Generation space and opportunity space
- Transfer
In this tutorial, I’ll talk about two terms that I use to describe procedural generators: the generative space and the possibility space . We will define these two terms, and then we will look at interactive examples in order to understand the difference between them. These terms are really useful for describing a procedural generator and for understanding the difference between two generators. Let's get started!
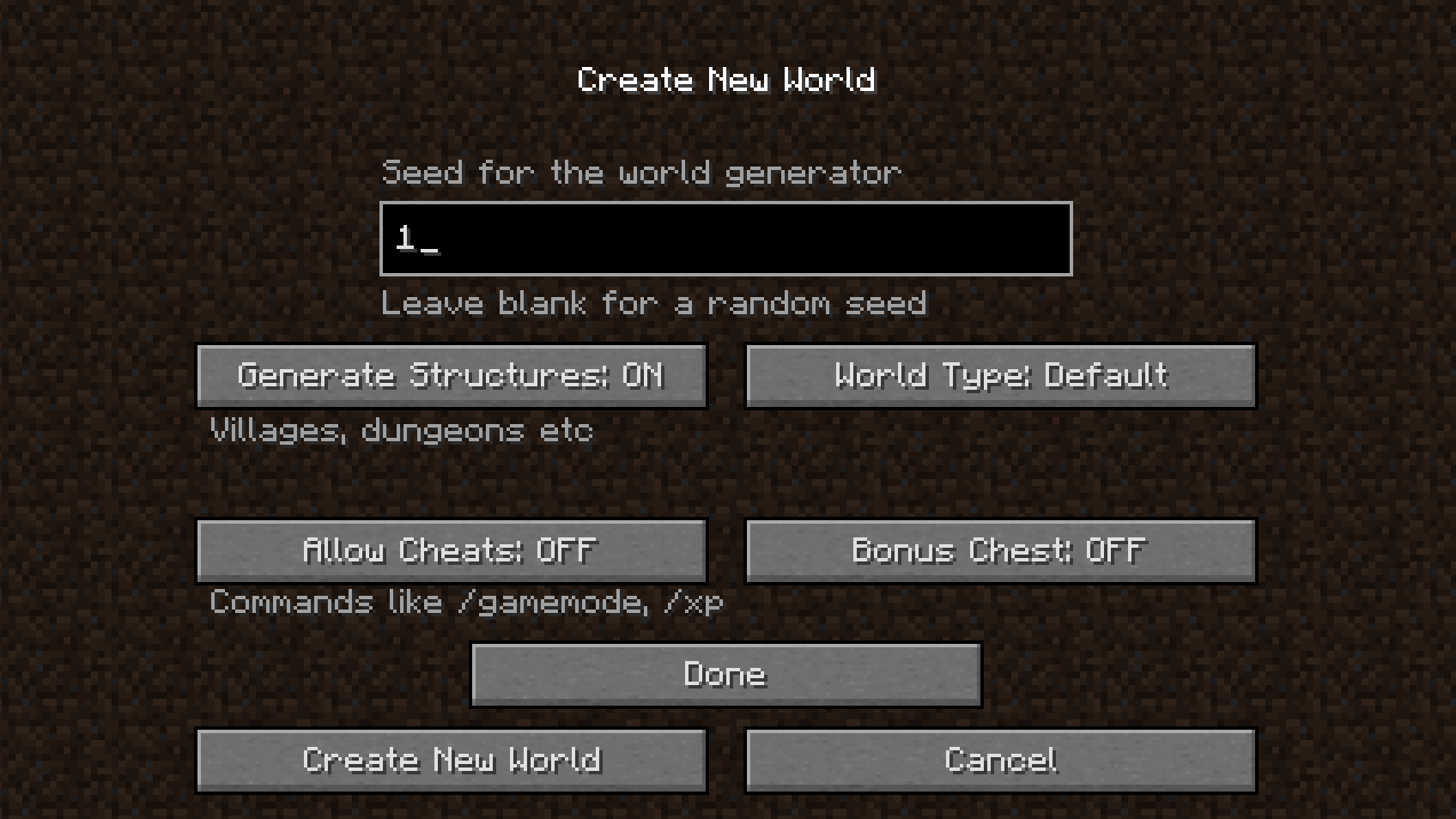
Imagine a huge book featuring a screenshot of every single Minecraft world. Each screenshot is labeled with a random seed , a unique number that can be entered into Minecraft and generate this world. The first page presents the world spawned from seed = 0, the next one - the world from seed = 1, and so on. In general, the Minecraft Worlds Generator contains 2 64 random random values, and this is a huge number: the game can generate 18,446,744,073,709,551,616 worlds. Every time you click on "New World", a world is created based on one of these seed. The number 2 64 is the size of the Minecraft generation space , the set of everything that the game can generate.

Now imagine that the world of Minecraft consists only of ordinary grass, infinitely extending in all directions. Under it there are no caves, no stone, no trees and hills, no animals. Just one layer of grass tiles. Besides the fact that it is very boring, such a world will never be generated in Minecraft (if you do not use modding). We can imagine it, we can describe it, even open Minecraft and create it ourselves manually - but Minecraft cannot generate it.How do we know this? You can prove it in many different ways, but the easiest thing to remember is the Minecraft biomes — areas like the desert or jungle scattered around each world. In our example with the world of grass, they are not there, so we know that it cannot be created with a standard generator.
The world of grass is in what we call the space of possibilities of the worlds of Minecraft - the set of all possible worlds of Minecraft that we can imagine, imagine or describe. But the grass world is not in the generation space.Minecraft, because it cannot be created by a procedural game generator. We can imagine the world of Minecraft with a completely exact copy of Persepolis to scale, or the world in the form of your stone sculpture, sitting and reading this article - all are possible worlds of Minecraft, but they will not be generated by the game.
