
How Fukuoka Airport found out which measures would be effective to reduce queues
Before you is not a fictional picture from the advertising magazine "Airports of the Future." This is a real operating airport in the Japanese city of Fukuoka, which offers passengers a lot of free space to move. Thanks to the special modeling technology, the reasons for queuing were identified and optimization measures were taken. Who cares how this technology works, please, under cat.
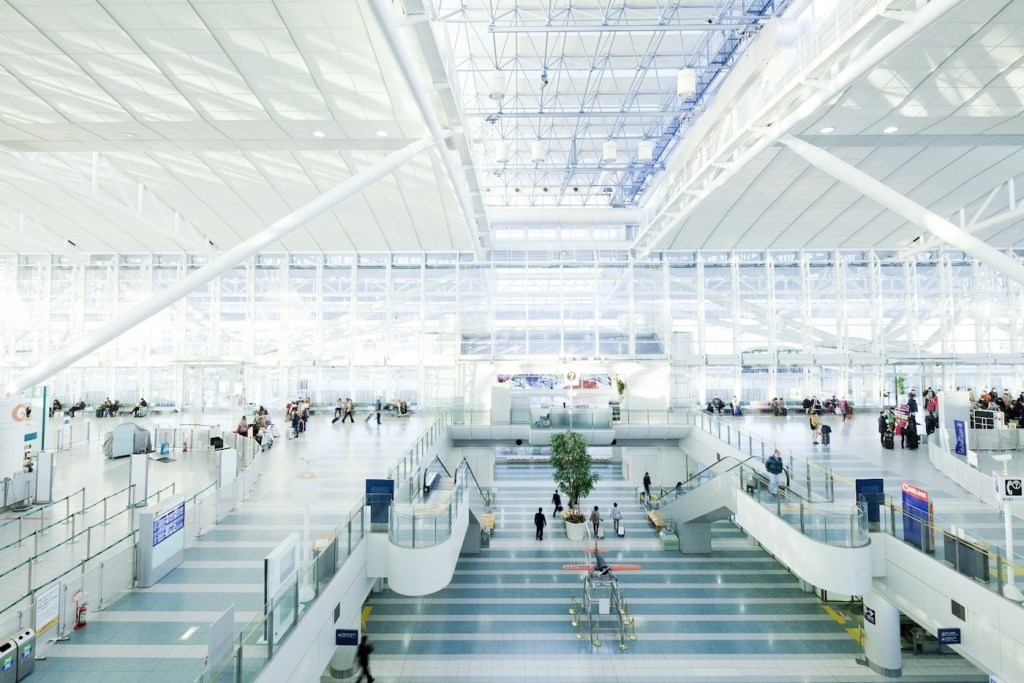
Photo courtesy of Fukuoka
Professor Shingo Takahashi, head of the Department of Industrial Systems and Control Systems Engineering at Waseda University and Fujitsu Laboratories, have developed a technology that identifies the reasons for crowding in public places. The solution automatically analyzes the factors that led to the formation of a large mass of people, based on the results of modeling human behavior.
Back in 2015, Fujitsu and Professor Takahashi used this technology in a system of modeling human behavior, which analyzed measures to eliminate queues at the airport in Fukuoka. They were able to detect 4 times more causes of crowds in comparison with the analysis of experts. For example, when analyzing a crowd of people during a screening of passengers and baggage, the system was able to detect for the first time that passengers gathered at a particular check-in desk caused an unexpected crowd of people in the check-up area. During the simulation, it was confirmed that this technology reduces the number of people awaiting the passage of the inspection by one sixth. In addition, the number of staff can be reduced by a third. And the analysis time was significantly reduced,
The new development combines groups into categories that have a certain commonality and expresses the characteristics of the corresponding “agents” (simulated behavior, actions of people) in the form of a small number of combinations of categories without compiling lists of movement results or routes of tens or hundreds of thousands of agents. This approach makes it easier to distinguish the characteristics of agents associated with the causes of crowds, and makes it possible to create parameters related to certain characteristic signs and examples of movement.

