Computer vision: how AI is watching us
- Transfer
Recently, we talked about how we are analyzed in cinemas using computer vision technology: emotions, gestures, and that’s all. Today we are publishing a conversation with our colleague from Microsoft Research. He is creating the very same vision. Under the cat, details about the development of technology, a little about the GDPR, as well as about the applications Join now!

From a technical point of view, computer vision experts "create algorithms and systems for automatically analyzing images and extracting information from the visible world." From the layman’s point of view, they create machines that they can see. This is exactly what the main research officer and head of the research department, Dr. Gang Hua, and the team of computer vision experts are doing. For devices such as personal robots, unmanned vehicles and drones, which we encounter more and more often in everyday life, vision is very important.
Today, Dr. Hua will tell us how recent advances in the field of AI and machine learning have helped improve image recognition technology and “understanding” video, and also contributed to the development of art. He will also explain the essence of the distributed ensemble approach to active learning, in which people and machines work together in the lab to create computer vision systems that can see and recognize an open world.

Gan Hua, Principal Researcher and Head of Research and Development. Photo courtesy of Maryatt Photography.
If we look back ten or fifteen years ago, we will see that there was more diversity in the computer vision community. To consider the problem from different angles and find its solution, various methods of machine learning and knowledge from various fields, such as physics and optics, were used. We stress the importance of diversity in all areas of activity, so I think the scientific community will benefit if we have more different points of view.
We introduce you to advanced technology research and the scientists behind it.
From a technical point of view, computer vision experts "create algorithms and systems for automatically analyzing images and extracting information from the visible world." From the layman’s point of view, they create machines that they can see. This is what the main research officer and head of the research department, Dr. Gan Hua, and a team of computer vision experts are doing. For devices such as personal robots, unmanned vehicles and drones, which we encounter more and more often in everyday life, vision is very important.
Today, Dr. Hua will tell us how recent advances in the field of AI and machine learning have helped improve image recognition technology and “understanding” video, and also contributed to the development of art. He will also explain the essence of the distributed ensemble approach to active learning, in which people and machines work together in the lab to create computer vision systems that can see and recognize an open world. This and much more is in the new release of the Microsoft Research podcast.
You are the chief scientist and head of research at MSR (Microsoft Research), and your specialty is computer vision.
Yes.
If in general terms, why does a computer vision specialist get up in the morning? What is its main goal?
Computer vision is a relatively young area of research. In short, we are trying to create such machines that will be able to see the world and perceive it just like a person. Speaking more technical language, information that enters the computer in the form of simple images and video, can be represented as a sequence of numbers. We want to extract from these numbers some structures that describe the world, some semantic information. For example, I can say that some part of the image corresponds to a cat. And the other part corresponds to the car, I mean the interpretation of this kind. Here it is, the goal of computer vision. It seems simple to people, but in order to teach computers this, we had to do a lot of work over the past 10 years. However, computer vision as an area of research for 50 years.
Yes. 5 years ago you said the following: I paraphrase: “Why, after 30 years of research, are we still working on the problem of facial recognition?” Tell us how you answered this question then and what has changed during that time.
If you answer from the perspective of five years ago, I would say that in the 30 years that have passed since the start of research in the field of computer vision and facial recognition, we have achieved a lot. But for the most part we are talking about a controlled environment, where when capturing faces, you can adjust the lighting, camera, scenery and the like. Five years ago, when we began to work more in natural conditions, in an uncontrollable environment, it turned out that there is a huge gap in the accuracy of recognition. However, over the past five years, our community has made great progress through the use of more advanced in-depth training methods. Even in the field of face recognition in natural conditions, we have made progress and really came to the point where it became possible to use these technologies for various commercial purposes.
It turns out that deep learning has really allowed us to achieve great success in the fields of computer vision and image recognition over the past few years.
Right.
When we started talking about the difference in conditions in fully controlled and unpredictable environments, I remembered several scientists, guests of the podcast, who noted that computers fail when the data are not enough ... for example, the sequence “dog, dog, dog, dog with three legs "- the computer begins to doubt whether the latter is also a dog?
Yes.
After all the truth? So, what exactly is inaccessible earlier, deep learning methods allow you to do today in the field of recognition?
This is a great question. From a research perspective, deep learning offers several possibilities. First, it is possible to conduct comprehensive training in order to determine the correct representation of the semantic image. For example, back to the dog. Suppose we look at different photos of dogs, for example, images of 64 × 64 pixels, where each pixel can take about two hundred and fifty different values. If you think about it, this is a huge number of combinations. But if we speak of a dog as a pattern, where the pixels correlate with each other, then the number of combinations corresponding to the “dog” will be much less.
With the help of complex methods of deep learning, you can teach the system to determine the correct numerical representation of a “dog”. Due to the depth of the structures, we can create really complex models that can master a large amount of data for training. Thus, if my training data covers all possible variants and representations of a template, then in the end I will be able to recognize it in a wider context, because I have considered almost all possible combinations. This is the first.
Another opportunity for deep learning is a kind of compositional behavior. There is a structure layer and a presentation layer, therefore, when information or an image gets into deep networks and the extraction of low-level primitive images begins, the model can gradually collect semantic structures of higher and higher complexity from these primitive images. In-depth learning algorithms identify smaller patterns that correspond to larger patterns and put them together to form the final pattern. Therefore, it is a very powerful tool, especially for tasks of visual recognition.
So, this means that the main topic of the CVPR conference is the recognition of patterns by computer vision.
Yeah, right.
And pattern recognition is what technology really aspires to.

Yes of course. In fact, the goal of computer vision is to grasp the meaning in pixels. Speaking from a technical point of view, the computer needs to understand what the image is, and we get a certain numerical or symbolic result from it. For example, a numerical result may be a three-dimensional cloud of points, which describes the structure of space or the shape of an object. It can also be associated with some semantic labels, such as "dog" or "cat", as I said earlier.