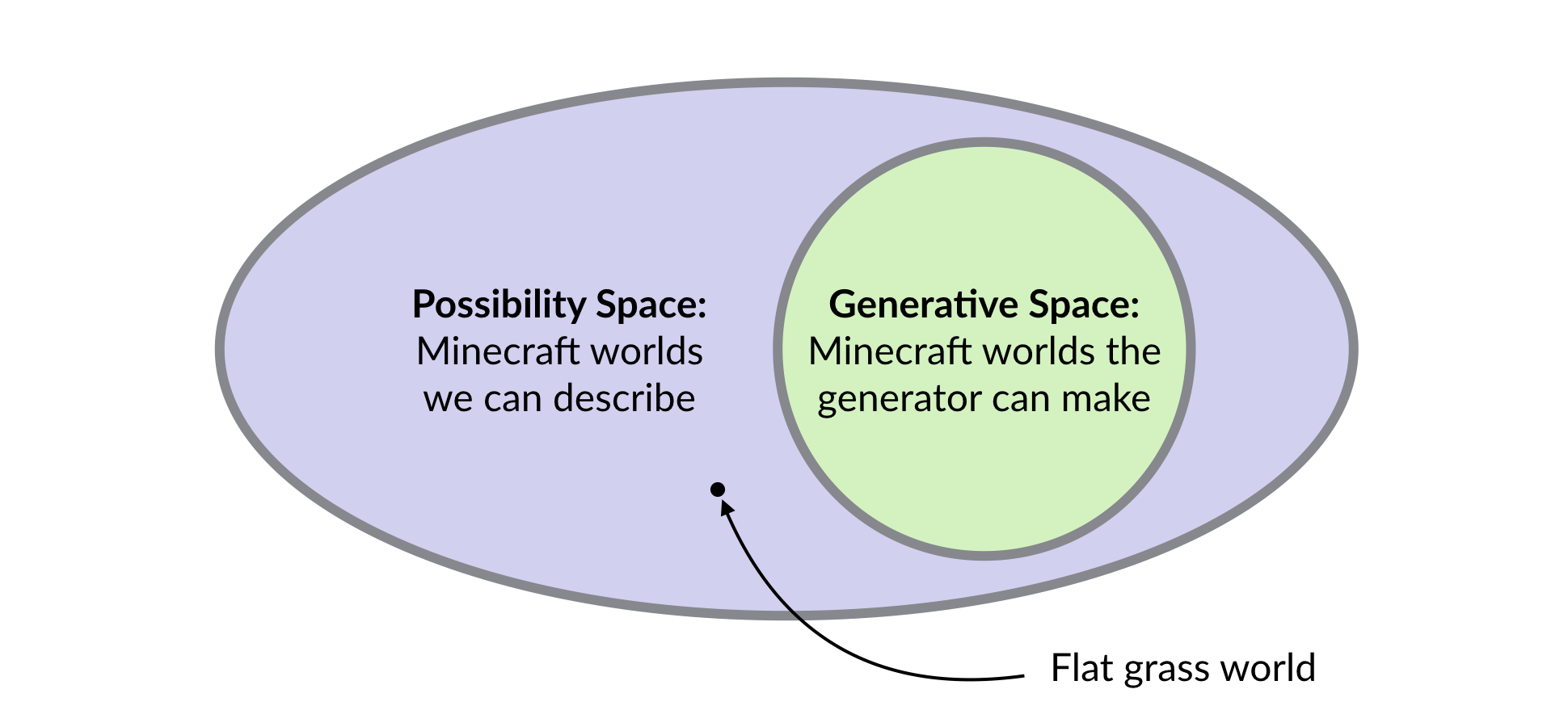
The scheme shown above gives us an idea of how it all looks. Everything that is in the Minecraft generation space should also be in its capability space, because if Minecraft can generate something, then it should be possible to create it in Minecraft! But not all of the space of possibilities is in the space of generation, including our example of the world consisting of grass. But in this scheme the scale is not respected. In fact, the space of Minecraft's capabilities is much, much larger than its generation space.
To understand how big it is, let's calculate the size of a separate Minecraft world. Volume is the width multiplied by the height multiplied by the depth. By default, Minecraft worlds have a height of 256 tiles, and they begin to break up into approximately 30,000,000 tiles in all directions from the starting point, that is, the approximate volume of the world is:
Each of these blocks can be any element: stone, grass, water, air. For our assessment, we will be generous and use only "blocks of the world", which, according to the Minecraft wiki, are 64 types . This means that every block in the world of Minecraft has 64 possible states. The formula for calculating the number of possible worlds is equal to the number of variants of the states of each block raised to the power of the number of blocks:
This number is so large that I could not find a calculator on the Internet that can calculate it.

To go deeper into the spaces of generation and possibilities, we need an example that we can better put in our head. Therefore, in the remainder of the tutorial, we will consider the levels of a 2D platformer for games like Super Mario Bros. or spelunky . We will look at several level generators for 2D platformers. In the original article, they are all interactive, so you can generate your own levels. In the process of their study, we will ask questions about their generation space. All examples are available on our GitHub as an open source sketch written in the Processing .
In the process of studying, I will ask questions in bold, for example:What is the size of the space of opportunities for these levels of platform? Feel free to skip them if you just want to read the article and play with examples. I also sometimes add hints to help in answering questions, for example:
Hint: levels are 40 tiles wide and 10 tiles high, and each tile can be one of three elements: void, ground, or a bonus block.
