35% stock return on alternative data
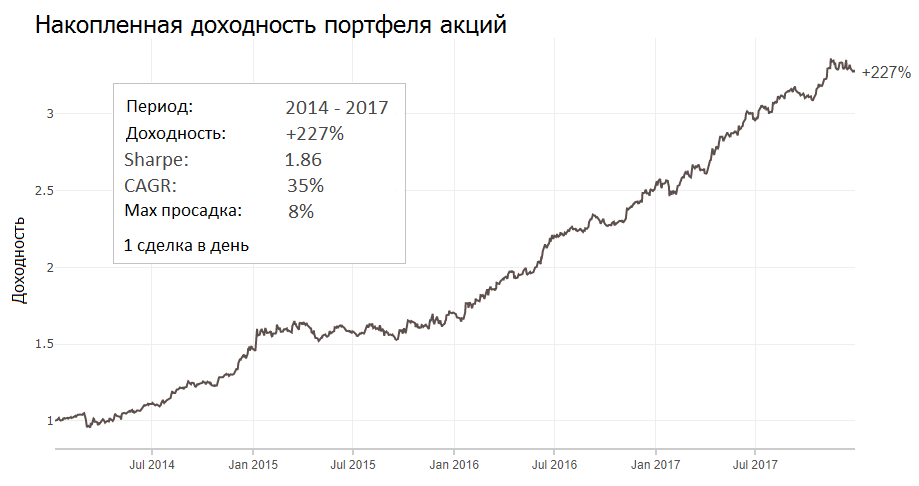
Trading on alternative (non-standard) data is becoming fashionable and promising. The other day I got into the hands of a curious dataset from the Moscow Exchange on popular stocks. After a superficial study, we managed to get an attractive result with good returns. Details under the cut
Dataset content
The dataset contains three values pv30, pv70, pv100 for each day and shows the difference in purchases and sales of a group of the top 30, 70 and 100 largest traders for the current day. Those. answers the question, what did the big players do today? More bought or sold? For example, pv100 = 500 means that a group of top 100 traders in the aggregate bought 500 units. more than sold.
On the exchange website you can see the full description of the dataset and get historical values
Data coverage:
- 10 shares: SBER, GAZP, LKOH, GMKN, MGNT, ALRS, AFLT, ROSN, SBERP, VTBR
- 4 years: 2014 - 2017 (open data on the exchange website)
Further, we will consider data only on SBER , the results of the remaining 9 shares in the appendix below
Data Overview
Statistical description of pv values for SBER: Dynamic

data and their distribution:

All three pv30, pv70 and pv100 values are strongly correlated (> 0.95) with each other and distributed close to normal with a center near zero. The largest interquartile range for pv30.
SBER price and cumulative pv100:

