
Machine Learning as a New Business Analysis Tool
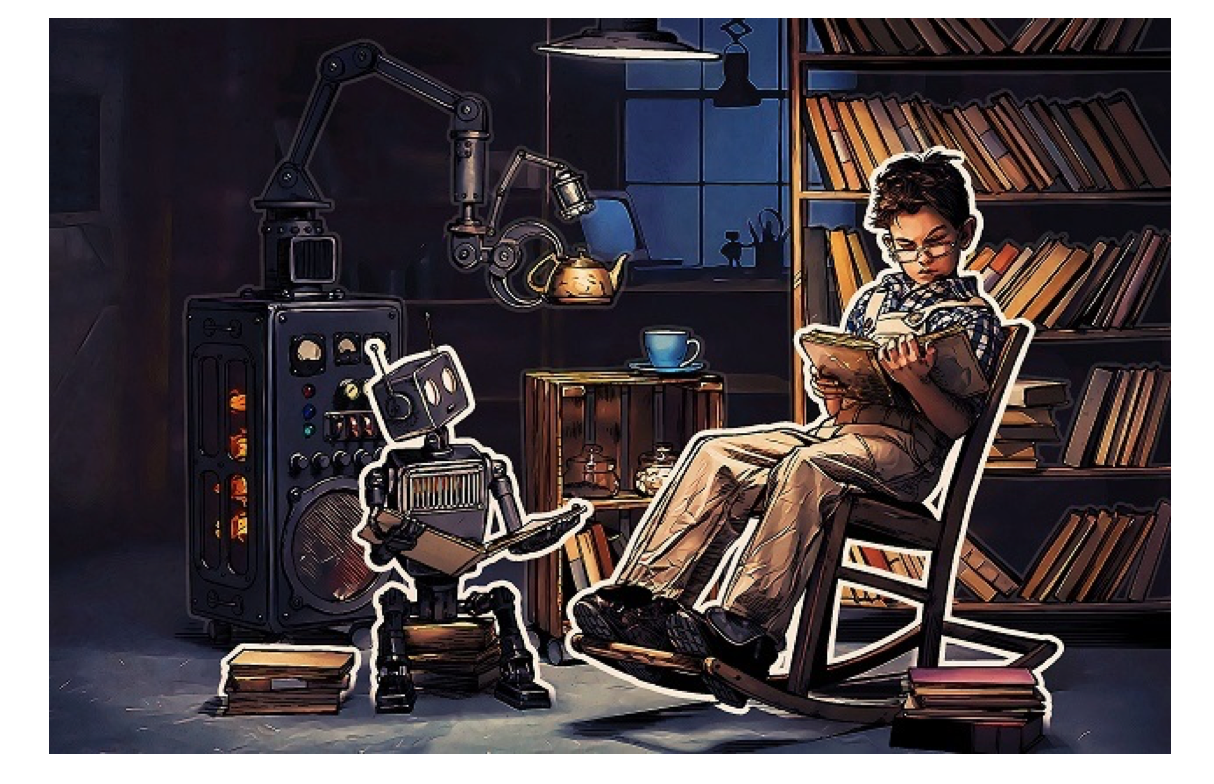
Business analysis has evolved from static reports telling what happened to interactive dashboards that help you dig deeper into data and try to understand why this happened. New sources of big data, including the Internet of things devices, are pushing businesses to move from passive analytics - when we look at a period in the past and look for trends, or check once a day for problems - to active analytics that can warn of something in advance and allowing you to create dashboards with real-time updates. This helps to make better use of operational data, which is much more useful if it was received “just now”, while conditions have not changed yet.
Many companies are interested in such active analytics, which allows you to keep abreast of the pulse of your business. But even dashboards show only what has already happened.
For this reason, various areas of in-depth analytics, including predictive, are developing most rapidly. According to a Gartner report, by 2018, in order to maintain their competitiveness, more than half of large organizations around the world will use in-depth analytics techniques (and algorithms based on them).
In-depth, predictive analytics is the calculation of trends and future opportunities, forecasting the final results and making recommendations. It goes beyond the usual queries and reports in familiar tools like SQL Server Reporting Services, Business Objects, and Tableau. It uses sophisticated statistical calculations, descriptive and predictive data mining, machine learning, simulations and optimizations. And all this for the sake of searching for signs of likely trends and patterns in the data, both structured and unstructured.
Similar tools are used today by marketers and analysts to understand the processes of outflow of customers, customer life cycles, opportunities for cross-selling, customer preferences, evaluating borrowers and identifying fraudsters. For example, many telecommunication companies today are trying to switch from passive to active analytics, in order to determine which subscribers want to switch to competitors based on user profiles and call history. Companies of almost any market segment are interested in such tools.
