The amazing creativity of digital evolution
This article contains descriptions obtained directly from researchers who have recorded similar situations in the field of artificial life and evolutionary modeling. We present significant evidence that the presence and significance of "surprises evolution" beyond the world of wildlife ...
Collection of case studies of evolutionary computation and research of artificial life
abridged translation from the English articles : of The Surprising Creativity of the Digital Evolution:
A Collection of Anecdotes from the Evolutionary Computation and Artificial Life Research Communities
The process of evolution is a swirling source of diverse adaptation options. The creative potential of evolution is not limited to the world of living nature: artificial organisms generated by computer modeling, can puzzle their own creators.
Evolutionary algorithms help to go beyond the framework on which they arise. Moreover, many researchers in this field can provide examples of how the algorithms and artificial organisms they have created, in a striking way, destroy expectations and intentions. This happens due to the manifestation of unrecognized errors in the program code and the creation of unexpected adaptation options. Or, in the behavior of digital creatures and the results of research, there is a similarity with observations in wildlife. Such stories are astonishing at the evolution taking place in the digital world, but they rarely fit into accepted scientific interpretation. Instead, they are often treated as just some of the obstacles to getting results with known goals.
Errors are eliminated, experiments change direction, and some surprising moments are reduced to a specific particular result. Such stories are transmitted orally in the environment of researchers, but this way of transmitting information is not effective, which leads to distortion and loss. At the same time, such stories spread, as a rule, among the experimenters themselves, therefore many researchers of living nature do not
often manage to get an idea of how interesting and realistic digital creatures and processes of their evolution can be.
According to our data, collections of descriptions of similar stories have not been previously published. This article contains descriptions obtained directly from researchers who have recorded similar situations in the field of artificial life and evolutionary modeling.
In this regard, we present substantial evidence that the presence and significance of the “surprises of evolution” go beyond the world of living nature, and this may in fact be a manifestation of the universal properties of all complex evolving systems.
Evolution presents us with countless examples of paradoxically creative ways of obtaining the most complex decisions concerning the variability of living nature. For example, some species of flowers have a function similar to an acoustic beacon to attract bats oriented through echolocation; extremophile microbes modify their own DNA for successful growth under
strong radiation; bombardier beetles repel raids of predators through rapid chemical reactions, parasites reprogram the brains of those creatures on which they settle, which causes the death of these creatures, but ensures the well-being of the parasites themselves.
Many other examples relate to a wide range of biological systems. And even biologists with great experience sometimes express surprise when learning about newly discovered methods of adaptation. So, the process of biological evolution is remarkable for its amazing creativity, at least in the sense of generating unimaginable and complex solutions, which, one would think, can be compared with solutions created by people. At the same time, the creativity of the processes of evolution is not limited to the sphere of the organic world. Regardless of the physical carrier, manifestations of evolution can be observed wherever such functions as replication, variability, and selection are intertwined.
In this regard, evolution can be confirmed by examples from the world of digital reality - in the form of computer programs designed for experiments on the study of evolution or in solving design problems due to the generation of digital creatures. Like biological evolution in experiments involving digital evolution, surprising results are often obtained. Sometimes evolution reveals hidden program code errors or displays a striking convergence with biological manifestations. And sometimes, through evolution, decisions arise that the researchers did not anticipate at all or that were considered impossible.
Stories related to digital evolution and “astounding” researchers who study it are more than just minor artifacts. Such cases contribute to acts of enlightenment and to obtaining knowledge useful for practical activity, since they show the omnipresence of such problems and the possibility of overcoming them
of necessity.
Moreover, such situations show that spent digital models of evolution do not simply reflect the requirements imposed by the creators, as well as certain distortions. However, they are quite reasonable for the purpose of obtaining unexpected results and new knowledge.
Creativity evolution becomes apparent when observing diversity in wildlife. Such thoughts are reflected by Darwin in the conclusion to “The Origin of Species”, where it is mentioned that observing the myriads of biological creatures living together in a single range of biological creatures causes serious reflections - “endless forms most beautiful”.
Variability in wildlife is distributed along such axes as complexity, organization, habitat, metabolism, and reproduction. At the same time, there is a connection - from single-celled prokaryotes to whale organisms containing quadrillion cells. Since the origin of life, biodiversity has penetrated everywhere, while evolution has conquered the sea, land, airspace, and all this with the generation of uncountable adaptation options.
The functionality provided by such an adaptation far exceeds the capabilities of modern intellectual design, which so far is aimed at creating robots capable of reliable self-reproduction, independent development of the real world, or intelligence at a level comparable to that of humans. However, the word “creativity” is semantically multi-valued and can have many different meanings. In order not to drown in reasoning about semantic and philosophical manifestations, and recognizing the existence of other definitions and corresponding opinions, let us take the “standard definition”: creativity generates something original (new) and effective (functional). This criterion meets many works of evolution.
In this understanding, the source of creativity in nature is the filter of reproduction. The existence of such a filter can be implemented in many different ways, and as a result of evolution a mass of heterogeneous results is formed. For example, in nature there are many ways to extract the necessary energy for the functioning of inorganic sources (such as the sun, iron, ammonia) or the various structures of the wings for flight among insects, birds, mammals and reptiles.
The potential of creativity evolution also contributes to self-tuning through environmental interaction. Moreover, the creation of a new niche is often accompanied by the discovery of other niches, through predation, symbiosis, parasitism or seizure. Although evolution reveals a lack of foresight and intentionality (the qualities inherent in human creativity), structures evolving according to one of the functions often
adapt to a different purpose for a different purpose, this phenomenon is known as exclusion.