You can pause at the tooltip and do not scroll down the page if you do not want to read the finished answer. So let's take a look at our first generator.
Our first level generator is very simple - it is completely random. Each card has a start tile from the left edge and an exit tile from the right edge. All other tile cards have a probability of 33% to be empty, 33% to be occupied, and 33% to be a bonus block.

You probably noticed that this is not a very good level generator. In fact, in most of these levels it is even impossible to play, not to mention their interestingness. Generate a few more levels and think about the question: what size does the generation space of such a generator have in comparison with the space of possibilities?
Hint: can you think of a level that is in the space of possibilities, but not in the space of generation? Can you think of a level that this generator cannot create?
The correct answer is that the generation space of this generator is the samewith space of opportunity. Any tile on the map can take on any value, so any level that we can imagine is in the generation space. The most fascinating, the most interesting levels are in this generation space, even the map, on which the bonus blocks say SUPER MARIO IS AWESOME. Unfortunately, the generation space is so large that finding a good level is extremely unlikely. As you can be sure, when you press the generator, most levels are just rubbish.
Our next algorithm is a bit more complicated, and it creates levels that are more like what you can apply in the game. Starting from the empty level, he selects a random point somewhere, and then draws a line or a square of blocks of bonuses or earth. He performs this operation 20 times, creating several shapes, randomly drawn at the level. As before, you can click on the example in the original article to run the algorithm again and generate a new level.
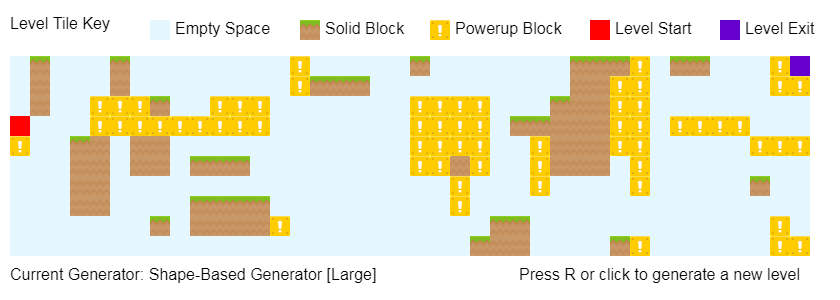
This is starting to resemble the level for the platformer, right? When generating levels, mentally imagine how you play them (be generous if necessary - add the ability to double jump, if necessary, or the ability to jump outside the ceiling of the map).
How many levels need to be generated before you find at least one that you can pass?
Do not strive for absolute accuracy in your mental walkthroughs, just make a rough estimate.
When I do this exercise with an audience, it usually takes about 10 attempts to find a level on average. And how much did you need? Remember this number, and now look at the variation of the same generator shown below. The generator is the same as we have just seen, but now it draws at the level of not 20, but 10 figures. Now count again: how many levels do you need to generate before you find the one you can pass?
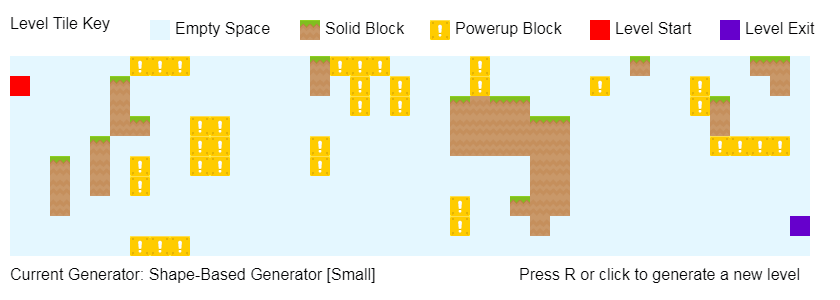
Probably, it took you less time to find a playable level. Let's stop for a minute and think about the differences between these two generators, which are almost identical, except for a small difference in parameters. Which generator has more generation space - a generator with 20 figures, or 10?
Hint: think about the level created by a 10-figure generator - can a generator with 20 figures be able to create it? And then think about the opposite example.
The correct answer is that a 20-figure generator has a larger generation space; in fact, it contains the entire generator generation space with 10 figures. To show this, imagine any level from a 10-figure generator. Our generator with 20 figures can create it by accidentally drawing the same ten figures, and then accidentally drawing them a second time on top of each other. However, no level with twenty individual figures can be drawn with a 10-figure generator.
This means that a generator with 20 figures has more levels, including interesting levels. But it is worth remembering that finding a playable level was much easier with a generator with 10 figures. If you had to choose a generator for your video game, which one would you use?There are no right answers here, but this is a very important decision that often has to be done when working with procedural generators. One generator can generate more variability and has more surprises, the other is more reliable and less annoying to the player.
This algorithm is based on the level generator used in Spelunky; You can read more about it here . The level generator randomly selects the level fragment created by the person (me) and inserts it into the level. He then selects another random fragment of the level and inserts it side by side, and so on, until the level is completed. Here is the generator, the background is darkened, so that the borders of the fragments are more clearly visible:

How long will it take to generate a playable level now? You will find that almost every level is playable. We know exactly what is in each fragment, that is, we can guarantee that at the level there will be interesting objects that can be jumped on and obstacles that need to be avoided. At the same time, it is more dynamic than the static level - we do not know which fragments will appear in it and in what order. The Spelunky algorithm is even richer than this, it takes into account the movement along the vertical, and random variations are added to each fragment. Darius Kazemi created Spelunky’s awesome interactive level generator that can be found here .