Clear. So let's talk a little about tags. An interesting and important feature of the machine learning process is the fact that the computer needs to provide both pixels and tags.
Yes of course.
You talked about three things that are most interesting to you in the context of computer vision. Videos, faces, as well as art and multimedia. Let's talk about each of them separately, and let's start with your current research, with what you call “understanding” the video.
Yes. The expression "video understanding" speaks for itself. We use video instead of images as input. Here it is important not only to recognize the pixels, but also to consider how they move. For computer vision, image recognition is a spatial problem. In the case of video, it becomes a space-time, because the third, temporary, dimension appears. And if you look at many real-world tasks related to streaming video, be it indoor surveillance cameras or road cameras on the highway, then the point is that the object moves as part of a constant flow of personnel. And we need to extract information from this stream.
These cameras create a huge amount of video. Security cameras that shoot around the clock in supermarkets and the like. What benefits for people can you get from these records?
My team is working on one incubation project, under which we are creating a fundamental technology. In this project we are trying to analyze traffic on the roads. In cities, a huge number of road cameras have been installed, but most of the video they recorded is wasted. However, these cameras can be helpful. Let's look at one example: you want to control traffic lights more efficiently. Usually, the change of red and green signals is determined by the established schedule. However, if I saw that in one direction much less machines are moving than in others, then in order to optimize the movement, I could keep the green color on in overloaded directions longer. This is just one of the uses.
Please implement this idea!
We will try!
Which of us did not stand on the red signal of the traffic light, although almost no one passed to the green in another direction?
That's it!
Just now, you ask yourself: why do I have to wait?
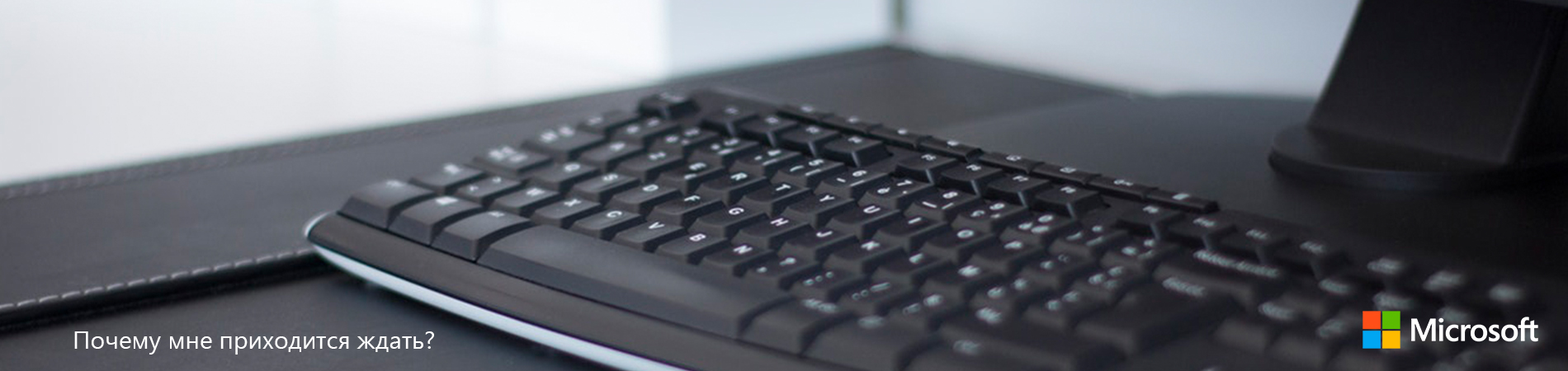
I agree. This technology can also be applied in other cases, for example, when we have accumulated large archives of video. Suppose citizens asked for additional bike lanes. We could use video footage, analyze traffic data, and then decide whether to make a bike lane in this place. By implementing this technology, we could significantly influence traffic flows and help cities make such decisions.
I think this is a great idea, because in most cases we make such decisions based on our own ideas, and not on data, looking at which we could say: "Hey, and you know, here the bike lane would have by the way. And here it will only complicate the movement. ”
Exactly. Sometimes other sensors are used for this. They hire a company that installs special equipment on the roads. But it is economically inefficient. But the road cameras are already installed and just hang around. Video streams are already available. Right? So why not take advantage of this?
Agree. This is a great example of how machine learning and “understanding” video can be applied.
Exactly.
So, another important area of application is face recognition. We again return to the question “Why are we still working on the problem of facial recognition?”.
Exactly.
By the way, such technologies in some cases can be applied in a very interesting way. Tell us what is happening in the field of facial recognition. Who does this and what's new?
Looking back, the face recognition technology was studied by Microsoft when I was still working at Live Labs Research. Then we created the first face recognition library that could be used by various product development teams. For the first time, this technology began to be used in the Xbox. Then the developers tried to use facial recognition to automatically log into the system. I think it was the first time. Over time, the center for the study of facial recognition shifted to Microsoft Research Asia, where we still have a group of researchers with whom I work.
We are constantly trying to expand the boundaries of the possible. Now we work together with technical services that help us collect more data. Based on this data, we train more advanced models. Recently, we have focused on the direction of research, which we call "the synthesis of individuals with preservation of recognition." The community of in-depth training experts has also achieved great success. They use deep networks to train generative models that can simulate the distribution of images so that data can be extracted from it, that is, they can actually synthesize an image. So you can create deep networks that create images.
But we want to go one step further. We want to synthesize faces. At the same time, we want to preserve the recognition of these persons. Our algorithms should not just create an arbitrary set of faces without any semantic meaning. Suppose we want to recreate the face of Brad Pitt. You need to create a face that really looks like him. If you need to recreate the face of a person I know, then the result must be accurate.
So you want to preserve the recognition of the face you are trying to recreate?
Right.
By the way, I wonder if this technology will work for a long time, as a person ages, or will you have to constantly update the database with people?
