
Recognition of numbers. How we got 97% accuracy for Ukrainian numbers. Part 2
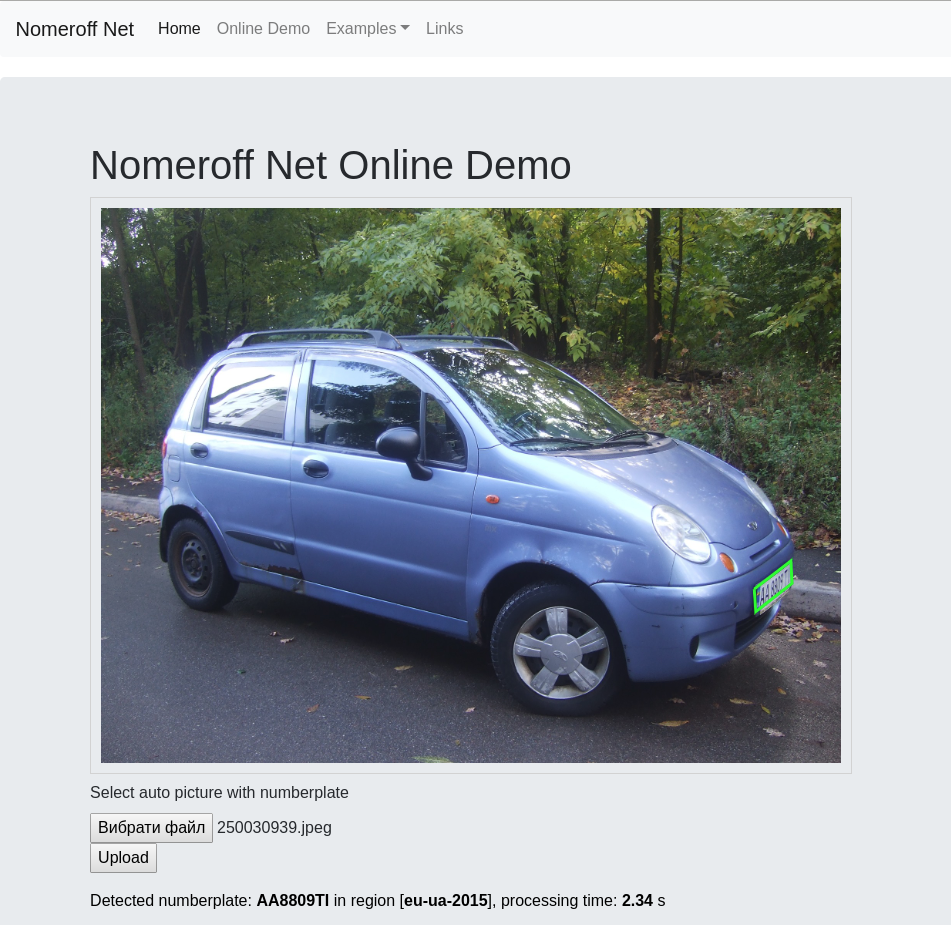
We continue the story of how to recognize license plates for those who can write the hello world application in python! In this part, we will learn how to train models that are looking for a region of a given object, and also learn how to write a simple RNN network that will cope with reading numbers better than some commercial counterparts.
In this part I will tell you how to train Nomeroff Net for your data, how to get high recognition quality, how to configure GPU support and speed up everything by an order of magnitude ...
We train Mask RCNN to find the area with the number
Of course, you can find not only a number, but any other object that you need to find. For example, you can, by analogy, look for a credit card and read its details. In general, finding the mask into which the object is inscribed in the image is called the “Instance Segmentation” task (I already wrote about this in the first part).
Now we will figure out how to train the network to solve this problem. In fact, there is little programming here, it all comes down to a monotonous, tedious, uniform data markup. Yes, yes, after you mark your first hundred you will understand what I mean :)
So, the data preparation algorithm is as follows:
- We take images of at least 300x300 in size, dump everything into one folder
- Download the VGG Image Annotator (VIA) markup tool , you can mark it up online , the output will be a directory with a photo and the json file you created with the markup. There are two such folders, in the one called train put the main part of the examples, in the second val about 20-30% of the number of examples of the first pack (Of course, these folders should not have the same photos). You can see an example of tagged data for the Nomeroff Net project . By quantity - the more the better. Some experts recommend 5,000 examples, we are lazy, typing a little more than 1,000 as the result was quite fine with us.