In addition to predicting customer behavior, there are many other areas of application for in-depth analytics. For example, the timely implementation of preventive maintenance, which implies the search for anomalies in products and the operation of services. But one of the most interesting areas is the creation of systems to support decision-making that answer the questions “what can happen?” And “what should I do?”
The main difficulty for the business remains the same: how to extract from the accumulated data some deep causal relationships or non-obvious information that allows you to perform some actions or move the business forward.
The usual way to move from data to solution is to create a static report. For example, the sales director wants to know how the company was trading in the last quarter in different regions. And in order to make further decisions, it is necessary to perform a series of procedures for compiling a report. But to make decisions, you need not only to know what happened in the last quarter, but also why it happened. Let's say sales have fallen. Because the last three deals have not burned out? Or because the average transaction volume has decreased? What do I need to do with the available statistics to figure this out?
For example, in the Cortana Analytics dashboard, you can generate all kinds of reports that reduce the number of manual data manipulations. Thanks to machine learning technologies, the system can predict a decline in sales in advance, for example, in a month, and not report it in fact. Also, automation of making recommendations and making decisions is widely used here.

Such in-depth analytics systems that use machine learning technologies to predict and support decision making should ideally inform you in advance not only of what might happen, but also what you can do about it.
For example, the system has detected that your sales forecast for the next week will not be fulfilled. At the same time, you have two marketing campaigns planned in CRM. Due to the expected recession, you have the opportunity to launch stocks a week earlier in order to support sales. You activate promotions in the system and start automated business processes so that all necessary activities start a week earlier.
Such in-depth analytics systems can be used to get answers to questions like which of the clients are most likely to leave you in the next quarter, and can also warn that a large client will be 90% likely to leave to competitors within the next 30 days.
Smart tips
Today, companies are accumulating more and more data on the basis of which business decisions can be made. And the next stage in the development of decision-making systems based on available data are automated decision support systems. That is - intelligent electronic assistants giving advice on maintaining and developing a business. But are we ready for this?
According to a recent studyconducted among 50,000 American manufacturing companies from 2005 to 2010, the number of enterprises in which business decisions were made based on data has tripled. True, this is only 30% of companies. And when in 2015, Colt telecommunications provider conducted a survey among European IT company executives, then 71% said that intuition and personal experience in making decisions work better than data analysis (although 76% said that their intuition did not always coincide with the received their data). Avanade research
, on the other handshowed that company executives generally plan to use digital assistants and automated intelligent systems to solve problems, analyze data, collaborate and make decisions. And they expect this to increase revenue by more than a third. Moreover, 54% of executives said they would be happy to work with such systems.
Pioneer companies that already use machine learning to manage customer service, financial resources, risk and compliance, both in sales and marketing, and in emerging business areas, have found “ significant, even exponential increases in benefits” in terms of costs, revenue and consumer properties. These campaigns use what is known as perceptual intelligence — a combination of speech and voice recognition, deep analytics, and business decision support.
According to the study, quick tracking of customer behavior, increasing their satisfaction by speeding up and increasing the accuracy of call processing, can reduce costs by 70% and achieve a 20-fold increase in revenue.
The involvement of business users in their construction will also facilitate the implementation of such systems. The demand for specialists in the field of data analysis exceeds the supply, which means that companies that do not have their own serious developments will turn to third-party analytical services. And experienced users (referred to in Gartner as “citizen data scientists”) will take over these tools and create their own deep analytics systems.
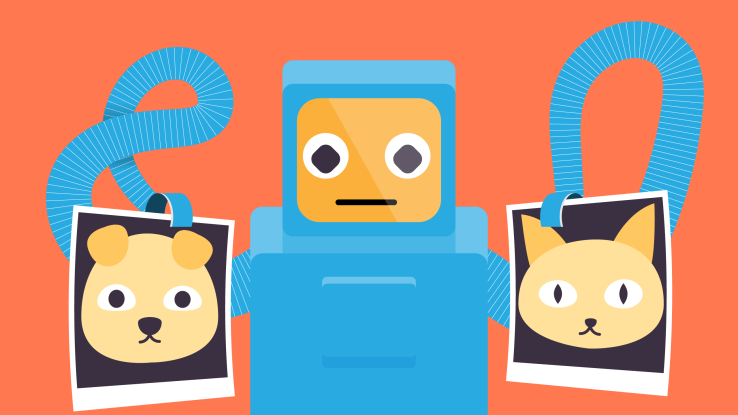