This is a very good question. We are currently conducting research to solve this problem. At the current level of technology, it is still necessary to update the base from time to time. Especially if the face has changed a lot. For example, if a plastic surgery was performed, the modern system will not be able to produce the correct result.
Wait, it's not you.
Yes, absolutely not like. This issue can be approached from several sides. Human faces do not really change very much between the ages of 17–18 and about 50. But what happens immediately after birth? The faces of children vary greatly because bones grow and the shape of the face and skin change. But as soon as a person grows up and enters the stage of maturity, changes begin to occur very slowly. Now we are conducting research in which we develop models of the aging process. They will help create an improved face recognition system with age. In fact, it is a very useful technology that can be applied in law enforcement, for example, in order to recognize children who were abducted many years ago, who ...
They look quite different.
Yes, it looks different. If clever face recognition algorithms could look at the original photo ...
And say, how would they look at 14 years old if they were stolen much earlier, or something like that?
Yes yes exactly.
This is a great use. Let's talk about another area that you are actively exploring - multimedia and art. Tell us how science intersects with art, and especially about your work in the field of deep transfer of artistic style.
Good. Take a look at people's needs. First of all, we need food, water and sleep, right? After the basic needs are satisfied, the person manifests a strong desire for art ...
And the desire to create.
And create art objects. Within this research, we want to connect computer vision with artistic objects of multimedia and art. We can use computer vision to deliver artistic enjoyment to people. Within the framework of a separate research project on which we have been working for the last two years, we have created a sequence of algorithms with the help of which you can create an image in any artistic style if samples of this style are provided. For example, we can create an image in the style of Van Gogh.
Van Gogh?
Yes, or any other artist ...
Renoir or Monet ... or Picasso.
Yes, any of them. Anyone you can remember ...
I wonder. Using pixels?
Yes, using pixels. This is also created by deep networks using some of the deep learning technologies that we have developed.
It seems that this study requires knowledge from a variety of areas. Where do you find professionals who are capable of ...
I would say that in a sense, our goal is to ... You know, works of art are not always accessible to all. Some of the artwork is really very expensive. With the help of such digital technologies, we are trying to make such works accessible to ordinary people.
Democratize them.
Yes, democratize art, as you say.
It is impressive.
Our algorithm allows you to create a clear numerical model of each style. And we can even mix them if we want to create new styles. This is reminiscent of the creation of an artistic space where we can explore intermediate options and see how the techniques change as we move from one artist to another. And we can even take a deeper look and try to understand what exactly determines the style of an artist.
My particular interest is that, on the one hand, we are talking about working with numbers: computer science, algorithms, mathematics. On the other hand, it’s about art — a much more metaphysical category. And yet you have combined them, and this shows that the brain of a scientist can have an artistic side.
Exactly. I think that the most important tool we use that helped us put everything together is statistics.
Interesting.
All sorts of algorithms for machine learning actually only collect statistics on pixels.
We have already talked about the technical side of the question, but let us go a little further into technical details ... In some of your recently published works - which our students can find on the MSR website, as well as on your site - you talked about a new distributed ensemble approach to active learning. Tell us what are the peculiarities of this approach and what advantages does it give?
Great question. When we talk about active learning, we mean a process in which a certain warden is involved. In traditional active learning, we have ... a learning machine. This machine can intelligently select some sample data, and then ask the warden to provide additional data. The learning machine selects the samples and asks the person-warden to provide, for example, a label for the image. The process of ensemble machine learning is much more complicated. We are trying to implement active learning in a crowdsourced environment.
Consider, for example, the Amazon Mechanical Turk platform. People send their data to it and ask other users to assign a tag to this data. However, if you are not careful and do not follow the process, the result can be very nasty. You will not be able to use the resulting tags. To solve such problems, we are trying to achieve two goals. First, we want to intelligently distribute the data in order to make tagging as cost effective as possible. Secondly, we need to evaluate the quality of the work done, so that later the user can send his data only to good employees.
This is how our model works. We have a distributed ensemble model. Each crowdsourced employee is associated with one of the learning machines. We are also trying to carry out statistical checks on all models in order to immediately receive a qualitative assessment for each of the employees. In this case, we will be able to use the model not only to select samples, but also to send data for the placement of tags to the best employees. This way you can quickly get a good model.
But this brings us to the problem associated with the need for human-machine interaction within the model. We need a certain system of regulation of such interactions. In addition to what you have already described, how does the joint work of people and machines help to solve quality control problems?
I have been thinking about this problem for a long time, mainly in the context of robotics. Any intellectual system, if it is not in a completely closed world, can work autonomously. But as soon as it enters the open world (like modern intellectual systems based on machine learning), we notice that the system does not always manage to cope with the problems that arise, because something often happens that it has never encountered before.
And there are variables that you haven't thought of.
Exactly. I was thinking about how to involve people in the process so that they could help the car when necessary, and at the same time form some mechanism that would help it cope with similar situations in the future. I will give a very specific example. When I worked at the Stevens Institute of Technology, I was involved in a project from NIH, which we called co-robots.
What kind of robots?
Co-robots. They were essentially robotic wheelchairs. The idea was to control the stroller with head movements. A special camera was installed on the head, which made it possible to track its position. And if a person kept at least the mobility of the neck, then he could manage the wheelchair himself. However, we did not need the user to do this all the time. Suppose a person is at home. We wanted the wheelchair robot for the most part to independently move the user, only after receiving an indication of where to go.
For example, if a user wants to go to another room, then the robot must get there independently. But what if he faces a situation that he doesn’t know how to handle? For example, do not know how to get around an obstacle? In such a situation, the robot must ask the person to take control. Then the user will start to control the robot and resolve the difficult situation for the machine. Perhaps the next time when this robot encounters similar difficulties, it will be able to cope with them himself.
What did you do before working at Microsoft Research and how did you find yourself here?
