How to distinguish a good repair from a bad one, or how in SRG we made a multi-threaded Java library from Tomit Parser
This article will discuss how we integrated the Tomita-parser developed by Yandex into our system, turned it into a dynamic library, made friends with Java, made it multi-threaded and solved the problem of text classification for real estate valuation using it.
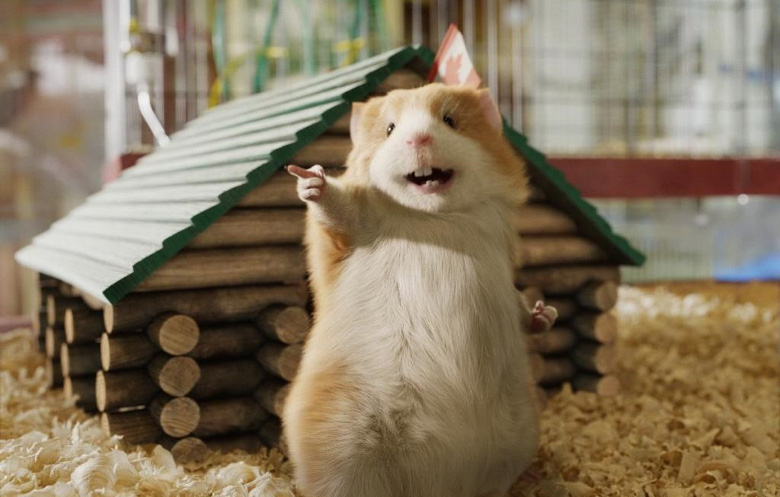
In the evaluation of real estate is of great importance analysis of ads. From the ad you can get all the necessary information about the property, including information about the state of repair in the apartment. Usually this information is contained in the ad text. It is very important in the assessment, since a good repair can add several thousand to the price per square meter.
So, we have the ad text, which must be classified into one of the categories according to the condition of the repair in the apartment (without finishing, finishing, medium, good, excellent, exclusive). One or two sentences, a couple of words or nothing can be said about the repair in the ad, so it does not make sense to classify the text completely. Due to the specificity of the text and a limited set of words relating to the context of the repair, the only sensible solution was to extract all the necessary information from the text and classify it already.
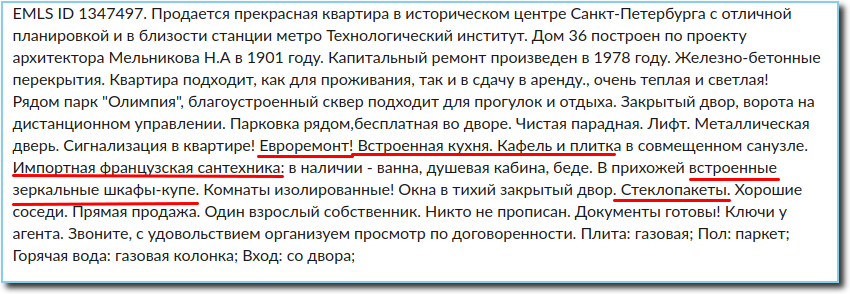
Now we must learn to extract from the text all the facts about the state of the finish. Specifically, that directly concerns the repair, as well as everything that can indirectly speak about the condition of the apartment - the presence of stretch ceilings, built-in appliances, plastic windows, a jacuzzi, the use of expensive finishing materials, etc.
In this case, it is necessary to extract only the information about the repairs in the apartment itself, because we are not interested in the condition of the entrances, basements and attics. You also need to take into account the fact that the text is written in natural language with all its inherent errors, typos, abbreviations and other features - I personally found three spellings of the words “linoleum” and “laminate” and five spellings of the word “pre-finishing”; Some people do not understand why we need spaces between words, while others have not heard about commas. Therefore, the most simple and reasonable solution was a parser with context free grammars.
Thus, as far as the decision was made, the second big and interesting task was formed - to learn how to extract all the sufficient and necessary information about the repair from the advertisement, namely, to provide a quick syntactic and morphological analysis of the text that can work in parallel under load in the library mode.
From the available tools for extracting facts from the text based on context-free grammars capable of working with the Russian language, Tomita-parser and the Yagry library on python attracted our attention. Yagry was immediately rejected because it was written entirely on python and is hardly well optimized. And Tomita initially looked very attractive: she had detailed documentation for the developer and a lot of examples, C ++ promised an acceptable speed. Understanding the rules of writing grammar was not difficult, and the first version of the classifier with its use was ready the next day.
Examples of rules from our grammars, extracting adjectives and verbs related to the context of the repair:
The rules that serve to ensure that information on the status of common areas is not retrieved:
By default, the weight of the rule is 1, assigning less weight to the rule, we set the order of their execution.
