
Algorithm + crowd - this is not enough
- Transfer
In the last decade, two related forces have ruled the online world: Crowd and Algorithm. The collective “users” of the Internet (Crowd) create content, click and vote, while the mathematical equations bring scalability and the ability to search through this huge data array (Algorithm).
Like the moon over the ocean, the interaction of these two forces creates waves of popularity (and oblivion) on the Internet. Information is more accessible, useful and egalitarian than ever.
But over time (at least to me) the weaknesses of the system "algorithm + crowdsourcing" became visible. The next revolution seems inevitable.
Let's start by looking at some examples of crowdsourcing, algorithmic progress:
• Netflix
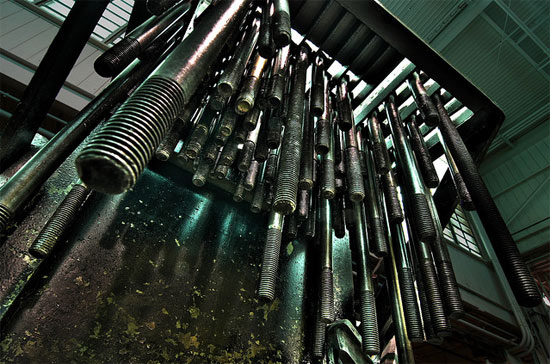
- we look, put ratings and reviews. Netflix recommends (they even crowded their algorithm ).
• Amazon - we select, buy, put ratings and reviews. Amazon analyzes the data until it knows exactly what to recommend (obviously, this is a critical part of their business ).
• Expedia - we fly, rent rooms, sleep and relax. Expedia machines analyze, set prices and calculate how to maximize profits.
• Google - we create content, cite others and share links. Google crawls, sorts, issues and ranks (based mainly on the link graph created by people ).
• Facebook- we are friends, update and like. Facebook is building the perfect system for targeting content and ads.
• Reddit / Digg / StumbleUpon - we offer themes and vote for the content. They accept them and determine how long the topic should hang on the main page and for whom it will be visible.
• Yelp - we have dinner, visit establishments and make purchases, leave feedback. Yelp classifies, ranks and recommends.
We are not completely dependent on these algorithms, because we have the ability to dig deeper, search further and collect more data to make a decision (and often we do). But, as machines make assumptions about what we need, it can be easier to use the default solution than to look for an alternative path.
Compare this with alternative methodologies before the era of the Internet:
• Video rental - a cassette for viewing was helped by choosing the sections “Personnel Recommendations”, “Critics' Choice” and “Award Nominee”.
• Journal of technology (Consumer Reports) - experts analyzed and tested the product from all sides, and then rated it and recommended the best from their point of view.
• Travel agents - travel was booked by professionals who had unique access to databases with information about tickets and hotels.
• Restaurant guide - such guides included restaurant ratings and reviews from anonymous appraisers from dozens of cities.
• Personal recommendations- when there was not a single reference resource, then friends, relatives, colleagues and various service personnel (concierge in the house, telephone inquiry) could offer their options so personal, relevant and valuable that this methodology is often used even today.
Do not get me wrong, I believe that in most cases these predecessors of the modern algorithm + crowdsourcing approach cannot be compared in terms of quality, reliability and usefulness. Their range was often too limited, sometimes distorted by commercial gain and at times simply wrong.
But these predecessors had real advantages - the main thing was that everyone could understand what the recommendation was based on. Compare this to the mystery of our algorithm + crowdsourcing services.
Why is this page displayed first for a specific Google request? Why does one link stay on the Reddit home page for hours, and the other with the same number of votes disappears in a few minutes? Why is Facebook showing me Comcast support job advertisements? Why does Amazon recommend buying whole milk with this homemade tank ?
If we do not understand the reason for such recommendations, can this reduce our confidence in future recommendations of these services?
Fred Wilson recently wrote an interesting story about why we should not invest in something we do not understand:
... the venture capital market sectors are filled with investors seeking profit. And some of them do not understand what they are investing in. A few weeks ago a private investor called me who wanted to invest in one company from our portfolio. He asked several questions about the company, and it became quite clear that the person did not fully understand the essence of its activities. But then he offered his investment. That scares me.
