As for a flock of drones developed collective intelligence
- Transfer
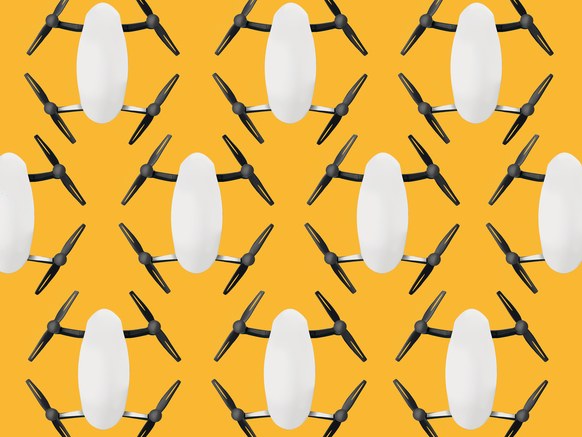
A group of 30 drones simultaneously rise into the air, and the lower parts of the bodies of their chassis glow in 30 different shades - they look like luminescent candies scattered across the gray, cloudy sky. Then they freeze suspended in the air. After a couple of seconds, they all begin to move as one.
When a newly formed flock moves, the luminous tummies of its members change by one color — green. They decide to go east. The first drones are approaching the barrier, and their tums turn greenish-blue when turning south. Soon and the color of the last members of the pack changes to the same.


It is beautiful and surprising in its own way: these drones independently organized a cohesive pack, and fly without colliding with each other, and — most surprisingly — without any central control module.
This cardinally distinguishes them from flocks of drones, which you could see somewhere at the opening of the Super Bowl or the Olympics. Yes, those quadcopter fleets can consist of thousands of units, but the movement and location of each of them is programmed in advance. And each of these 30 drones independently tracks its location, speed, and at the same time shares this information with all the other members of the pack. They have no leader; they decide together where to go - and do it, quite literally, on the fly.
In this sense, they are like birds. Or bees, or locusts. Or on any creatures capable of independently, stately and somewhat mysteriously organizing into cohesive groups - this is the emergent property of the actions of individual individuals. Several years ago, researchers were able to achieve this from 10 drones. Today this number has tripled.
But it was not just three times harder to do this. Drones are able to create a formation thanks to the realistic model of knocking down in the pack, described in the latest issue of Science Robotics. “The numbers themselves cannot express how much more difficult it was,” says Gabor Vazarely[Gabor Vásárhelyi], director of the Robotic Lab of the Department of Biological Physics at the University of Budapest and the first author of the study. “Parents who have three children, imagine how difficult it is to manage them than one child. And if you have 20-30 children, then the complexity increases by orders of magnitude. I know I have three sons. ”
The Vazareloi team developed a model based on thousands of simulations using evolutionary algorithms with hundreds of generations. “The fact that they were able to organize this in a decentralized way is very cool,” says robotics specialist from SUNY Buffalo, Kartik Danto [Karthik Dantu], an expert in coordinating the work of several robots, unrelated to this study. "Each robot is engaged in its own business, and at the same time there is a mass behavior."
In coordinated systems, an increase in the number of participants leads to an increase in the possibility of errors. A gust of wind can knock one drone off course, and the rest will follow. The quadcopter may incorrectly determine its location, or lose contact with its neighbors. Such errors cascade spread throughout the system; one small delay can be intensified by flying tracks - like a traffic jam, starting from the only braked car. Small failures can quickly create chaos.
But the team of Vazarely made such a model of churning in a flock, which can predict the maximum possible number of failures. Therefore, their drones can swarm not only in simulations, but also in the real world. “This is quite impressive,” says robotics specialist Tonns Nygaard, who is not related to research. Nygaard is a researcher in the Engineering Predictability With Embodied Cognition project at the University of Oslo, and he is working to narrow the gap between the simulations of walking robots and real artificial quadrupeds. “Simulations are great, of course,” he says, “because they make it easier to simplify the working conditions of your robots, and you can isolate and investigate problems.” But the problem is that researchers can quickly slide into oversimplification,
Instead of removing complications from their churning pattern in flocks, Basaret and the team add them. Where other models impose two or three restrictions on the work of drones, they impose 11. Together, for example, they determine how quickly a drone should line up in formation relative to other members of the fleet, how much distance it should keep to its neighbors, and how active he should try to support him.
To find the best values for all 11 parameters, Vazarely with the team used an evolutionary strategy. They created random versions of the model with 11 parameters on a supercomputer, and watched how 100 packs of drones would behave with each of the options. Then they chose the models of the most successful packs, adjusted the parameters, and restarted the simulations.
Sometimes a promising set of parameters led to a dead end. They took a few steps back, perhaps combining the properties of two different promising sets of rules, and conducted simulations again. After several years of work, 150 generations and 15,000 simulations, they came up with a set of parameters that, they were sure, should work with real drones.
And so far, these drones are doing an excellent job; tests of their model in the real world so far have not led to any collision. The flights were carried out not only brilliantly, but also in different colors - the color of the drone's chassis indicated the direction of its movement. Initially this was done for light shows with the participation of drones, but then the researchers at the last moment decided to add such an opportunity to test devices. Vazarely says that this greatly facilitated the task of visualizing the state of the drone, and also it became easier for them to notice errors and correct them in the system.
It is also very beautiful - roboluminescent visualization of complex system coordination.