Today's yield strongly correlates with today's pv values of ~ 0.8. Thus, we can assume that the price is moved by participants gaining a large position. The correlation between the price movement tomorrow and the pv value for today is ~ 0.1, which means that there is a weak correlation between them and you can try to predict the direction of tomorrow's price movement according to pv data for today.
Trading model
We will construct a simple model if today the value pv> 0, we assume that the price will rise tomorrow, otherwise it will fall. The pv values are compared with zero, as the average and median pv values are distributed around zero. Simply put, if today major players bought (pv> 0) the next day we also buy and vice versa.
Features of the model:
- Only pv values are used in the model, and asset price information is not used.
- We will open the position at 18:40 - 18:50 at the closing auction and close the next day, at the same time. The position opening time is chosen as pv values are published at 18:30
- If pv> 0, open a long position (buy). If pv <0, open a short position (sell)
- If pv is positive / negative for two or more days in a row, do nothing (hold). Thus, the size of an open position is always constant
- The transaction fee is assumed to be 0.025%
- Daily returns will be taken from close to close (close to close)
Trading Model Results
Let us compare the profitability of the “buy and hold” strategy (Base) and the strategy according to the values of pv30, pv70 and pv100 for a period of 4 years according to SBER:
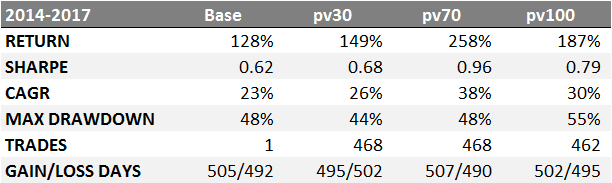
RETURN - profitability of the model for 4 yearsComparison of models in dynamics: A
SHARPE - Sharpe ratio, risk-free profitability rf = 6%
CAGR - average annual growth rate
MAX DRAWDOWN - maximum drawdown
TRADES - number of completed transactions
GAIN / LOSS DAYS - number of days when the price movement guessed and did not guess
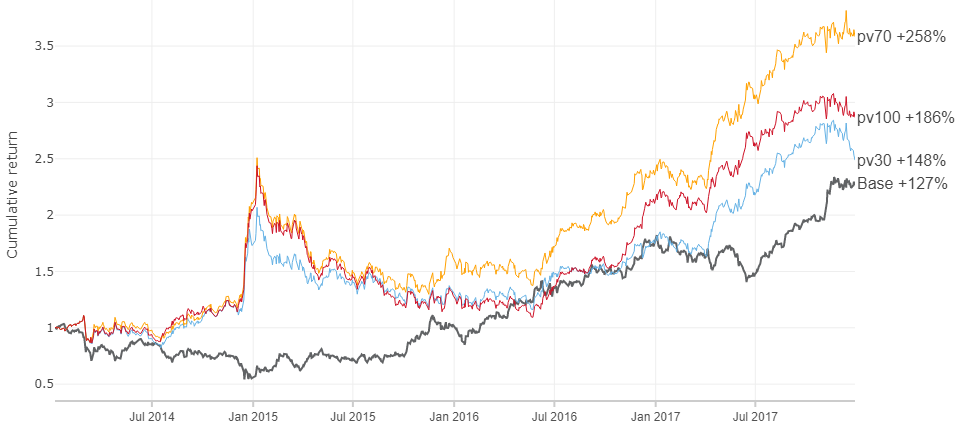
quarterly comparison of the returns of the basic “buy and hold” model against the pv indicator. You

can see similar results for the other nine instruments in the appendix below.
Stock portfolio
Using SBER as an example, we received high returns relative to the asset itself, but nevertheless, we observe a large drawdown for the entire 2015. This picture is also observed for other stocks at different times (see the appendix). But what if you scatter money on all ten shares? Then, probably, we can avoid large drawdowns.
You can scatter equally, but also in proportion to the corresponding liquidity and capacity of the instrument.
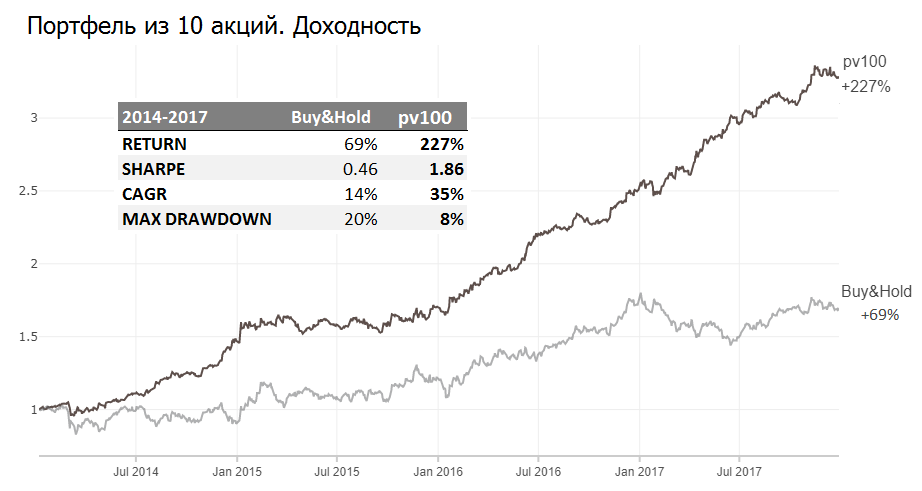
portfolio of 10 securities controlled by pv100 showed a yield of 35% per annum and less drawdown than the buy strategy and hold it. "
Going over weights you can get both 15% and 50%, but it’s important that by scattering funds over many assets, we avoid large drawdowns in our trading model.
Observations left outside this material
- Derivative values based on pv also show good returns (good = higher than the market): sma with short periods, pulses, volume rationing, and other similar techniques from technical analysis
- During periods of reduced volatility, pulses of pv values are better manifested
- Pv values have good sensitivity to rare strong price movements, i.e. the percentage of guessing price movements over 3% reaches ~ 75%, while the total percentage of guessing is ~ 50%
- The distribution of pv by day of the week is different, especially Mon. from Fri. Probably, at the beginning of the week there is a set of positions, and by the end of the closing
- In the trading model discussed above, the pv value was compared with 0, although 0 is not the most optimal point for maximizing profitability
- Each of the 10 shares has its own characteristics in terms of pv
My findings
The hypothesis of following large players in terms of pv with a lag of one day showed a result above the market in the segment 2014-2017. It would be presumptuous to say that this will always be so. What will be on the new data? On the one hand, there is no good reason why everything can break, and on the other, who knows :) For more confidence, you need more points and fresh data.
The model could be made more complex and get fantastic returns by adjusting to the available data, but then the risk of overfit is high.
A couple of questions to the audience, what do you think:
- Why can this data work?
- Why may this data not work?
application