Such generators are a good compromise between pleasant surprises and the possibility of control. When a generator makes a selection from a catalog of elements and connects them together (sometimes according to special rules, as in Spelunky), we call it a grammar-based generator . Grammar allows us to decide which building blocks a procedural generator should use, but less strictly as to how they should be put together. But this control and security come at a price. Look at the generator again, and then think about the previous examples. How often will such a level generator surprise us?
Initially, the generator is full of surprises, because we meet each piece for the first time. But if you play more, then quickly you start to notice where one ends and another fragment begins. Even if there are hundreds of combinations of fragments in this generator, after just a few levels you begin to feel as if you saw them all. The simplicity and ease of understanding grammar fragments are great for developers, but can be a disadvantage in terms of fun for players.
As a final example, we will consider not an algorithm, but a very different kind of creative process: the work of people in level design. Click on the generator in the original article, and you will receive one of the first two levels of Super Mario Bros. (more precisely, their very beginning).
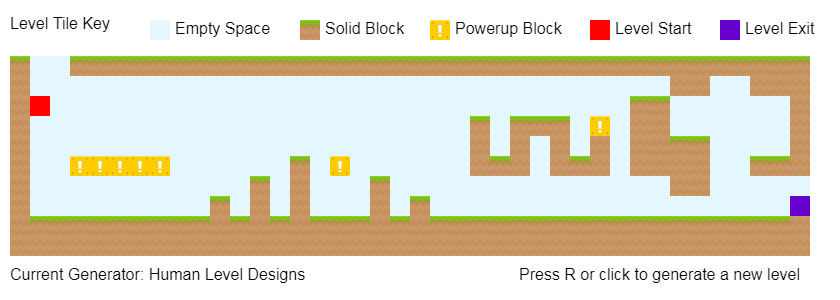
Starting with an absolute chance, we ended up with this, one of the most famous examples of 2D platformer design in history. Of course, this is not a generator, but in a sense, these levels can be perceived as generators, creating only one, high-quality level. Maximum compromise between control and quality. Looking at this level, think about all the generators that we considered today, and think about the question: which of these generators contain in their generation space these famous levels? .
Only the first generator is able to create such levels. Generators of forms could create them if we increased the number of possible drawn shapes, but this would also significantly increase the number of bad or strange levels. The level fragment generator could do this if we gave it a different set of fragments, but would all the other combinations of these fragments be playable?
This shows us how difficult it is to create a procedural generator. We want to think of our generators as normal game content, to imagine how a player passes a certain level and gets pleasure from it. But as generation designers, we need to think about the whole generation space, and not just about a single example of it. How big is the space? How rich is it for surprises? How often does it create something boring or bad? Can we detect and filter these cases, or do we need to find a workaround? These are just some of the issues that need to be considered when creating something for generation.
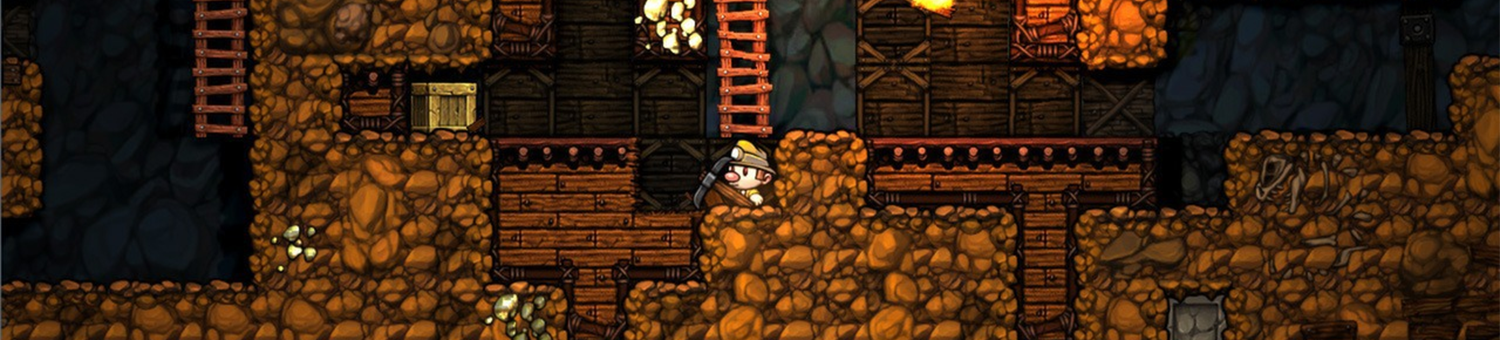
Today we learned about the following concepts:
The generation space of a procedural content generator (for example, the Minecraft world generator) is the set of all the results it can generate. If we change the algorithm or specify a different value for the variable, the generation space will change.
The space of opportunities for a certain type of content (for example, the world of Minecraft) is the set of all examples of such content that we can present or describe. Usually, but not always, it is much larger than the generation space of the procedural generator.
A generator with a large generation space usually has more good content, more unexpected content and more diverse content. But he usually has more junk content, more boring content, and more unusable content.
A generator with less generation space is easier to control, easier to test, and easier to understand. But it can make it more predictable and less surprising.
Finding ways to balance the strengths and weaknesses of these two opposites is the art of creating generation software!
This ends my tutorial! Thanks for reading.
