X-ray recognition: precision = 0.84, recall = 0.96. Do we need more doctors?
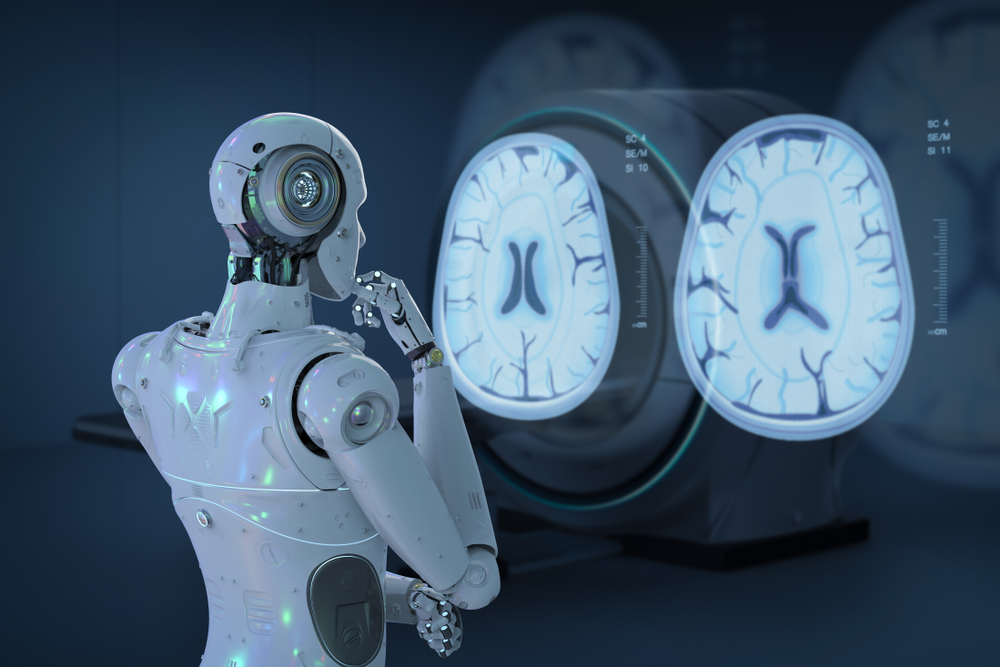
Recently, the use of AI in medicine has been increasingly discussed. And, of course, the field of medicine, which directly begs for such an application, is the field of diagnosis.
It seems that earlier it was possible to apply expert systems and classification algorithms to the tasks of diagnosis. However, there is one area of AI that has achieved the greatest success in recent years, namely the area of image recognition and convolutional neural networks. On some tests, AI algorithms in image recognition have surpassed humans. Here are two examples: Large Scale Visual Recognition Challenge and German Traffic Sign Recognition Benchmark .
Accordingly, the idea arose to apply AI to the area of image recognition where the doctors are engaged in image recognition, namely, in the analysis of images and, for a start, X-rays.
Roentgenoscopy is used to diagnose a wide range of diseases and injuries: lung damage (pneumonia, cancer), fractures and other bone damage, part of the diagnosis of the digestive system, and much more.
It is important that in the diagnosis of some of these diseases, an X-ray and its interpretation is the prevailing tool in the diagnosis.
Interpretation of the image, in turn, is done by the radiologist based on visual image analysis. The question arises: what if we apply the progress in image analysis using AI to the analysis and interpretation of X-ray images. What happens?
Will we be able to achieve a quality comparable to doctors? Or, maybe, the classification accuracy will exceed the accuracy of doctors, as it exceeded in the recognition of pictures in the Large Scale Visual Recognition Challenge ?
At Kaggle, there are now several X-ray analysis competitions to diagnose pneumonia. For example, one of them.
Here, 5,863 images were labeled by doctors, each of the images was labeled by two doctors, and only if they coincided in the diagnosis was the image added to the data set. Patients for images were not specifically selected (all images were taken as part of normal work with patients). The set of classes is balanced towards pneumonia, which is probably close to real life, since the pictures are taken by patients already with suspected pneumonia.