Simulation diagram of people’s behavior and forecast of congestion at the airport
Previously, due to the fact that the data related to the distinguishing features, perceptions and actions of agents (for example, the agent’s task is to “have lunch” or the agent saw a pointer at a point), which should be expressed in the form of dozens of records in the database, were combined to create agent characteristics, this process created a large number of combinatorial examples. With the new technology, which creates logical groups that include similarities in characteristics, and creates clusters of agent characteristics for each group, the ability to reduce the number of combinatorial examples was realized. This allows you to search for causes that are directly related to countermeasures, and get an answer to the question of what measures will be effective to reduce crowds.
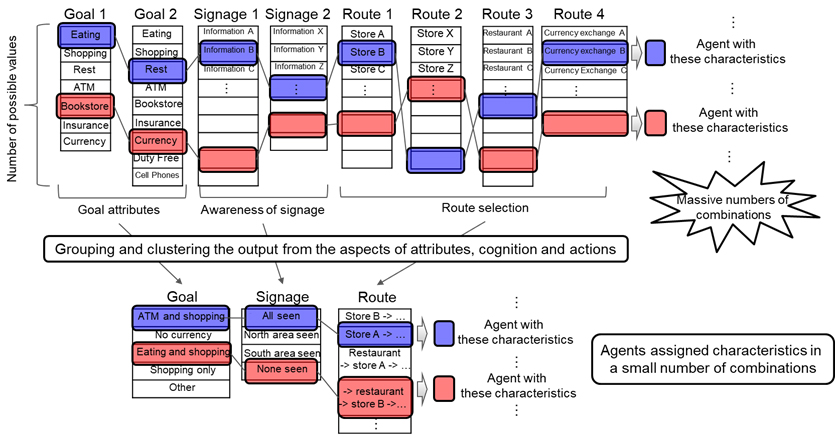
Identifying the full range of reasons for the formation of crowds, based on the distinctive features, actions and ways of perception.
For example, with regard to crowds that occur in store A and B in a shopping center, when a crowd is detected, it can be determined that the crowd in store A was caused by people, who saw the sign, and the congestion in store B was caused by people who had finished lunch in the cafe and all came to the store together. Thus, congestion in store A can be eliminated by installing new signs, and congestion in store B can be eliminated by increasing the number of staff and speed of service.

Identification of the causes and examples of counteractions that have received thanks to technology
When modeling human behavior, experts usually repeat the trial and error process, analyzing the large amounts of data that have been obtained as a result of modeling, and suggest hypotheses of the causes of crowds and possible countermeasures based on their experience and information, and then re-performing the simulation to evaluate suggested hypotheses. Accordingly, an analysis of the proposed causes and determination of countermeasures can take several months. And in some cases, when the analyst missed certain reasons, additional problems may arise. The new technology of professors Takahashi and Fujitsu Laboratories provides a comprehensive identification of the characteristics of agents that are related to crowds. Consequently, the number of combinatorial examples decreases. This allows you to search for reasons
The technology allows for the rapid assessment of parameters to prevent the accumulation of people in places of trade, places of various events and in other places in which crowds can form due to their high attendance or centralization. Thus, the new development makes it possible to increase the safety and comfort of urban environments.
Venues, airports and shopping centers, where large masses of people are often formed, can negatively affect the level of customer satisfaction and, ultimately, sales. Currently, in addition to ways to solve this problem, such as increasing the number of personnel deployed at entrances, exits and points of sale to assist visitors, there are a number of other measures that include the installation of special signs and patterns to move visitors to less busy premises. However, to implement more effective means of reducing the flow, it is important to understand what types of people will take what types of actions in response to what types of information.