It was a little embarrassing that only a console application and a ton of C ++ code were shared. But the undoubted advantage was the ease of use and quick results on experiments. Therefore, it was decided to think about the possible difficulties of introducing it into our system closer to the implementation itself.
Almost immediately managed to achieve high-quality extraction of almost all the necessary information about the repair. “Almost”, because initially some words were not extracted under any conditions and grammars. However, it was difficult to immediately assess the scale of this problem, to what extent it can affect the quality of the solution to the classification problem as a whole.
Making sure that Tomita provides us with the necessary functionality in the first approximation, we realized that using a console application is not an option to use it: first, the console application was unstable and sometimes fell for unknown reasons, and secondly, it would not provide the necessary load of parsing several million ads per day. Thus, it became definitely exactly clear what to do with the library of it.
Our system is written in Java, tomita-parser in C ++. We needed to be able to call ads text from Java.
The development of java-bindings for Tomita-parser can be divided into two components: the implementation of the ability to use Tomits as a shared library and, in fact, the writing of an integration layer with jvm. The main difficulty concerned the first part. Tomita herself was originally designed for execution in a separate process. From this it followed that two factors were the main obstacles to the use of the parser in the application process.
After eliminating these two factors, the parser was available for use as a shared library from any environment. Write jni-bindings and java-wrapper is no longer difficult.
Tomita-parser must be configured before use. Configuration options are similar to those used when invoking the console utility. Directly parsing is to call the parse () method, which accepts parsing documents as input and returns xml as a string with the results of the parser.
The multithreaded version of Tomita - TomitaPooledParser uses for the parsing a pool of TomitaParser objects that are configured in the same way. For parsing, the first free parser is used. Since the number of generated parsers is equal to the number of threads in the pool, there will always be at least one available parser for the task. The parse method performs asynchronous parsing of the provided documents in the first free parser.
An example of calling the Tomita library from Java:
In response, an XML string with the result of parsing.
So, the library is ready, we start the service with its use on a large amount of data and remember the problem of not extracting some words, realizing that this is very critical for our task.
Among such words were “pre-final”, as well as “made”, “produced” and other reduced participles. That is, the words that occur in the ad very often, and sometimes it is the only or very important information about the repair. The reason for this behavior is that the word “pre final” turned out to be a word with an unknown morphology, that is, Tomita simply cannot determine what part of speech this is and, accordingly, cannot extract it. And for the reduced participles, we had to write a separate rule, and the problem was solved, but some time was spent to figure out that these are the reduced participles, for the extraction of which a special rule is needed. And for the long-suffering “pre-finishing” finishing, we had to write a separate rule as for a word with an unknown morphology.
In order to solve the problems of parsing with the help of grammars, add a word with an unknown morphology to the gazetter:
For abbreviated adverts, we use the grammatical characteristics of partcp, brev.
And now we can write the rules for these cases:
And the last problem we found - a service with multithreaded use of the Tomita library spawns myStem processes that are not destroyed and after a while fill the entire memory. The easiest solution was to limit the maximum and minimum number of threads in Tomcat.
So now we have repair information extracted from the text. It was easy to classify it using one of the gradient boost algorithms. We will not dwell here for a long time on this topic, a lot has already been said and written about this and we have not done anything fundamentally new in this area. I will give only the quality indicators of the classification that we obtained on the tests:
The implemented service using Tomita-parser in library mode is currently running stably, parsing and classifying several million ads per day.
All the Tomita code written by us in the framework of this project is laid out on the githab. I hope it will be useful to someone, and this person will save some time for something even more useful.
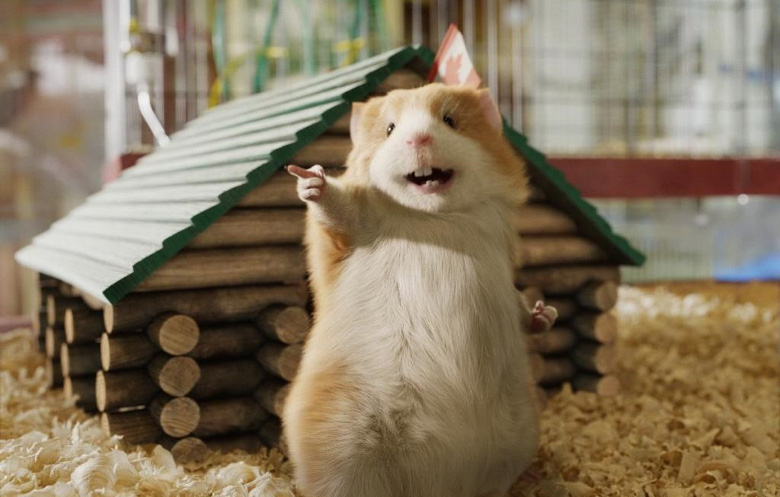
Formulation of the problem
In the evaluation of real estate is of great importance analysis of ads. From the ad you can get all the necessary information about the property, including information about the state of repair in the apartment. Usually this information is contained in the ad text. It is very important in the assessment, since a good repair can add several thousand to the price per square meter.
