any use other than creating a universal algorithm."
“The brain is the only example of a universal algorithm,” he added. "So why not learn from him."