For this, technologies of "modeling human behavior" are increasingly being used. Specialists perform modeling of distinctive features, perceptions and actions of various groups of people as “agents”. And with the help of computer-based virtual modeling of queuing situations, scientists can analyze the causes and evaluate solutions that will prevent crowds.
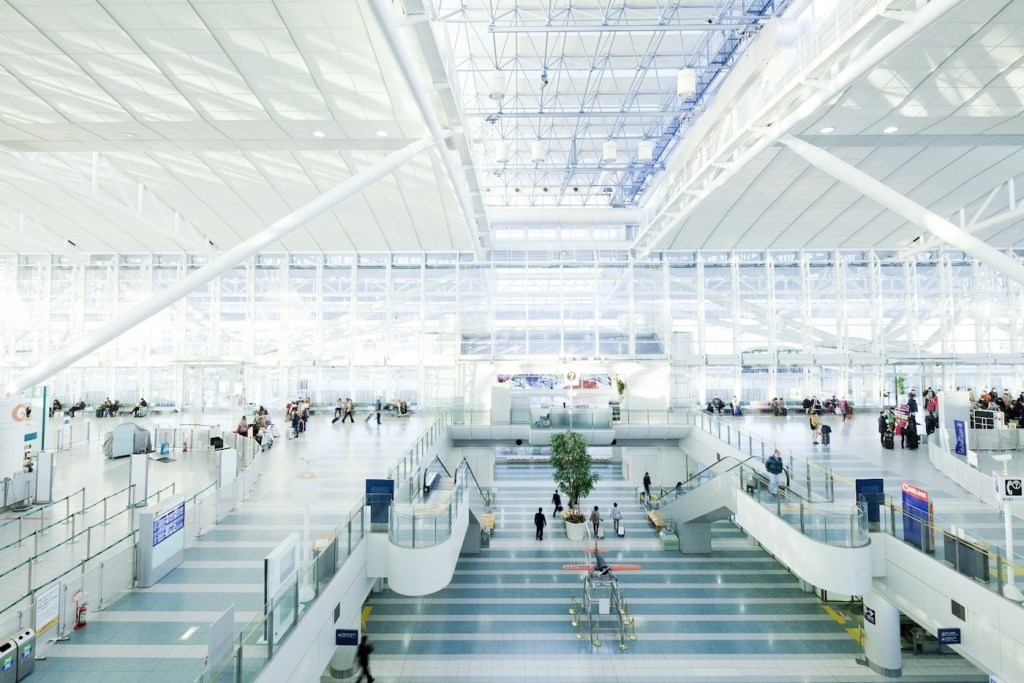
Photo courtesy of Fukuoka
Professor Shingo Takahashi, head of the Department of Industrial Systems and Control Systems Engineering at Waseda University and Fujitsu Laboratories, have developed a technology that identifies the reasons for crowding in public places. The solution automatically analyzes the factors that led to the formation of a large mass of people, based on the results of modeling human behavior.
Back in 2015, Fujitsu and Professor Takahashi used this technology in a system of modeling human behavior, which analyzed measures to eliminate queues at the airport in Fukuoka. They were able to detect 4 times more causes of crowds in comparison with the analysis of experts. For example, when analyzing a crowd of people during a screening of passengers and baggage, the system was able to detect for the first time that passengers gathered at a particular check-in desk caused an unexpected crowd of people in the check-up area. During the simulation, it was confirmed that this technology reduces the number of people awaiting the passage of the inspection by one sixth. In addition, the number of staff can be reduced by a third. And the analysis time was significantly reduced,
The new development combines groups into categories that have a certain commonality and expresses the characteristics of the corresponding “agents” (simulated behavior, actions of people) in the form of a small number of combinations of categories without compiling lists of movement results or routes of tens or hundreds of thousands of agents. This approach makes it easier to distinguish the characteristics of agents associated with the causes of crowds, and makes it possible to create parameters related to certain characteristic signs and examples of movement.