For example, a widespread theory is that the evolution of feather carriers first turned them into dinosaurs due to thermoregulation and only later led them to adaptability to flight and the generation of birds. But even in the absence of direct foresight, research on the ability to develop suggests
that the genome architecture itself may contribute to the deviation in the direction of increasing the potential of creativity. One of the components of evolution is the pressure of selection, which causes the species to adapt to improve embedding in the environment, and that often leads to creativity within the given species. That is, coexistence
Evolutionary changes produce effective solutions, such as improving protection against predators or against natural manifestations such as wind or radiation.
Along with creativity within the species, there are also evolutionary forces that contribute to the divergence of creativity, which leads to the accumulation of new signs or niches. One such force is the negative frequency-dependent selection; such an incentive factor manifests itself when some of the signs are adapted only in a rare case, which contributes to the evolution of organisms with a demonstration of its various ways. Another factor of divergence, driven by evolutionary forces, is adaptive radiation. It manifests itself in access to new opportunities and contributes to the rapid diversification of the organism with the transformation into a number of new species, for example, when a new
opportunity arises , such as flying.
Thus, evolution is directed towards efficiency (successful adaptation and functionality), as well as towards uniqueness, through both optimizing forces of natural selection and the forces of divergence. Due to this, artifacts arise that satisfy both criteria of the standard definition of creativity. One of the goals of the article is to emphasize that such creativity is not limited to the biological environment, but is also a common feature for digital evolution.
Stimulated by the study of biological evolution, researchers in the field of digital evolution study the processes illustrated by computational methods. The main idea is that there are abstract principles underlying biological evolution that are independent of the physical carrier, and that such principles can be effectively applied and studied through computing devices.
In the words of one of the researchers, Daniel Dennett, “evolution happens always and everywhere where three conditions are combined: replication, variability (mutations) and different fitness (competition)”; it does not require the presence of particular molecules (for example, DNA or RNA) or substrates (for example, special physical shells).
In nature, heredity is organized by replicating genetic molecules, and variability by means of mechanisms similar to those that copy errors, as well as genetic recombination. Selection in biological evolution essentially depends on how much survival and reproduction are the logical conditions for the existence of the
genetic material of an organism. Comparison with digital evolution leads to the opinion that the processes that perform the functions of replication, variability and selection can be implemented on computers, in the form of evolutionary algorithms (EA).
For example, replication can be simulated simply by copying a data structure (that is, the digital genome) into memory; variability can be represented by random perturbations of elements within such a data structure. The selection in EA is possible to obtain in various ways, with the two most common being digital analogs of artificial
and natural selection in biological evolution. Similarity and difference for these types of selection pressures is important for understanding a lot of the results of digital evolution, as described in more detail later.
Artificial selection in biological evolution is used, for example, by horse manufacturers, who decide which breeds to bring together, in the hope of improving certain characteristics, for example, when mixing the fastest or smallest individuals. With this method, the selection reflects the goals set by people. Similarly, in digital evolution, a researcher may enter the fitness function as a criterion for automatic selection. The fitness function is a metric that describes which phenotypes are preferred among others, and this reflects the goal for performing digital evolution. For example, when using EA for designing
With a stable gait of a walking robot, a visual function of fitness would help measure the distance that the controlled robot will move before it falls. The selection with such an EA should be reduced to the controllers of robots that realize the greatest distance, in the hope that their descendants will be able to overcome even greater distances. This selection method is the most common in engineering applications, when the use of digital evolution is intended to achieve the desired result.
Another common method of digital selection is the use of natural selection, similar to biological, when evolution is not limited in advance. The main difference from the previous one is that with this method there is no definite specified result and a given fitness function. At the same time, digital creatures compete for limited resources, such as artificial nutrients. It requires cyclic operations of the central processor to replicate the creatures' code or the storage location on the digital media for recording genomes. When setting variability in a population, some creatures will survive long enough for reproduction with the reproduction of their genetic material, while others will disappear, this provides an evolution similar to nature.
Typically, digital evolution systems and similar experiments are not implemented directly for 4/30 engineering purposes, but are used as a means to learn the basic principles of life and evolution with simpler settings than in biology; that is, they provide models of artificial life for use in evolutionary experiments. One of the persistent misinterpretations of digital evolution is because it
is initiated on a computer carrier, then it loses its correspondence with the studies of biological evolution. However, by means of both philosophical arguments and significant specialized publications, it is suggested that digital evolution can be a useful tool that facilitates and complements the study of biological evolution. In fact, such evolving systems can be considered as real embodiments of evolution, rather than just as its simulations.
First, it may not seem obvious that a class of algorithms can systematically surprise their compilers. At the same time, we give a fairly broad definition of surprise, as the observation of a result that differs significantly from expectations, whether it is expectations due to intuition, predictions from experience, or
theoretical models. Since the algorithm is a formal list of unambiguous instructions that are executed in a certain order, it seems quite sufficient to check the description of the algorithm in order to predict the full range of possible results, counteracting any possibility for the occurrence of surprises. At the same time, from the theory of computer science it follows that for many computer programs the result of
can not be predicted without running the program. In the field of researching complex systems, it is also known that, when executed, simple programs can produce complex and sometimes even surprising results.