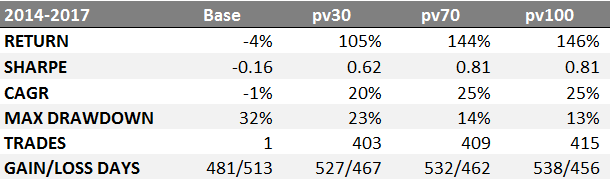


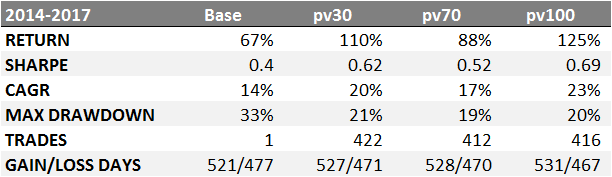

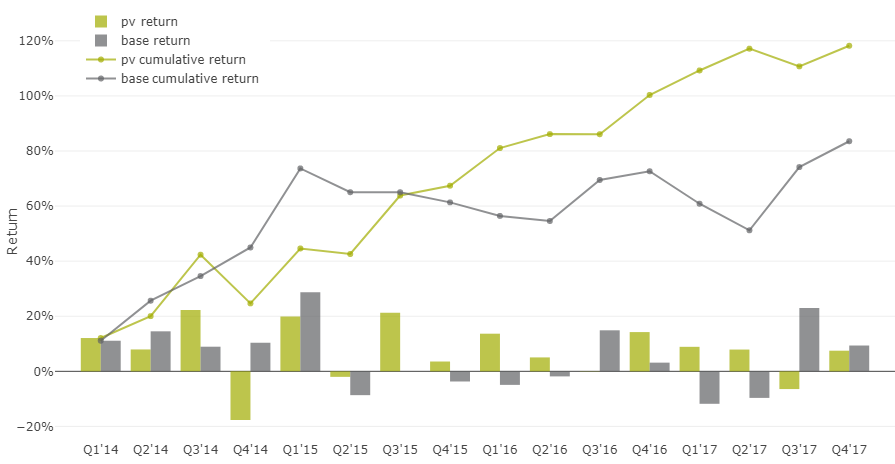
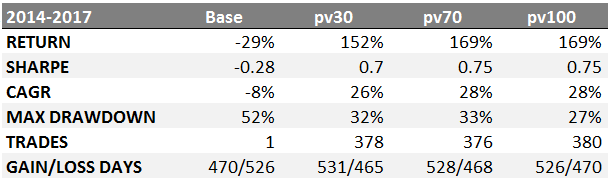
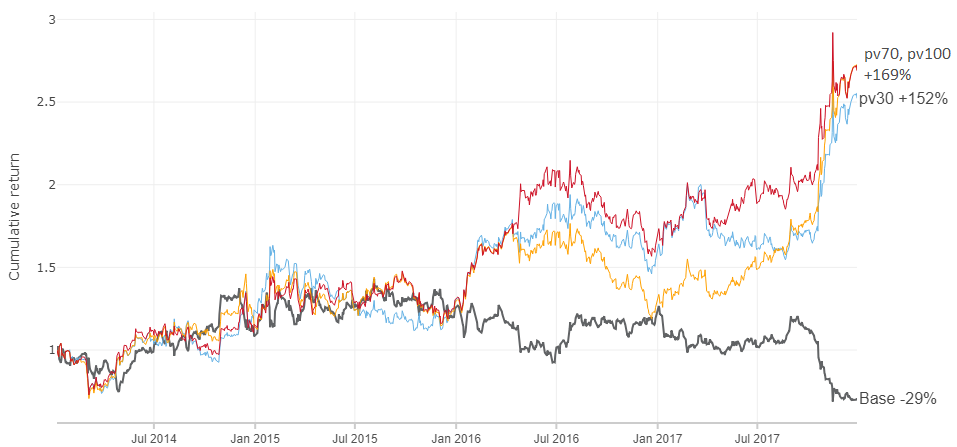