I work at Microsoft a second time. I already mentioned that I worked here in 2006-2009 in a lab called Live Labs. This was my first time. Then I created the first facial recognition library. After that, I wanted to explore the outside world, so to speak. I worked at Nokia Research, IBM Research and eventually stayed at the Stevens Institute of Technology as a teacher ...
It's in New Jersey, right?
Yes, it is in New Jersey, on the east coast. I returned to Microsoft Research in 2015 and began working in a laboratory in Beijing. My family stayed here, so in 2017 I transferred back.
So after Beijing, you ended up in Redmond. How did this happen?
My family always lived in Seattle. Microsoft Research Lab in Beijing is a great place. I really liked it there. One of the unique advantages of the laboratory is an incredibly dynamic internship program. Several hundred interns work in the laboratory all year round. And they all work closely with their mentors. Very dynamic environment. I experimented a little away from home, but my family lived in Seattle, so when the Intelligent Group created a computer vision team here, I joined it.
And you live in Seattle again.
Yes.
I ask this question to all the scientists who come to the podcast, and I will ask it to you too. Is there something in your work that we need to worry about? I mean, is there something that keeps you awake at night?
I would say that privacy is the biggest problem, especially when we talk about computer vision. There are hundreds of millions of cameras around the world. They are everywhere: in public places and in buildings. If we take into account the speed with which technology develops, then the assumptions that it is possible to track a person, no matter where he is, are no longer something of science fiction. Everything has two sides. Yes, on the one hand, computer vision can help us, for example, to cope with crime. But for ordinary citizens, it presents huge risks associated with confidentiality.
What can I do ... I ask this question because it makes people think: So, I have this powerful technology, how can it harm? So what can you do, what laws to adopt to solve this problem?
Microsoft takes the general data protection policy (GDPR) very seriously. And I think it’s great, because this mechanism is designed to ensure that everything we produce complies with certain rules. On the other hand, it is necessary to find a balance between the practicality of the technology and security or privacy. When you use any online service, all your actions leave a mark. This is a way to make your life easier in the future. If you want convenience, sometimes you have to disclose some of the information. But no one wants to provide all the information about themselves, is not it? This is a complex question, and the answer is ambiguous, it goes beyond black and white. You need to carefully monitor what is happening. We must receive only the information that is necessary for better customer service,
Yes, today it is important to get permission from the user. He should be able to say: “I am not against this. But I don't like that. ”
Yeah, right.
Gan, at the end of our conversation, share with us your idea of what is waiting for a new generation of specialists in the field of computer vision in the near future. Resolving what big problems can lead to an incredible breakthrough? What is to work in the next 10 years?
This is an excellent and very deep question. There really are big problems that we have to solve. Right now, computer vision experts rely heavily on statistical machine learning methods. We can train models of recognition that can achieve great success. But this process is still largely based on visual signs. We need to better understand the recognition process and the fundamental principles of computer vision, such as three-dimensional geometry.
There are other moments, especially when it comes to "understanding" the video. This is a complex problem, for the solution of which it is necessary to work with spatial-temporal categories and take into account concepts of cognition, such as a causal effect. If something happened, then what really caused it? Machine learning methods mainly work with correlation between data. Correlation and causality are two completely different concepts. I think it's worth working on it. There are some other fundamental problems, such as learning from small data and language, that need to be addressed in the future. Pay attention to how people are trained. We learn from experience, but there is another way. We learn through language. We learn in the process of conversation. For example, today I have already learned a lot of new things from you ...
And I from you.
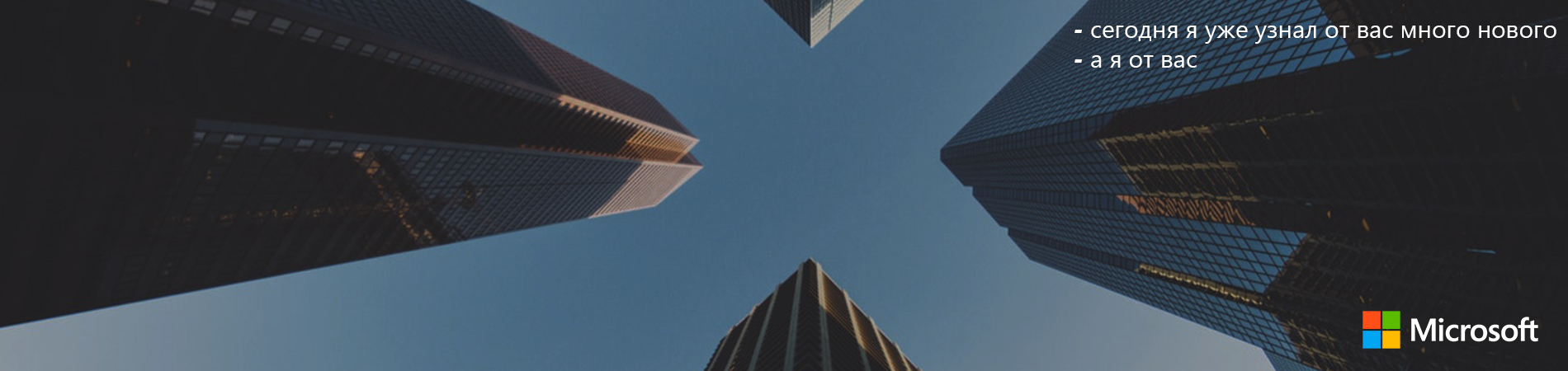
That's it. This is a very compact stream of information. Now we pay the most attention to deep learning. But if you go back 10-15 years ago, you can see that there was more diversity in the computer vision community. To consider the problem from different angles and find its solution, various methods of machine learning and knowledge from various fields, such as physics and optics, were used. We stress the importance of diversity in all areas of activity, so I think the scientific community will benefit if we have more different points of view.
This is very good advice. The community of researchers welcomes a new generation of scientists, people who think broadly and in different directions, who will be able to prepare the ground for the next big breakthrough.