This is a basic fact, which, above all, is contrary to intuition. The interaction between several modern software products, which is specifically designed to produce predictable results, seems to preclude the appearance of any surprises in advance. But still, if the manifestation of striking innovations is characteristic of
biological evolution, then such manifestations should be expected from computer models that illustrate the fundamental aspects of the process of evolution. We offer informal evidence of the possibilities of digital evolution to produce unpredictable results; and the various manifestations presented below demonstrate how common and widespread such surprising results are in practice. It is also important to note such an aspect of human
psychology as a tendency to belated judgments, which often makes it difficult to understand the significance of a surprise because of its subjective perception by another person. That is, people often exaggerate how predictable an event is, after its occurrence. However, many of the following cases may trigger an understanding
results after the fact, which may cause the reader to deny some surprising manifestation.
Below are 27 interesting cases related to the work of 50 researchers.
We conditionally divided these cases into 4 categories:
When solving practical problems, the most common approach among experimenters is the choice of the fitness function, which reflects the required goal of the research. As such fitness functions, simple quantitative measurements are often set, which seem obvious in order to cover the main characteristics for obtaining a successful result.

Fig. 1 Use of potential energy for movement. Evolution reveals that it is easier to create tall structures that perform a rational fall than to look for strategies for active movement. Left: being at the beginning of the attempt. Right: moments of falling and tumbling to save momentum.
Such procedures are a trigger EA, as they serve as "funnels" for direct search. "Breeding a breed" is directed towards breeding creatures with a higher fitness rating, in the hope that this will lead to further improvements in fitness and certainly to the desired result. This is similar to the process of breeding animals and is based on the same principles of evolution to obtain the desired
result.
However, as shown below, quantitative measurements performed for good purposes are often maximized by non-obvious means. At the same time, experimenters often overestimate how accurately quantitative measurements reflect the underlying qualitative result implied by the experimenters themselves. This is an error known as a map and territory conflict (for example, the metric is a map, and the researcher implies real territory;).
Namely, it is often functionally simpler for evolution to exploit gaps in the procedure of performing quantitative measurements than to achieve the actual desired result. Also, the scorecards in human society that are precisely created with good goals can be destroyed with a direct impact on their optimization (the so-called Campbell's law or Goodhart's law). And digital evolution often acts to fulfill the letter of the law (that is, the function of fitness)
while ignoring its very meaning.
In the foundational work (1994, Karl Sims), 3-dimensional virtual creatures evolve that are able to discover such types of behavior as walking, swimming, and jumping in a simulated physical environment. The bodies of creatures are made of connected blocks. Their brains are implemented through simple neural networks that generate a variable moment on the joints based on the sensations of the limbs, with
movements that look realistic. The structure and control systems evolved simultaneously, which made it possible to obtain all kinds of bodies and movement strategies. In fact, such creatures remain the most common products of digital evolution.
However, when Sims first tried to realize the evolution of behavior during movement, difficulties arose. In a simulated area with the presence of gravity and friction, the fitness of creatures was measured as the average speed of movement over the surface over a life span of 10 simulated seconds. Instead of creating smart joints or snakelike movements, which could help push them
along the surface (as expected), the creatures became tall and stiff. When simulating, they sought to roll over, using their own initial potential energy to achieve high speed. And some even tumbled to increase the horizontal speed (Fig. 1). In order to prevent such “quirks”, it is necessary to assign a time interval at the beginning of each simulation in order to release the
potential energy inherent in the creatures in the initial position, before their movement will produce any results.

Fig. 2. Using potential energy for pole vaulting.
Evolution discovers that it is easier to create creatures that fall and roll over than to create mechanisms for activating jumps.
Based on the work of the Sims author, but using another simulation platform, the Krcah researcher led creatures to perform jumps above the surface to the maximum possible height. In the first series of experiments, the fitness of each organism was calculated as the maximum lift of the center of gravity to a height. As a result, creatures 15 cm in height were created, jumping up to 7 cm from the surface. At the same time, creatures also accidentally appeared, reaching a high degree of fitness due to the creation of a body in the form of a high static tower, providing ascent without any movement. In an attempt to correct such a gap in the next series of experiments, fitness was calculated as the greatest distance from the surface to the node that was originally closer to the surface. When evaluating quantitative experimental results,
almost tenfold improvement in jumping! At the same time, it turned out that evolution shows such a “trick” as tumbling without any jumps. The evolving body consisted of several large blocks, and resembled a head supported by a long vertical mast (Fig. 2).
At the beginning of the simulation, the creature was repelled by a long foot from the surface and began falling headfirst, with a tumbling of the foot (initially the lowest point from which the jump value was calculated) from the surface. This created a large gap between the surface and the lower point, while maintaining a high value of fitness without any training in obvious jumping skills.
Link to video .
The following examples show how failed elements of virtual creatures can become unpredictable.
EA called MAP-Elites was used to study the possible interaction of a robot arm with a small cube located on the table. The purpose of the study is the generation and accumulation of various controllers that could move the cube to all sorts of places on the table. At normal settings, MAP-Elites software is able to move the cube around the table, capture it and even throw it into the basket located in front of the
robot's hand . For the subsequent experiment, the capture of the robot was damaged, preventing its opening / closing. It was expected that the hand would be able to move the cube only in a certain way, that is, to push it stupidly. However, MAP-Elites discovered a tricky way: to push the cube only in a certain direction, encouraging the opening of the grip so that
it holds the cube securely (Fig. 4)!