Among the interesting materials on the topic reviewed by us, I recommend the following:
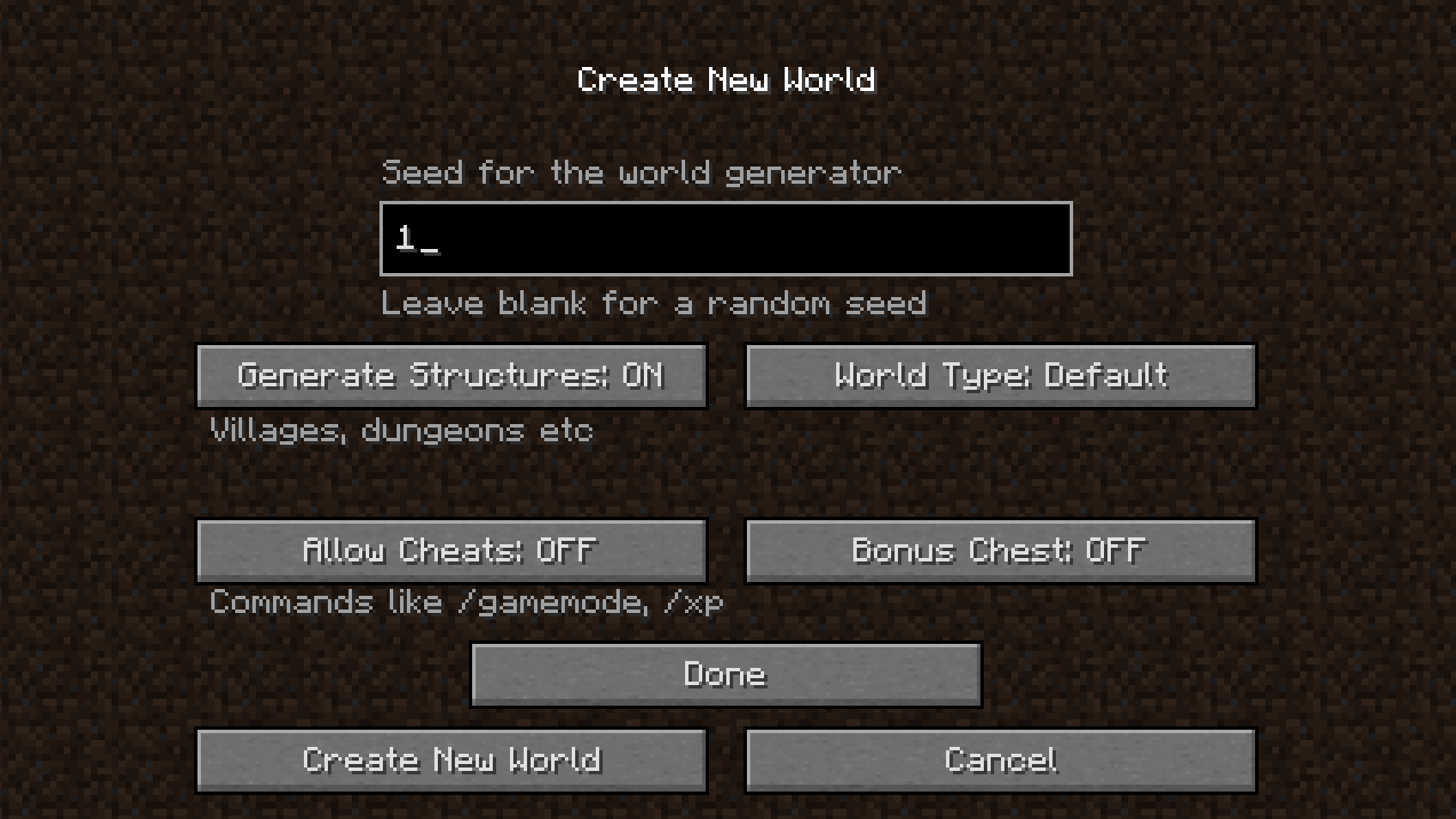
Imagine a huge book featuring a screenshot of every single Minecraft world. Each screenshot is labeled with a random seed , a unique number that can be entered into Minecraft and generate this world. The first page presents the world spawned from seed = 0, the next one - the world from seed = 1, and so on. In general, the Minecraft Worlds Generator contains 2 64 random random values, and this is a huge number: the game can generate 18,446,744,073,709,551,616 worlds. Every time you click on "New World", a world is created based on one of these seed. The number 2 64 is the size of the Minecraft generation space , the set of everything that the game can generate.

Now imagine that the world of Minecraft consists only of ordinary grass, infinitely extending in all directions. Under it there are no caves, no stone, no trees and hills, no animals. Just one layer of grass tiles. Besides the fact that it is very boring, such a world will never be generated in Minecraft (if you do not use modding). We can imagine it, we can describe it, even open Minecraft and create it ourselves manually - but Minecraft cannot generate it.How do we know this? You can prove it in many different ways, but the easiest thing to remember is the Minecraft biomes — areas like the desert or jungle scattered around each world. In our example with the world of grass, they are not there, so we know that it cannot be created with a standard generator.
The world of grass is in what we call the space of possibilities of the worlds of Minecraft - the set of all possible worlds of Minecraft that we can imagine, imagine or describe. But the grass world is not in the generation space.Minecraft, because it cannot be created by a procedural game generator. We can imagine the world of Minecraft with a completely exact copy of Persepolis to scale, or the world in the form of your stone sculpture, sitting and reading this article - all are possible worlds of Minecraft, but they will not be generated by the game.