Yes exactly!
To learn more about Dr. Gan Hua, as well as amazing advances in computer vision, visit our website: Microsoft.com/research

From a technical point of view, computer vision experts "create algorithms and systems for automatically analyzing images and extracting information from the visible world." From the layman’s point of view, they create machines that they can see. This is exactly what the main research officer and head of the research department, Dr. Gang Hua, and the team of computer vision experts are doing. For devices such as personal robots, unmanned vehicles and drones, which we encounter more and more often in everyday life, vision is very important.
Today, Dr. Hua will tell us how recent advances in the field of AI and machine learning have helped improve image recognition technology and “understanding” video, and also contributed to the development of art. He will also explain the essence of the distributed ensemble approach to active learning, in which people and machines work together in the lab to create computer vision systems that can see and recognize an open world.

Gan Hua, Principal Researcher and Head of Research and Development. Photo courtesy of Maryatt Photography.
Interview
If we look back ten or fifteen years ago, we will see that there was more diversity in the computer vision community. To consider the problem from different angles and find its solution, various methods of machine learning and knowledge from various fields, such as physics and optics, were used. We stress the importance of diversity in all areas of activity, so I think the scientific community will benefit if we have more different points of view.
We introduce you to advanced technology research and the scientists behind it.
From a technical point of view, computer vision experts "create algorithms and systems for automatically analyzing images and extracting information from the visible world." From the layman’s point of view, they create machines that they can see. This is what the main research officer and head of the research department, Dr. Gan Hua, and a team of computer vision experts are doing. For devices such as personal robots, unmanned vehicles and drones, which we encounter more and more often in everyday life, vision is very important.
Today, Dr. Hua will tell us how recent advances in the field of AI and machine learning have helped improve image recognition technology and “understanding” video, and also contributed to the development of art. He will also explain the essence of the distributed ensemble approach to active learning, in which people and machines work together in the lab to create computer vision systems that can see and recognize an open world. This and much more is in the new release of the Microsoft Research podcast.
You are the chief scientist and head of research at MSR (Microsoft Research), and your specialty is computer vision.
Yes.
If in general terms, why does a computer vision specialist get up in the morning? What is its main goal?
Computer vision is a relatively young area of research. In short, we are trying to create such machines that will be able to see the world and perceive it just like a person. Speaking more technical language, information that enters the computer in the form of simple images and video, can be represented as a sequence of numbers. We want to extract from these numbers some structures that describe the world, some semantic information. For example, I can say that some part of the image corresponds to a cat. And the other part corresponds to the car, I mean the interpretation of this kind. Here it is, the goal of computer vision. It seems simple to people, but in order to teach computers this, we had to do a lot of work over the past 10 years. However, computer vision as an area of research for 50 years.
Yes. 5 years ago you said the following: I paraphrase: “Why, after 30 years of research, are we still working on the problem of facial recognition?” Tell us how you answered this question then and what has changed during that time.
If you answer from the perspective of five years ago, I would say that in the 30 years that have passed since the start of research in the field of computer vision and facial recognition, we have achieved a lot. But for the most part we are talking about a controlled environment, where when capturing faces, you can adjust the lighting, camera, scenery and the like. Five years ago, when we began to work more in natural conditions, in an uncontrollable environment, it turned out that there is a huge gap in the accuracy of recognition. However, over the past five years, our community has made great progress through the use of more advanced in-depth training methods. Even in the field of face recognition in natural conditions, we have made progress and really came to the point where it became possible to use these technologies for various commercial purposes.
It turns out that deep learning has really allowed us to achieve great success in the fields of computer vision and image recognition over the past few years.
Right.
When we started talking about the difference in conditions in fully controlled and unpredictable environments, I remembered several scientists, guests of the podcast, who noted that computers fail when the data are not enough ... for example, the sequence “dog, dog, dog, dog with three legs "- the computer begins to doubt whether the latter is also a dog?
Yes.
After all the truth? So, what exactly is inaccessible earlier, deep learning methods allow you to do today in the field of recognition?
This is a great question. From a research perspective, deep learning offers several possibilities. First, it is possible to conduct comprehensive training in order to determine the correct representation of the semantic image. For example, back to the dog. Suppose we look at different photos of dogs, for example, images of 64 × 64 pixels, where each pixel can take about two hundred and fifty different values. If you think about it, this is a huge number of combinations. But if we speak of a dog as a pattern, where the pixels correlate with each other, then the number of combinations corresponding to the “dog” will be much less.
With the help of complex methods of deep learning, you can teach the system to determine the correct numerical representation of a “dog”. Due to the depth of the structures, we can create really complex models that can master a large amount of data for training. Thus, if my training data covers all possible variants and representations of a template, then in the end I will be able to recognize it in a wider context, because I have considered almost all possible combinations. This is the first.
Another opportunity for deep learning is a kind of compositional behavior. There is a structure layer and a presentation layer, therefore, when information or an image gets into deep networks and the extraction of low-level primitive images begins, the model can gradually collect semantic structures of higher and higher complexity from these primitive images. In-depth learning algorithms identify smaller patterns that correspond to larger patterns and put them together to form the final pattern. Therefore, it is a very powerful tool, especially for tasks of visual recognition.
So, this means that the main topic of the CVPR conference is the recognition of patterns by computer vision.
Yeah, right.
And pattern recognition is what technology really aspires to.

Yes of course. In fact, the goal of computer vision is to grasp the meaning in pixels. Speaking from a technical point of view, the computer needs to understand what the image is, and we get a certain numerical or symbolic result from it. For example, a numerical result may be a three-dimensional cloud of points, which describes the structure of space or the shape of an object. It can also be associated with some semantic labels, such as "dog" or "cat", as I said earlier.
Clear. So let's talk a little about tags. An interesting and important feature of the machine learning process is the fact that the computer needs to provide both pixels and tags.
Yes of course.