- To start training, you need to download the Nomeroff Net project from Github , install Mask RCNN with all the dependencies, and you can try running the train / mrcnn.ipynb training script on our data
- I immediately warn you, this does not work fast. If you do not have a GPU, this may take days. To significantly speed up the learning process, it is advisable to install tensorflow with GPU support .
- If the training on our dataset was successful, you can now safely switch to your own.
Please note - we don’t train everything from scratch, we train the model trained on COCO dataset data , which Mask RCNN downloads on first run
- You can train not coco, but our model mask_rcnn_numberplate_0700.h5 , and specify the path to this model in the WEIGHTS configuration parameter (by default, “WEIGHTS”: “coco”)
- Of the parameters that can be extended are: EPOCH, STEPS_PER_EPOCH
- The result after each era will be dumped to the folder ./logs/numberplate<date of launch> /
To test the trained model in practice, in the project examples, replace MASK_RCNN_MODEL_PATH with the path to your model.
Improving the license plate classifier to your requirements
After the areas with license plates are found, you need to try to determine which state / type of number we recognize. Here universalization works against the quality of recognition. Therefore, ideally, you need to train a classifier that not only determines which country the number is, but also the type of design of this number (location of characters, symbol options for a given type of number).
In our project, we implemented support for recognizing the numbers of Ukraine, the Russian Federation and European numbers in general. The recognition quality of European numbers is slightly worse, since there are numbers with different designs and an increased number of characters found. Perhaps, over time, there will be separate recognition modules for "eu-ee", "eu-pl", "eu-nl", ...
Before classifying a license plate, you need to “cut” it out of the image and normalize it, in other words, remove all distortions to the maximum and get a neat rectangle that will be subjected to further analysis. This task turned out to be quite non-trivial, I even had to recall school mathematics and write a specialized implementation of the k-means :) clustering algorithm :). The module that processes this is called RectDetector, this is how normalized numbers look, which we will further classify and recognize.
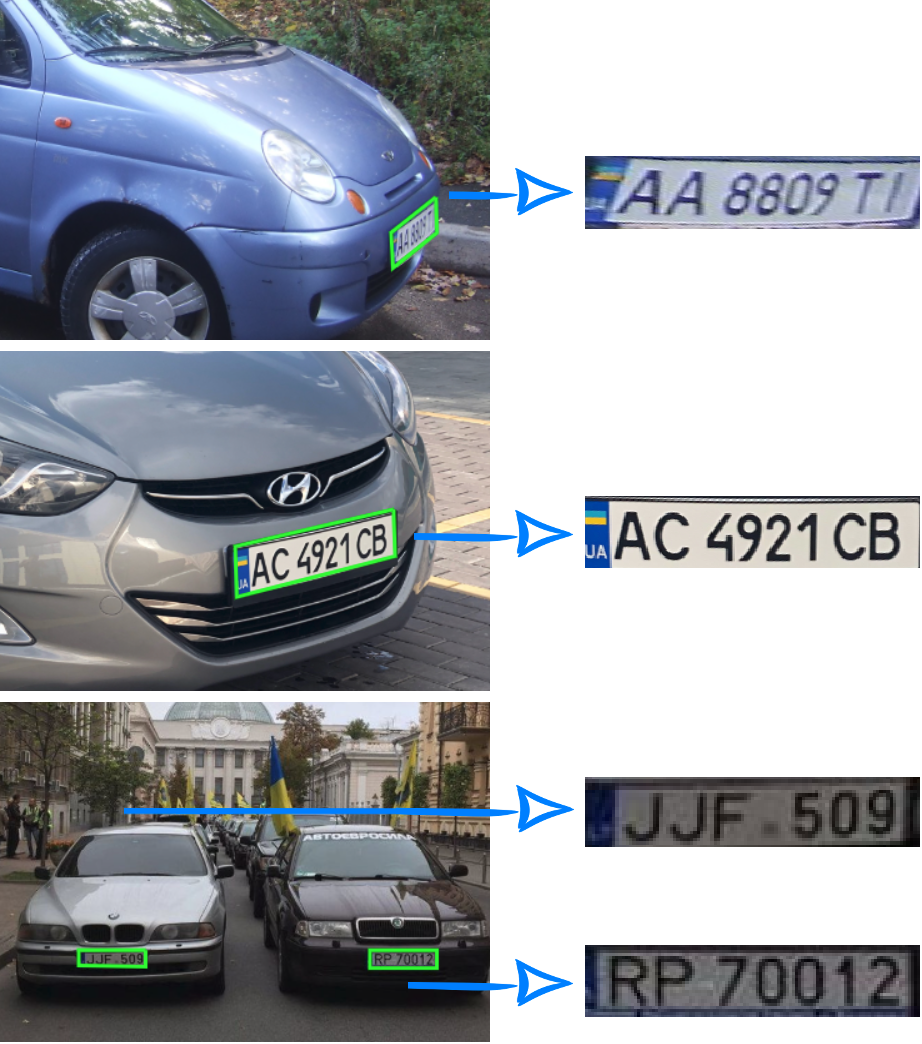
To somehow automate the process of creating a dataset for classifying numbers, we developed a small admin panel on nodejs . Using this admin panel, you can mark the inscription on the license plate and the class to which it belongs.
