Why artificial intelligence will not solve all the problems
- Transfer

Hysteria around the future of artificial intelligence (AI) captured the world. There is no shortage of sensational news about how AI can cure diseases , accelerate innovation, and improve human creativity . If you read the headlines of the media, you can decide that you already live in the future, in which the AI penetrated into all aspects of society.
And although it cannot be denied that the AI has opened a rich set of promising opportunities for us , it also led to the emergence of thinking, which can be described as faith in the omnipotence of AI. According to this philosophy, if there is enough data, machine learning algorithms can solve all the problems of mankind .
But this idea has a big problem. It does not support AI progress, but on the contrary, it puts the value of machine intelligence at risk, neglecting important safety principles and setting people on unrealistic expectations about AI capabilities.
Faith in the omnipotence of AI
In just a few years, the faith of the omnipotence of AI had snuck from the conversations of the technological evangelists of Silicon Valley into the minds of government representatives and lawmakers around the world. The pendulum has swung from the anti-utopian view of AI, which is destroying mankind, to the utopian belief in the coming of our algorithmic savior .
We are already seeing how governments provide support to national AI development programs and compete in the technological and rhetorical arms race in order to gain an advantage in the booming machine learning sector (MO). For example, the British government promised to invest £ 300 million.in AI research to become a leader in this field. Fascinated by the transformative potential of AI, the French president Emmanuel Macron decided to turn France into an international center of AI . The Chinese government is expanding its AI capabilities with a state plan to create a Chinese AI industry in the amount of $ 150 billion by 2030. Faith in the omnipotence of AI is gaining momentum and is not going to give up.

Neural networks - easier said than done
While many political statements extol the transformative effects of the impending " AI revolution, " they usually underestimate the complexity of implementing advanced MO systems in the real world.
One of the most promising varieties of AI technology is neural networks . This form of machine learning is based on an exemplary imitation of the neural structure of the human brain, but on a much smaller scale. Many AI-based products use neural networks to extract patterns and rules from large amounts of data. But many politicians do not understand that simply by adding a neural network to the problem, we will not necessarily immediately get its solution. So, adding a neural network to democracy, we will not make it instantly less discriminated, more honest or personalized.
Challenging data bureaucracy
AI systems need a huge amount of data to work, but in the public sector there is usually no suitable data infrastructure to support advanced MO systems. Most of the data is stored in offline archives. A small number of existing digitized data sources are drowning in bureaucracy. Data is most often spread across different government departments, each of which requires a special permit for access. Among other things, the public sector usually lacks talents equipped with the necessary technical abilities to fully reap the benefits of AI .
For these reasons, sensationalism associated with AIgets a lot of criticism. Stuart Russell, a professor of computer science at Berkeley, has long been preaching a more realistic approach, concentrating on the simplest, everyday applications of AI, instead of a hypothetical world capture by super-intelligent robots. Similarly, a professor of robotics from MIT, Rodney Brooks, writes that "almost all innovations in robotics and AI take much, much more time to actually implement than both specialists in this field and all others imagine."
One of the many problems of implementing MO systems is that AI is extremely susceptible to attacks.. This means that a malicious AI can attack another AI in order to force it to give out incorrect predictions or act in a certain way. Many researchers have warned that it is impossible to immediately roll out AI, without preparing the appropriate safety standards and protective mechanisms . But until now, the topic of AI security has not received due attention.
Machine learning is not magic
If we want to reap the benefits of AI and minimize potential risks, we need to start thinking about how we can meaningfully apply MOs to specific areas of government, business, and society. And this means that we need to start discussing the ethics of AI and the distrust of many people to the MO.
Most importantly, we need to understand the limitations of AI and those moments in which people still have to take control in their hands. Instead of drawing an unrealistic picture of the capabilities of AI, you need to take a step back and separate the real technological capabilities of AI from magic.
For a long time, Facebook countedthat problems such as spreading misinformation and hate speech can be algorithmically recognized and stopped. But under pressure from lawmakers, the company quickly promised to replace its algorithms with an army of 10,000 human reviewers .
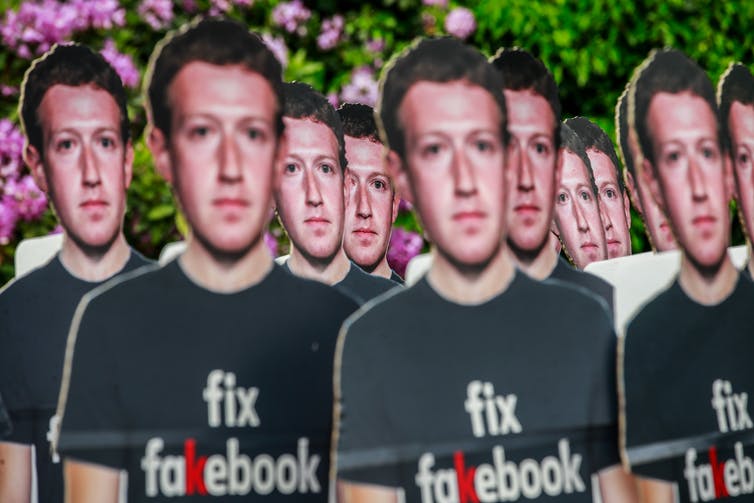
In medicine, they also recognize that AI cannot be considered the solution to all problems. The " IBM Watson for Oncology " program was an AI that was supposed to help doctors fight cancer. And although it was designed to give the best advice, experts find it difficult to trust the machine . As a result, the program was closed in most hospitals, where its trial launches took place.
Similar problems arise in the legislative area whenalgorithms were used in US courts for sentencing. The algorithms calculated the values of risks and gave recommendations on sentences to the judges . But it was found that the system increases structural racial discrimination, after which it was abandoned.
These examples show that there are no AI-based solutions for everything. The use of AI for the sake of AI itself is not always productive or useful. Not every problem is best solved by applying machine intelligence to it. This is the most important lesson for all those who intend to increase investments in government programs for the development of AI: all solutions have their own price, and not everything that can be automated can be automated.