American scientists have taught robots to use auxiliary tools

A monkey (chimpanzee) takes out termites from a termite using a stick. In the second photo, the gorilla uses a stick to collect the grass she needs.
Developers from the USA created a specialized algorithm for robots , which made it possible for the latter to use additional tools to complete the task. But it is relatively simple - in a certain way to move an object from point A to point B.
The algorithm consists of two parts. The first allows the robot to move objects randomly, performing a kind of experimentation. The second part makes it possible to assess the consequences of a particular action using a neural network. As it turned out, the robots, working according to the algorithm, effectively used auxiliary tools without training.

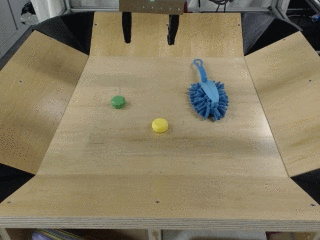


A person uses many additional tools every day. Animals are also capable of this - parrots, crows, monkeys and some other living creatures can use sticks, pebbles, thorns to achieve their desired tasks (for example, extracting a beetle larva from under the bark).
For us, the use of a knife for slicing bread is quite obvious. But for a robot that needs to explain every action in the form of a machine language - not at all.
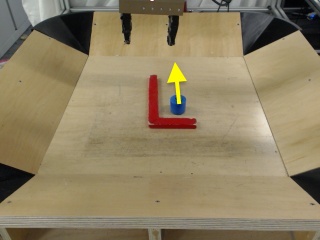
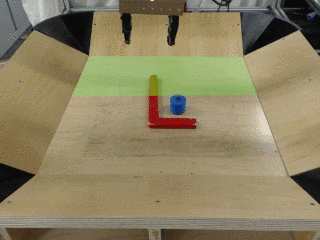
Scientists from the University of California at Berkeley have developed a way to train robots to use auxiliary tools and make decisions on their application in a given situation. The algorithm “visualizes” the task, showing the robot in which direction the example should be moved. And already the robot (this is an ordinary robotic manipulator) takes an instrument and moves an object. All movements are tracked on camera.

The algorithm is based on a neural network. A specialized program calculates different sequences of actions for a robot that are "fed" to a neural network. That generates a video that shows what will happen as a result of the execution of a particular action.
The manipulator is given a command to perform an action. If the real result coincides with the planned one, the task is counted. In the course of work, various video options are compared with the image that is provided by the user and displays the final result of the task. After the optimal sequence of actions is found, the robot proceeds to solve the problem.


This project combines machine learning approaches such as non-demonstration learning and simulation training. In the first case, the robot selects options randomly. As a result, a significant sample of data is generated, which helps to achieve an optimal result. In the second, the developers clearly showed the robot how to use the tool in one case or another. It is in this situation that the reference solution is recorded on the camera with which the robot compares the consequences of its actions.
In some cases, the robot "understands" that it is best to complete the task without auxiliary elements - and does it. Tools are used without training, even if the robot has not been shown before.