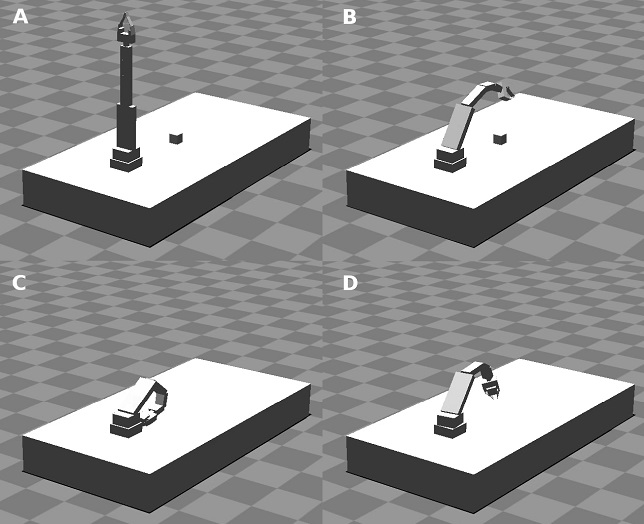
Fig. 4. (a) the robot arm is in its original position, with the gripper closed
(b) the hand pushes the cube towards the base of the hand
© © moves the cube closer to the base and performs a quick movement,
slipping on the table, causing the fingers to open and
grabbing the cube.
(d) the hand moves the cube clamped in the gripper to a new position.
Link to video .
An algorithm was presented that allows the damaged robot to successfully adapt within two minutes. Evolution was studied in the case of damage to a sixth robot - walking with damaged legs or engines (Fig. 5). Before the test, the researchers combined the digital evolution with a robot simulator for the purpose of primary learning of various walking strategies. After the damage, the robot had to use the experience gained from the simulated evolution for fast learning during tests in a real environment.
To implement the evolution of various ways of walking, the evolutionary algorithm MAP-Elites was used, which led to the search for the most fit organism for each specific combination of given dimensions (that is, possible methods implemented by phenotypes). In this case, the 6 dimensions of variation corresponded to the fractions of time (used by each of the legs); measured the relative proportion of time touching the surface of the feet of each leg. Thus, the evolutionary algorithm conducted the search for the fastest way of walking at each specific frequency of touching the surface of the feet of each leg of the sixth robot. At the same time, the variant of evolution was considered impossible, when none of the six legs touched the surface, that is, the touch corresponded to a time interval of 0%.

However, the robot turned over on his back and moved on his knees (turned back), with his feet hanging in the air! (Fig. 6).
The video demonstrates the various walking options found, including the one mentioned
(at time point 1:46).
Link to video .
Researchers Richard Watson and Sevan Ficici studied the behavior of robots, with two wheels, two engines and two sensors. Robots of this type are well known, they are mentioned in the book, where it is stated that the connection of the sensor inputs to the engine outputs, performed in a certain way, causes behavior similar to following a ray of
light. For example, when the right wheel motor moment is proportional to the intensity of light detected by the left sensor, and accordingly, for the left wheel - by the right sensor, the robot moves in the direction of the light source. In this experiment, the weights for the connections between sensor inputs and wheel speeds were determined through evolution. At the same time, the truth of the statement mentioned in the book was verified.
When the evolving robots moved in the direction of the light source, they often arrived in an unexpected way before. Some of them backed up to the source when the sensor was darkened, others searched for the source through an eccentric trajectory, rather than according to a verified statement (Fig. 7). It turned out that a similar trajectory with a circling is easier to adjust, due to the curvature or rectification of the curve when following the source. The authors found that the area of genetic search that causes circling is very broad, while the classical solution according to the statement asserted requires an exact balance (for example, with a slight change from clockwise to the opposite direction, Fig. 7) and corresponds to a relatively narrow area genetic search. Moreover, despite the observed inefficiency, spinning is maintained even at higher speeds, in contrast to the classical solution, when sufficiently fast regulation is not possible at high engine speeds. And besides, whirling is more reliable when using different drives for individual robots, as well as being less “stuck” on the sides of the test site.

Fig. 7. The robot following the light source. Left: the classic solution. Right: spinning by evolution.
Induced by biological evolution studies, digital evolution naturally exhibits similar basic principles, such as selection, variability, heritability. At the same time, there is no certainty that digital evolution will manifest itself in cases of specific behavior as well as in nature, due to a wide variety of lower
level factors : degree of mutations, genome sizes, genotype following genotypes (mapping), population sizes, morphology, types of interactions, the complexity of the environment. Moreover, in practice it is interesting to observe cases of such convergence.
In the study of virtual creatures, evolution showed strategies of movement similar to those observed in biological creatures (Fig. 11). Above shows the movement of a creature like an earthworm. Evolution rediscovers the benefits of using complementary (opposing) muscle groups, like biceps and triceps in humans, and their optimal placement. The bottom shows the result of the evolution of the bone-like material to support thinner nodes, which allows them to be longer and thinner without losing the carrying capacity. As a result, obtained something like a gallop, the usual for horses.
Fig. 11. Elements of three-dimensional image are shown in different colors:
red and green - muscle groups acting in the opposite way,
blue and blue - hard and soft supporting materials.
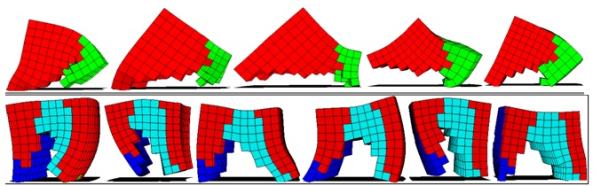
The above shows how different zones of each muscle evolve.
Crosspost
Collection of case studies of evolutionary computation and research of artificial life
abridged translation from the English articles : of The Surprising Creativity of the Digital Evolution:
A Collection of Anecdotes from the Evolutionary Computation and Artificial Life Research Communities
annotation
The process of evolution is a swirling source of diverse adaptation options. The creative potential of evolution is not limited to the world of living nature: artificial organisms generated by computer modeling, can puzzle their own creators.