There can be several classifiers. In our case, by type of number and by whether it is sketched / painted over in the photo.
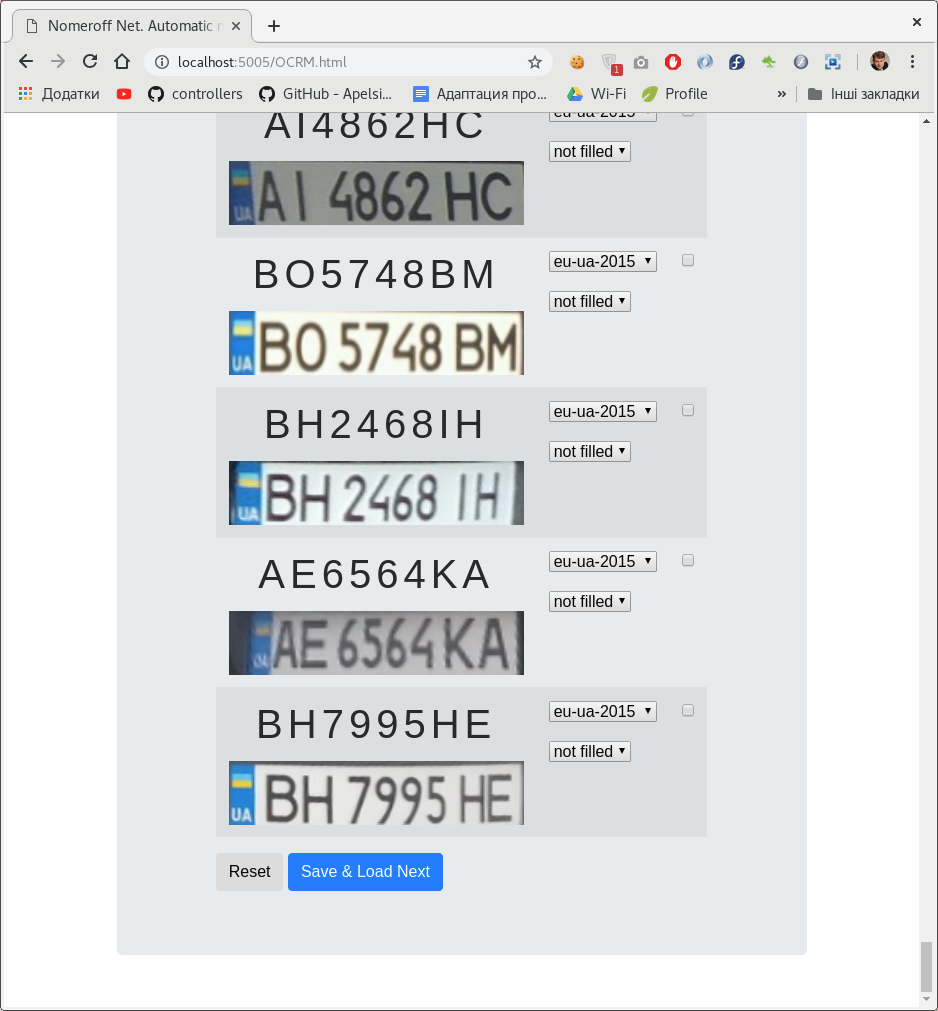
After we marked the dataset, we divide it into training, validation and test samples. As an example, download our autoriaNumberplateOptions3Dataset-2019-05-20.zip dataset to see how everything works there.