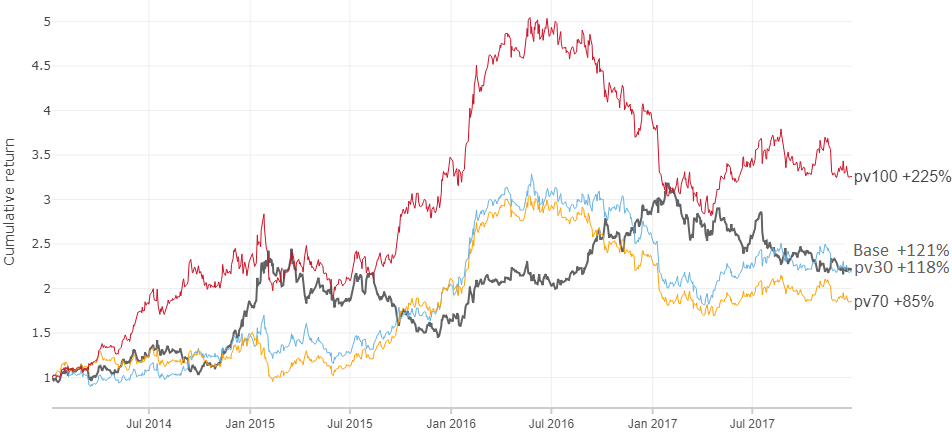
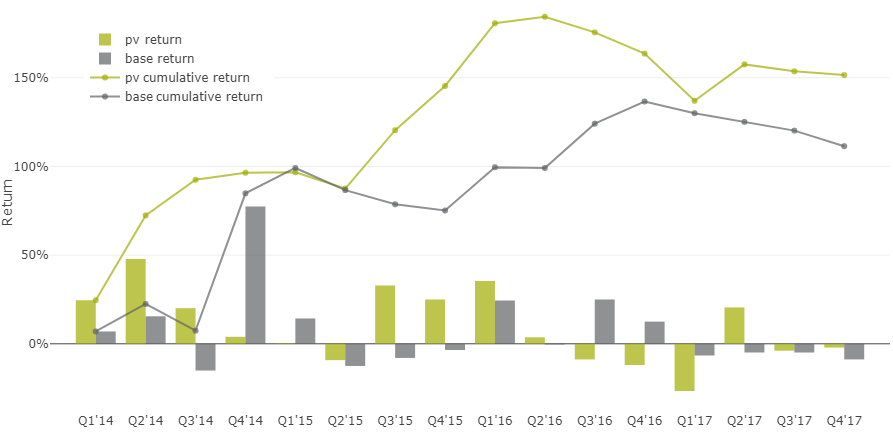