Evolutionary algorithms help to go beyond the framework on which they arise. Moreover, many researchers in this field can provide examples of how the algorithms and artificial organisms they have created, in a striking way, destroy expectations and intentions. This happens due to the manifestation of unrecognized errors in the program code and the creation of unexpected adaptation options. Or, in the behavior of digital creatures and the results of research, there is a similarity with observations in wildlife. Such stories are astonishing at the evolution taking place in the digital world, but they rarely fit into accepted scientific interpretation. Instead, they are often treated as just some of the obstacles to getting results with known goals.
Errors are eliminated, experiments change direction, and some surprising moments are reduced to a specific particular result. Such stories are transmitted orally in the environment of researchers, but this way of transmitting information is not effective, which leads to distortion and loss. At the same time, such stories spread, as a rule, among the experimenters themselves, therefore many researchers of living nature do not
often manage to get an idea of how interesting and realistic digital creatures and processes of their evolution can be.
According to our data, collections of descriptions of similar stories have not been previously published. This article contains descriptions obtained directly from researchers who have recorded similar situations in the field of artificial life and evolutionary modeling.
In this regard, we present substantial evidence that the presence and significance of the “surprises of evolution” go beyond the world of living nature, and this may in fact be a manifestation of the universal properties of all complex evolving systems.
Introduction
Evolution presents us with countless examples of paradoxically creative ways of obtaining the most complex decisions concerning the variability of living nature. For example, some species of flowers have a function similar to an acoustic beacon to attract bats oriented through echolocation; extremophile microbes modify their own DNA for successful growth under
strong radiation; bombardier beetles repel raids of predators through rapid chemical reactions, parasites reprogram the brains of those creatures on which they settle, which causes the death of these creatures, but ensures the well-being of the parasites themselves.
Many other examples relate to a wide range of biological systems. And even biologists with great experience sometimes express surprise when learning about newly discovered methods of adaptation. So, the process of biological evolution is remarkable for its amazing creativity, at least in the sense of generating unimaginable and complex solutions, which, one would think, can be compared with solutions created by people. At the same time, the creativity of the processes of evolution is not limited to the sphere of the organic world. Regardless of the physical carrier, manifestations of evolution can be observed wherever such functions as replication, variability, and selection are intertwined.
In this regard, evolution can be confirmed by examples from the world of digital reality - in the form of computer programs designed for experiments on the study of evolution or in solving design problems due to the generation of digital creatures. Like biological evolution in experiments involving digital evolution, surprising results are often obtained. Sometimes evolution reveals hidden program code errors or displays a striking convergence with biological manifestations. And sometimes, through evolution, decisions arise that the researchers did not anticipate at all or that were considered impossible.
Stories related to digital evolution and “astounding” researchers who study it are more than just minor artifacts. Such cases contribute to acts of enlightenment and to obtaining knowledge useful for practical activity, since they show the omnipresence of such problems and the possibility of overcoming them
of necessity.
Moreover, such situations show that spent digital models of evolution do not simply reflect the requirements imposed by the creators, as well as certain distortions. However, they are quite reasonable for the purpose of obtaining unexpected results and new knowledge.
Evolution and creativity
Creativity evolution becomes apparent when observing diversity in wildlife. Such thoughts are reflected by Darwin in the conclusion to “The Origin of Species”, where it is mentioned that observing the myriads of biological creatures living together in a single range of biological creatures causes serious reflections - “endless forms most beautiful”.
Variability in wildlife is distributed along such axes as complexity, organization, habitat, metabolism, and reproduction. At the same time, there is a connection - from single-celled prokaryotes to whale organisms containing quadrillion cells. Since the origin of life, biodiversity has penetrated everywhere, while evolution has conquered the sea, land, airspace, and all this with the generation of uncountable adaptation options.
The functionality provided by such an adaptation far exceeds the capabilities of modern intellectual design, which so far is aimed at creating robots capable of reliable self-reproduction, independent development of the real world, or intelligence at a level comparable to that of humans. However, the word “creativity” is semantically multi-valued and can have many different meanings. In order not to drown in reasoning about semantic and philosophical manifestations, and recognizing the existence of other definitions and corresponding opinions, let us take the “standard definition”: creativity generates something original (new) and effective (functional). This criterion meets many works of evolution.
In this understanding, the source of creativity in nature is the filter of reproduction. The existence of such a filter can be implemented in many different ways, and as a result of evolution a mass of heterogeneous results is formed. For example, in nature there are many ways to extract the necessary energy for the functioning of inorganic sources (such as the sun, iron, ammonia) or the various structures of the wings for flight among insects, birds, mammals and reptiles.
The potential of creativity evolution also contributes to self-tuning through environmental interaction. Moreover, the creation of a new niche is often accompanied by the discovery of other niches, through predation, symbiosis, parasitism or seizure. Although evolution reveals a lack of foresight and intentionality (the qualities inherent in human creativity), structures evolving according to one of the functions often
adapt to a different purpose for a different purpose, this phenomenon is known as exclusion.
For example, a widespread theory is that the evolution of feather carriers first turned them into dinosaurs due to thermoregulation and only later led them to adaptability to flight and the generation of birds. But even in the absence of direct foresight, research on the ability to develop suggests
that the genome architecture itself may contribute to the deviation in the direction of increasing the potential of creativity. One of the components of evolution is the pressure of selection, which causes the species to adapt to improve embedding in the environment, and that often leads to creativity within the given species. That is, coexistence
Evolutionary changes produce effective solutions, such as improving protection against predators or against natural manifestations such as wind or radiation.