Since the selection has already been marked (moderated), then you need to change "isModerated": 1 to "isModerated" in the random json files: 0 and then start the admin panel .
We train the classifier:
The training script train / options.ipynb will help you get your version of the model. Our example shows that for the classification of regions / types of license plates, we obtained an accuracy of 98.8% , for the classification of “Is the number painted over?” 99.4% on our dataset. Agree, it turned out well.
Train your OCR (text recognition)
Well, we found the area with the number and normalized it into a rectangle that contains the inscription with the number. How do we read the text? The easiest way is to run it through FineReader or Tesseract. The quality will be “not very”, but with a good resolution of the area with the number you can get an accuracy of 80%. Actually, this is not bad accuracy, but if I tell you that you can get 97% and at the same time spend significantly less computer resources? Sounds good - let's try. A slightly unusual architecture is suitable for these purposes, in which both convolutional and recurrent layers are used. The architecture of this network looks something like this:
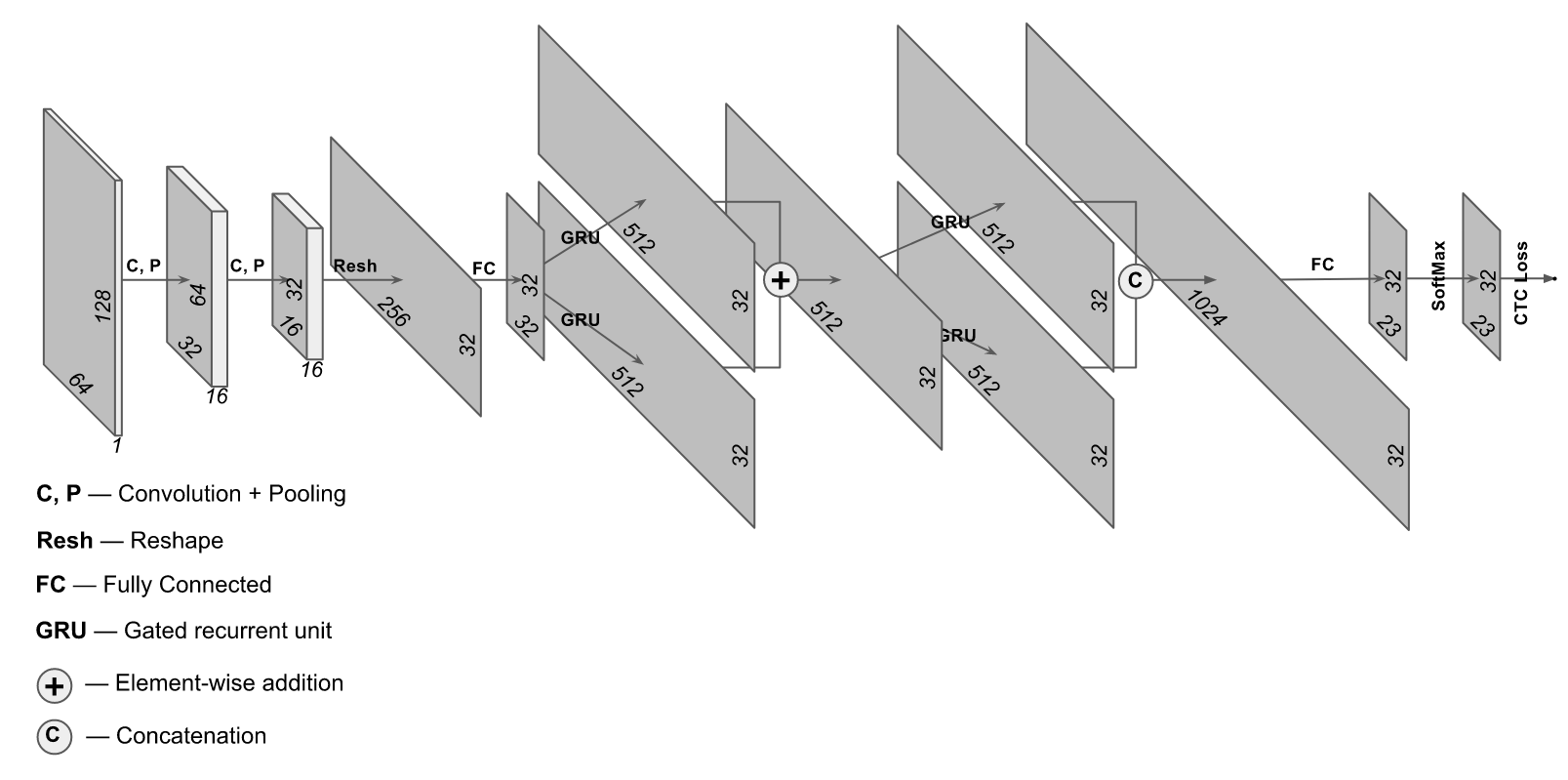
The implementation is taken from the site https://supervise.ly/, we modified it a bit for training on real photos (on the supervisely website an option for synthetic sampling was submitted)
Now the most exciting part begins, mark up at least 5,000 numbers :). We marked out about ~ 15,000 Ukrainian , ~ 6,500 European and ~ 5,000 RF . This was the most difficult part of the development. You can’t even imagine how many times I fell asleep on a computer chair moderating several hours a day for the next portion of numbers. But the real hero of the markup is dimabendera - he marked out 2/3 of all the content, (give him a plus if you understand how boring it was to do all this work :))
You can try to somehow automate this process, for example, having previously recognized each image with Tesseract, and then correct the errors using our admin panel .
Please note: the same admin panel is used to mark the classifier and OCR on the number. You can load the same data both there and there, except for the sketched numbers, of course.If you mark up at least 5000 numbers and can train your OCR - feel free to arrange a prize for yourself with your superiors, I'm sure this test is not for wimps!
Getting started training
The train / ocr-ru.ipynb script trains the model for Russian numbers, there are examples for Ukraine and Europe .
Please note that in the training settings there is only one era (one pass).