So, we have the ad text, which must be classified into one of the categories according to the condition of the repair in the apartment (without finishing, finishing, medium, good, excellent, exclusive). One or two sentences, a couple of words or nothing can be said about the repair in the ad, so it does not make sense to classify the text completely. Due to the specificity of the text and a limited set of words relating to the context of the repair, the only sensible solution was to extract all the necessary information from the text and classify it already.
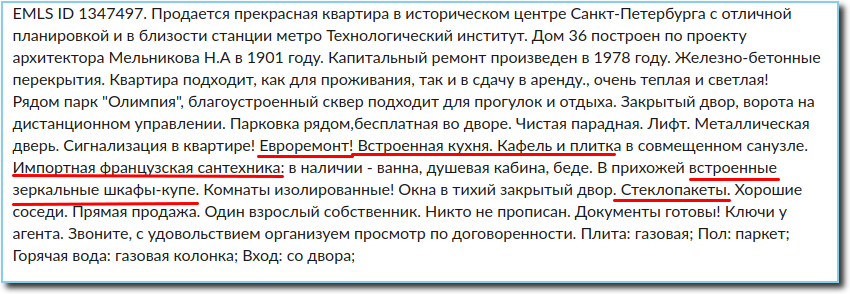
Now we must learn to extract from the text all the facts about the state of the finish. Specifically, that directly concerns the repair, as well as everything that can indirectly speak about the condition of the apartment - the presence of stretch ceilings, built-in appliances, plastic windows, a jacuzzi, the use of expensive finishing materials, etc.
In this case, it is necessary to extract only the information about the repairs in the apartment itself, because we are not interested in the condition of the entrances, basements and attics. You also need to take into account the fact that the text is written in natural language with all its inherent errors, typos, abbreviations and other features - I personally found three spellings of the words “linoleum” and “laminate” and five spellings of the word “pre-finishing”; Some people do not understand why we need spaces between words, while others have not heard about commas. Therefore, the most simple and reasonable solution was a parser with context free grammars.
Thus, as far as the decision was made, the second big and interesting task was formed - to learn how to extract all the sufficient and necessary information about the repair from the advertisement, namely, to provide a quick syntactic and morphological analysis of the text that can work in parallel under load in the library mode.
Go to the solution
From the available tools for extracting facts from the text based on context-free grammars capable of working with the Russian language, Tomita-parser and the Yagry library on python attracted our attention. Yagry was immediately rejected because it was written entirely on python and is hardly well optimized. And Tomita initially looked very attractive: she had detailed documentation for the developer and a lot of examples, C ++ promised an acceptable speed. Understanding the rules of writing grammar was not difficult, and the first version of the classifier with its use was ready the next day.
Examples of rules from our grammars, extracting adjectives and verbs related to the context of the repair:
RepairW -> "ремонт" | "состояние" | "отделка";
StopWords -> "подъезд" | "холл" | "фасад" | "вестибюль";
Repair -> RepairW<gnc-agr[1]> Adj<gnc-agr[1]>+ interp (Repair.AdjGroup {weight = 0.5});
Repair -> Verb<gnc-agr[1]> Adj<gnc-agr[1]>* interp (Repair.Verb) RepairW<gnc-agr[1]> {weight = 0.5};
The rules that serve to ensure that information on the status of common areas is not retrieved:
Repair -> StopWords Verb* Prep* Adj* RepairW;
Repair -> Adj+ RepairW Prep* StopWords;
By default, the weight of the rule is 1, assigning less weight to the rule, we set the order of their execution.
It was a little embarrassing that only a console application and a ton of C ++ code were shared. But the undoubted advantage was the ease of use and quick results on experiments. Therefore, it was decided to think about the possible difficulties of introducing it into our system closer to the implementation itself.
Almost immediately managed to achieve high-quality extraction of almost all the necessary information about the repair. “Almost”, because initially some words were not extracted under any conditions and grammars. However, it was difficult to immediately assess the scale of this problem, to what extent it can affect the quality of the solution to the classification problem as a whole.
Making sure that Tomita provides us with the necessary functionality in the first approximation, we realized that using a console application is not an option to use it: first, the console application was unstable and sometimes fell for unknown reasons, and secondly, it would not provide the necessary load of parsing several million ads per day. Thus, it became definitely exactly clear what to do with the library of it.