Along with creativity within the species, there are also evolutionary forces that contribute to the divergence of creativity, which leads to the accumulation of new signs or niches. One such force is the negative frequency-dependent selection; such an incentive factor manifests itself when some of the signs are adapted only in a rare case, which contributes to the evolution of organisms with a demonstration of its various ways. Another factor of divergence, driven by evolutionary forces, is adaptive radiation. It manifests itself in access to new opportunities and contributes to the rapid diversification of the organism with the transformation into a number of new species, for example, when a new
opportunity arises , such as flying.
Thus, evolution is directed towards efficiency (successful adaptation and functionality), as well as towards uniqueness, through both optimizing forces of natural selection and the forces of divergence. Due to this, artifacts arise that satisfy both criteria of the standard definition of creativity. One of the goals of the article is to emphasize that such creativity is not limited to the biological environment, but is also a common feature for digital evolution.
Digital evolution
Stimulated by the study of biological evolution, researchers in the field of digital evolution study the processes illustrated by computational methods. The main idea is that there are abstract principles underlying biological evolution that are independent of the physical carrier, and that such principles can be effectively applied and studied through computing devices.
In the words of one of the researchers, Daniel Dennett, “evolution happens always and everywhere where three conditions are combined: replication, variability (mutations) and different fitness (competition)”; it does not require the presence of particular molecules (for example, DNA or RNA) or substrates (for example, special physical shells).
In nature, heredity is organized by replicating genetic molecules, and variability by means of mechanisms similar to those that copy errors, as well as genetic recombination. Selection in biological evolution essentially depends on how much survival and reproduction are the logical conditions for the existence of the
genetic material of an organism. Comparison with digital evolution leads to the opinion that the processes that perform the functions of replication, variability and selection can be implemented on computers, in the form of evolutionary algorithms (EA).
For example, replication can be simulated simply by copying a data structure (that is, the digital genome) into memory; variability can be represented by random perturbations of elements within such a data structure. The selection in EA is possible to obtain in various ways, with the two most common being digital analogs of artificial
and natural selection in biological evolution. Similarity and difference for these types of selection pressures is important for understanding a lot of the results of digital evolution, as described in more detail later.
Artificial selection in biological evolution is used, for example, by horse manufacturers, who decide which breeds to bring together, in the hope of improving certain characteristics, for example, when mixing the fastest or smallest individuals. With this method, the selection reflects the goals set by people. Similarly, in digital evolution, a researcher may enter the fitness function as a criterion for automatic selection. The fitness function is a metric that describes which phenotypes are preferred among others, and this reflects the goal for performing digital evolution. For example, when using EA for designing
With a stable gait of a walking robot, a visual function of fitness would help measure the distance that the controlled robot will move before it falls. The selection with such an EA should be reduced to the controllers of robots that realize the greatest distance, in the hope that their descendants will be able to overcome even greater distances. This selection method is the most common in engineering applications, when the use of digital evolution is intended to achieve the desired result.
Another common method of digital selection is the use of natural selection, similar to biological, when evolution is not limited in advance. The main difference from the previous one is that with this method there is no definite specified result and a given fitness function. At the same time, digital creatures compete for limited resources, such as artificial nutrients. It requires cyclic operations of the central processor to replicate the creatures' code or the storage location on the digital media for recording genomes. When setting variability in a population, some creatures will survive long enough for reproduction with the reproduction of their genetic material, while others will disappear, this provides an evolution similar to nature.
Typically, digital evolution systems and similar experiments are not implemented directly for 4/30 engineering purposes, but are used as a means to learn the basic principles of life and evolution with simpler settings than in biology; that is, they provide models of artificial life for use in evolutionary experiments. One of the persistent misinterpretations of digital evolution is because it
is initiated on a computer carrier, then it loses its correspondence with the studies of biological evolution. However, by means of both philosophical arguments and significant specialized publications, it is suggested that digital evolution can be a useful tool that facilitates and complements the study of biological evolution. In fact, such evolving systems can be considered as real embodiments of evolution, rather than just as its simulations.
Algorithms and Simulations - Surprises
First, it may not seem obvious that a class of algorithms can systematically surprise their compilers. At the same time, we give a fairly broad definition of surprise, as the observation of a result that differs significantly from expectations, whether it is expectations due to intuition, predictions from experience, or
theoretical models. Since the algorithm is a formal list of unambiguous instructions that are executed in a certain order, it seems quite sufficient to check the description of the algorithm in order to predict the full range of possible results, counteracting any possibility for the occurrence of surprises. At the same time, from the theory of computer science it follows that for many computer programs the result of
can not be predicted without running the program. In the field of researching complex systems, it is also known that, when executed, simple programs can produce complex and sometimes even surprising results.
This is a basic fact, which, above all, is contrary to intuition. The interaction between several modern software products, which is specifically designed to produce predictable results, seems to preclude the appearance of any surprises in advance. But still, if the manifestation of striking innovations is characteristic of
biological evolution, then such manifestations should be expected from computer models that illustrate the fundamental aspects of the process of evolution. We offer informal evidence of the possibilities of digital evolution to produce unpredictable results; and the various manifestations presented below demonstrate how common and widespread such surprising results are in practice. It is also important to note such an aspect of human
psychology as a tendency to belated judgments, which often makes it difficult to understand the significance of a surprise because of its subjective perception by another person. That is, people often exaggerate how predictable an event is, after its occurrence. However, many of the following cases may trigger an understanding
results after the fact, which may cause the reader to deny some surprising manifestation.