A feature of training such a dataset will be a very different result for each attempt, before each training session, the data is mixed in random order, sometimes it’s more “not very good” for training. I recommend you try at least 5 times, while controlling accuracy on test data. With different launch attempts, our accuracy could “jump” from 87% to 97% .
A few recommendations :
- No need to initialize everything in a new way, just restart the line model = ocrTextDetector.train (mode = MODE) until we get the expected result
- One reason for poor accuracy is insufficient data. If you don’t like it, we mark it up again and again, at some point the quality stops growing, for each dataset it’s different, you can focus on the number of 10,000 labeled examples
- Training will be faster if you have the NVIDIA CuDNN driver installed , change the MODE = “gpu” value in the training script and CuDNNGRU will be connected instead of the GRU layer, which will lead to three-fold acceleration.
A bit about setting up tensorflow for NVIDIA GPUs
If you are a happy owner of a GPU from NVIDIA, then you can speed things up at times: both model training and inference (recognition mode) numbers. The problem is to install and compile everything correctly.
We use Fedora Linux on our ML servers (this happened historically).
The approximate sequence of actions for those who use this OS is as follows:
- We put the GPU driver for your OS version, here for Fedora
- We connect the NVIDIA repository and install the CUDA package from there, here for CentOS / Fedora
- We put bazel, and we collect tensorflow from sources on this dock
- It is also advisable to install the old version of the gcc compiler, called cuda-gcc, everything was going fine for me on cuda-gcc 6.4. When configuring the assembly, specify the path to cuda-gcc
If you cannot build tensorflow with gpu support, you can run everything through docker, and in addition to docker, you need to install the nvidia-docker2 package. Inside the docker container, you can run jupyter notebook, and then run everything there.
jupyter notebook --ip=0.0.0.0 --port=8888 --allow-root
useful links
I also want to thank habrayuzerov 2expres , glassofkvass for providing the photo numbers and dimabendera for what he wrote most of the code and has marked most of the data Nomeroff Net project.
UPD1: Since I and Dmitri are sent to the PM standard questions on recognition of numbers, a combination of tensorflow with gpu, etc. and Dmitry and I give the same answers, I want to somehow optimize this process.
We suggest making the correspondence in the comments more structured, divided by topic. There is convenient functionality on GitHub for this. In the future, please ask questions not in the comments, but in the thematic issue on github Nomeroff Net