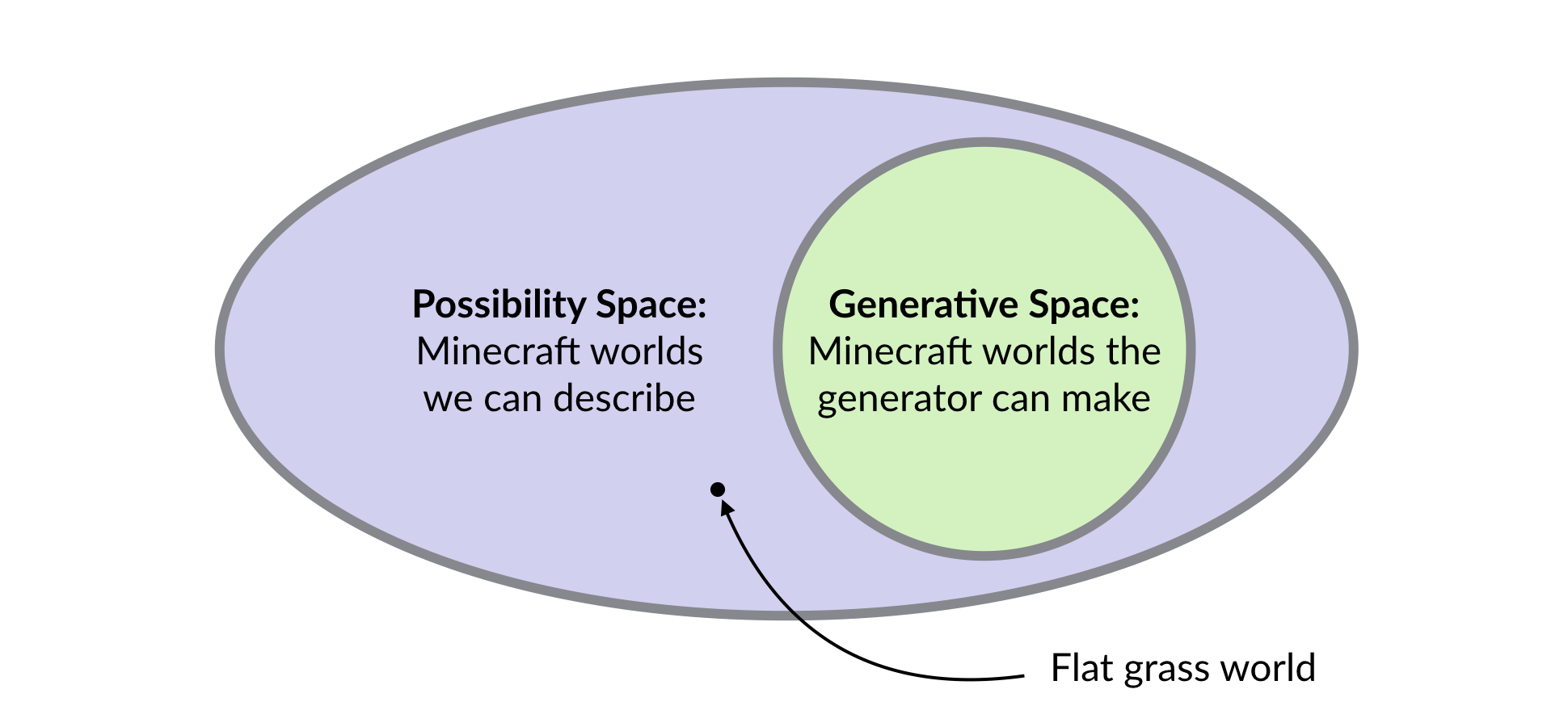
The scheme shown above gives us an idea of how it all looks. Everything that is in the Minecraft generation space should also be in its capability space, because if Minecraft can generate something, then it should be possible to create it in Minecraft! But not all of the space of possibilities is in the space of generation, including our example of the world consisting of grass. But in this scheme the scale is not respected. In fact, the space of Minecraft's capabilities is much, much larger than its generation space.
To understand how big it is, let's calculate the size of a separate Minecraft world. Volume is the width multiplied by the height multiplied by the depth. By default, Minecraft worlds have a height of 256 tiles, and they begin to break up into approximately 30,000,000 tiles in all directions from the starting point, that is, the approximate volume of the world is:
256 x 60 000 000 x 60 000 000 = 921 600 000 000 000 000 блоков
Each of these blocks can be any element: stone, grass, water, air. For our assessment, we will be generous and use only "blocks of the world", which, according to the Minecraft wiki, are 64 types . This means that every block in the world of Minecraft has 64 possible states. The formula for calculating the number of possible worlds is equal to the number of variants of the states of each block raised to the power of the number of blocks:
64921 600 000 000 000 000 возможных миров Minecraft
This number is so large that I could not find a calculator on the Internet that can calculate it.

To go deeper into the spaces of generation and possibilities, we need an example that we can better put in our head. Therefore, in the remainder of the tutorial, we will consider the levels of a 2D platformer for games like Super Mario Bros. or spelunky . We will look at several level generators for 2D platformers. In the original article, they are all interactive, so you can generate your own levels. In the process of their study, we will ask questions about their generation space. All examples are available on our GitHub as an open source sketch written in the Processing .
In the process of studying, I will ask questions in bold, for example:What is the size of the space of opportunities for these levels of platform? Feel free to skip them if you just want to read the article and play with examples. I also sometimes add hints to help in answering questions, for example:
Hint: levels are 40 tiles wide and 10 tiles high, and each tile can be one of three elements: void, ground, or a bonus block.
You can pause at the tooltip and do not scroll down the page if you do not want to read the finished answer. So let's take a look at our first generator.
Algorithm 1: Accident
Our first level generator is very simple - it is completely random. Each card has a start tile from the left edge and an exit tile from the right edge. All other tile cards have a probability of 33% to be empty, 33% to be occupied, and 33% to be a bonus block.

You probably noticed that this is not a very good level generator. In fact, in most of these levels it is even impossible to play, not to mention their interestingness. Generate a few more levels and think about the question: what size does the generation space of such a generator have in comparison with the space of possibilities?