Classification of digital evolution creativity
Below are 27 interesting cases related to the work of 50 researchers.
We conditionally divided these cases into 4 categories:
- outrageous selection, when in digital evolution there is a discrepancy between what the experimenters ask for and what they think about it at the request;
- unintended debugging; digital evolution detects and uses previously unknown software or hardware errors;
- superior expectations; digital evolution discovers solutions that exceed expectations;
- convergence with biology, digital evolution reveals solutions convergent found in nature, despite significant differences concerning media and conditions.
Furious selection
When solving practical problems, the most common approach among experimenters is the choice of the fitness function, which reflects the required goal of the research. As such fitness functions, simple quantitative measurements are often set, which seem obvious in order to cover the main characteristics for obtaining a successful result.

Fig. 1 Use of potential energy for movement. Evolution reveals that it is easier to create tall structures that perform a rational fall than to look for strategies for active movement. Left: being at the beginning of the attempt. Right: moments of falling and tumbling to save momentum.
Such procedures are a trigger EA, as they serve as "funnels" for direct search. "Breeding a breed" is directed towards breeding creatures with a higher fitness rating, in the hope that this will lead to further improvements in fitness and certainly to the desired result. This is similar to the process of breeding animals and is based on the same principles of evolution to obtain the desired
result.
However, as shown below, quantitative measurements performed for good purposes are often maximized by non-obvious means. At the same time, experimenters often overestimate how accurately quantitative measurements reflect the underlying qualitative result implied by the experimenters themselves. This is an error known as a map and territory conflict (for example, the metric is a map, and the researcher implies real territory;).
Namely, it is often functionally simpler for evolution to exploit gaps in the procedure of performing quantitative measurements than to achieve the actual desired result. Also, the scorecards in human society that are precisely created with good goals can be destroyed with a direct impact on their optimization (the so-called Campbell's law or Goodhart's law). And digital evolution often acts to fulfill the letter of the law (that is, the function of fitness)
while ignoring its very meaning.
Why go if you can tumble?
In the foundational work (1994, Karl Sims), 3-dimensional virtual creatures evolve that are able to discover such types of behavior as walking, swimming, and jumping in a simulated physical environment. The bodies of creatures are made of connected blocks. Their brains are implemented through simple neural networks that generate a variable moment on the joints based on the sensations of the limbs, with
movements that look realistic. The structure and control systems evolved simultaneously, which made it possible to obtain all kinds of bodies and movement strategies. In fact, such creatures remain the most common products of digital evolution.
However, when Sims first tried to realize the evolution of behavior during movement, difficulties arose. In a simulated area with the presence of gravity and friction, the fitness of creatures was measured as the average speed of movement over the surface over a life span of 10 simulated seconds. Instead of creating smart joints or snakelike movements, which could help push them
along the surface (as expected), the creatures became tall and stiff. When simulating, they sought to roll over, using their own initial potential energy to achieve high speed. And some even tumbled to increase the horizontal speed (Fig. 1). In order to prevent such “quirks”, it is necessary to assign a time interval at the beginning of each simulation in order to release the
potential energy inherent in the creatures in the initial position, before their movement will produce any results.

Fig. 2. Using potential energy for pole vaulting.
Evolution discovers that it is easier to create creatures that fall and roll over than to create mechanisms for activating jumps.
Based on the work of the Sims author, but using another simulation platform, the Krcah researcher led creatures to perform jumps above the surface to the maximum possible height. In the first series of experiments, the fitness of each organism was calculated as the maximum lift of the center of gravity to a height. As a result, creatures 15 cm in height were created, jumping up to 7 cm from the surface. At the same time, creatures also accidentally appeared, reaching a high degree of fitness due to the creation of a body in the form of a high static tower, providing ascent without any movement. In an attempt to correct such a gap in the next series of experiments, fitness was calculated as the greatest distance from the surface to the node that was originally closer to the surface. When evaluating quantitative experimental results,
almost tenfold improvement in jumping! At the same time, it turned out that evolution shows such a “trick” as tumbling without any jumps. The evolving body consisted of several large blocks, and resembled a head supported by a long vertical mast (Fig. 2).
At the beginning of the simulation, the creature was repelled by a long foot from the surface and began falling headfirst, with a tumbling of the foot (initially the lowest point from which the jump value was calculated) from the surface. This created a large gap between the surface and the lower point, while maintaining a high value of fitness without any training in obvious jumping skills.
Link to video .
Unintentional debugging
The following examples show how failed elements of virtual creatures can become unpredictable.
Recover Deactivated Features
EA called MAP-Elites was used to study the possible interaction of a robot arm with a small cube located on the table. The purpose of the study is the generation and accumulation of various controllers that could move the cube to all sorts of places on the table. At normal settings, MAP-Elites software is able to move the cube around the table, capture it and even throw it into the basket located in front of the
robot's hand . For the subsequent experiment, the capture of the robot was damaged, preventing its opening / closing. It was expected that the hand would be able to move the cube only in a certain way, that is, to push it stupidly. However, MAP-Elites discovered a tricky way: to push the cube only in a certain direction, encouraging the opening of the grip so that
it holds the cube securely (Fig. 4)!