Recently I have been visited by some foreign representatives, many of whom invest billions of dollars on behalf of their bosses. All of them want to get into our funds and in our transactions. When I ask about the reasons, they cannot even formulate a convincing argument about the economic potential of the social network. But they see profit and want it too. That scares me.
I believe that for some people it is equally difficult and stupid to trust the recommendation services whose algorithms they cannot understand. These same people can turn for recommendations to sources that they understand, and the results of which they can infer logically.
My point is not that Google, Netflix, Amazon, Yelp or anyone else is doomed to failure. But I believe that there is a business opportunity for entrepreneurs, websites and companies to add an editorial component to the “algo-crowd” paradigm.
Many startups are already operating in this vein.
• Quora / FormSpring / StackExchange- unlike answers from Google or even Wikipedia, where the order of the results or the source of information is unknown and free of responsibility, modern Q + A systems have given power to the hands of crowds, algorithms and experts .
• Techmeme / Memeorandum / Mediagazer - a crowd of bloggers creates content and quotes each other. The algorithm compiles and sorts information, while curators provide quality and relevance.
• Alltop - A collection of feeds selected by Guy Kawasaki by industry. At the moment, the algorithmic element is missing, but I think it can not last so long.
• Oyster / Raveable- Travel sites are notorious for too many dubious comments and untrustworthy reviews of suspicious quality from commercial partners, financially motivated affiliates. Oyster acts by sending editorial experts to hotels to write their own reviews, and then applying user data and algorithmic ranking / optimization to help readers make the best choice. Raveable combines lightweight editorial supervision with powerful data mining and a sorting algorithm to help you find a hotel.
• Groupon / LivingSocial / Gilt Group- Sites with coupons and offers on the Web have been around for about ten years, but these three (and a bunch of similar ones) have revolutionized the industry and become one of the most famous brands online thanks to social features (crowdsourcing marketing and advertising of offers), algorithmic filtering for personalization / localization of proposals and editorial supervision (so that the deals they offer are really worthy).
• TheSixtyOne / Pandora / Last.fm / Spotify - Music recommendations are getting better, and the latest generation of such services is a mixture of algorithms, crowdsourcing and editorial choice, which gives symphonic delight to many users.
I think we will see even more examples of such an approach in the future, perhaps even on the sites / services that I mentioned at the very beginning (Yelp, Amazon, Netflix, etc.). I am sure that algorithms and crowdsourcing can be made stronger with the addition of the third pillar - confident, supportive editors.
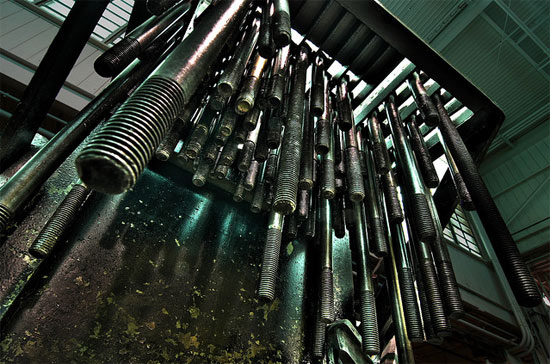
- we look, put ratings and reviews. Netflix recommends (they even crowded their algorithm ).
• Amazon - we select, buy, put ratings and reviews. Amazon analyzes the data until it knows exactly what to recommend (obviously, this is a critical part of their business ).
• Expedia - we fly, rent rooms, sleep and relax. Expedia machines analyze, set prices and calculate how to maximize profits.
• Google - we create content, cite others and share links. Google crawls, sorts, issues and ranks (based mainly on the link graph created by people ).
• Facebook- we are friends, update and like. Facebook is building the perfect system for targeting content and ads.
• Reddit / Digg / StumbleUpon - we offer themes and vote for the content. They accept them and determine how long the topic should hang on the main page and for whom it will be visible.
• Yelp - we have dinner, visit establishments and make purchases, leave feedback. Yelp classifies, ranks and recommends.
We are not completely dependent on these algorithms, because we have the ability to dig deeper, search further and collect more data to make a decision (and often we do). But, as machines make assumptions about what we need, it can be easier to use the default solution than to look for an alternative path.