How we made Tomita a multi-threaded library and made friends with Java
Our system is written in Java, tomita-parser in C ++. We needed to be able to call ads text from Java.
The development of java-bindings for Tomita-parser can be divided into two components: the implementation of the ability to use Tomits as a shared library and, in fact, the writing of an integration layer with jvm. The main difficulty concerned the first part. Tomita herself was originally designed for execution in a separate process. From this it followed that two factors were the main obstacles to the use of the parser in the application process.
- Data exchange was carried out through various kinds of IO. It was necessary to implement the ability to communicate with the parser through memory. And it was necessary to do this in such a way as to minimally affect the code of the parser itself. Tomita's architecture suggested a way to implement reading of input documents from memory as the implementation of CDocStreamBase and CDocListRetrieverBase interfaces. With the output it was more difficult - I had to touch the code of the xml generator.
- The second factor arising from the principle “one parser - one process” is a global state modified from different instances of the parser. If you look at the src / util / generic / singleton.h file , you see the mechanism for using the shared state. It is easy to imagine that when using two instances of the parser in the same address space, there will be a race condition. In order not to rewrite the entire parser, it was decided to modify this class, replacing the global state with a local state relative to the thread (thread_local). Accordingly, before any parser call in the JTextMiner wrapper, we set these thread_local variables to the current instance of the parser, after which the parser code works with the addresses of the current instance of the parser.
After eliminating these two factors, the parser was available for use as a shared library from any environment. Write jni-bindings and java-wrapper is no longer difficult.
Tomita-parser must be configured before use. Configuration options are similar to those used when invoking the console utility. Directly parsing is to call the parse () method, which accepts parsing documents as input and returns xml as a string with the results of the parser.
The multithreaded version of Tomita - TomitaPooledParser uses for the parsing a pool of TomitaParser objects that are configured in the same way. For parsing, the first free parser is used. Since the number of generated parsers is equal to the number of threads in the pool, there will always be at least one available parser for the task. The parse method performs asynchronous parsing of the provided documents in the first free parser.
An example of calling the Tomita library from Java:
/**
* @param threadAmount number of threads in the pool
* @param tomitaConfigFilename tomita config.proto
* @param configDirname dir with configs: grammars, gazetteer, facttypes.proto
*/
tomitaPooledParser = new TomitaPooledParser(threadAmount, new File(configDirname), new String[]{tomitaConfigFilename});
Future<String> result = tomitaPooledParser.parse(documents);
String response = result.get();
In response, an XML string with the result of parsing.
The problems we faced and how we solved them
So, the library is ready, we start the service with its use on a large amount of data and remember the problem of not extracting some words, realizing that this is very critical for our task.
Among such words were “pre-final”, as well as “made”, “produced” and other reduced participles. That is, the words that occur in the ad very often, and sometimes it is the only or very important information about the repair. The reason for this behavior is that the word “pre final” turned out to be a word with an unknown morphology, that is, Tomita simply cannot determine what part of speech this is and, accordingly, cannot extract it. And for the reduced participles, we had to write a separate rule, and the problem was solved, but some time was spent to figure out that these are the reduced participles, for the extraction of which a special rule is needed. And for the long-suffering “pre-finishing” finishing, we had to write a separate rule as for a word with an unknown morphology.
In order to solve the problems of parsing with the help of grammars, add a word with an unknown morphology to the gazetter:
TAuxDicArticle "adjNonExtracted"
{
key = "предчистовая" | "пред-чистовая"
}
For abbreviated adverts, we use the grammatical characteristics of partcp, brev.
And now we can write the rules for these cases:
Repair -> RepairW<gnc-agr[1]> Word<gram="partcp,brev",gnc-agr[1]> interp (Repair.AdjGroup) {weight = 0.5};
Repair -> Word<kwtype="adjNonExtracted",gnc-agr[1]> interp (Repair.AdjGroup) RepairW<gnc-agr[1]> Prep* Adj<gnc-agr[1]>+;
And the last problem we found - a service with multithreaded use of the Tomita library spawns myStem processes that are not destroyed and after a while fill the entire memory. The easiest solution was to limit the maximum and minimum number of threads in Tomcat.
A couple of words about the classification
So now we have repair information extracted from the text. It was easy to classify it using one of the gradient boost algorithms. We will not dwell here for a long time on this topic, a lot has already been said and written about this and we have not done anything fundamentally new in this area. I will give only the quality indicators of the classification that we obtained on the tests:
- Accuracy = 95%
- F1 score = 93%
Conclusion
The implemented service using Tomita-parser in library mode is currently running stably, parsing and classifying several million ads per day.
PS
All the Tomita code written by us in the framework of this project is laid out on the githab. I hope it will be useful to someone, and this person will save some time for something even more useful.