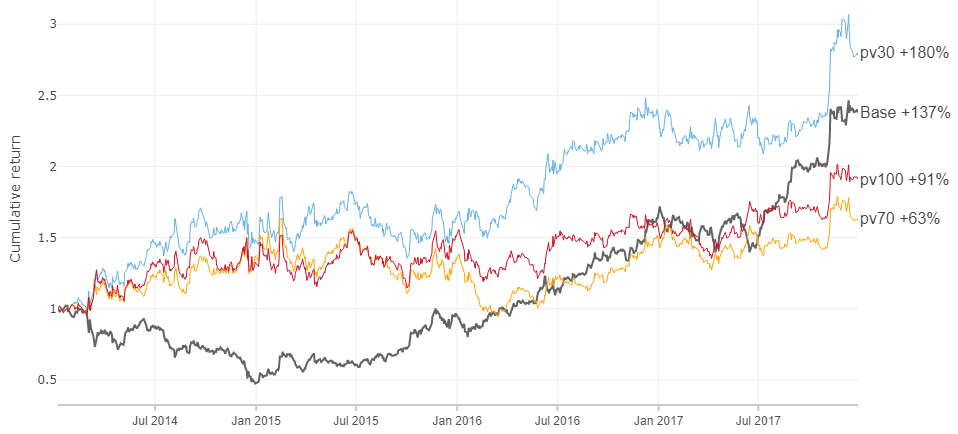

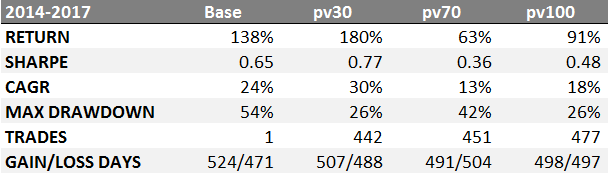



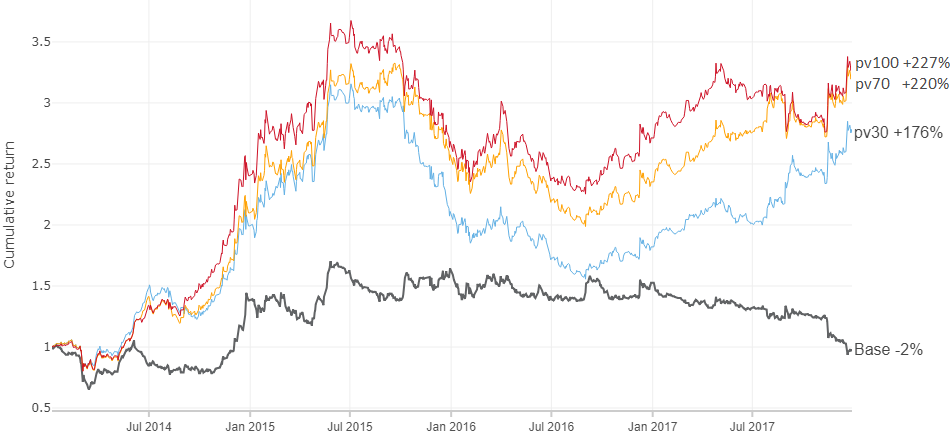

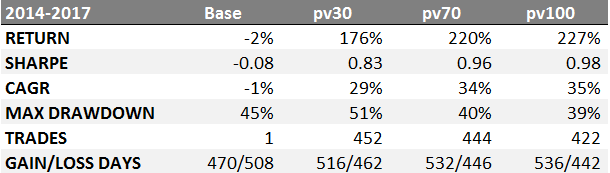
application
Gazp


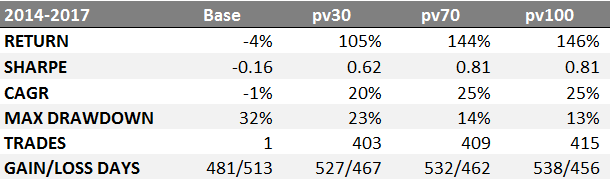
Lkoh