You talked about three things that are most interesting to you in the context of computer vision. Videos, faces, as well as art and multimedia. Let's talk about each of them separately, and let's start with your current research, with what you call “understanding” the video.
Yes. The expression "video understanding" speaks for itself. We use video instead of images as input. Here it is important not only to recognize the pixels, but also to consider how they move. For computer vision, image recognition is a spatial problem. In the case of video, it becomes a space-time, because the third, temporary, dimension appears. And if you look at many real-world tasks related to streaming video, be it indoor surveillance cameras or road cameras on the highway, then the point is that the object moves as part of a constant flow of personnel. And we need to extract information from this stream.
These cameras create a huge amount of video. Security cameras that shoot around the clock in supermarkets and the like. What benefits for people can you get from these records?
My team is working on one incubation project, under which we are creating a fundamental technology. In this project we are trying to analyze traffic on the roads. In cities, a huge number of road cameras have been installed, but most of the video they recorded is wasted. However, these cameras can be helpful. Let's look at one example: you want to control traffic lights more efficiently. Usually, the change of red and green signals is determined by the established schedule. However, if I saw that in one direction much less machines are moving than in others, then in order to optimize the movement, I could keep the green color on in overloaded directions longer. This is just one of the uses.
Please implement this idea!
We will try!
Which of us did not stand on the red signal of the traffic light, although almost no one passed to the green in another direction?
That's it!
Just now, you ask yourself: why do I have to wait?
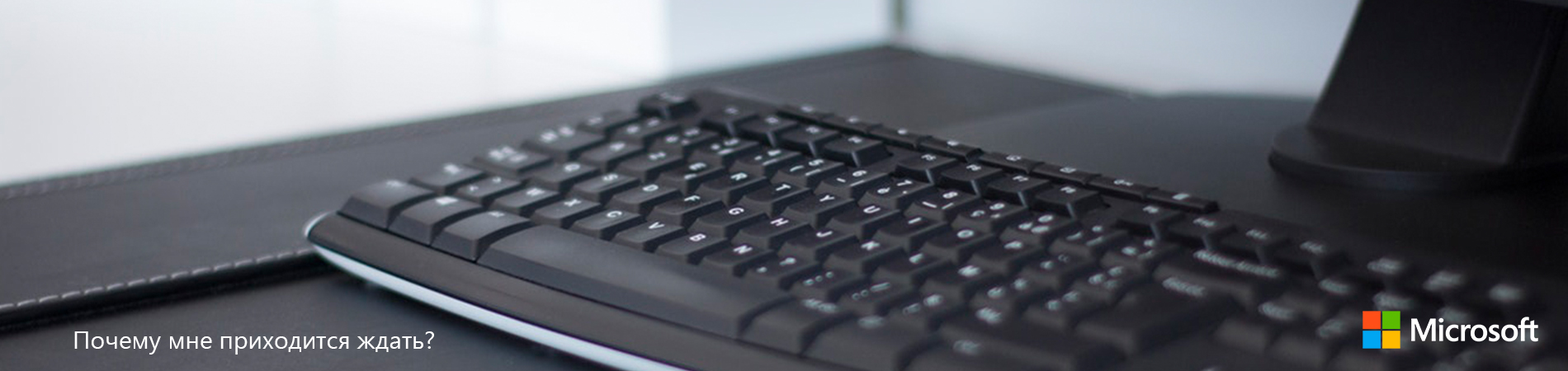
I agree. This technology can also be applied in other cases, for example, when we have accumulated large archives of video. Suppose citizens asked for additional bike lanes. We could use video footage, analyze traffic data, and then decide whether to make a bike lane in this place. By implementing this technology, we could significantly influence traffic flows and help cities make such decisions.
I think this is a great idea, because in most cases we make such decisions based on our own ideas, and not on data, looking at which we could say: "Hey, and you know, here the bike lane would have by the way. And here it will only complicate the movement. ”
Exactly. Sometimes other sensors are used for this. They hire a company that installs special equipment on the roads. But it is economically inefficient. But the road cameras are already installed and just hang around. Video streams are already available. Right? So why not take advantage of this?
Agree. This is a great example of how machine learning and “understanding” video can be applied.
Exactly.
So, another important area of application is face recognition. We again return to the question “Why are we still working on the problem of facial recognition?”.
Exactly.
By the way, such technologies in some cases can be applied in a very interesting way. Tell us what is happening in the field of facial recognition. Who does this and what's new?
Looking back, the face recognition technology was studied by Microsoft when I was still working at Live Labs Research. Then we created the first face recognition library that could be used by various product development teams. For the first time, this technology began to be used in the Xbox. Then the developers tried to use facial recognition to automatically log into the system. I think it was the first time. Over time, the center for the study of facial recognition shifted to Microsoft Research Asia, where we still have a group of researchers with whom I work.
We are constantly trying to expand the boundaries of the possible. Now we work together with technical services that help us collect more data. Based on this data, we train more advanced models. Recently, we have focused on the direction of research, which we call "the synthesis of individuals with preservation of recognition." The community of in-depth training experts has also achieved great success. They use deep networks to train generative models that can simulate the distribution of images so that data can be extracted from it, that is, they can actually synthesize an image. So you can create deep networks that create images.
But we want to go one step further. We want to synthesize faces. At the same time, we want to preserve the recognition of these persons. Our algorithms should not just create an arbitrary set of faces without any semantic meaning. Suppose we want to recreate the face of Brad Pitt. You need to create a face that really looks like him. If you need to recreate the face of a person I know, then the result must be accurate.
So you want to preserve the recognition of the face you are trying to recreate?
Right.
By the way, I wonder if this technology will work for a long time, as a person ages, or will you have to constantly update the database with people?
This is a very good question. We are currently conducting research to solve this problem. At the current level of technology, it is still necessary to update the base from time to time. Especially if the face has changed a lot. For example, if a plastic surgery was performed, the modern system will not be able to produce the correct result.