The best solution is precision = 0.84 and recall = 0.96. Then the question arises: is it a lot or a little ... A good question is.
Just in case, we remind that precision is what percentage of those patients whom the model has identified as having pneumonia, are really sick with pneumonia (and, accordingly, what percentage of this will be the doctors, so they will mistakenly treat for the wrong disease). Recall - this is the percentage of all patients with pneumonia that the model finds (the opposite of this percentage is how many patients with pneumonia the model will mark as healthy).
So, is it a lot or a little? Well, you can look at this question like this: what about doctors? They have some precision and recall.
To do this, you would need to plant a group of doctors, give them pictures for the markup, and then compare the quality of their markup with the quality of the markup by an algorithm similar to the German Traffic Sign Recognition Benchmark , where the quality of recognition of road signs was compared. As far as I know, no one has yet done this with the doctors.
But suppose that we did this and it turned out that the quality of the markup using the algorithm is comparable to the quality of the markup by the doctor. If this is not the case now (which is not a fact), then I am sure that this will happen in the near future. What's next?
Replace radiologists with artificial intelligence? This has been especially long dreamed of in the United States, where radiologists are very highly paid and probably deserved, given their importance in the formulation of a certain type of diagnoses.
Let's see how the process of using the algorithm should look like in practice?
- Firstly, it would be necessary to standardize the format and quality of the issuance of images on various X-ray equipment. It may be standardized now (I am not an expert), but for some reason it seems to me that it is not. If this standardization is not, then it will be impossible to guarantee the stability of the model during the transition from one installation to another.
- Secondly, it will be necessary to add regular quality control models. That is, the model should regularly receive a test sample marked by the doctors at the entrance and the quality of its work must constantly be validated. On all models used in all clinics. This means that there must be one centralized model (or a very small number of them), since otherwise too many resources are needed for all models to validate. Logically, probably, manufacturers of X-ray equipment will come to the conclusion that together with the X-ray machine they will supply the model.
- Thirdly, confidence thresholds should be built into the model; if they are exceeded, the image is still sent to the doctor for classification.
As you understand, even if the models are now comparable in quality or superior to doctors, their (doctors) replacement or, more precisely, staff reductions require a set of process steps. I'm not talking about a set of regulatory and certification steps, which are generally necessary for the implementation in practice of such a solution.
In general, we are still far from the scenario, as it seems to me.
Is another scenario possible? I think so. Let us recall the Condorcet Theorem ( Condorcet Jury Theorem ), which suggests that the probability of a correct decision being made by a group of people is higher than each of them individually. Thus, the quality of the classification together of the doctor and the model is higher than the quality of the classification of any one of them.
Thus, the doctor can use the model as an adviser. What for? Because the doctor himself has his own precision and recall. Suppose that doctors do not call it that, but there are mistakes. Some mistakes lead to the fact that some of the diseases are missing. I think there are fewer such errors, as the doctors are just trying to minimize the error of the first kind. Other errors lead to the fact that people are being treated for pneumonia, which they do not have, and some places in hospitals are occupied unnecessarily. How many total errors are unknown, but they exist.
Accordingly, let us imagine that we use two different models and the opinion of the doctor regarding some snapshot. Plus from Condorcet's theorem that she not only claims that two heads are better than one, but also allows you to calculate how much.
Let each of them (each model and the doctor) have an accuracy of 0.84 (of course, we do not know the accuracy of the doctor, but suppose that it is not lower than the accuracy of the models). Then, by the Condorcet theorem, the total accuracy is equal to 0.84 ^ 3 + 3 * 0.84 ^ 2 * (1 - 0.84) = 0.93, which gives a clear increase compared to the original accuracy of 0.84. Thus, applying the model, the doctor becomes clearly more accurate in his predictions.
Note that in this paradigm we keep the holy of holies, we leave the final decision for the doctor, not shifting it to the car. This, I think, will facilitate the implementation of such solutions and open the way for AI advisers in medicine.
What do you think?
As usual, you want to master the use of convolutional neural networks and computer vision in practice - come to us atcourse for analysts , start January 28th. There is also an introductory course if you need to tighten up the basics.