Hint: can you think of a level that is in the space of possibilities, but not in the space of generation? Can you think of a level that this generator cannot create?
The correct answer is that the generation space of this generator is the samewith space of opportunity. Any tile on the map can take on any value, so any level that we can imagine is in the generation space. The most fascinating, the most interesting levels are in this generation space, even the map, on which the bonus blocks say SUPER MARIO IS AWESOME. Unfortunately, the generation space is so large that finding a good level is extremely unlikely. As you can be sure, when you press the generator, most levels are just rubbish.
Algorithm 2: drawing random shapes
Our next algorithm is a bit more complicated, and it creates levels that are more like what you can apply in the game. Starting from the empty level, he selects a random point somewhere, and then draws a line or a square of blocks of bonuses or earth. He performs this operation 20 times, creating several shapes, randomly drawn at the level. As before, you can click on the example in the original article to run the algorithm again and generate a new level.
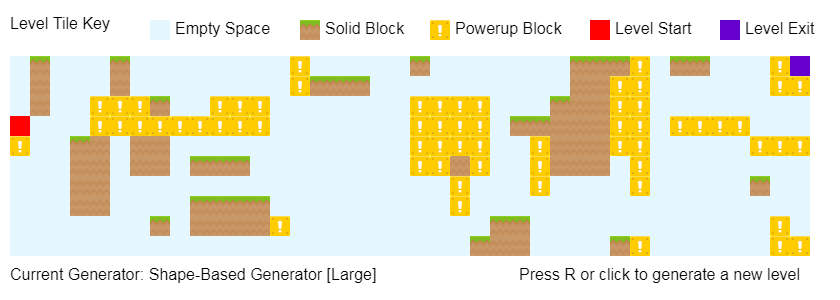
This is starting to resemble the level for the platformer, right? When generating levels, mentally imagine how you play them (be generous if necessary - add the ability to double jump, if necessary, or the ability to jump outside the ceiling of the map).
How many levels need to be generated before you find at least one that you can pass?
Do not strive for absolute accuracy in your mental walkthroughs, just make a rough estimate.
When I do this exercise with an audience, it usually takes about 10 attempts to find a level on average. And how much did you need? Remember this number, and now look at the variation of the same generator shown below. The generator is the same as we have just seen, but now it draws at the level of not 20, but 10 figures. Now count again: how many levels do you need to generate before you find the one you can pass?
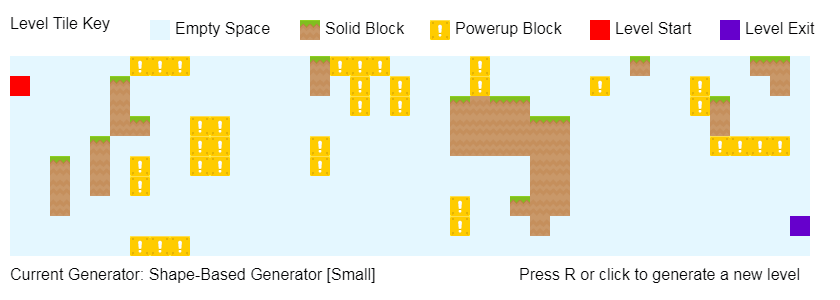
Probably, it took you less time to find a playable level. Let's stop for a minute and think about the differences between these two generators, which are almost identical, except for a small difference in parameters. Which generator has more generation space - a generator with 20 figures, or 10?
Hint: think about the level created by a 10-figure generator - can a generator with 20 figures be able to create it? And then think about the opposite example.
The correct answer is that a 20-figure generator has a larger generation space; in fact, it contains the entire generator generation space with 10 figures. To show this, imagine any level from a 10-figure generator. Our generator with 20 figures can create it by accidentally drawing the same ten figures, and then accidentally drawing them a second time on top of each other. However, no level with twenty individual figures can be drawn with a 10-figure generator.
This means that a generator with 20 figures has more levels, including interesting levels. But it is worth remembering that finding a playable level was much easier with a generator with 10 figures. If you had to choose a generator for your video game, which one would you use?There are no right answers here, but this is a very important decision that often has to be done when working with procedural generators. One generator can generate more variability and has more surprises, the other is more reliable and less annoying to the player.
Algorithm 3: level fragments
This algorithm is based on the level generator used in Spelunky; You can read more about it here . The level generator randomly selects the level fragment created by the person (me) and inserts it into the level. He then selects another random fragment of the level and inserts it side by side, and so on, until the level is completed. Here is the generator, the background is darkened, so that the borders of the fragments are more clearly visible:

How long will it take to generate a playable level now? You will find that almost every level is playable. We know exactly what is in each fragment, that is, we can guarantee that at the level there will be interesting objects that can be jumped on and obstacles that need to be avoided. At the same time, it is more dynamic than the static level - we do not know which fragments will appear in it and in what order. The Spelunky algorithm is even richer than this, it takes into account the movement along the vertical, and random variations are added to each fragment. Darius Kazemi created Spelunky’s awesome interactive level generator that can be found here .
Such generators are a good compromise between pleasant surprises and the possibility of control. When a generator makes a selection from a catalog of elements and connects them together (sometimes according to special rules, as in Spelunky), we call it a grammar-based generator . Grammar allows us to decide which building blocks a procedural generator should use, but less strictly as to how they should be put together. But this control and security come at a price. Look at the generator again, and then think about the previous examples. How often will such a level generator surprise us?