Compare this with alternative methodologies before the era of the Internet:
• Video rental - a cassette for viewing was helped by choosing the sections “Personnel Recommendations”, “Critics' Choice” and “Award Nominee”.
• Journal of technology (Consumer Reports) - experts analyzed and tested the product from all sides, and then rated it and recommended the best from their point of view.
• Travel agents - travel was booked by professionals who had unique access to databases with information about tickets and hotels.
• Restaurant guide - such guides included restaurant ratings and reviews from anonymous appraisers from dozens of cities.
• Personal recommendations- when there was not a single reference resource, then friends, relatives, colleagues and various service personnel (concierge in the house, telephone inquiry) could offer their options so personal, relevant and valuable that this methodology is often used even today.
Do not get me wrong, I believe that in most cases these predecessors of the modern algorithm + crowdsourcing approach cannot be compared in terms of quality, reliability and usefulness. Their range was often too limited, sometimes distorted by commercial gain and at times simply wrong.
But these predecessors had real advantages - the main thing was that everyone could understand what the recommendation was based on. Compare this to the mystery of our algorithm + crowdsourcing services.
Why is this page displayed first for a specific Google request? Why does one link stay on the Reddit home page for hours, and the other with the same number of votes disappears in a few minutes? Why is Facebook showing me Comcast support job advertisements? Why does Amazon recommend buying whole milk with this homemade tank ?
If we do not understand the reason for such recommendations, can this reduce our confidence in future recommendations of these services?
Fred Wilson recently wrote an interesting story about why we should not invest in something we do not understand:
... the venture capital market sectors are filled with investors seeking profit. And some of them do not understand what they are investing in. A few weeks ago a private investor called me who wanted to invest in one company from our portfolio. He asked several questions about the company, and it became quite clear that the person did not fully understand the essence of its activities. But then he offered his investment. That scares me.
Recently I have been visited by some foreign representatives, many of whom invest billions of dollars on behalf of their bosses. All of them want to get into our funds and in our transactions. When I ask about the reasons, they cannot even formulate a convincing argument about the economic potential of the social network. But they see profit and want it too. That scares me.
I believe that for some people it is equally difficult and stupid to trust the recommendation services whose algorithms they cannot understand. These same people can turn for recommendations to sources that they understand, and the results of which they can infer logically.
My point is not that Google, Netflix, Amazon, Yelp or anyone else is doomed to failure. But I believe that there is a business opportunity for entrepreneurs, websites and companies to add an editorial component to the “algo-crowd” paradigm.
Many startups are already operating in this vein.
• Quora / FormSpring / StackExchange- unlike answers from Google or even Wikipedia, where the order of the results or the source of information is unknown and free of responsibility, modern Q + A systems have given power to the hands of crowds, algorithms and experts .
• Techmeme / Memeorandum / Mediagazer - a crowd of bloggers creates content and quotes each other. The algorithm compiles and sorts information, while curators provide quality and relevance.
• Alltop - A collection of feeds selected by Guy Kawasaki by industry. At the moment, the algorithmic element is missing, but I think it can not last so long.
• Oyster / Raveable- Travel sites are notorious for too many dubious comments and untrustworthy reviews of suspicious quality from commercial partners, financially motivated affiliates. Oyster acts by sending editorial experts to hotels to write their own reviews, and then applying user data and algorithmic ranking / optimization to help readers make the best choice. Raveable combines lightweight editorial supervision with powerful data mining and a sorting algorithm to help you find a hotel.
• Groupon / LivingSocial / Gilt Group- Sites with coupons and offers on the Web have been around for about ten years, but these three (and a bunch of similar ones) have revolutionized the industry and become one of the most famous brands online thanks to social features (crowdsourcing marketing and advertising of offers), algorithmic filtering for personalization / localization of proposals and editorial supervision (so that the deals they offer are really worthy).
• TheSixtyOne / Pandora / Last.fm / Spotify - Music recommendations are getting better, and the latest generation of such services is a mixture of algorithms, crowdsourcing and editorial choice, which gives symphonic delight to many users.
I think we will see even more examples of such an approach in the future, perhaps even on the sites / services that I mentioned at the very beginning (Yelp, Amazon, Netflix, etc.). I am sure that algorithms and crowdsourcing can be made stronger with the addition of the third pillar - confident, supportive editors.