Wait, it's not you.
Yes, absolutely not like. This issue can be approached from several sides. Human faces do not really change very much between the ages of 17–18 and about 50. But what happens immediately after birth? The faces of children vary greatly because bones grow and the shape of the face and skin change. But as soon as a person grows up and enters the stage of maturity, changes begin to occur very slowly. Now we are conducting research in which we develop models of the aging process. They will help create an improved face recognition system with age. In fact, it is a very useful technology that can be applied in law enforcement, for example, in order to recognize children who were abducted many years ago, who ...
They look quite different.
Yes, it looks different. If clever face recognition algorithms could look at the original photo ...
And say, how would they look at 14 years old if they were stolen much earlier, or something like that?
Yes yes exactly.
This is a great use. Let's talk about another area that you are actively exploring - multimedia and art. Tell us how science intersects with art, and especially about your work in the field of deep transfer of artistic style.
Good. Take a look at people's needs. First of all, we need food, water and sleep, right? After the basic needs are satisfied, the person manifests a strong desire for art ...
And the desire to create.
And create art objects. Within this research, we want to connect computer vision with artistic objects of multimedia and art. We can use computer vision to deliver artistic enjoyment to people. Within the framework of a separate research project on which we have been working for the last two years, we have created a sequence of algorithms with the help of which you can create an image in any artistic style if samples of this style are provided. For example, we can create an image in the style of Van Gogh.
Van Gogh?
Yes, or any other artist ...
Renoir or Monet ... or Picasso.
Yes, any of them. Anyone you can remember ...
I wonder. Using pixels?
Yes, using pixels. This is also created by deep networks using some of the deep learning technologies that we have developed.
It seems that this study requires knowledge from a variety of areas. Where do you find professionals who are capable of ...
I would say that in a sense, our goal is to ... You know, works of art are not always accessible to all. Some of the artwork is really very expensive. With the help of such digital technologies, we are trying to make such works accessible to ordinary people.
Democratize them.
Yes, democratize art, as you say.
It is impressive.
Our algorithm allows you to create a clear numerical model of each style. And we can even mix them if we want to create new styles. This is reminiscent of the creation of an artistic space where we can explore intermediate options and see how the techniques change as we move from one artist to another. And we can even take a deeper look and try to understand what exactly determines the style of an artist.
My particular interest is that, on the one hand, we are talking about working with numbers: computer science, algorithms, mathematics. On the other hand, it’s about art — a much more metaphysical category. And yet you have combined them, and this shows that the brain of a scientist can have an artistic side.
Exactly. I think that the most important tool we use that helped us put everything together is statistics.
Interesting.
All sorts of algorithms for machine learning actually only collect statistics on pixels.
We have already talked about the technical side of the question, but let us go a little further into technical details ... In some of your recently published works - which our students can find on the MSR website, as well as on your site - you talked about a new distributed ensemble approach to active learning. Tell us what are the peculiarities of this approach and what advantages does it give?
Great question. When we talk about active learning, we mean a process in which a certain warden is involved. In traditional active learning, we have ... a learning machine. This machine can intelligently select some sample data, and then ask the warden to provide additional data. The learning machine selects the samples and asks the person-warden to provide, for example, a label for the image. The process of ensemble machine learning is much more complicated. We are trying to implement active learning in a crowdsourced environment.
Consider, for example, the Amazon Mechanical Turk platform. People send their data to it and ask other users to assign a tag to this data. However, if you are not careful and do not follow the process, the result can be very nasty. You will not be able to use the resulting tags. To solve such problems, we are trying to achieve two goals. First, we want to intelligently distribute the data in order to make tagging as cost effective as possible. Secondly, we need to evaluate the quality of the work done, so that later the user can send his data only to good employees.
This is how our model works. We have a distributed ensemble model. Each crowdsourced employee is associated with one of the learning machines. We are also trying to carry out statistical checks on all models in order to immediately receive a qualitative assessment for each of the employees. In this case, we will be able to use the model not only to select samples, but also to send data for the placement of tags to the best employees. This way you can quickly get a good model.
But this brings us to the problem associated with the need for human-machine interaction within the model. We need a certain system of regulation of such interactions. In addition to what you have already described, how does the joint work of people and machines help to solve quality control problems?
I have been thinking about this problem for a long time, mainly in the context of robotics. Any intellectual system, if it is not in a completely closed world, can work autonomously. But as soon as it enters the open world (like modern intellectual systems based on machine learning), we notice that the system does not always manage to cope with the problems that arise, because something often happens that it has never encountered before.
And there are variables that you haven't thought of.
Exactly. I was thinking about how to involve people in the process so that they could help the car when necessary, and at the same time form some mechanism that would help it cope with similar situations in the future. I will give a very specific example. When I worked at the Stevens Institute of Technology, I was involved in a project from NIH, which we called co-robots.
What kind of robots?
Co-robots. They were essentially robotic wheelchairs. The idea was to control the stroller with head movements. A special camera was installed on the head, which made it possible to track its position. And if a person kept at least the mobility of the neck, then he could manage the wheelchair himself. However, we did not need the user to do this all the time. Suppose a person is at home. We wanted the wheelchair robot for the most part to independently move the user, only after receiving an indication of where to go.
For example, if a user wants to go to another room, then the robot must get there independently. But what if he faces a situation that he doesn’t know how to handle? For example, do not know how to get around an obstacle? In such a situation, the robot must ask the person to take control. Then the user will start to control the robot and resolve the difficult situation for the machine. Perhaps the next time when this robot encounters similar difficulties, it will be able to cope with them himself.
What did you do before working at Microsoft Research and how did you find yourself here?
I work at Microsoft a second time. I already mentioned that I worked here in 2006-2009 in a lab called Live Labs. This was my first time. Then I created the first facial recognition library. After that, I wanted to explore the outside world, so to speak. I worked at Nokia Research, IBM Research and eventually stayed at the Stevens Institute of Technology as a teacher ...