Initially, the generator is full of surprises, because we meet each piece for the first time. But if you play more, then quickly you start to notice where one ends and another fragment begins. Even if there are hundreds of combinations of fragments in this generator, after just a few levels you begin to feel as if you saw them all. The simplicity and ease of understanding grammar fragments are great for developers, but can be a disadvantage in terms of fun for players.
Algorithm 4: Human Design
As a final example, we will consider not an algorithm, but a very different kind of creative process: the work of people in level design. Click on the generator in the original article, and you will receive one of the first two levels of Super Mario Bros. (more precisely, their very beginning).
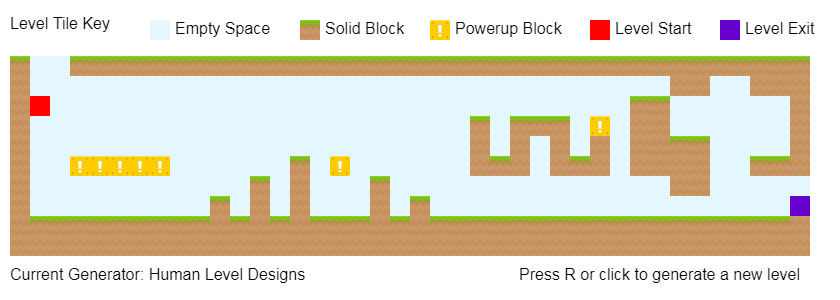
Starting with an absolute chance, we ended up with this, one of the most famous examples of 2D platformer design in history. Of course, this is not a generator, but in a sense, these levels can be perceived as generators, creating only one, high-quality level. Maximum compromise between control and quality. Looking at this level, think about all the generators that we considered today, and think about the question: which of these generators contain in their generation space these famous levels? .
- Random generation
- Generation of 20 figures
- Generation of 10 figures
- Level Fragments
Only the first generator is able to create such levels. Generators of forms could create them if we increased the number of possible drawn shapes, but this would also significantly increase the number of bad or strange levels. The level fragment generator could do this if we gave it a different set of fragments, but would all the other combinations of these fragments be playable?
This shows us how difficult it is to create a procedural generator. We want to think of our generators as normal game content, to imagine how a player passes a certain level and gets pleasure from it. But as generation designers, we need to think about the whole generation space, and not just about a single example of it. How big is the space? How rich is it for surprises? How often does it create something boring or bad? Can we detect and filter these cases, or do we need to find a workaround? These are just some of the issues that need to be considered when creating something for generation.
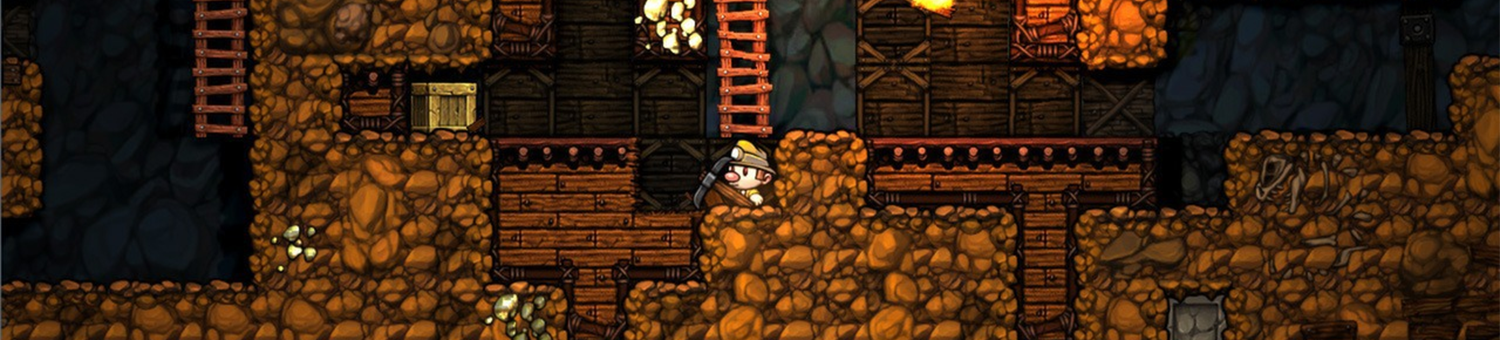
Summarize
Today we learned about the following concepts:
Generation space
The generation space of a procedural content generator (for example, the Minecraft world generator) is the set of all the results it can generate. If we change the algorithm or specify a different value for the variable, the generation space will change.
Space of opportunity
The space of opportunities for a certain type of content (for example, the world of Minecraft) is the set of all examples of such content that we can present or describe. Usually, but not always, it is much larger than the generation space of the procedural generator.
More is not always better
A generator with a large generation space usually has more good content, more unexpected content and more diverse content. But he usually has more junk content, more boring content, and more unusable content.
A generator with less generation space is easier to control, easier to test, and easier to understand. But it can make it more predictable and less surprising.
Finding ways to balance the strengths and weaknesses of these two opposites is the art of creating generation software!
Additional reading and thanks
This ends my tutorial! Thanks for reading.
Among the interesting materials on the topic reviewed by us, I recommend the following:
- The Spelunky book by Derek Yu is a great story about how Spelunky design was created, including a discussion of the procedural generator of the game.
- Tracery Tutorials, by Kate Compton - learn how to write text-based grammar, a great way to practice thinking about the generation space!
- The head of Dr. Gillian Smith's excellent book on the range of expressiveness (which we will look at in a future tutorial).
- Dr. Smith has a lot of free resources, for example, her excellent report at GDC on compromises in procedural generation.
- And finally, do not forget that all the generators from the article can be found in the Processing-sketch with open source ( here ).