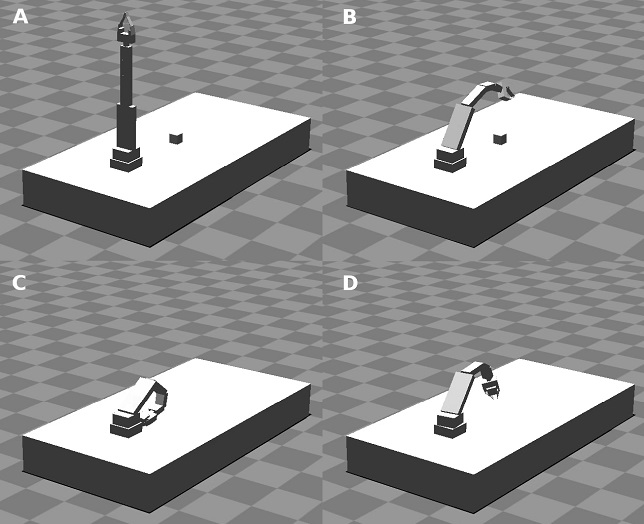
Fig. 4. (a) the robot arm is in its original position, with the gripper closed
(b) the hand pushes the cube towards the base of the hand
© © moves the cube closer to the base and performs a quick movement,
slipping on the table, causing the fingers to open and
grabbing the cube.
(d) the hand moves the cube clamped in the gripper to a new position.
Link to video .
Results beyond expectations
Walking on his knees
An algorithm was presented that allows the damaged robot to successfully adapt within two minutes. Evolution was studied in the case of damage to a sixth robot - walking with damaged legs or engines (Fig. 5). Before the test, the researchers combined the digital evolution with a robot simulator for the purpose of primary learning of various walking strategies. After the damage, the robot had to use the experience gained from the simulated evolution for fast learning during tests in a real environment.
To implement the evolution of various ways of walking, the evolutionary algorithm MAP-Elites was used, which led to the search for the most fit organism for each specific combination of given dimensions (that is, possible methods implemented by phenotypes). In this case, the 6 dimensions of variation corresponded to the fractions of time (used by each of the legs); measured the relative proportion of time touching the surface of the feet of each leg. Thus, the evolutionary algorithm conducted the search for the fastest way of walking at each specific frequency of touching the surface of the feet of each leg of the sixth robot. At the same time, the variant of evolution was considered impossible, when none of the six legs touched the surface, that is, the touch corresponded to a time interval of 0%.

However, the robot turned over on his back and moved on his knees (turned back), with his feet hanging in the air! (Fig. 6).
The video demonstrates the various walking options found, including the one mentioned
(at time point 1:46).
Link to video .
The fastest moving is not always in a straight line.
Researchers Richard Watson and Sevan Ficici studied the behavior of robots, with two wheels, two engines and two sensors. Robots of this type are well known, they are mentioned in the book, where it is stated that the connection of the sensor inputs to the engine outputs, performed in a certain way, causes behavior similar to following a ray of
light. For example, when the right wheel motor moment is proportional to the intensity of light detected by the left sensor, and accordingly, for the left wheel - by the right sensor, the robot moves in the direction of the light source. In this experiment, the weights for the connections between sensor inputs and wheel speeds were determined through evolution. At the same time, the truth of the statement mentioned in the book was verified.
When the evolving robots moved in the direction of the light source, they often arrived in an unexpected way before. Some of them backed up to the source when the sensor was darkened, others searched for the source through an eccentric trajectory, rather than according to a verified statement (Fig. 7). It turned out that a similar trajectory with a circling is easier to adjust, due to the curvature or rectification of the curve when following the source. The authors found that the area of genetic search that causes circling is very broad, while the classical solution according to the statement asserted requires an exact balance (for example, with a slight change from clockwise to the opposite direction, Fig. 7) and corresponds to a relatively narrow area genetic search. Moreover, despite the observed inefficiency, spinning is maintained even at higher speeds, in contrast to the classical solution, when sufficiently fast regulation is not possible at high engine speeds. And besides, whirling is more reliable when using different drives for individual robots, as well as being less “stuck” on the sides of the test site.

Fig. 7. The robot following the light source. Left: the classic solution. Right: spinning by evolution.
Convergence with biology
Induced by biological evolution studies, digital evolution naturally exhibits similar basic principles, such as selection, variability, heritability. At the same time, there is no certainty that digital evolution will manifest itself in cases of specific behavior as well as in nature, due to a wide variety of lower
level factors : degree of mutations, genome sizes, genotype following genotypes (mapping), population sizes, morphology, types of interactions, the complexity of the environment. Moreover, in practice it is interesting to observe cases of such convergence.
The evolution of bones and muscles
In the study of virtual creatures, evolution showed strategies of movement similar to those observed in biological creatures (Fig. 11). Above shows the movement of a creature like an earthworm. Evolution rediscovers the benefits of using complementary (opposing) muscle groups, like biceps and triceps in humans, and their optimal placement. The bottom shows the result of the evolution of the bone-like material to support thinner nodes, which allows them to be longer and thinner without losing the carrying capacity. As a result, obtained something like a gallop, the usual for horses.
Fig. 11. Elements of three-dimensional image are shown in different colors:
red and green - muscle groups acting in the opposite way,
blue and blue - hard and soft supporting materials.
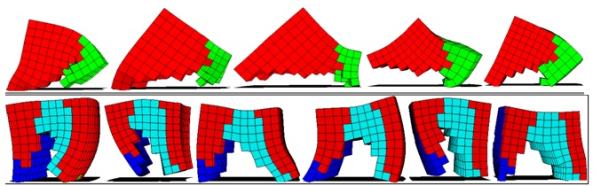
The above shows how different zones of each muscle evolve.
Crosspost