It's in New Jersey, right?
Yes, it is in New Jersey, on the east coast. I returned to Microsoft Research in 2015 and began working in a laboratory in Beijing. My family stayed here, so in 2017 I transferred back.
So after Beijing, you ended up in Redmond. How did this happen?
My family always lived in Seattle. Microsoft Research Lab in Beijing is a great place. I really liked it there. One of the unique advantages of the laboratory is an incredibly dynamic internship program. Several hundred interns work in the laboratory all year round. And they all work closely with their mentors. Very dynamic environment. I experimented a little away from home, but my family lived in Seattle, so when the Intelligent Group created a computer vision team here, I joined it.
And you live in Seattle again.
Yes.
I ask this question to all the scientists who come to the podcast, and I will ask it to you too. Is there something in your work that we need to worry about? I mean, is there something that keeps you awake at night?
I would say that privacy is the biggest problem, especially when we talk about computer vision. There are hundreds of millions of cameras around the world. They are everywhere: in public places and in buildings. If we take into account the speed with which technology develops, then the assumptions that it is possible to track a person, no matter where he is, are no longer something of science fiction. Everything has two sides. Yes, on the one hand, computer vision can help us, for example, to cope with crime. But for ordinary citizens, it presents huge risks associated with confidentiality.
What can I do ... I ask this question because it makes people think: So, I have this powerful technology, how can it harm? So what can you do, what laws to adopt to solve this problem?
Microsoft takes the general data protection policy (GDPR) very seriously. And I think it’s great, because this mechanism is designed to ensure that everything we produce complies with certain rules. On the other hand, it is necessary to find a balance between the practicality of the technology and security or privacy. When you use any online service, all your actions leave a mark. This is a way to make your life easier in the future. If you want convenience, sometimes you have to disclose some of the information. But no one wants to provide all the information about themselves, is not it? This is a complex question, and the answer is ambiguous, it goes beyond black and white. You need to carefully monitor what is happening. We must receive only the information that is necessary for better customer service,
Yes, today it is important to get permission from the user. He should be able to say: “I am not against this. But I don't like that. ”
Yeah, right.
Gan, at the end of our conversation, share with us your idea of what is waiting for a new generation of specialists in the field of computer vision in the near future. Resolving what big problems can lead to an incredible breakthrough? What is to work in the next 10 years?
This is an excellent and very deep question. There really are big problems that we have to solve. Right now, computer vision experts rely heavily on statistical machine learning methods. We can train models of recognition that can achieve great success. But this process is still largely based on visual signs. We need to better understand the recognition process and the fundamental principles of computer vision, such as three-dimensional geometry.
There are other moments, especially when it comes to "understanding" the video. This is a complex problem, for the solution of which it is necessary to work with spatial-temporal categories and take into account concepts of cognition, such as a causal effect. If something happened, then what really caused it? Machine learning methods mainly work with correlation between data. Correlation and causality are two completely different concepts. I think it's worth working on it. There are some other fundamental problems, such as learning from small data and language, that need to be addressed in the future. Pay attention to how people are trained. We learn from experience, but there is another way. We learn through language. We learn in the process of conversation. For example, today I have already learned a lot of new things from you ...
And I from you.
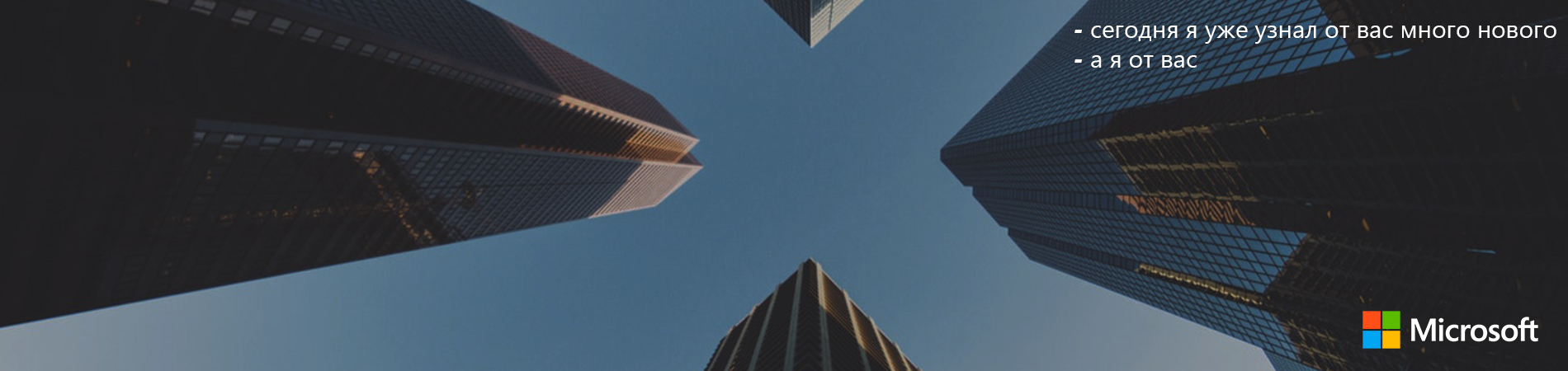
That's it. This is a very compact stream of information. Now we pay the most attention to deep learning. But if you go back 10-15 years ago, you can see that there was more diversity in the computer vision community. To consider the problem from different angles and find its solution, various methods of machine learning and knowledge from various fields, such as physics and optics, were used. We stress the importance of diversity in all areas of activity, so I think the scientific community will benefit if we have more different points of view.
This is very good advice. The community of researchers welcomes a new generation of scientists, people who think broadly and in different directions, who will be able to prepare the ground for the next big breakthrough.
Yes exactly!
To learn more about Dr. Gan Hua, as well as amazing advances in computer vision, visit our website: Microsoft.com/research