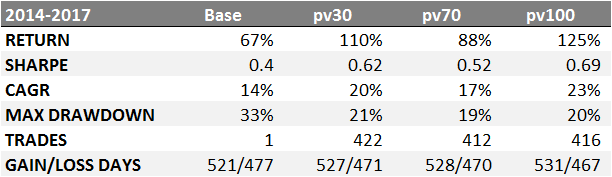
GMKN

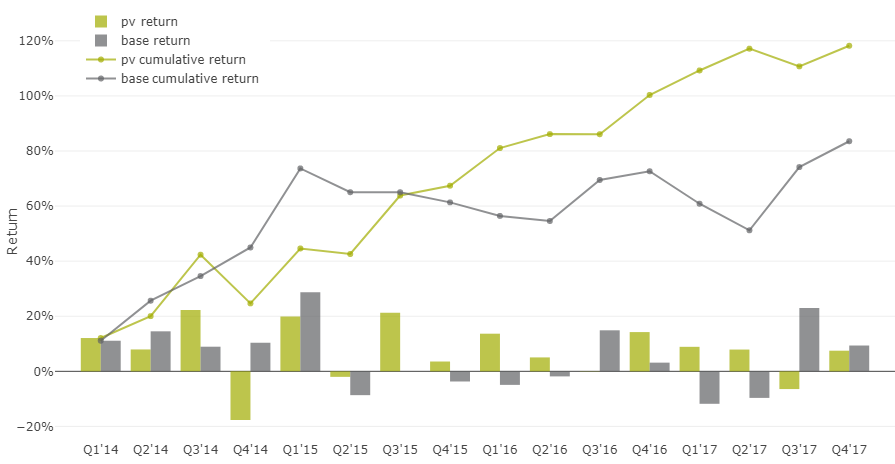
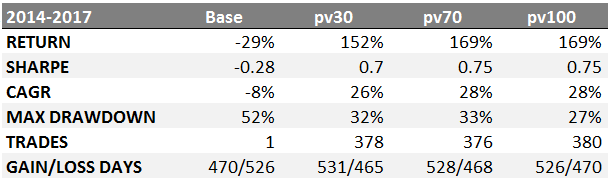
MGNT
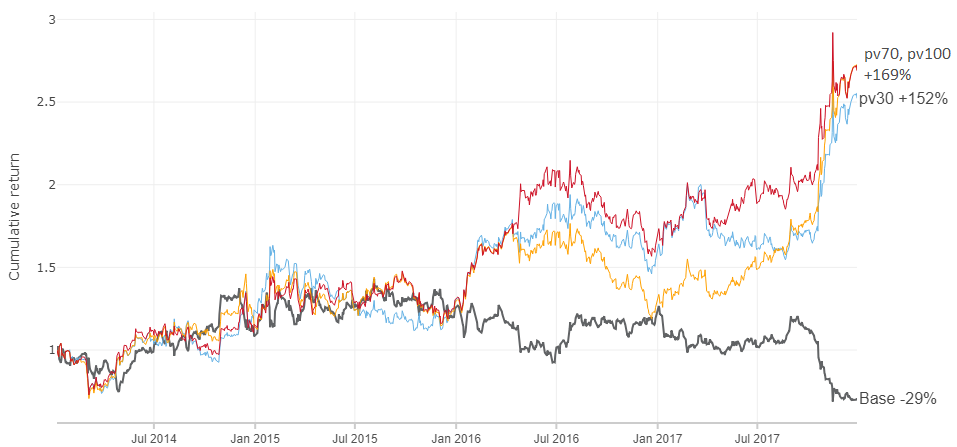


Rosn



ALRS
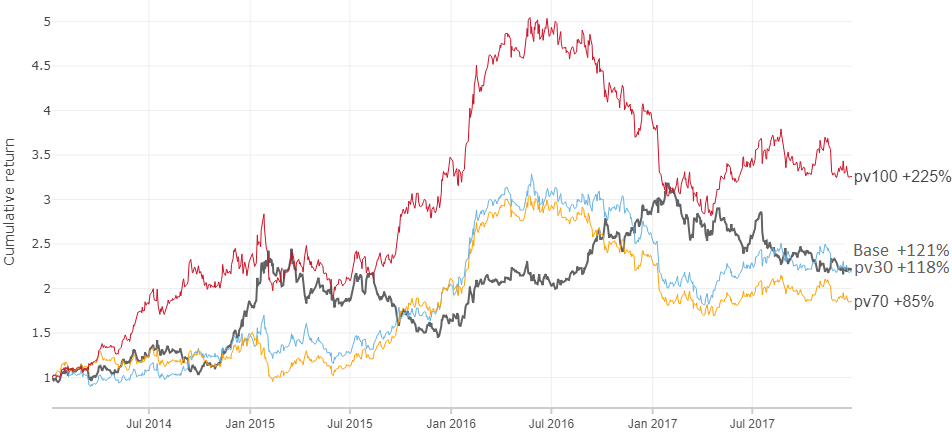
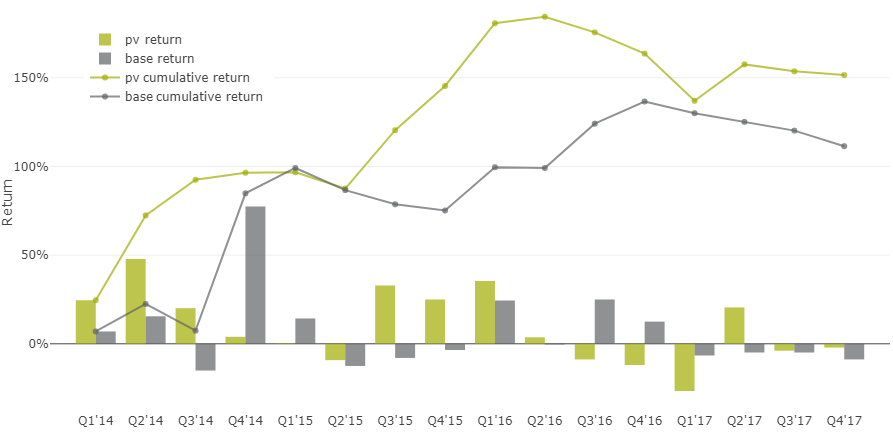