Simulation diagram of people’s behavior and forecast of congestion at the airport
Previously, due to the fact that the data related to the distinguishing features, perceptions and actions of agents (for example, the agent’s task is to “have lunch” or the agent saw a pointer at a point), which should be expressed in the form of dozens of records in the database, were combined to create agent characteristics, this process created a large number of combinatorial examples. With the new technology, which creates logical groups that include similarities in characteristics, and creates clusters of agent characteristics for each group, the ability to reduce the number of combinatorial examples was realized. This allows you to search for causes that are directly related to countermeasures, and get an answer to the question of what measures will be effective to reduce crowds.
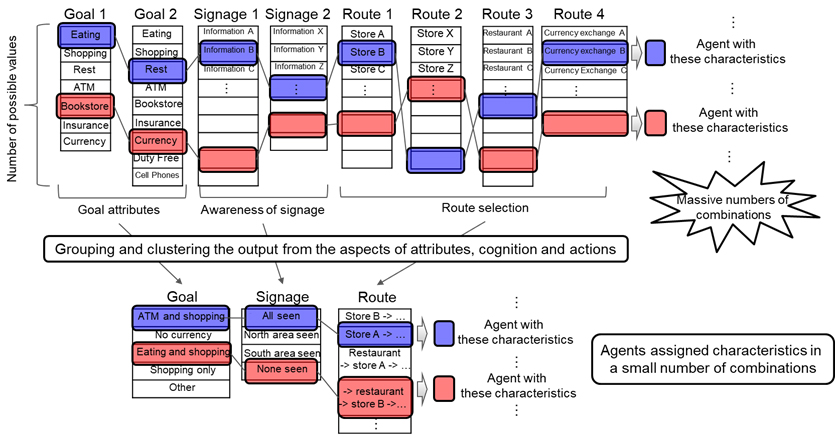
Identifying the full range of reasons for the formation of crowds, based on the distinctive features, actions and ways of perception.
For example, with regard to crowds that occur in store A and B in a shopping center, when a crowd is detected, it can be determined that the crowd in store A was caused by people, who saw the sign, and the congestion in store B was caused by people who had finished lunch in the cafe and all came to the store together. Thus, congestion in store A can be eliminated by installing new signs, and congestion in store B can be eliminated by increasing the number of staff and speed of service.

Identification of the causes and examples of counteractions that have received thanks to technology
When modeling human behavior, experts usually repeat the trial and error process, analyzing the large amounts of data that have been obtained as a result of modeling, and suggest hypotheses of the causes of crowds and possible countermeasures based on their experience and information, and then re-performing the simulation to evaluate suggested hypotheses. Accordingly, an analysis of the proposed causes and determination of countermeasures can take several months. And in some cases, when the analyst missed certain reasons, additional problems may arise. The new technology of professors Takahashi and Fujitsu Laboratories provides a comprehensive identification of the characteristics of agents that are related to crowds. Consequently, the number of combinatorial examples decreases. This allows you to search for reasons
The technology allows for the rapid assessment of parameters to prevent the accumulation of people in places of trade, places of various events and in other places in which crowds can form due to their high attendance or centralization. Thus, the new development makes it possible to increase the safety and comfort of urban environments.
Relevance
Venues, airports and shopping centers, where large masses of people are often formed, can negatively affect the level of customer satisfaction and, ultimately, sales. Currently, in addition to ways to solve this problem, such as increasing the number of personnel deployed at entrances, exits and points of sale to assist visitors, there are a number of other measures that include the installation of special signs and patterns to move visitors to less busy premises. However, to implement more effective means of reducing the flow, it is important to understand what types of people will take what types of actions in response to what types of information.
For this, technologies of "modeling human behavior" are increasingly being used. Specialists perform modeling of distinctive features, perceptions and actions of various groups of people as “agents”. And with the help of computer-based virtual modeling of queuing situations, scientists can analyze the causes and evaluate solutions that will prevent crowds.