SBERP
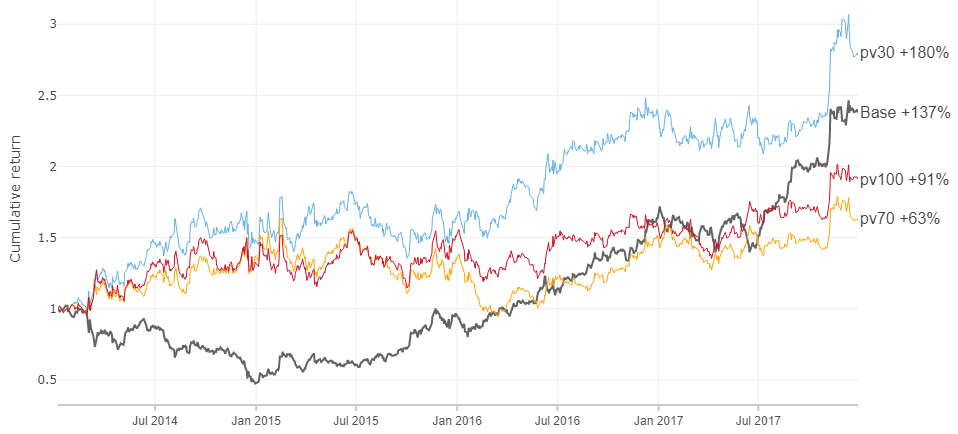

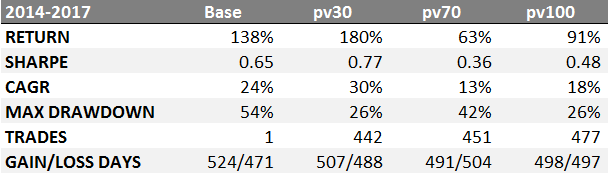
AFLT



VTBR
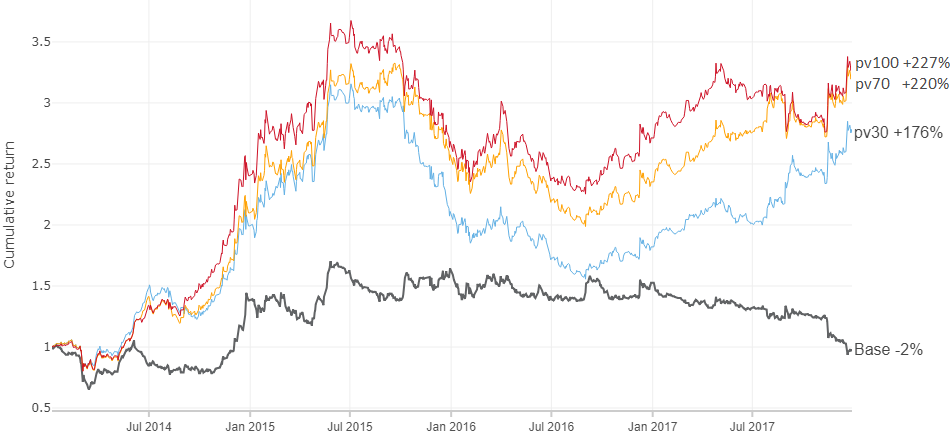

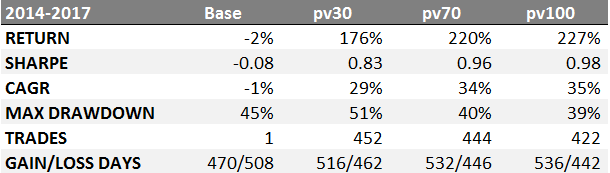